- 1Department of Biopsychology, Faculty of Psychology, Institute of Cognitive Neuroscience, Ruhr-University Bochum, Bochum, Germany
- 2Department of Comparative Biomedicine and Food Science (BCA), University of Padova, Legnaro, Italy
- 3Department of Infectious Diseases and Public Health, Jockey Club College of Veterinary Medicine and Life Sciences, City University of Hong Kong, Hong Kong, Hong Kong SAR, China
Diffusion-weighted Imaging (DWI) is an effective and state-of-the-art neuroimaging method that non-invasively reveals the microstructure and connectivity of tissues. Recently, novel applications of the DWI technique in studying large brains through ex-vivo imaging enabled researchers to gain insights into the complex neural architecture in different species such as those of Perissodactyla (e.g., horses and rhinos), Artiodactyla (e.g., bovids, swines, and cetaceans), and Carnivora (e.g., felids, canids, and pinnipeds). Classical in-vivo tract-tracing methods are usually considered unsuitable for ethical and practical reasons, in large animals or protected species. Ex-vivo DWI-based tractography offers the chance to examine the microstructure and connectivity of formalin-fixed tissues with scan times and precision that is not feasible in-vivo. This paper explores DWI’s application to ex-vivo brains of large animals, highlighting the unique insights it offers into the structure of sometimes phylogenetically different neural networks, the connectivity of white matter tracts, and comparative evolutionary adaptations. Here, we also summarize the challenges, concerns, and perspectives of ex-vivo DWI that will shape the future of the field in large brains.
Introduction
Recent advances in magnetic resonance imaging (MRI) have made it a powerful tool for studying brain anatomy and function in different animals ranging from humans (Pfefferbaum et al., 2004; Dawe et al., 2009; McNab et al., 2009), non-human primates (D’Arceuil et al., 2007), rodents (Zhang J. et al., 2012; Russo et al., 2021), birds (Behroozi et al., 2020; Hamaide et al., 2020; Orije et al., 2021), reptiles (Behroozi et al., 2018; Billings et al., 2020), and even marine mammals (Berns et al., 2015; Gerussi et al., 2023, 2024). Diffusion-weighted imaging (DWI) distinguishes out from other approaches because it can non-invasively analyze neuroanatomical connectivity and white matter microstructure via measuring the restricted diffusion of water molecules in tissue (Le Bihan, 1996; Assaf et al., 2019). Given that water makes up around 60–70% of the body, understanding diffusion is critical. Diffusion refers to the random, thermally-driven, Brownian motion of molecules (Mukherjee et al., 2008). Water diffusivity in a homogeneous medium occurs randomly and isotropically with equal probability in all directions. On the other hand, water diffusivity in restricted environments surrounded by walls or membranes is anisotropic, and thus the probability of diffusion is higher along one direction over the others. It is therefore the case in brain tissue where water in the extracellular space or inside the somata diffuses isotropically, while water molecules inside neuronal axons are narrowly limited by axonal membranes, and thus the probability of diffusion parallel to the major axes is higher than the perpendicular (Moseley et al., 1991; Baliyan et al., 2016).
This random Brownian motion can be accurately estimated through DWI-MR sequences, employing gradients at different high amplitudes and combinations, i.e., directions. These sequences basically measure the anisotropic characteristics of water molecules which can reflect either the normal condition of the brain parenchyma or alterations directly from the raw data (anisotropy) in case of some pathologies (Benjamin et al., 2017; Mallon et al., 2020; Makada and Matang, 2023). The development of the algorithms encoding DWI sequences can then offer insights into the micro-architectural details of white matter at each voxel. Consequently, fiber tractography enables the elucidation of white matter integrity and the delineation of axonal tracts throughout the brain.
While in-vivo DWI has revolutionized the study of brain connectivity, ex-vivo DWI approaches offer distinct advantages, particularly in: (i) enhanced resolution: ex-vivo imaging allows for significantly higher voxel resolutions with enhanced signal-to-noise ratios (SNR), often reaching scales of hundreds of micrometers (Roebroeck et al., 2019). This level of resolution surpasses the constraints of in-vivo scans, typically limited to a range of 1–3 millimeters. The finer granularity of ex-vivo imaging facilitates an accurate mapping of intricate neural pathways and structures. Recent advances in DWI approaches have focused on optimizing SNR, voxel resolution, MR sequence, and the capacity to image big whole brains. These developments include the use of stronger gradients, higher magnetic field strengths, and customized pulse sequences that improve the contrast and resolution of DWI images, allowing the observation of previously undetectable microstructural features. (ii) Exploring rare and extinct species: ex-vivo DWI provides an invaluable tool to study the brains of rare, endangered, and “extinct in the wild” animals. Specimens preserved in brain banks, museums, or available from veterinary services after the natural death of zoological park specimens offer researchers a unique opportunity to delve into the neural architecture of species outside of current neuroanatomical models. (iii) Investigating large brains: some animals possess brains and bodies that often exceed the dimensions accommodated by current in-vivo imaging technology. One of the main challenges lies in the absence of coils large enough to adequately capture these dimensions from the animal’s head. Ex-vivo approaches bypass this limitation by allowing the study of large-brain species without spatial constraints, through extraction of the brain or section of the head. This capability is particularly advantageous to investigate the neural basis of complex cognitive abilities and behavioral repertoires in species with expansive brain sizes or extreme habitats, such as cetaceans (Gerussi et al., 2023) and elephants (Hakeem et al., 2005).
This review aims to highlight the indispensable role of ex-vivo DWI in studying the structural architecture of the brain. By employing this technique, researchers can uncover patterns of brain organization and connectivity, shedding light on evolutionary adaptations, behavioral complexities, and ecological dynamics. Ex-vivo DWI thus emerges as an essential tool integrating advanced imaging methodologies to potentiate ambitious comparative neuroanatomy, furthering our understanding of brain connectivity.
Assessing white matter connectivity
Fiber tractography algorithms use data from DWI scans to predict the pathways of white matter tracts based on the directional diffusion of water molecules. This method gives crucial insights into the brain’s structural connectivity, allowing researchers to investigate the structure and integrity of neuronal circuits. At the moment, there are two main physics-based approaches to modeling white matter diffusion anisotropy (i) diffusion tensor imaging (DTI) (Basser et al., 1994), and (ii) constrained spherical deconvolution (CSD) (Tournier et al., 2007). DTI analyses fiber orientation through voxel-wise evaluations (voxel refers to the smallest analytic unit), generating a singular tensor model that estimates a single three-dimensional orientation per voxel (Basser et al., 1994; Basser, 1995). This method demands minimal data points (a minimum of six independent measurements in different directions) for estimation, making it computationally efficient. While this approach is robust when there is a clear dominating diffusion direction within a voxel, it loses sensitivity when faced with multiple fiber pathways, such as crossing or branching fibers (Basser et al., 2000). In contrast, CSD as a tensor-free modeling approach, estimates the fiber orientation distribution (FOD) response function (Tournier et al., 2007, 2012) within each voxel, revealing the orientations and contributions of different fiber populations to observed diffusion behavior, and therefore does not assume isotropic diffusion (Farquharson et al., 2013). FOD does not lose information by averaging to obtain a single tensor, allowing for multiple fiber orientations to be identified. In contrast to the CSD, the simplified version of the Ball-and-Stick model proposed by Yang et al. (2019) offers notable advantages in terms of computing efficiency and implementation simplicity. While it is less intricate compared to CSD, this model operates effectively by splitting the diffusion-weighted MR signal within each voxel into multiple anisotropic components, representing different fiber orientations, along with a single isotropic component. This simplicity not only decreases processing needs but also allows for quicker implementation, making it especially helpful in cases where computational resources are restricted or swift analysis is necessary. The choice of method is determined by the individual research needs, the complexity of the fiber topologies under consideration, and the computational resources (Figure 1).
