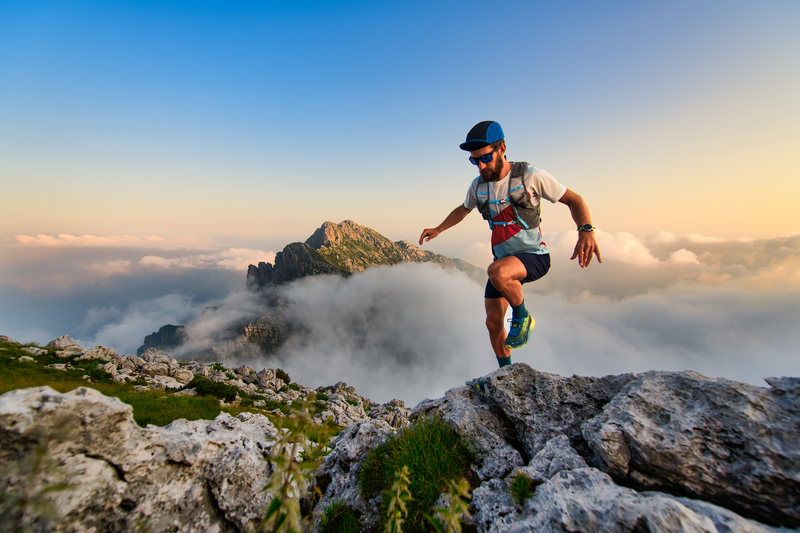
95% of researchers rate our articles as excellent or good
Learn more about the work of our research integrity team to safeguard the quality of each article we publish.
Find out more
ORIGINAL RESEARCH article
Front. Neurosci. , 20 March 2025
Sec. Brain Imaging Methods
Volume 19 - 2025 | https://doi.org/10.3389/fnins.2025.1546236
Background: Detecting multiple sclerosis (MS) relapses remains challenging due to symptom variability and confounding factors, such as flare-ups and infections. Methylprednisolone (MP) is used for severe relapses, decreasing the number of contrast-enhancing lesions on MRI. The influx constant (Ki) derived from dynamic contrast-enhanced MRI (DCE-MRI), a marker of blood–brain barrier (BBB) permeability, has shown promise as a predictor of disease activity in relapsing–remitting MS (RRMS).
Objectives: To investigate the predictive value of Ki in relation to clinical MS relapses and MP treatment, comparing its performance with traditional MRI markers.
Methods: We studied 20 RRMS subjects admitted for possible relapse, using DCE-MRI on admission to assess Ki in normal-appearing white matter (NAWM) via the Patlak model. Mixed-effects modeling compared the predictive accuracy of Ki, the presence of contrast-enhancing lesions (CEL), evidence of brain lesions (EBL; defined as the presence of CEL or new T2 lesions), and MP treatment on clinical relapse events. Five models were evaluated, including combinations of Ki, CEL, EBL, and MP, to determine the most robust predictors of clinical relapse. Model performance was assessed using accuracy, sensitivity, specificity, positive predictive value (PPV), and negative predictive value (NPV), with bootstrapped confidence intervals.
Results: Superior predictive accuracy was demonstrated with the inclusion of EBL and Ki, alongside MP treatment (AIC = 66.12, p = 0.006), outperforming other models with a classification accuracy of 83% (CI: 73–92%), sensitivity of 78% (CI: 60–94%), and specificity of 86% (CI: 74–97%). This model showed the highest combined PPV (78%, CI: 60–94%) and NPV (86%, CI: 74–98%) compared to models with EBL or CEL alone, suggesting an added value of Ki in enhancing predictive reliability.
Conclusion: These results support the use of Ki alongside conventional MRI imaging metrics, to improve clinical relapse prediction in RRMS. The findings underscore the utility of Ki as a marker of MS-related neuroinflammation, with potential for integration into relapse monitoring protocols. Further validation in larger cohorts is recommended to confirm the model’s generalizability and clinical application.
Multiple sclerosis (MS) is a chronic autoimmune disorder of the central nervous system characterized by inflammation, demyelination, and neurodegeneration. Relapses are the hallmark of the relapsing–remitting form (RRMS), and their detection is central to diagnosis and management. However, detecting clinical relapses can be challenging due to the wide range of presentations and confounding factors. One such factor is Uthoff’s phenomenon, which describes transient neurological symptoms triggered by factors such as heat exposure, overexertion, or simple infections (Thrower, 2009). While systemic infections may cause pseudo-relapses, evidence also suggests an association with true relapse activity (Correale et al., 2006; Thrower, 2009) and disease progression (Stuart et al., 2024), further complicating the identification of genuine relapses. Furthermore, early initiation of efficacious disease modifying treatment has been shown to improve long-term outcome in MS patients (Cobo-Calvo et al., 2023). In this context, accurate and timely identification of relapses is crucial for initiating appropriate treatment and monitoring disease activity and progression.
Methylprednisolone (MP), a synthetic glucocorticoid, is commonly used for symptomatic treatment of severe relapses (Myhr and Mellgren, 2009; Smets et al., 2017) and has been shown to reduce inflammation and the number of contrast-enhancing lesions on T1-weighted magnetic resonance imaging (MRI) scans (Sellebjerg et al., 2003). However, the impact of MP treatment on long-term clinical outcomes remains limited (Hommes et al., 1996; Myhr and Mellgren, 2009; Smets et al., 2017).
