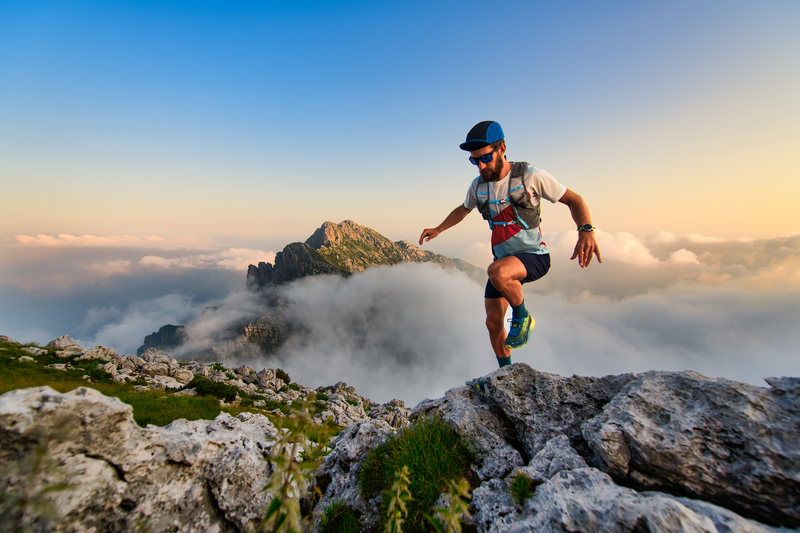
94% of researchers rate our articles as excellent or good
Learn more about the work of our research integrity team to safeguard the quality of each article we publish.
Find out more
ORIGINAL RESEARCH article
Front. Neurosci.
Sec. Brain Imaging Methods
Volume 19 - 2025 | doi: 10.3389/fnins.2025.1554015
This article is part of the Research Topic Advancing Early Alzheimer's Detection Through Multimodal Neuroimaging Techniques View all 5 articles
The final, formatted version of the article will be published soon.
You have multiple emails registered with Frontiers:
Please enter your email address:
If you already have an account, please login
You don't have a Frontiers account ? You can register here
Alzheimer's Disease (AD) is a progressive neurodegenerative disorder and the leading cause of dementia worldwide, affecting over 55 million people globally, with numbers expected to rise dramatically. Early detection and classification of AD are crucial for improving patient outcomes and slowing disease progression. However, conventional diagnostic approaches often fail to provide accurate classification in the early stages. This paper proposes a novel approach using advanced computer-aided diagnostic (CAD) systems and the YOLOv11 neural network for early detection and classification of AD. The YOLOv11 model leverages its advanced object detection capabilities to simultaneously localize and classify AD-related biomarkers by integrating multimodal data fusion of T2-weighted MRI and DTI images from the Alzheimer's Disease Neuroimaging Initiative (ADNI) database. Regions of interest (ROIs) were selected and annotated based on known AD biomarkers, and the YOLOv11 model was trained to classify AD into four stages: Cognitively Normal (CN), Early Mild Cognitive Impairment (EMCI), Late Mild Cognitive Impairment (LMCI), and Mild Cognitive Impairment (MCI). The model achieved exceptional performance, with 93.6% precision, 91.6% recall, and 96.7% mAP50, demonstrating its ability to identify subtle biomarkers by combining MRI and DTI modalities. This work highlights the novelty of using YOLOv11 for simultaneous detection and classification, offering a promising strategy for early-stage AD diagnosis and classification.
Keywords: Alzheimer's disease, YOLOv11, Early detection, four stages, Classification, Precision
Received: 03 Jan 2025; Accepted: 24 Feb 2025.
Copyright: © 2025 Hechkel and Helali. This is an open-access article distributed under the terms of the Creative Commons Attribution License (CC BY). The use, distribution or reproduction in other forums is permitted, provided the original author(s) or licensor are credited and that the original publication in this journal is cited, in accordance with accepted academic practice. No use, distribution or reproduction is permitted which does not comply with these terms.
* Correspondence:
Wided Hechkel, Faculte des Sciences de Monastir (FSM), Monastir, Tunisia
Disclaimer: All claims expressed in this article are solely those of the authors and do not necessarily represent those of their affiliated organizations, or those of the publisher, the editors and the reviewers. Any product that may be evaluated in this article or claim that may be made by its manufacturer is not guaranteed or endorsed by the publisher.
Research integrity at Frontiers
Learn more about the work of our research integrity team to safeguard the quality of each article we publish.