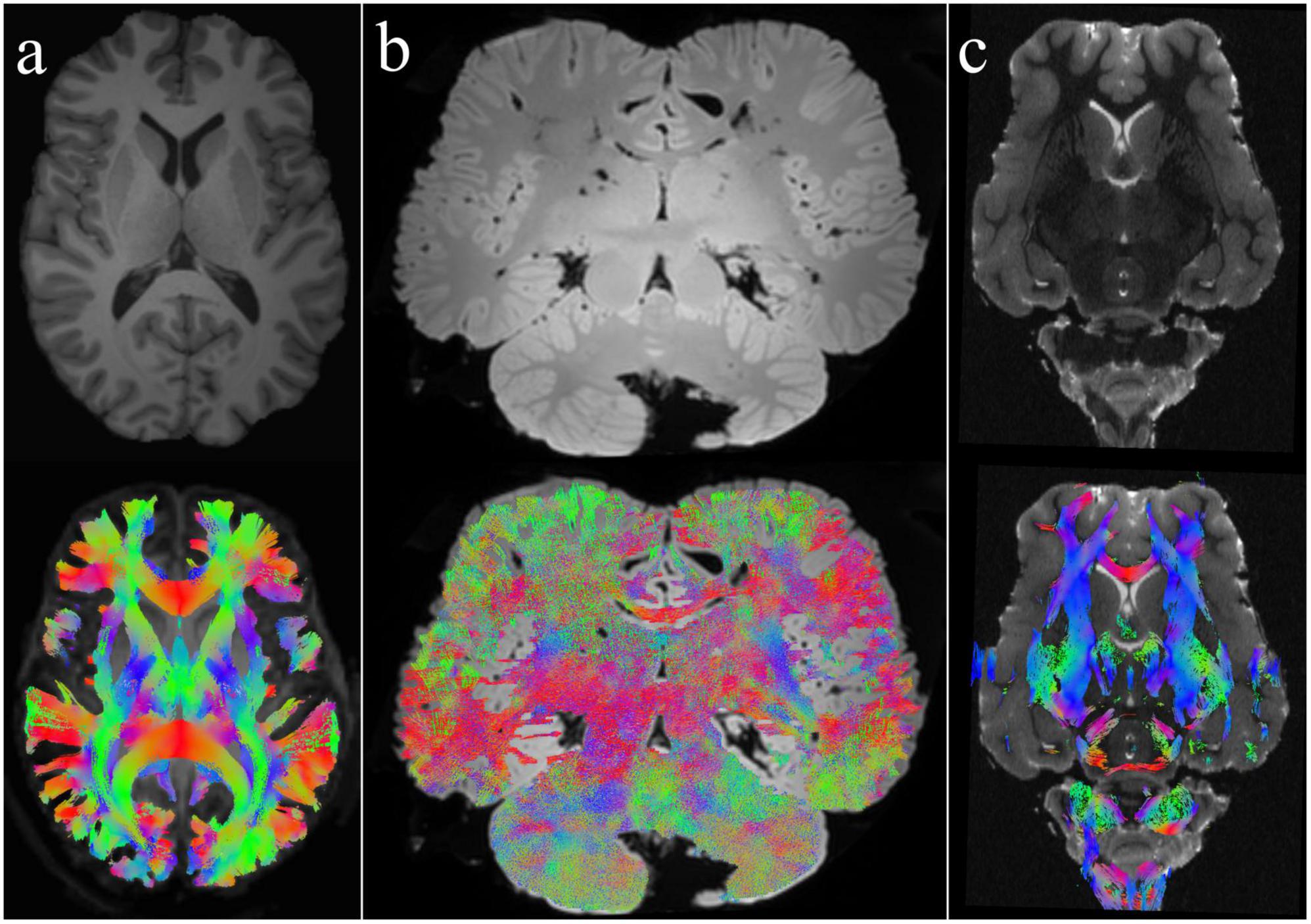
Figure 1. Whole-brain tractography in different species using CSD and DTI methods. (a) Human brain: This image represents the classical in-vivo T1-weighted structural image and the whole-brain tractography of a human brain shown here as a comparison with the other animals. The tractogram was created using CSD. (b) Bottlenose dolphin brain: Depicted here is an ex-vivo T2-weighted brain of a bottlenose dolphin along with its whole-brain tractogram, generated using the CSD method. (c) Sheep brain: The top image shows a T2-weighted anatomical view of an ex-vivo sheep brain, while the bottom image presents its corresponding whole-brain tractogram employing DTI. For more details, refer to Gerussi et al. (2022, 2023, 2024) and Graïc et al. (2023).
In this mini-review, we focused on physical-based diffusion models, specifically DTI and CSD, due to their established clinical use, computational efficiency, and robustness to noise (Tournier et al., 2007). These models require less stringent data acquisition parameters, making them suitable for studies with limited scanning time or lower magnetic field strengths. However, other algorithms have been developed to overcome the drawbacks of DTI (or CSD) such as Diffusion Spectrum Imaging (DSI) (Wedeen et al., 2012), Ball-and-Stick model (Yang et al., 2019), and Q-ball imaging (QBI) (Tuch, 2004). As the community seeks to gain a deeper knowledge of neuronal connections, it must look beyond DTI and tensor-free methods such as CSD to accurately model diffusion behavior furthering our understanding of brain anatomy and function. To this aim, we emphasize the potential of biophysical models like Neurite Orientation Dispersion and Density Imaging (NODDI) (Zhang H. et al., 2012) and Composite Hindered and Restricted Model of Diffusion (CHARMED) (Assaf and Basser, 2005) to provide more nuanced insights into tissue composition. However, their application is often constrained by the need for high-quality, multi-shell diffusion data, greater computational resources, and acquisition protocols that must support the model’s assumptions (Jelescu et al., 2020). We believe that future studies with access to advanced imaging modalities may benefit from incorporating biophysical models to further elucidate the complexities of neural tissue.
From DWI data, fiber pathways can be reconstructed using various models tailored to the research requirements and objectives, such as probabilistic vs. deterministic models or ROI analysis vs. whole-brain tractography [for details, see (Jeurissen et al., 2019; Zhang et al., 2022)]. Once fiber pathways have been reconstructed, quantitative assessment of specific tracts can reveal important insights into brain anatomy and function. This assessment requires examining several metrics generated from tractography data to evaluate parameters such as total tract volume, total length of reconstructed fiber tract, curvature, and connectivity strength. However, quantitative assessment of selected tracks using diffusion tensor metrics can provide information about white matter microstructural integrity. Fractional Anisotropy (FA) is a widely used parameter that measures the degree of directionality of water diffusion within a voxel and is sensitive to changes in white matter microstructure, such as myelination, crossing fiber orientation, and axonal density (Beaulieu, 2002). Furthermore, axial (AD) and radial (RD) diffusivities are the water diffusion coefficients parallel and perpendicular to the axons indicating axonal integrity and fiber myelination, respectively (Tournier et al., 2012). Furthermore, recent studies have demonstrated the potential of relaxometry parameters, such as effective transverse relaxation rate (R2*) and longitudinal relaxation rate (R1), as they provide additional information regarding tissue microstructure and composition. R2* is sensitive to iron concentration and myelin integrity (Straub et al., 2020), which are critical to the proper functioning of neural fibers. R1 values provide information on the longitudinal relaxation properties of tissue, which can indicate water content and macromolecule presence (Weiskopf et al., 2013). Understanding the fiber-specific relaxometry properties can offer enhanced specificity and sensitivity in differentiating the white matter architecture, especially in regions with crossing fibers (De Santis et al., 2016). Overall, a quantitative evaluation of selected tracts enables the characterization of white matter pathways in terms of size, shape, symmetry, and microstructural features, which is essential to understanding brain connections.
Tissue properties and preparation
Fixed samples are frequently employed in ex-vivo tissue imaging because they provide the longitudinal stability necessary for longer scanning sessions. There are significant factors and trade-offs to consider when scanning ex-vivo tissues compared to in-vivo.
While fixed tissue samples present certain challenges (see below), they offer the distinct advantage of temporal stability, facilitating time-dependent enhancements in image resolution that enable meticulous analysis of structures at the micron scale. Such precision would be nearly impossible to reach in current scanning times in-vivo. The absence of rhythmic blood flow, and virtually any movement allows for any sequence length to be performed to ideal standards, in as many diffusion directions as desired, optimizing results while reducing artifacts. This also implies that nearly identical scans can be made repeatedly over long periods of time, on more modern machines, in bores that would not fit a whole head, or after transport from a remote location. Once the sample has been extracted and fixed, it can be kept safely available in a bank for years without significant deterioration, thus providing any researcher with brains from species they would have no access to otherwise. To be also considered is the absence of a medical or veterinary care team to monitor anesthetized in-vivo subjects, or trained animals in rare cases (Andics and Miklósi, 2018), and therefore the absence of risk for them, the subject, or the machine. Even in the best case, most large species are very challenging to scan because of physical, medical, and time constraints. In practical terms, an ex-vivo specimen is brought to room temperature, scanned, and removed at will, depending on the scanning procedure, and not the other way around.