A recent retrospective study of 199 MS patients with acute relapse activity, investigated the correlation between symptoms of a clinical MS relapse and the anatomical location of new lesional activity, finding a clinical-radiological mismatch in 43% of cases. In these cases, the symptoms did not correspond to new MRI lesional activity in the relevant anatomical brain region. Furthermore, in 33% of all cases, no new MRI lesional activity could be identified on brain or spinal cord MRI (Dünschede et al., 2023). This clinico-radiological paradox emphasizes the need for biomarkers capable of detecting clinical disease activity (Galea et al., 2015), particularly to guide decisions regarding symptomatic MP treatment of acute relapses and the escalation of disease-modifying therapies (DMT). While serum-derived biomarkers—especially neurofilament light chain - have shown considerable promise in monitoring disease activity and treatment response, findings on their ability to predict relapses remain conflicting (Højsgaard Chow et al., 2024). Therefore, imaging-derived biomarkers may still hold potential for future benefit.
Dynamic contrast-enhanced magnetic resonance imaging (DCE-MRI) is a non-invasive imaging technique that allows the measurement of blood–brain barrier (BBB) permeability (Larsson et al., 1990; Sourbron et al., 2009). The influx constant, Ki, derived from DCE-MRI data, reflects the rate at which the contrast agent leaks into the brain parenchyma and can serve as an imaging marker of subtle changes in BBB integrity (Larsson et al., 2009). Previous studies have demonstrated the predictive capabilities of Ki in the context of conversion from optic neuritis to MS (Cramer et al., 2015) and in monitoring treatment effects in the normal-appearing white matter (NAWM) away from MS lesions (Cramer et al., 2018; Knudsen et al., 2022).
Given the potential of Ki as a sensitive marker of neuroinflammation, the present study aimed to investigate the impact of acute MS relapses and MP treatment on Ki. By prospectively analyzing a cohort of patients with relapsing–remitting MS (RRMS) suspected of acute relapse activity, we aim to elucidate the development of Ki in individual subjects, during relapse and MP treatment response. Additionally, we aimed to explore the relationship between Ki and conventional MRI markers, such as MRI contrast-enhancing lesions and new T2 lesions.
Twenty patients with established relapsing remitting MS (RRMS) were referred from the Department of Neurology Rigshospitalet, Glostrup, suspected of a MS relapse. The inclusion criteria were an established diagnosis of RRMS, clinical suspicion of relapse and willingness to participate in three consecutive scanning sessions. All subjects referred to the study were included. MRI scans were performed at time of study inclusion, again after 2 weeks, and the final scan was performed as a post-relapse scan after 3–4 months. For study timeline overview see Figure 1.
Figure 1. Study timeline. Twenty subjects with RRMS suspected of an acute MS relapse were included in the study and MRI scanned at baseline, and 2 weeks after MP treatment (when relevant from a clinical perspective) and again 3 months post-relapse. MP, Methylprednisolone.
Clinical MS relapse was defined as the development of a new symptom, or worsening of a pre-existing symptom, confirmed on neurologic examination, lasting at least 48 h, and preceded by stability or improvement lasting at least 30 days. Neurologic deterioration only temporarily associated with a period of fever was not considered a relapse (Schumacker et al., 1965). Relapses were categorized according to severity into major and minor relapses. Major relapses had an increase of >1 point on the Expanded Disability Status Scale (EDSS) (>0.5 points above EDSS 5.5) since the preceding visit. A minor relapse was defined as an exacerbation with an increase of >1 point in any of the functional system sub scores but not leading to a change in EDSS (Buljevac et al., 2002). Infections were defined as the presence of coryza, sore throat, flu-like symptoms, myalgia, fever, urinary infection, or diarrhea lasting more than 24 h. Urinary infections were defined as the presence of fever, chills, dysuria, increased need to void, suprapubic pain, and pathologic urine analysis or positive culture. Additionally, separate relapse activity occurring in proximity to any follow-up scan was defined as a new relapse event potentially influencing the outcome measures if occurring +/− 2 months before or after the scan date. MP treatment consisted of an oral or intravenous dose of 1 gram of methylprednisolone given once per day for three consecutive days (no tapering), initiated immediately after the baseline scan. Relapse classification and MP treatment decisions were made independently of the study by an experienced MS specialist consultant neurologist, who was blinded to the results of the DCE scan.
This study was approved by the Ethics Committee of Copenhagen County according to the standards of The National Committee on Health Research Ethics, protocol number H-D-2008-002. All experiments were conducted in accordance with the Helsinki Declaration of 1975 and all subjects gave written informed consent.
