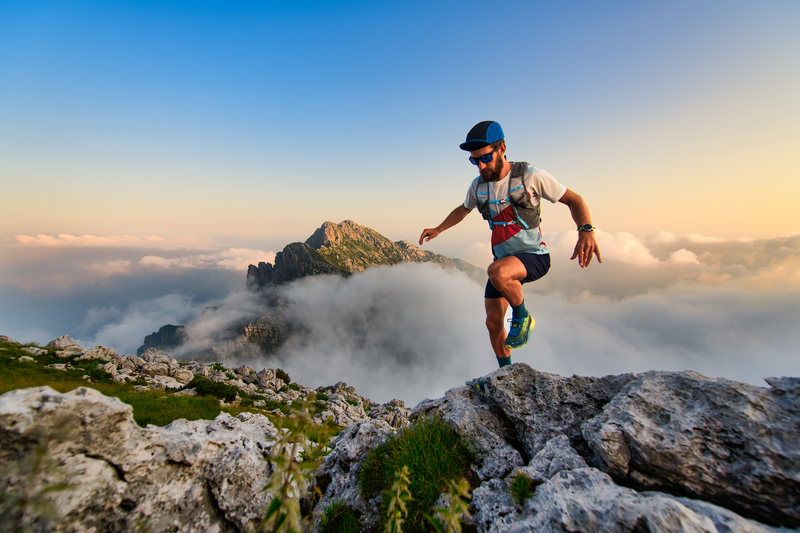
95% of researchers rate our articles as excellent or good
Learn more about the work of our research integrity team to safeguard the quality of each article we publish.
Find out more
ORIGINAL RESEARCH article
Front. Neurosci. , 15 January 2025
Sec. Neurodevelopment
Volume 18 - 2024 | https://doi.org/10.3389/fnins.2024.1508077
Objective: Repetitive and restricted behaviors (RRBs) are a core symptom of autism spectrum disorder (ASD), but effective treatment approaches are still lacking. Executive function (EF) has been identified as a promising target, as research increasingly shows a link between EF deficits and the occurrence of RRBs. However, the neural mechanisms that connect the two remain unclear. Since the orbitofrontal cortex (OFC) plays a role in both EF and RRBs, its functional connectivity dynamics could offer valuable insights into this relationship.
Methods: This study analyzed data from the Autism Brain Imaging Data Exchange (ABIDE) II database to explore brain function in 93 boys with ASD and 110 typically developing (TD) boys. Time-varying functional connectivity was analyzed between eight OFC subregions and other brain areas. By employing linear regression, the study assessed how atypical connectivity dynamics and EF influence RRBs. Additionally, mediation analysis with bootstrapping was used to determine how EF mediates the relationship between atypical connectivity and RRBs.
Results: We found significant differences in the variance of FC between ASD and TD groups, specifically in the OFC subregion in L-prefrontal and the left amygdala (t = 5.00, FDR q < 0.01). Regression analyses revealed that increased variance of this FC and EF significantly impacted RRBs, with inhibition, emotional control, and monitor showing strong associations (standardized β = 0.60 to 0.62, p < 0.01), which also had significant indirect effects on the relationship between the above dynamic FC and RRBs, which accounted for 59% of the total effect.
Conclusion: This study highlights the critical role of EFs as a key mechanism in addressing RRBs in ASD. Specifically, it points out that EFs mediate the influence of atypical time-varying interactions within the OFC-amygdala circuit on RRBs.
Repetitive and restricted behaviors (RRBs) include stereotyped or repetitive motor movements, insistence on sameness, highly restricted interests, and hyper- or hypo-reactivity to sensory input (American Psychiatric Association, 2013). Recent research has highlighted the potential role of executive functions (EF) in understanding RRBs within ASD populations (Jiujias et al., 2017). EF include high-level cognitive processes such as planning, organization, task management, impulse control, and the flexible adaptation to new information (Jones et al., 2018). Its main objective is to produce behavior that is coordinated, orderly, and purposeful. Deficits in EF are frequently observed in individuals with RRB. The previous meta-analyses found that high levels of RRBs related to poor performance on set-shifting and inhibitory control tasks (Iversen and Lewis, 2021). However, the relationship between EF and RRBs is complex and multifaceted, warranting further exploration.
The neural basis of EF is closely tied to several brain regions, especially the orbitofrontal cortex (OFC) (Li et al., 2024; Rudebeck and Rich, 2018). This region is central to EFs and plays a crucial role in emotional regulation, social cognition, and behavioral control (Rudebeck and Rich, 2018). Dysfunction in the OFC can exacerbate the behavioral traits seen in ASD (Liu et al., 2020). Previous research has found that increased volume of the OFC is associated with an increase in RRBs (Hegarty et al., 2020). Blocking the signal transduction of the 5-HT(2A) receptor in OFC results in an increase in these behaviors (Amodeo et al., 2017). In many tasks that indirectly represent repetitive stereotyped behaviors through response inhibition, the OFC shows heightened activity (Liu et al., 2020; Agam et al., 2010). However, these studies focus primarily on structural and genetic aspects, and while there are indirect task-related connections, the functional relationship between the OFC and RRBs remains unclear. Additionally, the role of executive functions EF in this process still requires further exploration.