The main issues usually reported with ex-vivo scanning regard the post-mortem interval (PMI, the time elapsed between death and tissue fixation) and the correct fixation of the brain. Given that tissue fixation processes either halt or significantly slow down metabolic decay, it is crucial to consider the PMI. A fundamental difference between large brains and those of small animals lies in the methods of brain tissue fixation and preservation. In small, laboratory animals, perfusion fixation via pre-mortem intracardiac injection of fixative, in addition to immersion fixation post-tissue extraction, reduces PMI to essentially zero. Conversely, in large animals, usually not kept in laboratory conditions, post-mortem perfusion fixation via mechanical pumping of fixative through the cadaver, often utilizing the femoral artery in humans or the carotid arteries and jugular veins in animals, results in a poorer diffusion of the fixative due to post-mortem blood clotting, amounting to a longer PMI (McFadden et al., 2019). In most studies on human brains, a “good” PMI is considered < 24 h (Maranzano et al., 2020). Moreover, mechanical perfusion fixation is not commonly used in larger brains due to the difficulty in washing blood clots and the possibility of microscopic damage from pumping pressure. Consequently, large brains are typically fixed via immersion. However, immersion fixation proves less effective for large brains compared to small animals due to the prolonged duration required for passive diffusion of fixative over greater distances (Dawe et al., 2009), resulting in lower-quality fixation (Werner et al., 2000; Kasukurthi et al., 2009; Gage et al., 2012). An optimized fixation protocol, taking into account all scenarios, would require the shortest PMI possible, refrigeration (but absolutely no freezing), vascular clearing using iso-osmotic solutions, then fixative perfusion and immersion. The fixative volume should be 10 times the brain volume and changed 24 to 48 h after immersion. There should be clarity on the facts that paraformaldehyde is a solid, which forms formaldehyde (a gas) in solution, and that a 4% formaldehyde solution is equal to a 10% formalin solution, with the exception that formalin contains methanol (about 1% in a 10% formalin solution) to prevent precipitation into paraformaldehyde, but which can alter immunohistochemical properties of the tissue in the long term.
Nonetheless, the use of fixatives such as formalin or other formaldehyde solutions is known to alter tissue diffusion characteristics by promoting intermolecular cross-linking (Pfefferbaum et al., 2004; Shepherd et al., 2009). Furthermore, fixed samples frequently have lower proton density and shorter T1 and T2 relaxation times than living subjects, which can be attributed to the combined effects of tissue fixation, post-mortem changes, and a lower temperature (typically room temperature, approximately 23°C) than the body’s normal temperature (Roebroeck et al., 2019). The shortened T2 relaxation time and diffusivity of fixed tissue have a considerable impact on the contrast-to-noise ratio in DWI, which is critical for high-quality ex-vivo DWI. To provide appropriate contrast with reduced diffusivity, higher diffusion weighting is required. Increased diffusion weighting often necessitates longer echo times, making signal loss from T2 relaxation time a limiting issue, especially when short T2 causes the unweighted-diffusion signal to decay quickly. To keep a good SNR, a possible solution could be the use of optimized sequences with shorter echo times while still keeping long diffusion times such as stimulated echo-acquisition mode [STEAM, (Merboldt et al., 1991; Rane et al., 2010)] or sequences which accumulate magnetization over multiple repetition times still keeping strong diffusion weighting such as steady-state free precession [SSFP, (McNab and Miller, 2010)]. Fixation by immersion revealed a notable decrease in T1, T2 relaxation times, and diffusivity within post-mortem brain tissue. For instance, Shepherd et al. (2009) discovered that rat cortical slices immersion-fixed in 4% paraformaldehyde had a 21% decrease in T1 and an 81% decrease in T2 compared to fresh, unfixed post-mortem values (Shepherd et al., 2009). Similar results were seen in ex vivo human white matter, where T1 and T2 relaxation times were measured at 340 ms and 45 ms, respectively, at 3 Tesla. These findings suggest reductions of approximately 35% in T1 and 60% in T2 (McNab et al., 2009; Miller et al., 2012). These studies show that a significant percentage of T2 drop may be attributable simply to the fixation process, which is likely caused by a combination of dehydration and the presence of unbound fixative. The decrease in T1 is integral to tissue fixation as it is predominantly influenced by the size of molecules within the tissue environment and cannot be reversed during rehydration. This reduction is likely attributed to the cross-linking of proteins, which is irreversible (Tovi and Ericsson, 1992; Shepherd et al., 2009). However, prolonged washing in saline or phosphate-buffered saline (PBS) to remove fixative and rehydrate the specimen has been shown to fully recover T2 decreases to levels equivalent to fresh, unfixed post-mortem samples [but not to in-vivo levels (Shepherd et al., 2009)].
In terms of the reduction in diffusivity observed in fixed samples, animal studies have shown a significant decrease, with a 50% reduction in apparent diffusion coefficients (ADC) observed in unfixed ex vivo white matter (WM) and an 80% reduction in fixed WM compared to in-vivo values (D’Arceuil et al., 2007). Additionally, washing fixed tissue in PBS has been demonstrated to partially reverse these effects, with reported increases in WM ADC by approximately 30%. Similar findings were observed in ex-vivo human brains, with WM diffusivity reduced by approximately 85% compared to in-vivo values (McNab et al., 2009; Foxley et al., 2014). The decreased temperature of ex-vivo tissues during scanning is a crucial factor contributing to the overall reduction in diffusivity. Le Bihan (1996) previously illustrated that a drop in tissue temperature by 1°C corresponds to an expected 2.4% decrease in water diffusivity. However, recent research has indicated that even when temperature adjustments are made in a manner akin to Le Bihan’s technique, differences in diffusivity persist (Dyrby et al., 2011; Walker et al., 2019). Controlling these factors using for example 40°C PBS could help alleviate part of the issue.
It should be noted that all existing challenges do not invalidate the utility and need of fixed tissue samples for studies concerning precise tissue cytoarchitecture or white matter connectivity. On the contrary, we aim to highlight the potential translational significance of fixed tissue as diffusion imaging gains prominence in examining neural microstructure and connectivity. However, when ex-vivo DWI-MR studies are validated against histology or compared to in-vivo DWI, it is necessary to consider all the challenges mentioned earlier.
Application of ex-vivo large brain DWI
DWI is a neuroimaging tool with very high potential and yet little used in large brains, from domestic to wild animals. In the field of Veterinary Medicine, DWI has emerged as a valuable tool for neuropathological diagnosis in domestic animals, especially dogs and cats. It facilitates the identification of brain injuries after hypoxic lesions (Davis et al., 1994; McConnell et al., 2005; Garosi et al., 2006; Kang et al., 2009; Crawford et al., 2023), brain oedema (Zhao et al., 2006) and epileptic seizures (Hasegawa et al., 2003). Furthermore, it has been also used to investigate the normal brain anatomy and neural connectivity patterns in live cats and dogs, as well as postmortem examinations of their brains (Hasegawa et al., 2003; Ronen et al., 2003; Shaibani et al., 2006; Takahashi et al., 2010, 2011; Jacqmot et al., 2013, 2017, 2020; Gray-Edwards et al., 2014; Anaya García et al., 2015; Dai et al., 2016; Robinson et al., 2016; Das and Takahashi, 2018; Johnson et al., 2020; Andrews et al., 2022), pig (Conrad et al., 2012; Schmidt et al., 2012), bovine (Schmidt et al., 2009), and horses (Schmidt et al., 2019; Boucher et al., 2020).