MRI was performed on a 3T MR unit (Philips Achieva) using a 32-element phased-array head coil. DCE-MRI used a T1-weighted saturation-recovery gradient-echo sequence with flip angle 30°, repetition time = 3.9 ms, echo time = 1.9 ms, centric phase ordering, parallel imaging factor 2, acquired matrix 96 × 61, acquired voxel-size 2.40 × 2.98 × 8 mm3 (interpolated to 0.90 × 0.89 × 8 mm3), field of view 230 × 182 mm2, five slices, slice thickness 8 mm (Supplementary Figure 1). Data for an initial measurement of relaxation time (T1) and equilibrium magnetization (M0) were generated using a series of saturation time delays from 120 ms to 10 s, covering the same slices as imaged during the bolus passage. The dynamic sequence used a saturation time delay of 120 ms, giving a time resolution of 1.25 s, and 750 time points, corresponding to a total sampling duration of 15.7 min. The dose of contrast agent (Gadobutrol 1 mmoL/mL) was 0.09 mmol/kg bodyweight, injected automatically (Spectris, Medrad; USA) with a speed of 3 mL/s followed by 20 mL saline, injected as two boli of each 0.045 mmol/kg bodyweight at time points 15 and 80, corresponding to 18.8 and 100 s. We acquired a separate slice at the level of the internal carotid artery (ICA) to obtain an arterial input function with minimal partial volume for every single subject. The remaining four DCE slices were used for defining regions of interest and subsequent estimation of tissue pharmacokinetic values.
We used an axial T2-weighted MRI sequence [five slices, echo time = 100 ms, repetition time = 3000 ms, acquired voxel-size 0.57 × 0.76 × 8 mm3 (interpolated to 0.45 × 0.45 × 8 mm3), field of view = 230 × 119 mm2] with same orientation and slice thickness (8 mm) as our DCE-MRI sequence, in order to manually draw regions of interest in the frontal white matter, and the thalamic grey matter in both hemispheres. Four regions of interest (ROIs) were placed in frontal white matter (two in the vicinity of the frontal ventricular horns, one in each hemisphere and two in the vicinity of the posterior horn, one in each hemisphere). Two ROIs were placed in the right and left thalamus. The DCE slices and ROIs were placed with the same angulation and anatomical position on consecutive scans (Supplementary Figure 1). We ensured consistent positioning and size of our ROIs across study time points by visual alignment with the previous scan.
The DCE MRI data was analysed with a semi-automated procedure (Larsson et al., 2008, 2009) using in-house Matlab-based software. The DCE time series was converted to units of contrast agent concentration using T1 and M0, as determined from the multiple saturation delay data, and a contrast agent relaxivity of 4 s−1 mM−1. The input function was measured in the voxel of the internal carotid artery with maximal signal change during the bolus passage and was corrected for partial volume by normalizing to a magnitude-and phase-derived venous outflow function, sampled in the sagittal sinus (Cramer et al., 2022). The mean signal-time curve for all voxels in the ROI was extracted and used to calculate permeability. Every subject was represented by one Ki, CBV and CBF value, calculated as the mean of the tissue specific ROIs, as previously described (Cramer and Larsson, 2014; Cramer et al., 2014, 2015, 2022). Tissue concentration-time curves were evaluated using a combination of model-free deconvolution, yielding CBF, and a Patlak model, yielding CBV and Ki, as described in previous work (Larsson et al., 2009). Permeability values, measured as Ki (full blood) relates to Ktrans (plasma) by Ki = Ktrans/(1-Hct). A fixed value of Hct = 0.45 was used throughout the study. Values of Ki are reported as ml/100 g/min assuming brain tissue density of 1 g/mL (Barber et al., 1970).
Histograms, probability plots and modified Kolmogorov–Smirnov (Lillefors) testing were used to analyze continuous variables for standard normal distribution fit (Lilliefors, 1967; Dallal and Wilkinson, 1986). For comparisons between continuous variables Wilcoxon signed-rank tests were performed and for categorical data Chi square tests were performed. For comparisons between more than two groups, ANOVA testing was used. To predict clinical relapse (binary variable; y/n), we evaluated the predictive accuracy of five mixed-effects models, each incorporating combinations of the following predictors: (1) Presence of contrast-enhancing lesions (CEL): A binary variable indicating the presence or absence of contrast-enhancing lesions. (2) “Evidence of brain lesion” (EBL), defined as either the presence of either CEL or new T2 lesions(s) when compared to a previous MRI. (3) Ki in normal-appearing white matter. (4) MP use (y/n) was added as a confounder to account for treatment effects, given its known influence on blood–brain barrier integrity. Finally, a random intercept for patient ID (subj) was added to account for repeated measurements within subjects.
The five models evaluated were:
We used the Akaike Information Criterion (AIC), the Bayesian Information Criterion (BIC), and likelihood ratio tests to compare model fits. These metrics allowed us to evaluate both model simplicity (AIC/BIC) and model improvement through nested comparisons (Chi-squared tests). Once the best model was selected, we further evaluated model performance using key classification metrics: Accuracy: proportion of correct predictions. Sensitivity: proportion of actual positive cases correctly identified. Specificity: proportion of actual negative cases correctly identified. Positive Predictive Value (PPV) and Negative Predictive Value (NPV): indicating the predictive accuracy for positive and negative relapse cases. Bootstrapping was applied to estimate confidence intervals around these metrics, providing robust measures of variability. To quantify the effect of relapse (y/n) and MP treatment (y/n) on Ki we performed a post-hoc mixed model general linear regression analysis with Ki as the dependent variable and relapse and MP treatment as explanatory variables. A p-value lower than 0.05 allowed rejection of the null hypothesis. Statistical analysis was performed using SPSS 28.0, with the assistance of a professional statistician from the Biostatistical Department at the University of Copenhagen.
