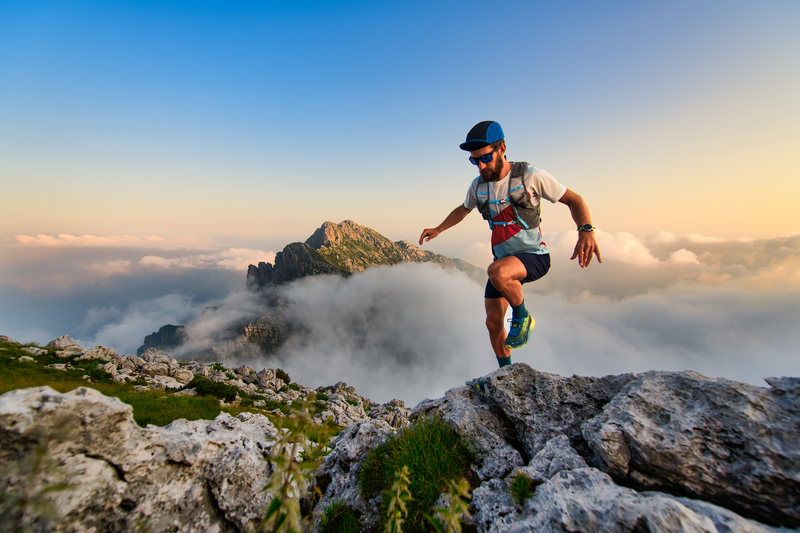
94% of researchers rate our articles as excellent or good
Learn more about the work of our research integrity team to safeguard the quality of each article we publish.
Find out more
ORIGINAL RESEARCH article
Front. Neurosci.
Sec. Neurodegeneration
Volume 19 - 2025 | doi: 10.3389/fnins.2025.1518984
The final, formatted version of the article will be published soon.
You have multiple emails registered with Frontiers:
Please enter your email address:
If you already have an account, please login
You don't have a Frontiers account ? You can register here
Mild cognitive impairment (MCI), often linked to early neurodegeneration, is associated with subtle disruptions in brain connectivity. The present study explores the applicability of persistent homology, a cutting-edge topological data analysis technique, for classifying MCI subtypes. This is done by examining brain network topology derived from fMRI time series data. In this regard, we investigate two methods for computing persistent homology: (1) Vietoris-Rips filtration, which leverages point clouds generated from fMRI time series to capture dynamic and global changes in brain connectivity, and (2) graph filtration, which examines connectivity matrices based on static pairwise correlations. The obtained persistent topological features are quantified using Wasserstein distance, which enables a detailed comparison of brain network structures. Our findings show that Vietoris-Rips filtration significantly outperforms graph filtration in brain network analysis. Specifically, it achieves a maximum accuracy of 85.7% in the Default Mode Network, for classifying MCI using in-house dataset. This study highlights the superior ability of Vietoris-Rips filtration to capture intricate brain network patterns, offering a robust tool for early diagnosis and precise classification of MCI subtypes.
Keywords: Mild Cognitive Impairment, fMRI time-series, Persistent homology, graph filtration, Vietoris-Rips filtration, Wasserstein distance, Classification
Received: 29 Oct 2024; Accepted: 10 Feb 2025.
Copyright: © 2025 Bhattacharya, Kaur, Aithal, Sinha and Gregor. This is an open-access article distributed under the terms of the Creative Commons Attribution License (CC BY). The use, distribution or reproduction in other forums is permitted, provided the original author(s) or licensor are credited and that the original publication in this journal is cited, in accordance with accepted academic practice. No use, distribution or reproduction is permitted which does not comply with these terms.
* Correspondence:
Debanjali Bhattacharya, Centre for Brain Research, Indian Institute of Science, Bangalore, 560012, Karnataka, India
Rajneet Kaur, Centre for Brain Research, Indian Institute of Science, Bangalore, 560012, Karnataka, India
Neelam Sinha, Centre for Brain Research, Indian Institute of Science, Bangalore, 560012, Karnataka, India
Thomas Gregor, Centre for Brain Research, Indian Institute of Science, Bangalore, 560012, Karnataka, India
Disclaimer: All claims expressed in this article are solely those of the authors and do not necessarily represent those of their affiliated organizations, or those of the publisher, the editors and the reviewers. Any product that may be evaluated in this article or claim that may be made by its manufacturer is not guaranteed or endorsed by the publisher.
Research integrity at Frontiers
Learn more about the work of our research integrity team to safeguard the quality of each article we publish.