In other domestic species, which emerged recently as models for translational medicine, DWI has also been adopted. It is the case of the sheep (Ovis aries) (Förschler et al., 2007; Lee et al., 2015; Peruffo et al., 2019; O’Connell et al., 2021; Pirone et al., 2021; Banstola and Reynolds, 2022; Gerussi et al., 2022; Graïc et al., 2023), the Göttingen (Knösche et al., 2015) and Yucatan (Wang et al., 2023) minipigs (Sus scrofa). It is worth noting that in these cases, most of the analyses were conducted using fixed brains. When compared to other laboratory animals, the brains of large ungulates such as horses, pigs, and domestic ruminants have received relatively little attention, despite their high availability following slaughter (Cozzi et al., 2020). In fact, these brains could potentially serve as a non-invasive source for the study of comparative and evolutionary neuroscience.
In the context of wild and exotic species, DWI analysis involves the study of both pathological conditions (Cook et al., 2018) and anatomical features (Cook et al., 2021; Cook and Berns, 2022) in pinnipeds but there are also investigations in one-humped camel (Camelus dromedarius) (Cartiaux et al., 2023) and dolphin brains (Berns et al., 2015; Wright et al., 2018; Orekhova et al., 2022; Gerussi et al., 2023, 2024). Table 1 summarizes the most important publications concerning the use of DWI in large brains.
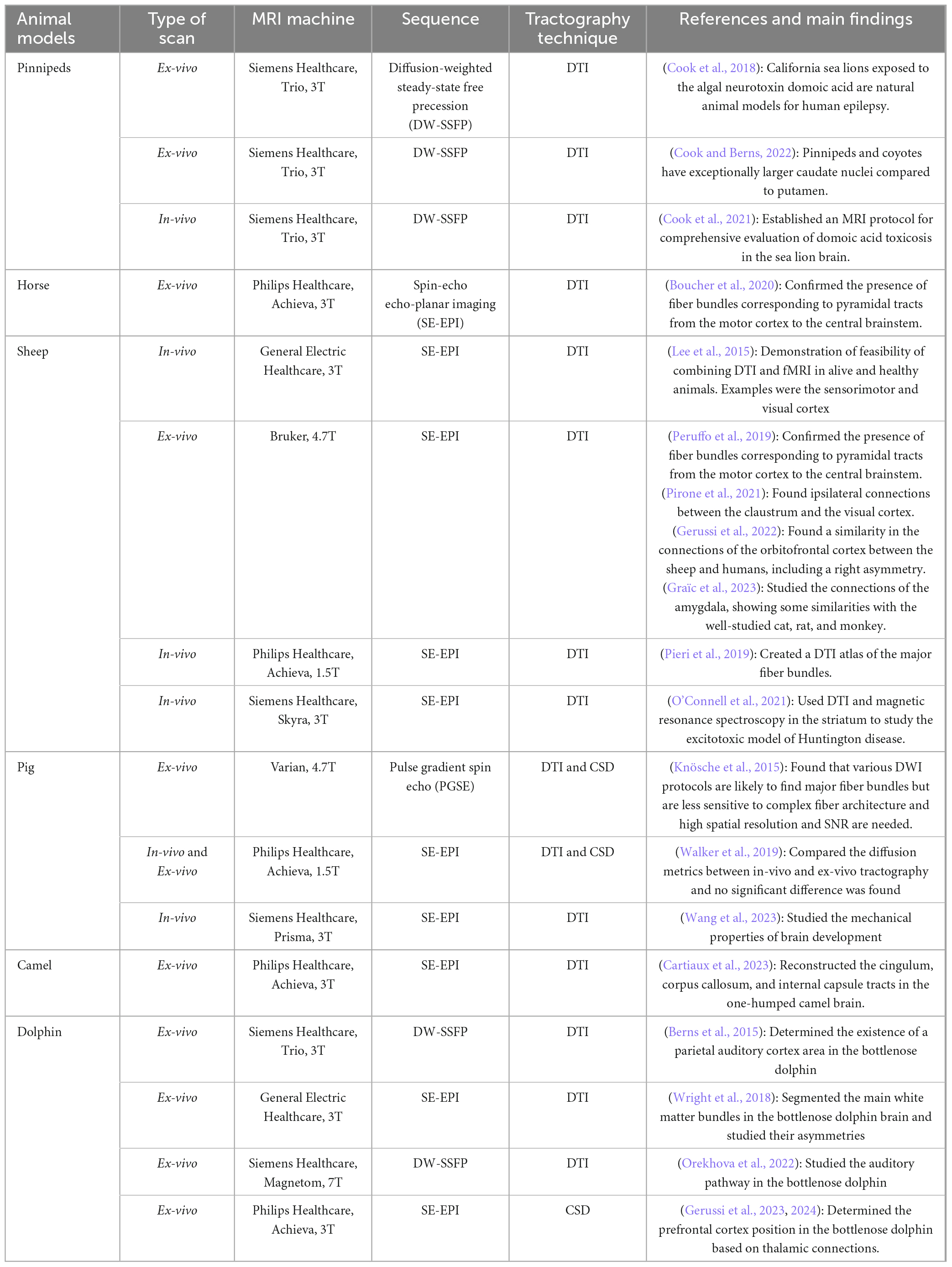
Table 1. Bibliographic references on DWI scans of large brains with correspondent MRI sequences and DWI algorithms used.
Discussion
MRI has established itself as an essential non-invasive technique in both neuroscientific research and clinical treatment (Assaf et al., 2019). The following development of DWI has expanded our understanding by digging into the microscopic characteristics of the white matter, allowing for the analysis of brain connections in both pathological and purely anatomico-physiological contexts using sophisticated tractography methods (Le Bihan, 1996). Clinical applications of DWI have not only transformed human medicine but have also proven beneficial in veterinary medicine, offering critical insights for neuropathological diagnoses in domestic animals (Hasegawa et al., 2003; Salma, 2015; Crawford et al., 2023). Furthermore, DWI has been effectively applied to domestic species often used in translational medicine, such as sheep and pigs, mostly demonstrating feasibility and setting standards for these species (Lee et al., 2015; Ella et al., 2019).
DWI, and consequently tractography, have merged as critical techniques for studying brain connections in large animals, including domestic and “wild” mammals such as dolphins or camels. Notably, DWI’s capacity to work in formalin-fixed tissues has broadened its application (D’Arceuil et al., 2007; Berns et al., 2015; Cook et al., 2018; Wright et al., 2018; Cartiaux et al., 2023; Gerussi et al., 2023, 2024; Graïc et al., 2023). Conducting invasive experiments and consequent euthanasia on these large, wild species are ethically nearly infeasible today. Furthermore, for the same reasons along with practical restrictions, potential in-vivo MRI in large species are extremely rare, and so far have not been published. As a result, ex-vivo imaging has emerged as the principal method for investigating the basis of complex cognitive abilities and behaviors in these species, providing outstanding neuroanatomical knowledge. Ex-vivo DWI also allows for significantly higher voxel resolutions maintaining a good SNR through adequate sequences such as STEM or SSFP, or re-hydration of the tissue with PBS, reaching scales of hundreds of micrometers. This level of resolution notably overcomes the limits of time-constrained in-vivo scans, allowing for an accurate mapping of brain structures and neural pathways.
This MRI technique has great potential for studying not only rare and endangered species but also extinct species whose unique specimens are currently fixed and preserved in museums or banks. Leveraging this option might allow the neuroscientific and broader scientific communities to investigate the evolution and development of brains across a wider range of taxa.
However, for DWI to give valid results, some factors must be considered. The first, and most important, is tissue fixation. There is no doubt that white matter integrity is central to any tract tracing algorithm (Beaulieu, 2002). In fact, the process of tissue fixation must happen as soon and swiftly as possible in order to block the autolytic mechanisms of the tissues which leads to the loss of integrity of the white matter. Fixation is a challenge and may not always be optimal in large animals for several factors such as the time between the death of the animal and awareness of park personnel (depending on the park, from minutes to several hours), the complexity of the vascular system supplying the brain (e.g., in cetaceans), the complex anatomy of the skull (such as that of rhinoceroses, elephants or giraffes) or ambient temperature (fast cooling of tissue seems to play a critical role in nervous tissue autolysis). All these factors are usually understood as a whole under the umbrella term of PMI, although wide variations can occur (e.g., a 24 h at 35°C ambient temperature is different in outcome from 24 h at 5°C), although a recent study seems to indicate minimal immunohistochemical effects (Koehler et al., 2024), and in details, PMI explains only part of the problems encountered in large brain fixation. For all these reasons, fixation by immersion seems to remain the most used alternative, as is the case in human brain preservation (Nardi et al., 2023). The fixative should be changed after the first 24 h, and a large volume is necessary (ideally 10 times the volume to be fixed). A topic still often discussed with confusion regards the type of fixative used. Finally, even after extraction, the size of some brains reaches ∼30 cm in diameter, and adapted coils may not exist.