Nineteen out of 20 subjects completed all three scanning sessions, while one subject (subj 14) declined to participate in the final three-month scan, giving a total of 59 scanning sessions with complete structural MRI and Ki measurement data. At study entry 16 out of 20 subjects (80%) were classified as having a clinical MS relapse at study entry, of which 14 received MP treatment. Of the 16 with a relapse, six were classified as having a major relapse (38%). Three subjects had received MP treatment within 6 weeks before study entry due to a previous relapse. During the follow-up period, four subjects were classified as having a new, separate relapse, three of which required a course of MP treatment. Subject demographics and clinical variables are shown in Table 1. Clinical description of the relapse symptoms, MP use pre-and post-baseline, and information on relapses during the follow-up period for each subject is described in Table 2. T1 contrast-enhancing lesions were present at baseline in eight out of 20 subjects (40%). Ten subjects (50%) had new T2 white matter lesions at baseline when compared to the most recent previous MRI scan, and eight out of 10 had MRI contrast enhancement in one or more of these new T2 lesions.
Table 1. Subject demographics, clinical data, and Ki values according to relapse status at baseline.
We evaluated the five constructed models on key classification metrics—accuracy, sensitivity, specificity, PPV, and NPV— using bootstrapping for confidence intervals. Since CEL and EBL showed strong colinearity we avoided adding them simultaneously to the same model. Model performance metrics and confidence intervals for the investigated models are depicted in Table 3. Model 4 [Relapse ~ Ki + EBL + MP use + (1 | subj)], demonstrated the best fit based on the AIC, BIC, and Chi-squared tests showing the lowest deviance and a significant improvement over the other models (p = 0.006). Regarding diagnostic accuracy Model 4 achieved the highest combined accuracy and PPV, at the expense of a slightly decreased sensitivity and NPV, suggesting that including Ki along with EBL enhances predictive accuracy. The development of Ki during the study according to relapse and MP treatment status is shown in Figure 2. An example of the development of voxel-wise Ki, CBV, and CBF from a representative subject with a major relapse can be seen in Figure 3. When further stratifying the subjects according to minor and major relapse, Ki did not significantly predict relapse severity but showed a trend for higher values in the major relapse group (see Figure 4). Post-hoc mixed model analysis showed a significant effect of relapse (β = 0.011, p = 0.03) and MP use (β = −0.018, p = 0.002) on Ki, meaning that Ki was 0.011 mL/100 g/min higher in the context of relapse and 0.018 mL/100 g/min lower in the context of MP treatment.
Figure 2. Spaghetti plots of Ki development during the study. Influx constant (Ki) in NAWM for each subject plotted over time divided by confirmed relapse and MP treatment. A post-hoc mixed model general linear regression analysis showed that Ki was 0.011 mL/100 g/min higher (p = 0.03) in the context of relapse and 0.018 mL/100 g/min lower (p = 0.002) after MP use. Four patients were classified as having a new relapse within +/− 2 months from the post-relapse months scan, marked with (*). Three subjects received MP treatment <6 weeks prior to study inclusion, marked with (+). MP, Methylprednisolone; NAWM, Normal appearing white matter.
Figure 3. Kinetic parameter maps. Three serial DCE-MRI scans of the same RRMS subject with a confirmed relapse (top row = at relapse onset; middle row = 2 weeks post MP treatment; bottom row = 3 months post relapse). Voxel wise cerebral blood flow (CBF; left column), cerebral blood volume (CBV; middle column) and Influx constant (Ki; right column) from one DCE slice. Note that CBF and CBV are unchanged, while Ki is initially high, low after 2 weeks of MP treatment, and increases again after 3 months. CBF, cerebral blood flow; CBV, cerebral blood volume; DCE-MRI, dynamic contrast-enhanced MRI; Ki, Influx constant; MP, Methylprednisolone.
Figure 4. Baseline Patlak Ki in NAWM according to relapse severity at time of admission. NAWM, Normal appearing white matter.
Eight out of 20 subjects (~40%) had one or more contrast-enhancing lesions on baseline MRI. When addressing MRI contrast-enhancing lesions in terms of both presence of 1 or more lesions (Supplementary Figure 2), or total lesion volume (Supplementary Figure 3), we did not observe any associations with Ki in neither NAWM nor in the thalamus. Nor did we find any significant associations between Ki and the presence of new T2 lesions or the total T2 lesion volume (ml).
The best performance for predicting clinical relapses was achieved by adding a combination of conventional MRI metrics (contrast-enhancing lesions and new T2 lesions) as well as Ki in NAWM into the model, with a significant improvement in predictive performance compared to the other investigated models.
The strong performance metrics, including high sensitivity and specificity, indicate that the proposed model could serve as an effective tool in clinical settings, offering a balanced predictive ability for both positive and negative relapse cases. The inclusion of MP use as a confounder was essential to account for its impact on the blood–brain barrier and potential alterations in Ki values, underscoring the importance of controlling for clinical interventions in predictive modeling.
