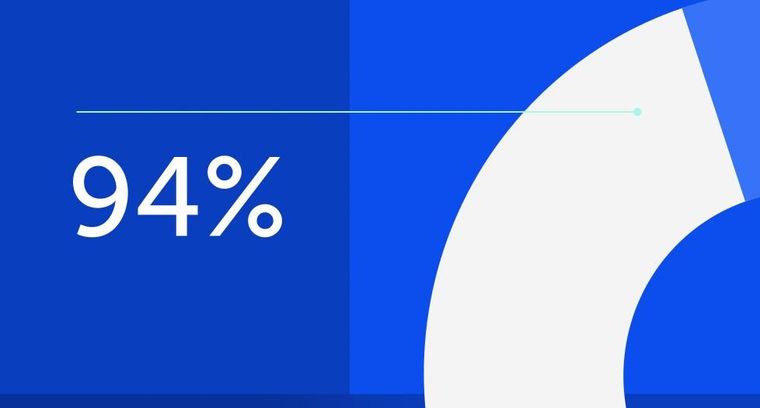
94% of researchers rate our articles as excellent or good
Learn more about the work of our research integrity team to safeguard the quality of each article we publish.
Find out more
ORIGINAL RESEARCH article
Front. Neurosci.
Sec. Sleep and Circadian Rhythms
Volume 19 - 2025 | doi: 10.3389/fnins.2025.1525417
The final, formatted version of the article will be published soon.
Select one of your emails
You have multiple emails registered with Frontiers:
Notify me on publication
Please enter your email address:
If you already have an account, please login
You don't have a Frontiers account ? You can register here
Automated machine-learning algorithms that analyze biomedical signals have been used to identify sleep patterns and health issues. However, their performance is often suboptimal, especially when dealing with imbalanced datasets. In this paper, we present a robust sleep state (SlS) classification algorithm utilizing electroencephalogram (EEG) signals. To this aim, we pre-processed EEG recordings from 33 healthy subjects. Then, functional connectivity features and recurrence quantification analysis were extracted from sub-bands. The graphical representation was calculated from phase locking value, coherence, and phase-amplitude coupling. Statistical analysis was used to select features with p-values of less than 0.05. These features were compared between four states: wakefulness, non-rapid eye movement (NREM) sleep, rapid eye movement (REM) sleep during presenting auditory stimuli, and REM sleep without stimuli. Eighteen types of different stimuli including instrumental and natural sounds were presented to participants during REM. The selected significant features were used to train a novel deep-learning classifiers. We designed a graph-informed convolutional autoencoder called GICA to extract high-level features from the functional connectivity features. Furthermore, an attention layer based on recurrence rate features extracted from EEGs was incorporated into the GICA classifier to enhance the dynamic ability of the model. The proposed model was assessed by comparing it to baseline systems in the literature. The accuracy of the SlS-GICA classifier is 99.92% on the significant feature set. This achievement could be considered in real-time and automatic applications to develop new therapeutic strategies for sleep-related disorders.
Keywords: auditory stimuli, Convolutional Neural Network, EEG, functional connectivity, graphical representation, Sleep preprocessing, feature extraction, Feature Selection
Received: 09 Nov 2024; Accepted: 04 Apr 2025.
Copyright: © 2025 Zakeri, Makouei and Danishvar. This is an open-access article distributed under the terms of the Creative Commons Attribution License (CC BY). The use, distribution or reproduction in other forums is permitted, provided the original author(s) or licensor are credited and that the original publication in this journal is cited, in accordance with accepted academic practice. No use, distribution or reproduction is permitted which does not comply with these terms.
* Correspondence:
Somayeh Makouei, University of Tabriz, Tabriz, Iran
Sebelan Danishvar, Brunel University London, Uxbridge, UB8 3PH, London, United Kingdom
Disclaimer: All claims expressed in this article are solely those of the authors and do not necessarily represent those of their affiliated organizations, or those of the publisher, the editors and the reviewers. Any product that may be evaluated in this article or claim that may be made by its manufacturer is not guaranteed or endorsed by the publisher.
Supplementary Material
Research integrity at Frontiers
Learn more about the work of our research integrity team to safeguard the quality of each article we publish.