The second main aspect to consider is the choice of DWI parameters. DTI, CSD, and other algorithms offer different approaches to analyze white matter diffusion anisotropy. Sequences used for DTI are fast, and do not require a high b-value or a high number of directions; however, DTI provides only a single 3D orientation per voxel and is, by definition, limited in the detection of multiple fiber populations within a voxel. On the other hand, sequences used for CSD require a long scan time with higher, multishelled b-values in a high number of directions. Comparatively, the CSD algorithm is able to identify multiple fiber orientations within a voxel without losing information, making it more robust for mapping complex fiber tracts. However, the DWI methods used for analysis must align with the research aims and objectives, fulfilling the specific requirements and questions posed by researchers, whether from a physics-based perspective or a biophysical-based perspective.
In conclusion, DWI and tractography have emerged as highly promising non-invasive MRI techniques for studying large animal brains. They offer remarkable insights into their complex and relatively understudied neuroanatomy and connectivity. Despite the challenges, drawbacks, and constraints, these tools represent a valuable addition to the limited toolbox available for enhancing our understanding of comparative brain anatomy, connections, and evolution.
Author contributions
MB: Conceptualization, Funding acquisition, Writing – original draft, Writing – review & editing. J-MG: Conceptualization, Writing – original draft, Writing – review & editing. TG: Conceptualization, Visualization, Writing – original draft, Writing – review & editing.
Funding
The authors declare that financial support was received for the research, authorship, and/or publication of this article. This work was supported by a grant from Deutsche Forschungsgemeinschaft (DFG, German Research Foundation)—Projektnummer 316803389—SFB 1280 (F02).
Acknowledgments
We would like to extend our heartfelt gratitude to FF and KM for their invaluable feedback and insightful suggestions, which significantly enhanced the quality of this study.
Conflict of interest
The authors declare that the research was conducted in the absence of any commercial or financial relationships that could be construed as a potential conflict of interest.
Publisher’s note
All claims expressed in this article are solely those of the authors and do not necessarily represent those of their affiliated organizations, or those of the publisher, the editors and the reviewers. Any product that may be evaluated in this article, or claim that may be made by its manufacturer, is not guaranteed or endorsed by the publisher.
References
Anaya García, M. S., Hernández Anaya, J. S., Marrufo Meléndez, O., Velázquez Ramírez, J. L., and Palacios Aguiar, R. (2015). In vivo study of cerebral white matter in the dog using diffusion tensor tractography. Vet. Radiol. Ultrasound 56, 188–195. doi: 10.1111/vru.12211
Andics, A., and Miklósi, Á (2018). Neural processes of vocal social perception: Dog-human comparative fMRI studies. Neurosci. Biobehav. Rev. 85, 54–64. doi: 10.1016/J.NEUBIOREV.2017.11.017
Andrews, E. F., Pascalau, R., Horowitz, A., Lawrence, G. M., and Johnson, P. J. (2022). Extensive connections of the canine olfactory pathway revealed by tractography and dissection. J. Neurosci. 42, 6392–6407. doi: 10.1523/JNEUROSCI.2355-21.2022
Assaf, Y., and Basser, P. J. (2005). Composite hindered and restricted model of diffusion (CHARMED) MR imaging of the human brain. Neuroimage 27, 48–58. doi: 10.1016/j.neuroimage.2005.03.042
Assaf, Y., Johansen-Berg, H., and Thiebaut de Schotten, M. (2019). The role of diffusion MRI in neuroscience. NMR Biomed. 32:62. doi: 10.1002/nbm.3762
Baliyan, V., Das, C. J., Sharma, R., and Gupta, A. K. (2016). Diffusion weighted imaging: Technique and applications. World J. Radiol. 8:785. doi: 10.4329/wjr.v8.i9.785
Banstola, A., and Reynolds, J. N. J. (2022). Mapping sheep to human brain: The need for a sheep brain atlas. Front. Vet. Sci. 9:961413. doi: 10.3389/fvets.2022.961413
Basser, P. J. (1995). Inferring microstructural features and the physiological state of tissues from diffusion-weighted images. NMR Biomed. 8, 333–344. doi: 10.1002/nbm.1940080707
Basser, P. J., Mattiello, J., and LeBihan, D. (1994). MR diffusion tensor spectroscopy and imaging. Biophys. J. 66, 259–267. doi: 10.1016/S0006-3495(94)80775-1
Basser, P. J., Pajevic, S., Pierpaoli, C., Duda, J., and Aldroubi, A. (2000). In vivo fiber tractography using DT-MRI data. Magn. Reson. Med. 44, 625–632. doi: 10.1002/1522-2594(200010)44:4<625::AID-MRM17>3.0.CO;2-O
Beaulieu, C. (2002). The basis of anisotropic water diffusion in the nervous system – A technical review. NMR Biomed. 15, 435–455. doi: 10.1002/nbm.782
Behroozi, M., Billings, B. K., Helluy, X., Manger, P. R., Güntürkün, O., and Ströckens, F. (2018). Functional MRI in the Nile crocodile: A new avenue for evolutionary neurobiology. Proc. R. Soc. B Biol. Sci. 285:20180178. doi: 10.1098/rspb.2018.0178
Behroozi, M., Helluy, X., Ströckens, F., Gao, M., Pusch, R., Tabrik, S., et al. (2020). Event-related functional MRI of awake behaving pigeons at 7T. Nat. Commun. 11, 1–12. doi: 10.1038/s41467-020-18437-1
Benjamin, P., Khan, F., and Mackinnon, A. D. (2017). The use of diffusion weighted imaging to evaluate pathology outside the brain parenchyma in neuroimaging studies. Br. J. Radiol. 90:821. doi: 10.1259/bjr.20160821
Berns, G. S., Cook, P. F., Foxley, S., Jbabdi, S., Miller, K. L., and Marino, L. (2015). Diffusion tensor imaging of dolphin brains reveals direct auditory pathway to temporal lobe. Proc. R. Soc. B Biol. Sci. 282:20151203. doi: 10.1098/rspb.2015.1203
Billings, B. K., Behroozi, M., Helluy, X., Bhagwandin, A., Manger, P. R., Güntürkün, O., et al. (2020). A three-dimensional digital atlas of the Nile crocodile (Crocodylus niloticus) forebrain. Brain Struct. Funct. 225:3. doi: 10.1007/s00429-020-02028-3
Boucher, S., Arribarat, G., Cartiaux, B., Lallemand, E. A., Péran, P., Deviers, A., et al. (2020). Diffusion tensor imaging tractography of white matter tracts in the equine brain. Front. Vet. Sci. 7:540253. doi: 10.3389/fvets.2020.00382
Cartiaux, B., Amara, A., Pailloux, N., Paumier, R., Malek, A., Elmehatli, K., et al. (2023). Diffusion tensor imaging tractography in the one-humped camel (Camelus dromedarius) brain. Front. Vet. Sci. 10:1231421. doi: 10.3389/fvets.2023.1231421
Conrad, M. S., Dilger, R. N., and Johnson, R. W. (2012). Brain growth of the domestic pig (Sus scrofa) from 2 to 24 weeks of age: A longitudinal MRI study. Dev. Neurosci. 34, 291–298. doi: 10.1159/000339311
Cook, P. F., and Berns, G. (2022). Volumetric and connectivity assessment of the caudate nucleus in California sea lions and coyotes. Anim. Cogn. 25, 1231–1240. doi: 10.1007/s10071-022-01685-7
Cook, P. F., Berns, G. S., Colegrove, K., Johnson, S., and Gulland, F. (2018). Postmortem DTI reveals altered hippocampal connectivity in wild sea lions diagnosed with chronic toxicosis from algal exposure. J. Comp. Neurol. 526, 216–228. doi: 10.1002/cne.24317
Cook, P. F., Hoard, V. A., Dolui, S., Frederick, B., de, B., Redfern, R., et al. (2021). An MRI protocol for anatomical and functional evaluation of the California sea lion brain. J. Neurosci. Methods 353:109097. doi: 10.1016/j.jneumeth.2021.109097