The current cognitive model hypothesizes that due to poor cognitive control, the brain is in a hyper-sensitive state, which contribute to increased attention to negative information and, consequently, an increase in RRBs (Iversen and Lewis, 2021). The brain’s hyper-sensitive state primarily refers to an enhanced response to external stimuli (Leekam et al., 2011), and the temporal characteristics of brain activity reflect the brain’s response to these stimuli (Northoff and Huang, 2017). Studying the temporal characteristics of functional connectivity between the OFC and related brain regions can help us understand the state of the OFC and associated regions in response to external stimuli (Dinstein et al., 2015). The time-varying characteristics of functional connectivity refer to the dynamic changes in connection strength between different brain regions over time (Allen et al., 2014). Research has shown that individuals with ASD exhibit increased time-varying characteristics of functional connectivity, which may be associated with their behavioral abnormalities (Li et al., 2020). Thus, we hypothesize that the time-varying characteristics of brain regions may increase, indicating greater hypersensitivity and potentially leading to RRBs.
The different subregions of the OFC represent distinct cognitive functions, which can help us gain a more precise understanding of its underlying mechanisms (Qiu et al., 2024). In the study by Evans et al. (2004), it is noted that the medial OFC is involved in regulating motivation, as it connects with the VTA and nucleus accumbens. The lateral OFC, connected to the caudate nucleus, plays a role in motor coordination. Both OFC-striatal pathways communicate with the thalamus and influence motivation and behavior through feedback to the frontal and motor cortex. Additionally, the OFC is linked to the anterior cingulate cortex (ACC), which is involved in executive functions. Understanding the functions of these subregions and their roles in high-functioning ASD can provide crucial neurological insights into the relationship between RRBs and EF (Iversen and Lewis, 2021). Additionally, identifying the specific functional differences among these subregions can inform the development of more targeted intervention strategies to help individuals with high-functioning ASD improve EF and reduce RRBs. Investigating how OFC influences the relationship between EF and RRBs is vital for uncovering ASD’s neurobiological mechanisms.
Given the central role of RRBs in ASD and the potential influence of EF, this study seeks to explore the dynamic relationship between the OFC and RRBs in children with ASD, with a focus on EF as a mediating factor. We will evaluate the time-varying functional connectivity of the OFC in conjunction with comprehensive EF assessments and RRB behavioral evaluations. By analyzing how EF mediates the relationship between OFC connectivity and RRB severity, this study aims to uncover the cognitive and neural mechanisms driving RRBs. Our findings could provide insights for developing targeted interventions to improve EF and reduce RRBs in children with ASD.
Using the Autism Brain Imaging Data Exchange (ABIDE) II database, this study analyzed 93 boys with ASD and 110 boys with typical developmental, aged 5–13 years, from eight independent sites. There was no significant difference in age [t (202) = 1.650, p = 0.101] between the children with ASD (mean age = 9.85 ± 2.4) and those with TDs (mean age = 10.09 ± 1.98). This database employs the ADOS-2 for diagnosis, with the ADI-R used as a supplementary assessment tool.
Two versions of diagnostic tools, i.e., the Autism Diagnostic Observation Schedule, First Edition (ADOS I) and Autism Diagnostic Observation Schedule, Second Edition (ADOS II), were used in the two-stage ABIDE database, while ADOS_II was found to be more reliable than ADOS_I (Medda et al., 2019); only ABIDE II was used in this study. Only 122 boys with ASD had Autism Diagnostic Interview-Revised (ADI_R) scores. Participants were included when they met the following conditions: (1) boys, as 94% of participants were boys; (2) no severe structural damage in the T1 images; (3) single-band fMRI data; (4) longer than 5 min for scans; (5) considering the challenges of MRI data collection in children with ASD and referencing previous studies (Xie et al., 2022), our head motion criteria were set as follows: the maximum head motion should be less than 5 mm and 5 degrees, and the mean framewise displacement (FD) should be less than 0.5 mm. Specifically, mean FD was computed as the average of the frame-to-frame head displacement across the time series (Power et al., 2012). (6) Full-brain coverage and successful spatial normalization; (7) full-scale IQ higher than 70; (8) sites with more than 5 people. To ensure that each site had an adequate sample size, we removed sites with fewer than five participants, aiming to guarantee the statistical effect and reliability of the analysis results. The participant inclusion process is shown in Figure 1. Information for each site is provided in Table 1.
The Autism Diagnostic Interview-Revised (ADI-R) is a structured interview used for diagnosing autism and planning treatment (Lord et al., 1994). Conducted by a trained psychologist in a quiet setting, the interview lasts one to 2 h and involves caregivers answering 93 questions across three sections: social interaction, communication and language, repetitive and restricted behaviors.
The Behavior Rating Inventory of Executive Function (BRIEF) is a widely used assessment tool designed to evaluate EF behaviors in children and adolescents aged 5–18 years (Gui et al., 2022). This tool assesses EF behaviors in both home and school environments, providing a comprehensive overview of a child’s capabilities. The BRIEF comprises theoretically and statistically derived scales, which form two primary indexes: Behavioral Regulation and Metacognition. The Behavioral Regulation Index includes three scales (BRIEF_INHIBIT, BRIEF_SHIFT, BRIEF_EMOTIONAL), while the Metacognition Index includes five scales (BRIEF_INITIATE, BRIEF_WORKING MEMORY, BRIEF_PLAN/ORGANIZE, BRIEF_ ORGANIZATION OF MATERIALS, BRIEF_ MONITOR).
