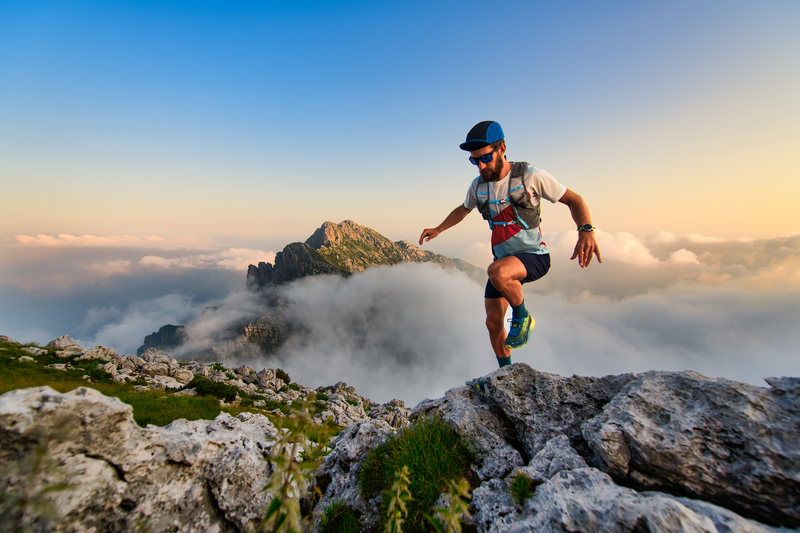
95% of researchers rate our articles as excellent or good
Learn more about the work of our research integrity team to safeguard the quality of each article we publish.
Find out more
ORIGINAL RESEARCH article
Front. Neurosci.
Sec. Neuroscience Methods and Techniques
Volume 19 - 2025 | doi: 10.3389/fnins.2025.1544452
The final, formatted version of the article will be published soon.
You have multiple emails registered with Frontiers:
Please enter your email address:
If you already have an account, please login
You don't have a Frontiers account ? You can register here
Brain-computer interfaces (BCIs) based on steady-state visual evoked potentials (SSVEP) rely on the brain's response to visual stimuli. However, accurately recognizing target frequencies using training-based methods remains challenging due to the time-consuming calibration sessions required by subject-specific training methods. To address this limitation, this study proposes a novel hybrid method called Hybrid task-related component and canonical correlation analysis (H-TRCCA). In the training phase, four spatial filters are derived using canonical correlation analysis (CCA) to maximize the correlation between the training data and reference signals. Additionally, a spatial filter is also computed using task-related component analysis (TRCA). In the test phase, correlation coefficients obtained from the CCA method are clustered using the k-means++ clustering algorithm. The cluster with the highest average correlation identifies the candidate stimuli. Finally, for each candidate, the correlation values are summed and combined with the TRCA-based correlation coefficients. The H-TRCCA algorithm was validated using two publicly available benchmark datasets. Experimental results using only two training trials per frequency with 1s data length showed that H-TRCCA achieved average accuracies of 91.44% for Dataset I and 80.46% for Dataset II. Additionally, it achieved maximum average information transfer rates of 188.36 bits/min and 139.96 bits/min for Dataset I and II, respectively. Remarkably H-TRCCA achieves comparable performance to other methods that require five trials, utilizing only two or three training trials. The proposed H-TRCCA method outperforms state-of-the-art techniques, showing superior performance and robustness with limited calibration data.
Keywords: brain-computer interface (BCI), electroencephalogram (EEG), Steady-state visual evoked potentials (SSVEP), Canonical correlation analysis (CCA), task-related component analysis (TRCA), Spatial Filtering
Received: 12 Dec 2024; Accepted: 02 Apr 2025.
Copyright: © 2025 Besharat, Samadzadehaghdam and Ghadiri. This is an open-access article distributed under the terms of the Creative Commons Attribution License (CC BY). The use, distribution or reproduction in other forums is permitted, provided the original author(s) or licensor are credited and that the original publication in this journal is cited, in accordance with accepted academic practice. No use, distribution or reproduction is permitted which does not comply with these terms.
* Correspondence:
Nasser Samadzadehaghdam, Tabriz University of Medical Sciences, Tabriz, Iran
Disclaimer: All claims expressed in this article are solely those of the authors and do not necessarily represent those of their affiliated organizations, or those of the publisher, the editors and the reviewers. Any product that may be evaluated in this article or claim that may be made by its manufacturer is not guaranteed or endorsed by the publisher.
Research integrity at Frontiers
Learn more about the work of our research integrity team to safeguard the quality of each article we publish.