Cozzi, B., Bonfanti, L., Canali, E., and Minero, M. (2020). Brain waste: The neglect of animal brains. Front. Neuroanat. 14:573934. doi: 10.3389/fnana.2020.573934
Crawford, A. H., Beltran, E., Danciu, C. G., and Yaffy, D. (2023). Clinical presentation, diagnosis, treatment, and outcome in 8 dogs and 2 cats with global hypoxic-ischemic brain injury (2010-2022). J. Vet. Intern. Med. 37, 1428–1437. doi: 10.1111/jvim.16790
D’Arceuil, H. E., Westmoreland, S., and de Crespigny, A. J. (2007). An approach to high resolution diffusion tensor imaging in fixed primate brain. Neuroimage 35, 553–565. doi: 10.1016/j.neuroimage.2006.12.028
Dai, G., Das, A., Hayashi, E., Chen, Q., and Takahashi, E. (2016). Regional variation of white matter development in the cat brain revealed by ex vivo diffusion MR tractography. Int. J. Dev. Neurosci. 54, 32–38. doi: 10.1016/j.ijdevneu.2016.08.004
Das, A., and Takahashi, E. (2018). Characterization of white matter tracts by diffusion MR tractography in cat and ferret that have similar gyral patterns. Cereb. Cortex 28, 1338–1347. doi: 10.1093/cercor/bhx048
Davis, D., Ulatowski, J., Eleff, S., Izuta, M., Mori, S., Shungu, D., et al. (1994). Rapid monitoring of changes in water diffusion coefficients during reversible ischemia in cat and rat brain. Magn. Reson. Med. 31, 454–460. doi: 10.1002/mrm.1910310416
Dawe, R. J., Bennett, D. A., Schneider, J. A., Vasireddi, S. K., and Arfanakis, K. (2009). Postmortem MRI of human brain hemispheres: T2 relaxation times during formaldehyde fixation. Magn. Reson. Med. 61, 810–818. doi: 10.1002/mrm.21909
De Santis, S., Barazany, D., Jones, D. K., and Assaf, Y. (2016). Resolving relaxometry and diffusion properties within the same voxel in the presence of crossing fibres by combining inversion recovery and diffusion-weighted acquisitions. Magn. Reson. Med. 75, 372–380. doi: 10.1002/mrm.25644
Dyrby, T. B., Baaré, W. F. C., Alexander, D. C., Jelsing, J., Garde, E., and Søgaard, L. V. (2011). An ex vivo imaging pipeline for producing high-quality and high-resolution diffusion-weighted imaging datasets. Hum. Brain Mapp. 32, 544–563. doi: 10.1002/hbm.21043
Ella, A., Barrière, D. A., Adriaensen, H., Palmer, D. N., Melzer, T. R., Mitchell, N. L., et al. (2019). The development of brain magnetic resonance approaches in large animal models for preclinical research. Anim. Front. 9, 44–51. doi: 10.1093/af/vfz024
Farquharson, S., Tournier, J. D., Calamante, F., Fabinyi, G., Schneider-Kolsky, M., Jackson, G. D., et al. (2013). White matter fiber tractography: Why we need to move beyond DTI. J. Neurosurg. 118, 1367–1377. doi: 10.3171/2013.2.JNS121294
Förschler, A., Boltze, J., Waldmin, D., Gille, U., and Zimmer, C. (2007). MRI of experimental focal cerebral ischemia in sheep. Rofo 179, 516–524. doi: 10.1055/s-2007-962977
Foxley, S., Jbabdi, S., Clare, S., Lam, W., Ansorge, O., Douaud, G., et al. (2014). Improving diffusion-weighted imaging of post-mortem human brains: SSFP at 7T. Neuroimage 102, 579–589. doi: 10.1016/j.neuroimage.2014.08.014
Gage, G. J., Kipke, D. R., and Shain, W. (2012). Whole animal perfusion fixation for rodents. J. Vis. Exp. 65:3564. doi: 10.3791/3564
Garosi, L., McConnell, J. F., Platt, S. R., Barone, G., Baron, J. C., de Lahunta, A., et al. (2006). Clinical and topographic magnetic resonance characteristics of suspected brain infarction in 40 dogs. J. Vet. Intern. Med. 20, 311–321. doi: 10.1111/j.1939-1676.2006.tb02862.x
Gerussi, T., Graïc, J. M., Cozzi, B., Schlaffke, L., Güntürkün, O., and Behroozi, M. (2024). Constrained spherical deconvolution on diffusion-weighted images of dolphin brains. Magn. Reson. Imaging 108, 104–110. doi: 10.1016/j.mri.2024.02.002
Gerussi, T., Graïc, J. M., Grandis, A., Peruffo, A., and Cozzi, B. (2022). The orbitofrontal cortex of the sheep. Topography, organization, neurochemistry, digital tensor imaging and comparison with the chimpanzee and human. Brain Struct. Funct. 227, 1871–1891. doi: 10.1007/S00429-022-02479-W
Gerussi, T., Graïc, J. M., Peruffo, A., Behroozi, M., Schlaffke, L., Huggenberger, S., et al. (2023). The prefrontal cortex of the bottlenose dolphin (Tursiops truncatus Montagu, 1821): a tractography study and comparison with the human. Brain Struct. Funct. 228, 1963–1976. doi: 10.1007/s00429-023-02699-8
Graïc, J. M., Tagliavia, C., Salamanca, G., Gerussi, T., Grandis, A., Cozzi, B., et al. (2023). Connections of the sheep basolateral amygdala: A diffusion tensor imaging study. J. Neurosci. Methods 393:109883. doi: 10.1016/j.jneumeth.2023.109883
Gray-Edwards, H. L., Salibi, N., Josephson, E. M., Hudson, J. A., Cox, N. R., Randle, A. N., et al. (2014). High resolution MRI anatomy of the cat brain at 3 Tesla. J. Neurosci. Methods 227, 10–17. doi: 10.1016/J.JNEUMETH.2014.01.035
Hakeem, A. Y., Hof, P. R., Sherwood, C. C., Switzer, R. C., Rasmussen, L. E. L., and Allman, J. M. (2005). Brain of the African elephant (Loxodonta africana): Neuroanatomy from magnetic resonance images. Anat. Rec. A Discov. Mol. Cell. Evol. Biol. 287, 1117–1127. doi: 10.1002/ar.a.20255
Hamaide, J., Lukacova, K., Orije, J., Keliris, G. A., Verhoye, M., and van der Linden, A. (2020). In vivo assessment of the neural substrate linked with vocal imitation accuracy. Elife 9:e49941. doi: 10.7554/eLife.49941
Hasegawa, D., Orima, H., Fujita, M., Nakamura, S., Takahashi, K., Ohkubo, S., et al. (2003). Diffusion-weighted imaging in kainic acid-induced complex partial status epilepticus in dogs. Brain Res. 983, 115–127. doi: 10.1016/S0006-8993(03)03041-5
Jacqmot, O., Van Thielen, B., Fierens, Y., Hammond, M., Willekens, I., Schuerbeek, P., et al. (2013). Diffusion tensor imaging of white matter tracts in the dog brain. Anatom. Rec. 296, 340–349. doi: 10.1002/ar.22638
Jacqmot, O., Van Thielen, B., Michotte, A., de Mey, J., Provyn, S., and Tresignie, J. (2020). Neuroanatomical reconstruction of the canine visual pathway using diffusion tensor imaging. Front. Neuroanat. 14:558240. doi: 10.3389/FNANA.2020.00054/BIBTEX
Jacqmot, O., Van Thielen, B., Michotte, A., Willekens, I., Verhelle, F., Goossens, P., et al. (2017). Comparison of several white matter tracts in feline and canine brain by using magnetic resonance diffusion tensor imaging. Anatom. Rec. 300, 1270–1289. doi: 10.1002/ar.23579
Jelescu, I. O., Palombo, M., Bagnato, F., and Schilling, K. G. (2020). Challenges for biophysical modeling of microstructure. J. Neurosci. Methods 344:108861. doi: 10.1016/j.jneumeth.2020.108861
Jeurissen, B., Descoteaux, M., Mori, S., and Leemans, A. (2019). Diffusion MRI fiber tractography of the brain. NMR Biomed. 32:e3785. doi: 10.1002/nbm.3785
Johnson, P. J., Pascalau, R., Luh, W. M., Raj, A., Cerda-Gonzalez, S., and Barry, E. F. (2020). Stereotaxic diffusion tensor imaging white matter atlas for the in vivo domestic feline brain. Front. Neuroanat. 14:1. doi: 10.3389/fnana.2020.00001
Kang, J. E., Lim, M. M., Bateman, R. J., Lee, J. J., Smyth, L. P., Cirrito, J. R., et al. (2009). Amyloid-β dynamics are regulated by orexin and the sleep-wake cycle. Science 326, 1005–1007. doi: 10.1126/science.1180962
Kasukurthi, R., Brenner, M. J., Moore, A. M., Moradzadeh, A., Ray, W. Z., Santosa, K. B., et al. (2009). Transcardial perfusion versus immersion fixation for assessment of peripheral nerve regeneration. J. Neurosci. Methods 184, 303–309. doi: 10.1016/J.JNEUMETH.2009.08.019