The Social Responsiveness Scale (SRS) is a tool used to assess ASD features in children and adolescents (Chan et al., 2017). It provides a total score and five subscale scores that indicate the severity of ASD symptoms. The total score ranges from 0 to 59 for normal, 60 to 75 for mild abnormalities, 76 to 90 for moderate, and 91 or above for severe abnormalities. The five subscales assess areas such as social interaction, social communication, social awareness, social cognition, and repetitive behaviors (social manners), each with a score range of 0 to 39.
T-scores in SRS and BRIEF are calculated by converting raw scores into standardized scores, with a mean of 50 and a standard deviation of 10 (Nguyen et al., 2019). The interpretation of T-scores is as follows: a T-score of 50 indicates that the individual’s social responsiveness level is at the median of the normal range; a T-score above 60 typically indicates higher than average levels, which may suggest difficulties or autism traits; a T-score below 40 usually indicates lower than average social responsiveness levels, suggesting fewer issues in social interaction. T-scores were obtained from the ABIDE database.
The resting-state fMRI data were pre-processed with a standardized pipeline using the Restplus V1.27 (Jia et al., 2019). Statistical Parametric Mapping (SPM12) was installed in MATLAB 2017b (Math Works, Natick, MA, United States). The processing pipeline included removing the first 10 repetition time (TR) images, slice-timing correction, motion realignment, spatial normalization to Montreal Neurological Institute (MNI) space, smoothing with a 6 mm3 Gaussian kernel, linear detrending, nuisance regression (for the following nuisance regressors: Friston’s 24 head-motion parameters, cerebrospinal fluid, white matter, and global signals), and temporal filtering (0.01–0.08 Hz). As the rsfMRI scanning duration varied between sites and the minimum was 5 min, we retained 5 min of the time course. This was done to ensure that the data from all participants remained consistent in terms of time series length, thereby avoiding the impact of time differences on the analysis results (Xie et al., 2022).
The OFC and whole brain regions were chosen based on the Shen_268 mask (Shen et al., 2013). According to Yale atlas (Noble et al., 2017; Demuru et al., 2017), these functional regions form 10 brain networks, including the medial frontal network (MFN), the frontoparietal network (FPN), the default mode network (DMN), the motor network (MON), the visual I network (Vis I), the visual II network (Vis II), the visual association network (VA), limbic, basal ganglia, and cerebellum. The eight subregions of the OFC form different functional networks (Table 2 and Figure 2). Therefore, in this study, the eight subregions of the OFC were defined as eight seed, and the functional connectivity between these subregions and the whole brain was calculated.
Dynamic BC2.2 (Liao et al., 2014) was utilized to examine time-varying functional connectivity between eight OFC subregions and other brain areas. The sliding window method, a standard approach for quantifying time-varying functional connectivity, was employed (Savva et al., 2019). This method involves segmenting the data into multiple equal-length segments to enhance temporal resolution. As a guideline, the minimum window length should exceed 1/fmin, where fmin is the lowest frequency of time courses (Leonardi and Van De Ville, 2015). Given a minimum TR value of 2 s in the dataset, we constructed functional connectivity matrix with a sliding window (rectangular time window) length of 30 TRs (60 s) and an overlap of 0.85, so if the first start time point is 1, the second window starts at 2.5 [floor (1 + (1 − 0.85) * 30)]. “Overlap” denotes the number of time points shared between consecutive windows. Within each window, we calculated the Pearson correlation analysis between eight OFC subregions and other brain areas. To assess time-varying functional connectivity, the variance matrix of functional connectivity in each window was analyzed for each participant.
ComBat harmonization was utilized to minimize site-related differences (Fortin et al., 2018). To maintain biologically relevant variability, we included age, group, mean FD, and IQ as covariates in the ComBat procedure. Then independent sample t-tests was used to assess the difference in the variance of functional connectivity between the ASD and TD groups (p < 0.05, FDR corrected), age and head movements were included as covariates to control for potential confounding factors. Performing statistical analysis using Graph Theoretical Network Analysis (GRETNA) toolbox (Wang et al., 2015).1 The tools Gretna and ComBat run in MATLAB 2017b.
To identify the relationship between atypical variance, EF and RRB, linear correlation was used in IBM SPSS Statistics 27. Furthermore, we employed a linear regression analysis. Variance and the scores from each BRIEF component were used as independent variables, while SRS_MANNERS scores served as the dependent variable. Age and head movements were included as covariates to control for potential confounding factors.
To investigate the mediating effect of EF on the relationship between atypical variance and behavior, we constructed a mediation model using Bootstrapping in IBM SPSS Amos 28. Variance were the independent variable, SRS_MANNERS were the dependent variable, and EF was the mediator. Perform bootstrap (number of bootstrap samples) is 2000. Bias corrected confidence intervals is 95.
After quality control, a total of 203 participants were enrolled in this study, including 93 boys with ASD and 110 TDs. All the participants were males. There was no significant difference in age [t (202) =1.650, p = 0.101] between the children with ASD (mean age = 9.85 ± 2.4) and those with TDs (mean age = 10.09 ± 1.98). The detailed demographics and clinical information of the participants are shown in Table 3.
Comparing the time-varying characteristics of functional connectivity between the eight OFC subregions and other brain regions in ASD and TD groups, we found that, after FDR correction (q < 0.05), only the OFC subregion in the L-prefrontal (Limbic network) and the left amygdala (motor network) (Noble et al., 2017) showed significant differences (Figure 3A and Table 4).