The relationship between Ki, MS relapses, and MP use observed in this study is consistent with previous research showing a similar association but in a cross-sectional study design (Cramer et al., 2014). Thus, here we can substantiate this observation in a serial study design, indicating that Ki may reflect changes on the individual subject level, related to disease activity and treatment. The proposed model outperformed the other investigated models in terms of accuracy and PPV, suggesting that both Ki and EBL capture relevant but distinct aspects of the underlying MS pathophysiology, making their combined inclusion valuable. Regarding diagnostic accuracy Model 4 (Ki + EBL) achieved the highest combined accuracy and PPV, at the expense of a slightly decreased sensitivity and NPV, suggesting that including Ki along with EBL enhances predictive accuracy. Although the predictive values of both Model 4 and Model 5 offer clinical insights, Model 4 stands out with a higher Positive Predictive Value (PPV), meaning it more reliably identifies true attacks when positive results are indicated. This is clinically valuable, as it minimizes the risk of missing cases that may require follow-up, treatment and intervention. Therefore, Model 4 is preferred, as it provides a balance of accuracy and clinical relevance, increasing the likelihood that detected relapses accurately reflect a patient’s condition.
Notably, models incorporating only a single predictor (EBL, CEL, or Ki) demonstrated inferior performance, underscoring the limitations of individual predictors in capturing the multifaceted clinical profile of MS relapses. For the conventional MR imaging metrics, this finding aligns with prior research reporting a modest yet statistically significant correlation with clinical relapses in RRMS over a short-term observation period, with a high sensitivity but relatively lower specificity (Kappos et al., 1999; Rovaris et al., 2003). It is perhaps noteworthy that the addition of Ki to the predictive model appears to improve specificity for relapse detection, thus providing unique and complementary information on the dynamics of subtle changes in MS inflammation beyond visible lesion activity.
A large degree of heterogeneity in Ki at the post-relapse scan was observed, where not all subjects exhibited the expected decrease in Ki despite being free of clinical relapse symptoms. This may have several possible interpretations, including, but not limited to (1) the effect of a relapse on Ki is delayed or lasts longer than 3 months, (2) subjects with an intrinsic aggressive MS phenotype show a persistently elevated Ki irrespective of current relapse activity, or (3) subjects currently receiving a suboptimal disease-modifying treatment have persistently elevated Ki. To investigate this question, additional follow-up scans, or ideally a pre-relapse baseline scan, would have been of benefit.
While it is well accepted that MP treatment reverses contrast-enhancement (Barkhof et al., 1991; Sellebjerg et al., 2003) and therefore large focal changes in BBB permeability, it has not yet been confirmed that this is the case with more widespread low-level BBB permeability change. Animal and human studies suggest that MP restores BBB integrity (Kesselring et al., 1989; Barkhof et al., 1991; Sellebjerg et al., 2003; Smets et al., 2017) while we have previously demonstrated a cross-sectional association between recent MP treatment and a less permeable blood–brain barrier, as measured by Ki, indicative of a therapeutic effect (Cramer et al., 2014, 2018). The results presented here confirm that MP also reverses more widespread low-level BBB leakage, as measured with Ki. This is in line with animal and human studies showing that MP exerts its therapeutic effects by suppressing immune cell infiltration (Myhr and Mellgren, 2009; Smets et al., 2017) and restoring BBB integrity (Kesselring et al., 1989; Barkhof et al., 1991; Sellebjerg et al., 2003; Smets et al., 2017). While MP treatment was significantly associated with Ki in the post-hoc mixed model, there was heterogeneity in the treatment response among patients. Some individuals exhibited a complete normalization of Ki values, with values close to those observed in healthy controls (Cramer et al., 2014; Varatharaj et al., 2019) while others showed only partial improvement. These variations in treatment response may reflect different trajectories in treatment response with respect to the extent of BBB disruption and baseline neuroinflammatory activity.
The repeated measures approach used in this study yielded a total of 59 measurement points for Ki as well as for structural MRI data. While this should give sufficient power for performing a model analysis with three predictors, a key limitation of this study is still the relatively limited sample size, of 20 subjects, which may affect the generalizability of our findings. A smaller sample can increase the likelihood of overfitting, especially in models with multiple predictors. This limitation restricts our ability to make broad conclusions about the predictive accuracy of Ki in diverse populations and across different clinical settings. Additionally, while we included MP use as a confounder to control for its effects, other unmeasured confounders may also influence the outcome variable potentially biasing our results. Moreover, since our data were collected from a specific clinical population with unique characteristics, the predictive performance of the proposed model may vary when applied to a broader cohort or in patients with different comorbidities or clinical histories. Future research should validate this model across larger, more diverse populations to assess its robustness and establish whether these findings hold true in different patient groups.
A potential confounder regarding the “outside-of-relapse” scan is the influence of DMT initiation during the study period. Six subjects started new DMT or changed DMT shortly before the three-month follow-up, which may have influenced the Ki values. However, as previously reported, the effect of DMT on Ki appears to be negligible after 3 months of treatment, with a significant effect observed only after 6 months (Cramer et al., 2016) indicating that any confounding impact is likely minimal.