Knösche, T. R., Anwander, A., Liptrot, M., and Dyrby, T. B. (2015). Validation of tractography: Comparison with manganese tracing. Hum. Brain Mapp. 36, 4116–4134. doi: 10.1002/hbm.22902
Koehler, J. W., Miller, A. D., and Rissi, D. R. (2024). Effects of autolysis and prolonged formalin fixation on histomorphology and immunohistochemistry of normal canine brain tissue: An experimental study. J. Vet. Diagn. Invest. 36, 169–176. doi: 10.1177/10406387231220649
Le Bihan, D. (1996). Diffusion, perfusion and functional mri: Functional MRI. Milano: Springer, 23–27. doi: 10.1007/978-88-470-2194-5_5
Lee, W., Lee, S. D., Park, M. Y., Foley, L., Purcell-Estabrook, E., Kim, H., et al. (2015). Functional and diffusion tensor magnetic resonance imaging of the sheep brain. BMC Vet. Res. 11:1–8. doi: 10.1186/s12917-015-0581-8
Makada, M., and Matang, M. (2023). Role of DWI in intracranial pathologies with its comparison to FLAIR and T2W imaging. Int. J. Acad. Med. Pharm. 5, 433–437. doi: 10.47009/jamp.2022.5.1.90
Mallon, D., Dixon, L., Campion, T., Dawe, G., Bhatia, K., Kachramanoglou, C., et al. (2020). Beyond the brain: Extra-axial pathology on diffusion weighted imaging in neuroimaging. J. Neurol. Sci. 415:900. doi: 10.1016/j.jns.2020.116900
Maranzano, J., Dadar, M., Bertrand-Grenier, A., Frigon, E. M., Pellerin, J., Plante, S., et al. (2020). A novel ex vivo, in situ method to study the human brain through MRI and histology. J. Neurosci. Methods 345:108903. doi: 10.1016/j.jneumeth.2020.108903
McConnell, J. F., Garosi, L., and Platt, S. R. (2005). Magnetic resonance imaging findings of presumed cerebellar cerebrovascular accident in twelve dogs. Vet. Radiol. Ultrasound 46, 1–10. doi: 10.1111/J.1740-8261.2005.00001.X
McFadden, W. C., Walsh, H., Richter, F., Soudant, C., Bryce, C. H., Hof, P. R., et al. (2019). Perfusion fixation in brain banking: A systematic review. Acta Neuropathol. Commun. 7:146. doi: 10.1186/s40478-019-0799-y
McNab, J. A., and Miller, K. L. (2010). Steady-state diffusion-weighted imaging: Theory, acquisition and analysis. NMR Biomed 23, 781–793. doi: 10.1002/nbm.1509
McNab, J. A., Jbabdi, S., Deoni, S. C. L., Douaud, G., Behrens, T. E. J., and Miller, K. L. (2009). High resolution diffusion-weighted imaging in fixed human brain using diffusion-weighted steady state free precession. Neuroimage 46, 775–785. doi: 10.1016/j.neuroimage.2009.01.008
Merboldt, K. D., Hänicke, W., and Frahm, J. (1991). Diffusion imaging using stimulated echoes. Magn. Reson. Med. 19, 233–239. doi: 10.1002/mrm.1910190208
Miller, K. L., McNab, J. A., Jbabdi, S., and Douaud, G. (2012). Diffusion tractography of post-mortem human brains: Optimization and comparison of spin echo and steady-state free precession techniques. Neuroimage 59, 2284–2297. doi: 10.1016/j.neuroimage.2011.09.054
Moseley, M. E., Kucharczyk, J., Asgari, H. S., and Norman, D. (1991). Anisotropy in diffusion-weighted MRI. Magn. Reson. Med. 19, 321–326. doi: 10.1002/mrm.1910190222
Mukherjee, P., Berman, J. I., Chung, S. W., Hess, C. P., and Henry, R. G. (2008). Diffusion tensor MR imaging and fiber tractography: Theoretic underpinnings. Am. J. Neuroradiol. 29, 632–641. doi: 10.3174/ajnr.A1051
Nardi, L., Schmeisser, M. J., and Schumann, S. (2023). Fixation and staining methods for macroscopical investigation of the brain. Front. Neuroanat. 17:1200196. doi: 10.3389/fnana.2023.1200196
O’Connell, A. B., Kuchel, T. R., Perumal, S. R., Sherwood, V., Neumann, D., Finnie, J. W., et al. (2021). Longitudinal magnetic resonance spectroscopy and diffusion tensor imaging in sheep (Ovis aries) with quinolinic acid lesions of the striatum: Time-dependent recovery of N-acetylaspartate and fractional anisotropy. J. Neuropathol. Exp. Neurol. 79, 1084–1092. doi: 10.1093/JNEN/NLAA053
Orekhova, K., Selmanovic, E., De Gasperi, R., Gama Sosa, M. A., Wicinski, B., Maloney, B., et al. (2022). Multimodal assessment of bottlenose dolphin auditory nuclei using 7-tesla MRI, immunohistochemistry and stereology. Vet. Sci. 9:692. doi: 10.3390/vetsci9120692
Orije, J., Cardon, E., Hamaide, J., Jonckers, E., Darras, V. M., Verhoye, M., et al. (2021). Uncovering a ‘sensitive window’ of multisensory and motor neuroplasticity in the cerebrum and cerebellum of male and female starlings. Elife 10:e66777. doi: 10.7554/eLife.66777
Peruffo, A., Corain, L., Bombardi, C., Centelleghe, C., Grisan, E., Graïc, J. M., et al. (2019). The motor cortex of the sheep: Laminar organization, projections and diffusion tensor imaging of the intracranial pyramidal and extrapyramidal tracts. Brain Struct. Funct. 224, 1933–1946. doi: 10.1007/s00429-019-01885-x
Pfefferbaum, A., Sullivan, E. V., Adalsteinsson, E., Garrick, T., and Harper, C. (2004). Postmortem MR imaging of formalin-fixed human brain. Neuroimage 21, 1585–1595. doi: 10.1016/j.neuroimage.2003.11.024
Pieri, V., Trovatelli, M., Cadioli, M., Zani, D. D., Brizzola, S., Ravasio, G., et al. (2019). In vivo diffusion tensor magnetic resonance tractography of the sheep brain: An atlas of the ovine white matter fiber bundles. Front. Vet. Sci. 6:485368. doi: 10.3389/fvets.2019.00345
Pirone, A., Graïc, J. M., Grisan, E., and Cozzi, B. (2021). The claustrum of the sheep and its connections to the visual cortex. J. Anat. 238, 1–12. doi: 10.1111/joa.13302
Rane, S., Nair, G., and Duong, T. Q. (2010). DTI at long diffusion time improves fiber tracking. NMR Biomed. 23, 459–465. doi: 10.1002/nbm.1482
Robinson, J. L., Baxi, M., Katz, J. S., Waggoner, P., Beyers, R., Morrison, E., et al. (2016). Characterization of structural connectivity of the default mode network in dogs using diffusion tensor imaging. Sci. Rep. 6, 1–7. doi: 10.1038/srep36851
Roebroeck, A., Miller, K. L., and Aggarwal, M. (2019). Ex vivo diffusion MRI of the human brain: Technical challenges and recent advances. NMR Biomed. 32:e3941. doi: 10.1002/nbm.3941
Ronen, I., Kim, K. H., Garwood, M., Ugurbil, K., and Kim, D. S. (2003). Conventional DTI vs. slow and fast diffusion tensors in cat visual cortex. Magn. Reson. Med. 49, 785–790. doi: 10.1002/mrm.10431
Russo, G., Helluy, X., Behroozi, M., and Manahan-Vaughan, D. (2021). Gradual restraint habituation for awake functional magnetic resonance imaging combined with a sparse imaging paradigm reduces motion artifacts and stress levels in rodents. Front. Neurosci. 15:805679. doi: 10.3389/fnins.2021.805679
Schmidt, M. J., Knemeyer, C., and Heinsen, H. (2019). Neuroanatomy of the equine brain as revealed by high-field (3Tesla) magnetic-resonance-imaging. PLoS One 14:e0213814. doi: 10.1371/journal.pone.0213814
Schmidt, M. J., Langen, N., Klumpp, S., Nasirimanesh, F., Shirvanchi, P., Ondreka, N., et al. (2012). A study of the comparative anatomy of the brain of domestic ruminants using magnetic resonance imaging. Vet. J. 191, 85–93. doi: 10.1016/j.tvjl.2010.12.026
Schmidt, M. J., Pilatus, U., Wigger, A., Kramer, M., and Oelschläger, H. A. (2009). Neuroanatomy of the calf brain as revealed by high-resolution magnetic resonance imaging. J. Morphol. 270, 745–758. doi: 10.1002/jmor.10717
Shaibani, A., Khawar, S., Shin, W., Cashen, T. A., Schirf, B., Rohany, M., et al. (2006). First results in an MR imaging-compatible canine model of acute stroke. Am. J. Neuroradiol. 27, 1788–1793.