Figure 3. (A) Difference in variance of functional connectivity between ASD and TD. Significant differences identified in the L-prefrontal (limbic network) and left amygdala after FDR correction (q < 0.05). (B) There was a significant relationship with atypical variance and SRS_MANNER scores (r = 0.3, p < 0.01).
There was a significant relationship with variance and RRB (r = 0.3, p < 0.01) (Figure 3B). Additionally, there was a significant relationship between variance and EF, with correlations ranging from r = 0.18 to r = 0.33, p < 0.05. The regression analyses reveal that atypical and EF significantly impacts RRBs, controlling for age and mFD. Specifically, BRIEF_INHIBIT, BRIEF_EMOTIONAL, BRIEF_ORGANIZATION and BRIEF_MONITOR, with standardized β coefficients ranging from 0.60 to 0.62 and p-values all less than 0.01 (Table 5).
The mediation analysis assessed the impact of several mediators on the relationship between the independent variable and the dependent variable. The direct effect was found to be significant with a coefficient of c′ = 0.14 (p < 0.05). The indirect effects through the mediators were as follows: BRIEF_INHIBIT yielded an indirect effect of 0.08 (p < 0.01); BRIEF_EMOTIONAL contributed an indirect effect of 0.05 (p < 0.05); BRIEF_MONITOR produced an indirect effect of 0.07 (p < 0.01). In contrast, BRIEF_ORGANIZATION did not show a statistically significant indirect effect (p > 0.05). The total indirect effect, summing across all mediators, was 0.20 (p < 0.01). Including this total indirect effect with the direct effect resulted in an overall effect of 0.34 (p < 0.01). The magnitude of the mediation effect was calculated as the ratio of the total indirect effect to the total effect, yielding 59%. This indicates that the mediators—BRIEF_INHIBIT, BRIEF_EMOTIONAL, and BRIEF_MONITOR—account for 59% of the total effect (Figure 4).
Figure 4. Mediation analysis results. The diagram illustrates the direct effect (c′) and indirect effects through mediators (BRIEF_INHIBIT, BRIEF_EMOTIONAL, BRIEF_MONITOR), as well as the total effect and the proportion of the mediation effect.
This study found that executive functions in children with ASD were poorer than those in typically developing children. The increased dynamic characteristics of OFC-amygdala functional connectivity in children with ASD were associated with reduced executive functions. Furthermore, the increased dynamic characteristics of OFC-amygdala functional connectivity, reduced executive functions were related to heightened RRBs. Mediation analysis further revealed that executive functions (BRIEF_INHIBIT, BRIEF_EMOTIONAL, BRIEF_MONITOR) mediated the relationship between the dynamic characteristics of OFC-amygdala connectivity and RRBs.
We found that executive functions, as measured by the BRIEF subscales, were significantly poorer in children with ASD compared to TD children. This is consistent with previous research that highlights EF deficits, such as inhibitory control, emotional regulation, and self-monitoring, as hallmark impairments in ASD (Iversen and Lewis, 2021; Blijd-Hoogewys et al., 2014). In addition to confirming findings from previous studies regarding reduced emotional regulation abilities of individuals with ASD, we further discovered that this reduction is associated with an increase in the dynamic features of OFC (in LN)-amygdala connectivity. The significantly higher variance in functional connectivity between the OFC and amygdala in the ASD group compared to the TD group suggests that individuals with ASD experience greater fluctuations in neural communication within this circuitry (Fu et al., 2021). The OFC and amygdala play critical roles in emotional regulation and decision-making (Gao et al., 2016; Zheng et al., 2018; Li et al., 2021). The OFC, particularly within the limbic network (LN) (Noble et al., 2022), is involved in monitoring and evaluating emotional experiences to guide goal-directed behavior (Rolls et al., 2020), while the amygdala is responsible for generating and regulating emotions, including managing anxiety (Wang et al., 2013). The projections from the OFC to the amygdala have been identified through both optogenetics (Malvaez et al., 2019) and fiber photometry (Li et al., 2021), and these projections are excitatory and glutamatergic. Different subregions of the OFC project differently: the medial OFC (mOFC) is more closely connected to the basolateral amygdala (BLA), while the lateral OFC (lOFC) is more likely to be related to the lateral amygdala (Malvaez et al., 2019). Given the key roles these brain regions play in emotional regulation, the increased dynamic features of OFC (in LN)-amygdala connectivity, reflecting heightened sensitivity or instability in this circuitry, are likely to contribute to poor emotional regulation in children with ASD.
The OFC-amygdala projection matures gradually around puberty and plays a crucial role in regulating social behavior, fear memory, and emotional responses (Li et al., 2021). Abnormal developmental patterns are associated with various neurodevelopmental disorders (Andrews et al., 2022; Hessl et al., 2021; Xu et al., 2020). For instance, in individuals with autism, the amygdala is larger during childhood but shows slower growth, which correlates with differential changes in anxiety related to traditional DSM anxiety and autism-related anxiety (Andrews et al., 2022). Furthermore, mouse models with KMT2E gene haploinsufficiency show alterations in the number and size of amygdala neurons, which may be a potential mechanism for the social deficits observed in autism (Li et al., 2023). Morphological MRI studies report that children and adolescents with autism have reduced total right OFC volume (i.e., gray matter plus white matter), whereas adults show an increase in right OFC volume (Li et al., 2023). This highlights the importance of studying this circuit around puberty.
