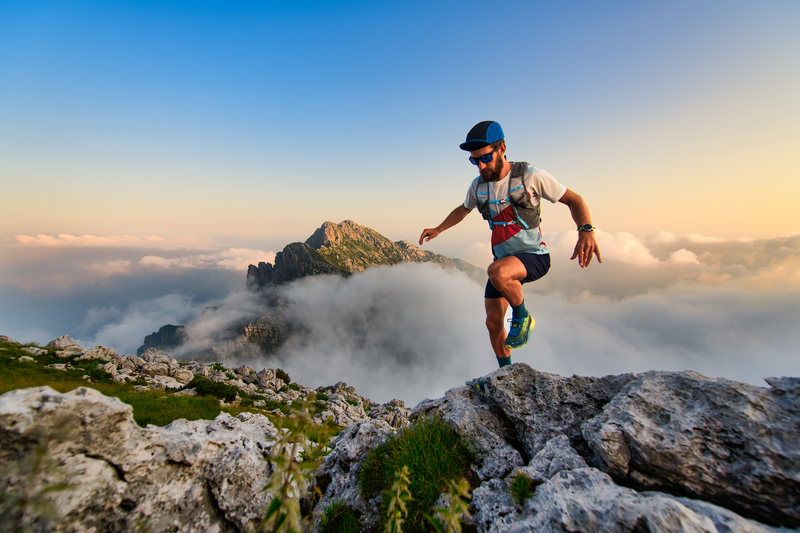
94% of researchers rate our articles as excellent or good
Learn more about the work of our research integrity team to safeguard the quality of each article we publish.
Find out more
ORIGINAL RESEARCH article
Front. Neurosci.
Sec. Sleep and Circadian Rhythms
Volume 19 - 2025 | doi: 10.3389/fnins.2025.1538479
The final, formatted version of the article will be published soon.
You have multiple emails registered with Frontiers:
Please enter your email address:
If you already have an account, please login
You don't have a Frontiers account ? You can register here
Idiopathic hypersomnia (IH) is a sleep disorder characterized by highly disruptive symptoms. Like narcolepsy type 1, a well-characterized sleep disorder, individuals with IH suffer from excessive daytime sleepiness, though there is little overlap in metabolic or neural biomarkers across these two disorders. This lack of common pathophysiology, combined with the clear overlap in symptoms presents an ideal paradigm for better understanding the impact of IH on an individual’s functional activity and organization, and potentially, the underlying pathophysiology. This study examines the observed functional connectivity in patients with IH, and patients with narcolepsy type 1 (NT1) against healthy control individuals. Static functional connectivity is compared, as are quasi-periodic patterns, acquired from the BOLD timecourse, for all groups. In addition to baseline data comparison, the study also included a post-nap condition, where the individuals included in this analysis napped for at least 10 minutes prior to the scanning session, to explore why individuals with IH do not feel “refreshed” after a nap like individuals with NT1 do. Assessing the groups’ spatiotemporal patterns revealed key differences across both disorders and conditions: static connectivity revealed at baseline higher subcortical connectivity in the NT1 group. There was also observably less connectivity in the IH group both at baseline and post-nap, though none of these static analyses survived multiple comparisons correction to reach significance. The quasi-periodic pattern (QPP) results however found significant differences in the IH group in key networks, particularly the DAN/FPCN correlation is significantly different at baseline vs. post-nap, a trend not observed in either the control or NT1 groups. The DAN and FPCN (task-positive correlates) are drastically altered both at baseline and post-nap when compared to the other groups, and may likely be a disorder-specific result. This study demonstrates that key networks for arousal are more heavily disrupted in IH patients, who are less affected by a nap, confirmed through both subject reporting and functional evidence through spatiotemporal patterns.
Keywords: idiopathic hypersomnia, Narcolepsy type 1 (NT1), functional MRI, functional connectivity (FC), Quasi-periodic pattern (QPP)
Received: 03 Dec 2024; Accepted: 03 Mar 2025.
Copyright: © 2025 Daley, Saini, Watters, Bassil, Schumacher, Trotti and Keilholz. This is an open-access article distributed under the terms of the Creative Commons Attribution License (CC BY). The use, distribution or reproduction in other forums is permitted, provided the original author(s) or licensor are credited and that the original publication in this journal is cited, in accordance with accepted academic practice. No use, distribution or reproduction is permitted which does not comply with these terms.
* Correspondence:
Lynn Marie Trotti, Department of Neurology, School of Medicine, Emory University, Atlanta, 30322, Georgia, United States
Shella Keilholz, Wallace H. Coulter Department of Biomedical Engineering, College of Engineering, Georgia Institute of Technology, Atlanta, 30332, Georgia, United States
Disclaimer: All claims expressed in this article are solely those of the authors and do not necessarily represent those of their affiliated organizations, or those of the publisher, the editors and the reviewers. Any product that may be evaluated in this article or claim that may be made by its manufacturer is not guaranteed or endorsed by the publisher.
Research integrity at Frontiers
Learn more about the work of our research integrity team to safeguard the quality of each article we publish.