Shepherd, T. M., Thelwall, P. E., Stanisz, G. J., and Blackband, S. J. (2009). Aldehyde fixative solutions alter the water relaxation and diffusion properties of nervous tissue. Magn. Reson. Med. 62, 26–34. doi: 10.1002/mrm.21977
Straub, S., Mangesius, S., Emmerich, J., Indelicato, E., Nachbauer, W., Degenhardt, K. S., et al. (2020). Toward quantitative neuroimaging biomarkers for Friedreich’s ataxia at 7 Tesla: Susceptibility mapping, diffusion imaging, R2 and R1 relaxometry. J. Neurosci. Res. 98, 2219–2231. doi: 10.1002/jnr.24701
Takahashi, E., Dai, G., Rosen, G. D., Wang, R., Ohki, K., Folkerth, R. D., et al. (2011). Developing neocortex organization and connectivity in cats revealed by direct correlation of diffusion tractography and histology. Cereb. Cortex 21, 200–211. doi: 10.1093/cercor/bhq084
Takahashi, E., Dai, G., Wang, R., Ohki, K., Rosen, G. D., Galaburda, A. M., et al. (2010). Development of cerebral fiber pathways in cats revealed by diffusion spectrum imaging. Neuroimage 49, 1231–1240. doi: 10.1016/j.neuroimage.2009.09.002
Tournier, J. D., Calamante, F., and Connelly, A. (2007). Robust determination of the fibre orientation distribution in diffusion MRI: Non-negativity constrained super-resolved spherical deconvolution. Neuroimage 35, 1459–1472. doi: 10.1016/j.neuroimage.2007.02.016
Tournier, J. D., Calamante, F., and Connelly, A. (2012). MRtrix: Diffusion tractography in crossing fiber regions. Int. J. Imaging Syst. Technol. 22, 53–66. doi: 10.1002/ima.22005
Tovi, M., and Ericsson, A. (1992). Measurements of T1 and T2 over time in formalin-fixed human whole-brain specimens. Acta Radiol. 33, 400–404. doi: 10.1177/028418519203300503
Walker, M. R., Zhong, J., Waspe, A. C., Looi, T., Piorkowska, K., Drake, J. M., et al. (2019). Acute ex vivo changes in brain white matter diffusion tensor metrics. PLoS One 14:e0223211. doi: 10.1371/journal.pone.0223211
Wang, S., Guertler, C. A., Okamoto, R. J., Johnson, C. L., McGarry, M. D. J., and Bayly, P. V. (2023). Mechanical stiffness and anisotropy measured by MRE during brain development in the minipig. Neuroimage 277:120234. doi: 10.1016/j.neuroimage.2023.120234
Wedeen, V. J., Rosene, D. L., Wang, R., Dai, G., Mortazavi, F., Hagmann, P., et al. (2012). The geometric structure of the brain fiber pathways. Science 335, 1628–1634. doi: 10.1126/science.1215280
Weiskopf, N., Suckling, J., Williams, G., Correia, M., Inkster, B., Tait, R., et al. (2013). Quantitative multi-parameter mapping of R1, PD*, MT, and R2* at 3T: A multi-center validation. Front. Neurosci. 7:46379. doi: 10.3389/fnins.2013.00095
Werner, M., Chott, A., Fabiano, A., and Battifora, H. (2000). Effect of formalin tissue fixation and processing on immunohistochemistry. Am. J. Surg. Pathol. 24, 1016–1019. doi: 10.1097/00000478-200007000-00014
Wright, A. K., Theilmann, R. J., Ridgway, S. H., and Scadeng, M. (2018). Diffusion tractography reveals pervasive asymmetry of cerebral white matter tracts in the bottlenose dolphin (Tursiops truncatus). Brain Struct. Funct. 223, 1697–1711. doi: 10.1007/s00429-017-1525-9
Yang, S., Ghosh, K., Sakaie, K., Sahoo, S. S., Ann Carr, S. J., and Tatsuoka, C. (2019). A simplified crossing fiber model in diffusion weighted imaging. Front. Neurosci. 13:430469. doi: 10.3389/fnins.2019.00492
Zhang, F., Daducci, A., He, Y., Schiavi, S., Seguin, C., Smith, R. E., et al. (2022). Quantitative mapping of the brain’s structural connectivity using diffusion MRI tractography: A review. Neuroimage 249:118870. doi: 10.1016/j.neuroimage.2021.118870
Zhang, J., Aggarwal, M., and Mori, S. (2012). Structural insights into the rodent CNS via diffusion tensor imaging. Trends Neurosci. 35, 412–421. doi: 10.1016/j.tins.2012.04.010
Zhang, H., Schneider, T., Wheeler-Kingshott, C. A., and Alexander, D. C. (2012). NODDI: Practical in vivo neurite orientation dispersion and density imaging of the human brain. Neuroimage 61, 1000–1016. doi: 10.1016/j.neuroimage.2012.03.072
Keywords: DWI, DTI, CSD, tractography, large brain, post-mortem imaging
Citation: Behroozi M, Graïc J-M and Gerussi T (2024) Beyond the surface: how ex-vivo diffusion-weighted imaging reveals large animal brain microstructure and connectivity. Front. Neurosci. 18:1411982. doi: 10.3389/fnins.2024.1411982
Received: 03 April 2024; Accepted: 12 June 2024;
Published: 26 June 2024.
Edited by:
Bramsh Qamar Chandio, University of Southern California, United StatesReviewed by:
Francisco J. Fritz, University Medical Center Hamburg-Eppendorf, GermanyKulam Najmudeen Magdoom, Eunice Kennedy Shriver National Institute of Child Health and Human Development (NIH), United States
Copyright © 2024 Behroozi, Graïc and Gerussi. This is an open-access article distributed under the terms of the Creative Commons Attribution License (CC BY). The use, distribution or reproduction in other forums is permitted, provided the original author(s) and the copyright owner(s) are credited and that the original publication in this journal is cited, in accordance with accepted academic practice. No use, distribution or reproduction is permitted which does not comply with these terms.
*Correspondence: Mehdi Behroozi, mehdi.behroozi@ruhr-uni-bochum.de; Tommaso Gerussi, tommaso.gerussi@phd.unipd.it; tgerussi@cityu.edu.hk
†ORCID: Mehdi Behroozi, orcid.org/0000-0001-8373-6168; Jean-Marie Graïc, orcid.org/0000-0002-1974-8356; Tommaso Gerussi, orcid.org/0000-0003-0263-5635