In addition, we found that the increased dynamic characteristics of OFC-amygdala connectivity were associated with an increase in RRBs. The association between increased OFC-amygdala connectivity variance and more pronounced RRBs further highlights the potential impact of neural instability on behavioral manifestations of ASD (Dinstein et al., 2015). Similar to previous research, our study found that increased time-varying characteristics of functional connectivity are positively correlated with ASD symptom severity (Li et al., 2020). The findings suggest that these behaviors could be exacerbated by the fluctuating connectivity patterns between key brain regions involved in executive functions.
We also found that the decline in executive functions was associated with an increase in RRBs. A decrease in emotional control ability was related to increased RRBs, further supporting the hypothesis that atypical changes in the OFC-amygdala circuitry are linked to emotional regulation abnormalities and may contribute to the development of RRBs (Iversen and Lewis, 2021). Inhibitory control deficits are commonly observed in individuals with ASD and are associated with RRBs (Schmitt et al., 2018). Inadequate inhibitory control can contribute to impulsive behaviors that disregard social norms (Diamond, 2013). Self-monitoring, on the other hand, reflects our ability to understand and adjust our behaviors based on our emotional states (Dorman et al., 2019). This function helps individuals recognize and manage their emotional responses, such as using techniques like deep breathing or cognitive reappraisal to alleviate anxiety.
The mediation analysis offers a deeper understanding of how these neural fluctuations might translate into RRBs (Lee et al., 2019). Specifically, increased variance in functional connectivity between the OFC and amygdala may disrupt inhibitory control, impairs emotional regulation, and reduces monitoring ability, leading to difficulties in stopping or managing behaviors, reducing anxiety and stress, and behavioral adjustment (Koch et al., 2018). These EFs dysregulation, in turn, exacerbates RRBs as a coping strategy (Wigham et al., 2015). While, previous research has highlighted the role of executive dysfunction in ASD behaviors (Jones et al., 2018; Cai et al., 2018), our results provide support for the hypothesis that instability in the OFC-amygdala connectivity may impair EFs, which are crucial for RRBs (Lindsay and Creswell, 2019; O’Malley et al., 2024).
By establishing a direct link between increased connectivity variance, EF impairment, and RRBs, our study suggests that therapeutic interventions targeting neural connectivity stability could be beneficial. Enhancing EF and improving emotional regulation might mitigate the negative effects of increased connectivity variance, potentially reducing RRBs and improving overall behavioral outcomes in individuals with ASD (Liang et al., 2022). Further research is needed to explore interventions that can stabilize neural connectivity and strengthen EFs in this population.
One limitation of this study is the cross-sectional design, which does not allow for causal inference regarding the relationship between executive functions, OFC-amygdala connectivity, and RRBs in children with ASD. Longitudinal studies are needed to better understand the temporal dynamics of these relationships and to explore potential causal pathways. Additionally, the participants in this study were all from Western countries, which may limit the generalizability of the findings to populations from other cultural or geographical regions. Future research should consider a more diverse sample to enhance the external validity of these results.
Publicly available datasets were analyzed in this study. This data can be found here: the imaging data for this study are available from the Autism Brain Imaging Data Exchange (ABIDE) II database (https://fcon_1000.projects.nitrc.org/indi/abide/abide_II.html).
The studies involving humans were approved by the ABIDE II project was carried out with approval from the local ethical committees at each participating site. The studies were conducted in accordance with the local legislation and institutional requirements. Written informed consent for participation in this study was provided by the participants’ legal guardians/next of kin.
XZ: Conceptualization, Investigation, Validation, Writing – original draft. XW: Validation, Writing – review & editing, Investigation, Software. RS: Writing – review & editing, Formal analysis, Investigation, Methodology. JT: Writing – review & editing, Formal analysis, Investigation, Methodology. LY: Supervision, Writing – review & editing, Data curation, Project administration.
The author(s) declare that no financial support was received for the research, authorship, and/or publication of this article.
We express our gratitude to all the participants for their collaboration in this study.
The authors declare that the research was conducted in the absence of any commercial or financial relationships that could be construed as a potential conflict of interest.
The authors declare that no Gen AI was used in the creation of this manuscript.
All claims expressed in this article are solely those of the authors and do not necessarily represent those of their affiliated organizations, or those of the publisher, the editors and the reviewers. Any product that may be evaluated in this article, or claim that may be made by its manufacturer, is not guaranteed or endorsed by the publisher.
Agam, Y., Joseph, R. M., Barton, J. J., and Manoach, D. S. (2010). Reduced cognitive control of response inhibition by the anterior cingulate cortex in autism spectrum disorders. NeuroImage 52, 336–347. doi: 10.1016/j.neuroimage.2010.04.010
Allen, E. A., Damaraju, E., Plis, S. M., Erhardt, E. B., Eichele, T., and Calhoun, V. D. (2014). Tracking whole-brain connectivity dynamics in the resting state. Cereb. Cortex 24, 663–676. doi: 10.1093/cercor/bhs352
American Psychiatric Association (2013). Force DT: diagnostic and statistical manual of mental disorders. Arlington, VA: American Psychiatric Association.
