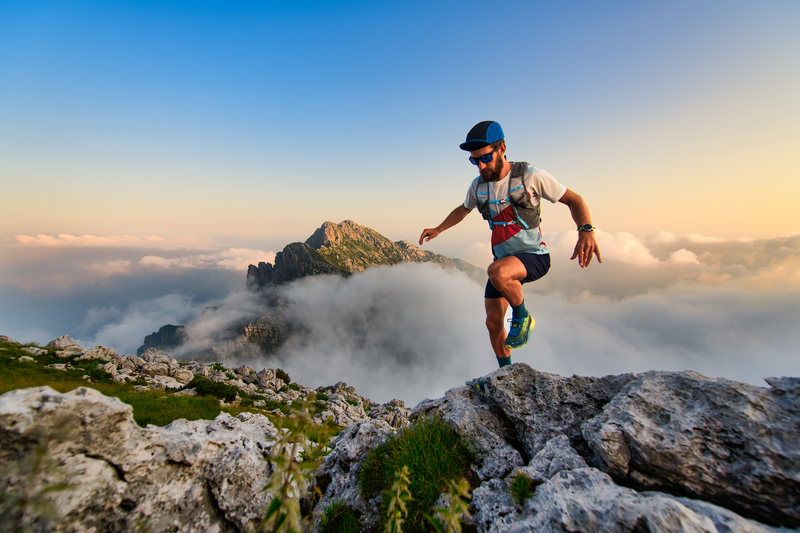
94% of researchers rate our articles as excellent or good
Learn more about the work of our research integrity team to safeguard the quality of each article we publish.
Find out more
ORIGINAL RESEARCH article
Front. Neurosci.
Sec. Translational Neuroscience
Volume 19 - 2025 | doi: 10.3389/fnins.2025.1554156
This article is part of the Research Topic Music and Medicine: From Basic Science to Clinical Practice View all 3 articles
The final, formatted version of the article will be published soon.
You have multiple emails registered with Frontiers:
Please enter your email address:
If you already have an account, please login
You don't have a Frontiers account ? You can register here
Anxiety and depression reduce autonomic system activity measured by Heart Rate Variability (HRV) and worsen cardiac morbidity. Music and mindfulness can increase HRV and clinical approaches that use music or mindfulness show promise as efficacious treatments for symptoms of anxiety and depression. Music mindfulness combines music listening with mindfulness activities and may have unique and synergistic therapeutic effects for stress management. However, no studies have evaluated the physiological mechanisms underlying a community-based music mindfulness paradigm. We found that music mindfulness sessions acutely enhanced multiple measures of Heart Rate Variability (HRV) and altered EEG power spectral density across multiple frequency bands in frontotemporal electrodes. Both live and virtual music mindfulness sessions also acutely decreased stress and altered participants’ state of consciousness, but only live sessions increased social connection. The physiological and psychological impact of music mindfulness was also different based on sex as self-reported by participants. Overall, we demonstrate that music mindfulness effectively targets autonomic and frontotemporal neural mechanisms that may treat symptoms of anxiety and depression.
Keywords: Music, mindfulness, Heart rate variability, Electroencephalography, Depression, Anxiety, stress
Received: 01 Jan 2025; Accepted: 10 Mar 2025.
Copyright: © 2025 Ramirez, Alayine, Akafia, Adichi, Watts, Galdamez, Harding and Allsop. This is an open-access article distributed under the terms of the Creative Commons Attribution License (CC BY). The use, distribution or reproduction in other forums is permitted, provided the original author(s) or licensor are credited and that the original publication in this journal is cited, in accordance with accepted academic practice. No use, distribution or reproduction is permitted which does not comply with these terms.
* Correspondence:
AZA Stephen Allsop, Yale University, New Haven, United States
Disclaimer: All claims expressed in this article are solely those of the authors and do not necessarily represent those of their affiliated organizations, or those of the publisher, the editors and the reviewers. Any product that may be evaluated in this article or claim that may be made by its manufacturer is not guaranteed or endorsed by the publisher.
Research integrity at Frontiers
Learn more about the work of our research integrity team to safeguard the quality of each article we publish.