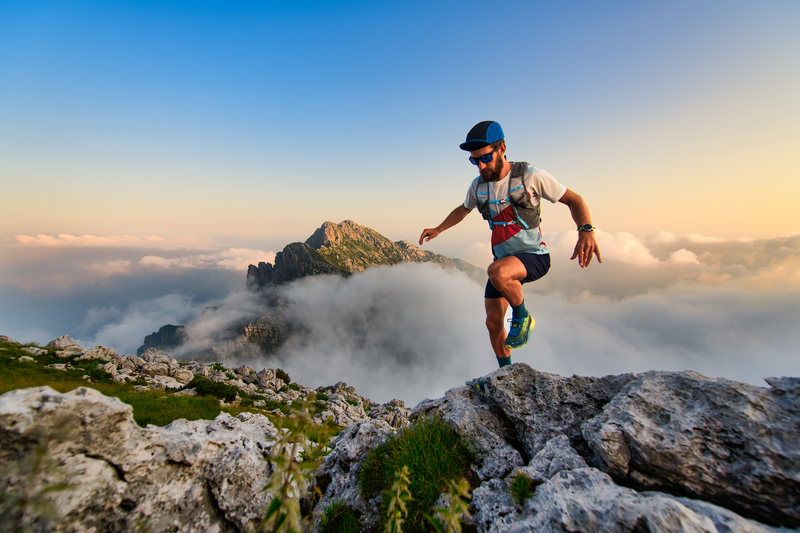
94% of researchers rate our articles as excellent or good
Learn more about the work of our research integrity team to safeguard the quality of each article we publish.
Find out more
ORIGINAL RESEARCH article
Front. Neurosci.
Sec. Translational Neuroscience
Volume 19 - 2025 | doi: 10.3389/fnins.2025.1512799
The final, formatted version of the article will be published soon.
You have multiple emails registered with Frontiers:
Please enter your email address:
If you already have an account, please login
You don't have a Frontiers account ? You can register here
With the increasing severity of mental health problems, the application of emotion recognition technology in mental health diagnosis and intervention has gradually gained attention.In this paper, we propose a multimodal emotion recognition method based on the fusion of electroencephalography (EEG) and electrocardiography (ECG) signals, aiming to achieve an accurate classification of emotional states.Aiming at the three dimensions of emotions (affect, arousal, and dominance), a composite neural network (Att-1DCNN-GRU) model combining a one-dimensional convolutional neural network (1DCNN) with an attentional mechanism and a gated recurrent unit (GRU) is designed in this study.The model extracts time-domain, frequency-domain and nonlinear features by fusing EEG and ECG signals, and uses the random forest method for feature screening to improve the accuracy and robustness of emotion recognition.In the experimental part, the proposed model is validated using the DREAMER dataset, and the results show that the model achieves high classification accuracy in all three emotion dimensions, VALENCE, AROUSAL and DOMINANCE, especially in the VALENCE dimension, where the accuracy reaches 95.95%.The fusion model significantly improves the recognition effect compared to the traditional methods using EEG or ECG signals alone.In addition, this study was validated on the DEAP dataset to further verify the generalisation ability and cross-dataset adaptability of the model.Through a series of comparison and ablation experiments, this study demonstrates the advantages of multimodal signal fusion in emotion recognition and shows the great potential of deep learning methods in processing complex physiological signals.The experimental results show that the Att-1DCNN-GRU model has a strong emotion recognition capability and provides valuable technical support for affective computing and mental health management.
Keywords: emotion recognition, EEG signal, ECG signal, multimodal, deep learning
Received: 17 Oct 2024; Accepted: 24 Feb 2025.
Copyright: © 2025 Wang and Wang. This is an open-access article distributed under the terms of the Creative Commons Attribution License (CC BY). The use, distribution or reproduction in other forums is permitted, provided the original author(s) or licensor are credited and that the original publication in this journal is cited, in accordance with accepted academic practice. No use, distribution or reproduction is permitted which does not comply with these terms.
* Correspondence:
Yihan Wang, Beijing University of Technology, Beijing, China
Disclaimer: All claims expressed in this article are solely those of the authors and do not necessarily represent those of their affiliated organizations, or those of the publisher, the editors and the reviewers. Any product that may be evaluated in this article or claim that may be made by its manufacturer is not guaranteed or endorsed by the publisher.
Research integrity at Frontiers
Learn more about the work of our research integrity team to safeguard the quality of each article we publish.