Amodeo, D. A., Rivera, E., Cook, E. J., Sweeney, J. A., and Ragozzino, M. E. (2017). 5HT2A receptor blockade in dorsomedial striatum reduces repetitive behaviors in BTBR mice. Genes Brain Behav. 16, 342–351. doi: 10.1111/gbb.12343
Andrews, D. S., Aksman, L., Kerns, C. M., Lee, J. K., Winder-Patel, B. M., Harvey, D. J., et al. (2022). Association of amygdala development with different forms of anxiety in autism spectrum disorder. Biol. Psychiatry 91, 977–987. doi: 10.1016/j.biopsych.2022.01.016
Blijd-Hoogewys, E. M., Bezemer, M. L., and van Geert, P. L. (2014). Executive functioning in children with ASD: an analysis of the BRIEF. J. Autism Dev. Disord. 44, 3089–3100. doi: 10.1007/s10803-014-2176-9
Cai, R. Y., Richdale, A. L., Uljarevic, M., Dissanayake, C., and Samson, A. C. (2018). Emotion regulation in autism spectrum disorder: where we are and where we need to go. Autism Res. 11, 962–978. doi: 10.1002/aur.1968
Chan, W., Smith, L. E., Hong, J., Greenberg, J. S., and Mailick, M. R. (2017). Validating the social responsiveness scale for adults with autism. Autism Res. 10, 1663–1671. doi: 10.1002/aur.1813
Demuru, M., Gouw, A. A., Hillebrand, A., Stam, C. J., van Dijk, B. W., Scheltens, P., et al. (2017). Functional and effective whole brain connectivity using magnetoencephalography to identify monozygotic twin pairs. Sci. Rep. 7:9685. doi: 10.1038/s41598-017-10235-y
Diamond, A. (2013). Executive functions. Annu. Rev. Psychol. 64, 135–168. doi: 10.1146/annurev-psych-113011-143750
Dinstein, I., Heeger, D. J., and Behrmann, M. (2015). Neural variability: friend or foe? Trends Cogn. Sci. 19, 322–328. doi: 10.1016/j.tics.2015.04.005
Dorman, I. S., Shafir, R., Birk, J. L., Bonanno, G. A., and Sheppes, G. (2019). Monitoring in emotion regulation: behavioral decisions and neural consequences. Soc. Cogn. Affect. Neurosci. 14, 1273–1283. doi: 10.1093/scan/nsaa001
Evans, D. W., Lewis, M. D., and Iobst, E. (2004). The role of the orbitofrontal cortex in normally developing compulsive-like behaviors and obsessive-compulsive disorder. Brain Cogn. 55, 220–234. doi: 10.1016/S0278-2626(03)00274-4
Fortin, J. P., Cullen, N., Sheline, Y. I., Taylor, W. D., Aselcioglu, I., Cook, P. A., et al. (2018). Harmonization of cortical thickness measurements across scanners and sites. NeuroImage 167, 104–120. doi: 10.1016/j.neuroimage.2017.11.024
Fu, Z., Sui, J., Turner, J. A., Du, Y., Assaf, M., Pearlson, G. D., et al. (2021). Dynamic functional network reconfiguration underlying the pathophysiology of schizophrenia and autism spectrum disorder. Hum. Brain Mapp. 42, 80–94. doi: 10.1002/hbm.25205
Gao, X., Gong, P., Liu, J., Hu, J., Li, Y., Yu, H., et al. (2016). COMT Val158Met polymorphism influences the susceptibility to framing in decision-making: OFC-amygdala functional connectivity as a mediator. Hum. Brain Mapp. 37, 1880–1892. doi: 10.1002/hbm.23142
Gui, Z., Yang, B., Bao, W., Zhang, J., Zhang, S., Zeng, X., et al. (2022). Association between household incense burning and executive function in Chinese children. Indoor Air 32:e13102. doi: 10.1111/ina.13102
Hegarty, I. J., Lazzeroni, L. C., Raman, M. M., Hallmayer, J. F., Cleveland, S. C., Wolke, O. N., et al. (2020). Genetic and environmental influences on corticostriatal circuits in twins with autism. J. Psychiatry Neurosci. 45, 188–197. doi: 10.1503/jpn.190030
Hessl, D., Libero, L., Schneider, A., Kerns, C., Winder-Patel, B., Heath, B., et al. (2021). Fear potentiated startle in children with autism spectrum disorder: association with anxiety symptoms and amygdala volume. Autism Res. 14, 450–463. doi: 10.1002/aur.2460
Iversen, R. K., and Lewis, C. (2021). Executive function skills are linked to restricted and repetitive behaviors: three correlational meta analyses. Autism Res. 14, 1163–1185. doi: 10.1002/aur.2468
Jia, X. Z., Wang, J., Sun, H. Y., Zhang, H., Liao, W., Wang, Z., et al. (2019). RESTplus: an improved toolkit for resting-state functional magnetic resonance imaging data processing. Sci. Bull. 64, 953–954. doi: 10.1016/j.scib.2019.05.008
Jiujias, M., Kelley, E., and Hall, L. (2017). Restricted, repetitive behaviors in autism spectrum disorder and obsessive-compulsive disorder: a comparative review. Child Psychiatry Hum. Dev. 48, 944–959. doi: 10.1007/s10578-017-0717-0
Jones, C., Simonoff, E., Baird, G., Pickles, A., Marsden, A., Tregay, J., et al. (2018). The association between theory of mind, executive function, and the symptoms of autism spectrum disorder. Autism Res. 11, 95–109. doi: 10.1002/aur.1873
