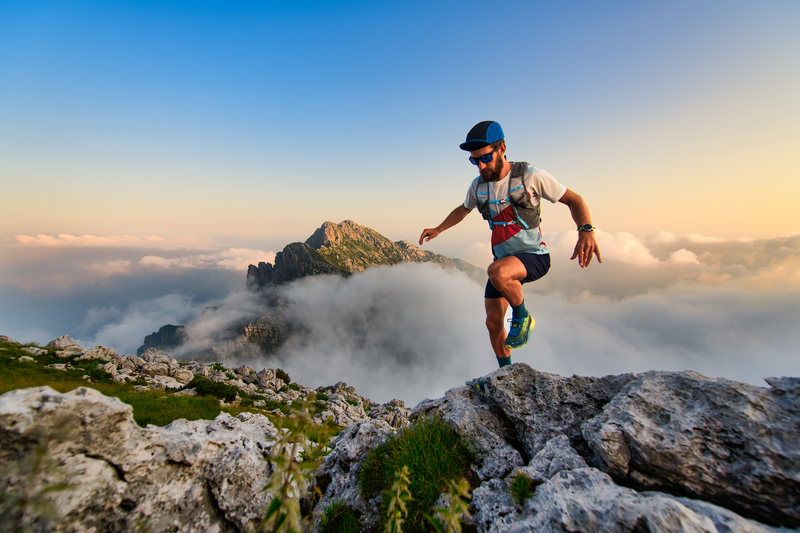
94% of researchers rate our articles as excellent or good
Learn more about the work of our research integrity team to safeguard the quality of each article we publish.
Find out more
ORIGINAL RESEARCH article
Front. Neurosci.
Sec. Translational Neuroscience
Volume 19 - 2025 | doi: 10.3389/fnins.2025.1558069
The final, formatted version of the article will be published soon.
You have multiple emails registered with Frontiers:
Please enter your email address:
If you already have an account, please login
You don't have a Frontiers account ? You can register here
The aim of this study was to investigate the characteristics of brain activity changes in patients with post-stroke balance dysfunction and their relationship with clinical assessment, and to construct a classification model based on the extreme Gradient Boosting (XGBoost) algorithm to discriminate between stroke patients and healthy controls (HCs).In the current study, twenty-six patients with post-stroke balance dysfunction and twentyfour HCs were examined by resting-state functional magnetic resonance imaging (rs-fMRI). Static amplitude of low frequency fluctuation (sALFF), static fractional ALFF (sfALFF), static regional homogeneity (sReHo), dynamic ALFF (dALFF), dynamic fALFF (dfALFF) and dynamic ReHo (dReHo) values were calculated and compared between the two groups. The values of the imaging metrics for the brain regions with significant differences were used in Pearson correlation analyses with the Berg Balance Scale (BBS) scores and as features in the construction of the XGBoost model.Results: Compared to HCs, the brain regions with significant functional abnormalities in patients with post-stroke balance dysfunction were mainly involved bilateral insula, right fusiform gyrus, right lingual gyrus, left thalamus, left inferior occipital gyrus, left inferior temporal gyrus, right calcarine fissure and surrounding cortex, left precuneus, right median cingulate and paracingulate gyri, right anterior cingulate and paracingulate gyri, bilateral supplementary motor area, right putamen, and left cerebellar crus II. XGBoost results show that the model constructed based on static imaging features has the best classification prediction performance.In conclusion, this study provided evidence of functional abnormalities in local brain regions in patients with post-stroke balance dysfunction. The results suggested that the abnormal brain regions were mainly related to visual processing, motor execution, motor coordination, sensorimotor control and cognitive function, which contributed to our understanding of the neuropathological mechanisms of post-stroke balance dysfunction. XGBoost is a promising machine learning method to explore these changes.
Keywords: resting-state functional magnetic resonance imaging, Stroke, balance dysfunction, amplitude of low frequency fluctuation, fractional amplitude of low frequency fluctuation, regional homogeneity, Extreme gradient boosting
Received: 09 Jan 2025; Accepted: 10 Mar 2025.
Copyright: © 2025 Tang, Liu, Long, Ren, Liu, Li, Han, Liao, Zhang, Lu and Zhang. This is an open-access article distributed under the terms of the Creative Commons Attribution License (CC BY). The use, distribution or reproduction in other forums is permitted, provided the original author(s) or licensor are credited and that the original publication in this journal is cited, in accordance with accepted academic practice. No use, distribution or reproduction is permitted which does not comply with these terms.
* Correspondence:
Haitao Lu, Capital Medical University, Beijing, China
Hao Zhang, Capital Medical University, Beijing, China
Disclaimer: All claims expressed in this article are solely those of the authors and do not necessarily represent those of their affiliated organizations, or those of the publisher, the editors and the reviewers. Any product that may be evaluated in this article or claim that may be made by its manufacturer is not guaranteed or endorsed by the publisher.
Research integrity at Frontiers
Learn more about the work of our research integrity team to safeguard the quality of each article we publish.