In conclusion, this study identifies a robust predictive model for detecting MS relapses, that combines conventional MRI lesional information with Ki in NAWM, derived from DCE-MRI. This suggests that adding DCE-MRI could improve reliability in early identification of MS relapses. Future studies should consider validating this model in independent datasets and exploring additional predictors that may further improve prediction accuracy.
The raw data supporting the conclusions of this article will be made available by the authors, without undue reservation.
The studies involving humans were approved by Videnskabsetisk Komite H-1-2014-132. The studies were conducted in accordance with the local legislation and institutional requirements. The participants provided their written informed consent to participate in this study.
SC: Conceptualization, Data curation, Formal analysis, Funding acquisition, Investigation, Methodology, Project administration, Resources, Software, Validation, Visualization, Writing – original draft, Writing – review & editing. NH: Formal analysis, Software, Writing – review & editing. HS: Data curation, Formal analysis, Project administration, Software, Writing – review & editing. MV: Methodology, Software, Validation, Writing – review & editing. AV: Conceptualization, Methodology, Validation, Writing – review & editing. IG: Conceptualization, Investigation, Validation, Writing – review & editing. UL: Conceptualization, Formal analysis, Investigation, Methodology, Software, Validation, Writing – review & editing. JF: Investigation, Supervision, Validation, Writing – review & editing. HL: Conceptualization, Formal analysis, Funding acquisition, Investigation, Methodology, Project administration, Resources, Software, Supervision, Validation, Writing – review & editing.
The author(s) declare that financial support was received for the research and/or publication of this article. This study was supported by the Danish Multiple Sclerosis Society (Grant nos. A42545 and A29895).
The authors wish to thank neurologists and multiple sclerosis patients from the multiple sclerosis clinic at Rigshospitalet and also thank radiographers K. E. Segers, R. H. Tavangar, and B. S. Møller.
HL and SC received funding from Sanofi Genzyme and the Danish Multiple Sclerosis Society. Sanofi Genzyme had no influence on the study design, inclusion of patients, data analysis or interpretation. IG received research funding from Merck Serono and Evgen, and travel funding from Teva and Novartis. JF served on scientific advisory boards for and received funding for travel related to these activities as well as honoraria from Biogen Idec, Merck Serono, Sanofi-Aventis, Teva, Novartis, and Almirall, received speaker honoraria from Biogen Idec, Teva, and Novartis, served as advisor on preclinical development for Takeda, and participates in advisory board meetings with Alexion and Chiesi. AV was funded by the National Institute for Health Research (UK) and has received travel funding from Teva.
The remaining authors declare that the research was conducted in the absence of any commercial or financial relationships that could be construed as a potential conflict of interest.
The author(s) declare that no Gen AI was used in the creation of this manuscript.
All claims expressed in this article are solely those of the authors and do not necessarily represent those of their affiliated organizations, or those of the publisher, the editors and the reviewers. Any product that may be evaluated in this article, or claim that may be made by its manufacturer, is not guaranteed or endorsed by the publisher.
The Supplementary material for this article can be found online at: https://www.frontiersin.org/articles/10.3389/fnins.2025.1546236/full#supplementary-material
SUPPLEMENTARY FIGURE 1 | DCE-MRI overview. Dynamic contrast-enhanced MRI at 3T; 5 slices; 8 mm slice thickness; time resolution 1.25 s, total scan time 15 minutes.
SUPPLEMENTARY FIGURE 2 | Ki according to presence on one or more contrast enhancing lesion. No difference in Ki in NAWM was observed in subjects with visually contrast enhancing lesions elsewhere in the cerebrum. NAWM, Normal appearing white matter.
SUPPLEMENTARY FIGURE 3 | Ki versus total contrast enhancing lesions volume. Patlak Ki in NAWM plotted against the total volume of visual contrast enhancing lesions in ml. MP, Methylprednisolone; NAWM, Normal appearing white matter.
Barber, T. W., Brockway, J. A., and Higgins, L. S. (1970). The density of tissues in and about the head. Acta Neurol. Scand. 46, 85–92. doi: 10.1111/j.1600-0404.1970.tb05606.x
Barkhof, F., Hommes, O., Scheltensh, P., and Valk, J. (1991). Quantitative MRI changes in gadolinium–DTPA enhancement after high–dose intravenous methylprednisolone in multiple sclerosis. Neurology 41, 1219–1222. doi: 10.1212/wnl.41.8.1219
Buljevac, D., Flach, H. Z., Hop, W. C. J., Hijdra, D., Laman, J. D., Savelkoul, H. F. J., et al. (2002). Prospective study on the relationship between infections and multiple sclerosis exacerbations. Brain 125, 952–960. doi: 10.1093/brain/awf098
Cobo-Calvo, A., Tur, C., Otero-Romero, S., Carbonell-Mirabent, P., Ruiz, M., Pappolla, A., et al. (2023). Association of Very Early Treatment Initiation with the risk of long-term disability in patients with a first demyelinating event. Neurology 101, E1280–E1292. doi: 10.1212/WNL.0000000000207664
Correale, J., Fiol, M., and Gilmore, W. (2006). The risk of relapses in multiple sclerosis during systemic infections. Neurology 67, 652–659. doi: 10.1212/01.wnl.0000233834.09743.3b
Cramer, S. P., Frederiksen, J. L., and Larsson, H. B. W. (2016). “Permeability of the blood-brain barrier in normal appearing white and grey matter predicts early inadequate treatment response to fingolimod og natalizumab,” in Multiple Sclerosis. Conference: 32nd Congress of the European Committee for Treatment and Research in Multiple Sclerosis, ECTRIMS.