Koch, S., Mars, R. B., Toni, I., and Roelofs, K. (2018). Emotional control, reappraised. Neurosci. Biobehav. Rev. 95, 528–534. doi: 10.1016/j.neubiorev.2018.11.003
Lee, H., Herbert, R. D., and McAuley, J. H. (2019). Mediation analysis. JAMA 321, 697–698. doi: 10.1001/jama.2018.21973
Leekam, S. R., Prior, M. R., and Uljarevic, M. (2011). Restricted and repetitive behaviors in autism spectrum disorders: a review of research in the last decade. Psychol. Bull. 137, 562–593. doi: 10.1037/a0023341
Leonardi, N., and Van De Ville, D. (2015). On spurious and real fluctuations of dynamic functional connectivity during rest. NeuroImage 104, 430–436. doi: 10.1016/j.neuroimage.2014.09.007
Li, A., Jing, D., Dellarco, D. V., Hall, B. S., Yang, R., Heilberg, R. T., et al. (2021). Role of BDNF in the development of an OFC-amygdala circuit regulating sociability in mouse and human. Mol. Psychiatry 26, 955–973. doi: 10.1038/s41380-019-0422-4
Li, Y. J., Li, C. Y., Li, C. Y., Hu, D. X., Xv, Z. B., Zhang, S. H., et al. (2023). KMT2E haploinsufficiency manifests autism-like behaviors and amygdala neuronal development dysfunction in mice. Mol. Neurobiol. 60, 1609–1625. doi: 10.1007/s12035-022-03167-w
Li, Y., Ma, S., Zhang, X., and Gao, L. (2024). ASD and ADHD: divergent activating patterns of prefrontal cortex in executive function tasks? J. Psychiatr. Res. 172, 187–196. doi: 10.1016/j.jpsychires.2024.02.012
Li, Y., Zhu, Y., Nguchu, B. A., Wang, Y., Wang, H., Qiu, B., et al. (2020). Dynamic functional connectivity reveals abnormal variability and hyper-connected pattern in autism spectrum disorder. Autism Res. 13, 230–243. doi: 10.1002/aur.2212
Liang, X., Li, R., Wong, S., Sum, R., Wang, P., Yang, B., et al. (2022). The effects of exercise interventions on executive functions in children and adolescents with autism spectrum disorder: a systematic review and meta-analysis. Sports Med. 52, 75–88. doi: 10.1007/s40279-021-01545-3
Liao, W., Wu, G. R., Xu, Q., Ji, G. J., Zhang, Z., Zang, Y. F., et al. (2014). DynamicBC: a MATLAB toolbox for dynamic brain connectome analysis. Brain Connect. 4, 780–790. doi: 10.1089/brain.2014.0253
Lindsay, E. K., and Creswell, J. D. (2019). Mindfulness, acceptance, and emotion regulation: perspectives from monitor and acceptance theory (MAT). Curr. Opin. Psychol. 28, 120–125. doi: 10.1016/j.copsyc.2018.12.004
Liu, X., Bautista, J., Liu, E., and Zikopoulos, B. (2020). Imbalance of laminar-specific excitatory and inhibitory circuits of the orbitofrontal cortex in autism. Mol. Autism. 11:83. doi: 10.1186/s13229-020-00390-x
Lord, C., Rutter, M., and Le Couteur, A. (1994). Autism diagnostic interview-revised: a revised version of a diagnostic interview for caregivers of individuals with possible pervasive developmental disorders. J. Autism Dev. Disord. 24, 659–685. doi: 10.1007/BF02172145
Malvaez, M., Shieh, C., Murphy, M. D., Greenfield, V. Y., and Wassum, K. M. (2019). Distinct cortical-amygdala projections drive reward value encoding and retrieval. Nat. Neurosci. 22, 762–769. doi: 10.1038/s41593-019-0374-7
Medda, J. E., Cholemkery, H., and Freitag, C. M. (2019). Sensitivity and specificity of the ADOS-2 algorithm in a large German sample. J. Autism Dev. Disord. 49, 750–761. doi: 10.1007/s10803-018-3750-3
Nguyen, P. H., Ocansey, M. E., Miller, M., Le, D. T. K., Schmidt, R. J., and Prado, E. L. (2019). The reliability and validity of the social responsiveness scale to measure autism symptomology in Vietnamese children. Autism Res. 12, 1706–1718. doi: 10.1002/aur.2179
Noble, S., Mejia, A. F., Zalesky, A., and Scheinost, D. (2022). Improving power in functional magnetic resonance imaging by moving beyond cluster-level inference. Proc. Natl. Acad. Sci. U.S.A. 119:e2091947177. doi: 10.1073/pnas.2203020119
Noble, S., Spann, M. N., Tokoglu, F., Shen, X., Constable, R. T., and Scheinost, D. (2017). Influences on the test-retest reliability of functional connectivity MRI and its relationship with behavioral utility. Cereb. Cortex 27, 5415–5429. doi: 10.1093/cercor/bhx230
Northoff, G., and Huang, Z. (2017). How do the brain’s time and space mediate consciousness and its different dimensions? Temporo-spatial theory of consciousness (TTC). Neurosci. Biobehav. Rev. 80, 630–645. doi: 10.1016/j.neubiorev.2017.07.013
O’Malley, B., Linz, R., Engert, V., and Singer, T. (2024). Testing the monitor and acceptance theory: the role of training-induced changes in monitoring- and acceptance-related capacities after attention-based, socio-emotional, or socio-cognitive mental training in reducing cortisol stress reactivity. Stress 27:2345906. doi: 10.1080/10253890.2024.2345906