Cramer, S. P., and Larsson, H. B. W. (2014). Accurate determination of blood-brain barrier permeability using dynamic contrast-enhanced T1-weighted MRI: a simulation and in vivo study on healthy subjects and multiple sclerosis patients. J. Cereb. Blood Flow Metab. 34, 1655–1665. doi: 10.1038/jcbfm.2014.126
Cramer, S. P., Larsson, H. B. W., Knudsen, M. H., Simonsen, H. J., Vestergaard, M. B., and Lindberg, U. (2022). Reproducibility and optimal arterial input function selection in dynamic contrast-enhanced perfusion MRI in the healthy brain. J. Magn. Reson. Imaging 57, 1229–1240. doi: 10.1002/jmri.28380
Cramer, S. P., Modvig, S., Simonsen, H. J., Frederiksen, J. L., and Larsson, H. B. W. (2015). Permeability of the blood-brain barrier predicts conversion from optic neuritis to multiple sclerosis. Brain 138, 2571–2583. doi: 10.1093/brain/awv203
Cramer, S. P., Simonsen, H., Frederiksen, J. L., Rostrup, E., and Larsson, H. B. W. (2014). Abnormal blood-brain barrier permeability in normal appearing white matter in multiple sclerosis investigated by MRI. Neuroimage Clin 4, 182–189. doi: 10.1016/j.nicl.2013.12.001
Cramer, S. P., Simonsen, H. J., Varatharaj, A., Galea, I., Frederiksen, J. L., and Larsson, H. B. W. W. (2018). Permeability of the blood-brain barrier predicts no evidence of disease activity at 2 years after natalizumab or fingolimod treatment in relapsing-remitting multiple sclerosis. Ann. Neurol. 83, 902–914. doi: 10.1002/ana.25219
Dallal, G. E., and Wilkinson, L. (1986). An analytic approximation to the distribution of Lilliefors’s test statistic for normality. Am. Stat. 40, 294–296. doi: 10.1080/00031305.1986.10475419
Dünschede, J., Ruschil, C., Bender, B., Mengel, A., Lindig, T., Ziemann, U., et al. (2023). Clinical-radiological mismatch in multiple sclerosis patients during acute relapse: discrepancy between clinical symptoms and active, topographically fitting MRI lesions. J. Clin. Med. 12:739. doi: 10.3390/jcm12030739
Galea, I., Ward-Abel, N., and Heesen, C. (2015). Relapse in multiple sclerosis. BMJ (Online) 350:h1765. doi: 10.1136/bmj.h1765
Højsgaard Chow, H., Petersen, E. R., Olsson, A., Hejgaard Laursen, J., Bredahl Hansen, M., Oturai, A. B., et al. (2024). Age-corrected neurofilament light chain ratio decreases but does not predict relapse in highly active multiple sclerosis patients initiating natalizumab treatment. Mult. Scler. Relat. Disord. 88:105701. doi: 10.1016/j.msard.2024.105701
Hommes, O., Barkhof, F., Jongen, P., and Frequin, S. (1996). Methylprednisolone treatment in multiple sclerosis: effect of treatment, pharmacokinetics, future. Mult. Scler. J. 1, 327–328. doi: 10.1177/135245859600100607
Kappos, L., Moeri, D., Radue, E. W., Schoetzau, A., Schweikert, K., Barkhof, F., et al. (1999). Predictive value of gadolinium-enhanced magnetic resonance imaging for relapse rate and changes in disability or impairment in multiple sclerosis: a meta-analysis. Lancet 353, 964–969. doi: 10.1016/S0140-6736(98)03053-0
Kesselring, J., Miller, D. H., MacManus, D. G., Johnson, G., Milligan, N. M., Scolding, N., et al. (1989). Quantitative magnetic resonance imaging in multiple sclerosis: the effect of high dose intravenous methylprednisolone. J. Neurol. Neurosurg. Psychiatry 52, 14–17. doi: 10.1136/jnnp.52.1.14
Knudsen, M. H., Lindberg, U., Frederiksen, J. L., Vestergaard, M. B., Simonsen, H. J., Varatharaj, A., et al. (2022). Blood-brain barrier permeability changes in the first year after alemtuzumab treatment predict 2-year outcomes in relapsing-remitting multiple sclerosis. Mult. Scler. Relat. Disord. 63:103891. doi: 10.1016/j.msard.2022.103891
Larsson, H. B. W., Courivaud, F., Rostrup, E., and Hansen, A. E. (2009). Measurement of brain perfusion, blood volume, and blood-brain barrier permeability, using dynamic contrast-enhanced T 1-weighted MRI at 3 tesla. Magn. Reson. Med. 62, 1270–1281. doi: 10.1002/mrm.22136
Larsson, H. B. W., Hansen, A. E., Berg, H. K., Rostrup, E., and Haraldseth, O. (2008). Dynamic contrast-enhanced quantitative perfusion measurement of the brain using T1-weighted MRI at 3T. J. Magn. Reson. Imaging 27, 754–762. doi: 10.1002/jmri.21328
Larsson, H. B., Stubgaard, M., Frederiksen, J. L., Jensen, M., Henriksen, O., and Paulson, O. B. (1990). Quantitation of blood-brain barrier defect by magnetic resonance imaging and gadolinium-DTPA in patients with multiple sclerosis and brain tumors. Magn. Reson. Med. 16, 117–131.