Power, J. D., Barnes, K. A., Snyder, A. Z., Schlaggar, B. L., and Petersen, S. E. (2012). Spurious but systematic correlations in functional connectivity MRI networks arise from subject motion. NeuroImage 59, 2142–2154. doi: 10.1016/j.neuroimage.2011.10.018
Qiu, L., Qiu, Y., Liao, J., Li, J., Zhang, X., Chen, K., et al. (2024). Functional specialization of medial and lateral orbitofrontal cortex in inferential decision-making. iScience 27:110007. doi: 10.1016/j.isci.2024.110007
Rolls, E. T., Cheng, W., and Feng, J. (2020). The orbitofrontal cortex: reward, emotion and depression. Brain Commun. 2:fcaa196. doi: 10.1093/braincomms/fcaa196
Rudebeck, P. H., and Rich, E. L. (2018). Orbitofrontal cortex. Curr. Biol. 28, R1083–R1088. doi: 10.1016/j.cub.2018.07.018
Savva, A. D., Mitsis, G. D., and Matsopoulos, G. K. (2019). Assessment of dynamic functional connectivity in resting-state fMRI using the sliding window technique. Brain Behav. 9:e1255. doi: 10.1002/brb3.1255
Schmitt, L. M., White, S. P., Cook, E. H., Sweeney, J. A., and Mosconi, M. W. (2018). Cognitive mechanisms of inhibitory control deficits in autism spectrum disorder. J. Child Psychol. Psychiatry 59, 586–595. doi: 10.1111/jcpp.12837
Shen, X., Tokoglu, F., Papademetris, X., and Constable, R. T. (2013). Groupwise whole-brain parcellation from resting-state fMRI data for network node identification. NeuroImage 82, 403–415. doi: 10.1016/j.neuroimage.2013.05.081
Wang, C. C., Lin, H. C., Chan, Y. H., Gean, P. W., Yang, Y. K., and Chen, P. S. (2013). 5-HT1A-receptor agonist modified amygdala activity and amygdala-associated social behavior in a valproate-induced rat autism model. Int. J. Neuropsychopharmacol. 16, 2027–2039. doi: 10.1017/S1461145713000473
Wang, J., Wang, X., Xia, M., Liao, X., Evans, A., and He, Y. (2015). GRETNA: a graph theoretical network analysis toolbox for imaging connectomics. Front. Hum. Neurosci. 9:386. doi: 10.3389/fnhum.2015.00386
Wigham, S., Rodgers, J., South, M., McConachie, H., and Freeston, M. (2015). The interplay between sensory processing abnormalities, intolerance of uncertainty, anxiety and restricted and repetitive behaviours in autism spectrum disorder. J. Autism Dev. Disord. 45, 943–952. doi: 10.1007/s10803-014-2248-x
Xie, Y., Xu, Z., Xia, M., Liu, J., Shou, X., Cui, Z., et al. (2022). Alterations in connectome dynamics in autism spectrum disorder: a harmonized mega- and meta-analysis study using the autism brain imaging data exchange dataset. Biol. Psychiatry 91, 945–955. doi: 10.1016/j.biopsych.2021.12.004
Xu, Q., Zuo, C., Liao, S., Long, Y., and Wang, Y. (2020). Abnormal development pattern of the amygdala and hippocampus from childhood to adulthood with autism. J. Clin. Neurosci. 78, 327–332. doi: 10.1016/j.jocn.2020.03.049
Keywords: executive function, time-varying functional connectivity, repetitive and restricted behaviors, orbitofrontal cortex, autism spectrum disorder
Citation: Zheng X, Wang X, Song R, Tian J and Yang L (2025) Executive function, limbic circuit dynamics and repetitive and restricted behaviors in children with autism spectrum disorder. Front. Neurosci. 18:1508077. doi: 10.3389/fnins.2024.1508077
Received: 08 October 2024; Accepted: 31 December 2024;
Published: 15 January 2025.
Edited by:
Kazuhiko Sawada, Tsukuba International University, JapanReviewed by:
Zakaria Ouhaz, University of Oxford, United KingdomCopyright © 2025 Zheng, Wang, Song, Tian and Yang. This is an open-access article distributed under the terms of the Creative Commons Attribution License (CC BY). The use, distribution or reproduction in other forums is permitted, provided the original author(s) and the copyright owner(s) are credited and that the original publication in this journal is cited, in accordance with accepted academic practice. No use, distribution or reproduction is permitted which does not comply with these terms.
*Correspondence: Li Yang, eWFuZ2xpX3BrdWltaEBiam11LmVkdS5jbg==
Disclaimer: All claims expressed in this article are solely those of the authors and do not necessarily represent those of their affiliated organizations, or those of the publisher, the editors and the reviewers. Any product that may be evaluated in this article or claim that may be made by its manufacturer is not guaranteed or endorsed by the publisher.
Research integrity at Frontiers
Learn more about the work of our research integrity team to safeguard the quality of each article we publish.