Lilliefors, H. W. (1967). On the Kolmogorov-Smirnov test for normality with mean and variance unknown. J. Am. Stat. Assoc. 62:399. doi: 10.2307/2283970
Myhr, K. M., and Mellgren, S. I. (2009). Corticosteroids in the treatment of multiple sclerosis. Acta Neurol. Scand. 120, 73–80. doi: 10.1111/j.1600-0404.2009.01213.x
Rovaris, M., Comi, G., Ladkani, D., Wolinsky, J. S., and Filippi, M. (2003). Short-term correlations between clinical and MR imaging findings in relapsing-remitting multiple sclerosis. AJNR Am. J. Neuroradiol. 24, 75–81.
Schumacker, G. A., Beebe, G., Kibler, R. F., Kurland, L. T., Kurtzke, J. F., Mcdowell, F., et al. (1965). Problems of experimental trials of therapy in multiple sclerosis: report by the panel on the evaluation of experimental trials of therapy in multiple sclerosis. Ann. N. Y. Acad. Sci. 122, 552–568. doi: 10.1111/j.1749-6632.1965.tb20235.x
Sellebjerg, F., Jensen, C. V., Larsson, H. B. W., and Frederiksen, J. L. (2003). Gadolinium-enhanced magnetic resonance imaging predicts response to methylprednisolone in multiple sclerosis. Mult. Scler. 9, 102–107. doi: 10.1191/1352458503ms880sr
Smets, I., Van Deun, L., Bohyn, C., van Pesch, V., Vanopdenbosch, L., Dive, D., et al. (2017). Corticosteroids in the management of acute multiple sclerosis exacerbations. Acta Neurol. Belg. 117, 623–633. doi: 10.1007/s13760-017-0772-0
Sourbron, S., Ingrisch, M., Siefert, A., Reiser, M., and Herrmann, K. (2009). Quantification of cerebral blood flow, cerebral blood volume, and blood-brain-barrier leakage with DCE-MRI. Magn. Reson. Med. 62, 205–217. doi: 10.1002/mrm.22005
Stuart, C. M., Varatharaj, A., Zou, Y., Darekar, A., Domjan, J., Gandini Wheeler-Kingshott, C. A. M., et al. (2024). Systemic inflammation associates with and precedes cord atrophy in progressive multiple sclerosis. Brain Commun 6:fcae143. doi: 10.1093/braincomms/fcae143
Thrower, B. W. (2009). Relapse management in multiple sclerosis. Neurologist 15, 1–5. doi: 10.1097/NRL.0b013e31817acf1a
Keywords: MRI, DCE MRI, perfusion MRI, blood–brain barrier, multiple sclerosis: multiple sclerosis relapses, methylprednisolone
Citation: Cramer SP, Hamrouni N, Simonsen HJ, Vestergaard MB, Varatharaj A, Galea I, Lindberg U, Frederiksen JL and Larsson HBW (2025) Insights from DCE-MRI: blood–brain barrier permeability in the context of MS relapses and methylprednisolone treatment. Front. Neurosci. 19:1546236. doi: 10.3389/fnins.2025.1546236
Received: 16 December 2024; Accepted: 03 March 2025;
Published: 20 March 2025.
Edited by:
Mario Sansone, University of Naples Federico II, ItalyReviewed by:
S. Senthil Kumaran, All India Institute of Medical Sciences, IndiaCopyright © 2025 Cramer, Hamrouni, Simonsen, Vestergaard, Varatharaj, Galea, Lindberg, Frederiksen and Larsson. This is an open-access article distributed under the terms of the Creative Commons Attribution License (CC BY). The use, distribution or reproduction in other forums is permitted, provided the original author(s) and the copyright owner(s) are credited and that the original publication in this journal is cited, in accordance with accepted academic practice. No use, distribution or reproduction is permitted which does not comply with these terms.
*Correspondence: Stig P. Cramer, c3RpZy5wcmFlc3Rla2phZXIuY3JhbWVyQHJlZ2lvbmguZGs=
Disclaimer: All claims expressed in this article are solely those of the authors and do not necessarily represent those of their affiliated organizations, or those of the publisher, the editors and the reviewers. Any product that may be evaluated in this article or claim that may be made by its manufacturer is not guaranteed or endorsed by the publisher.
Research integrity at Frontiers
Learn more about the work of our research integrity team to safeguard the quality of each article we publish.