- 1Department of Otorhinolaryngology Head and Neck Surgery, Key Laboratory of Otolaryngology Head and Neck Surgery (Capital Medical University), Ministry of Education, Beijing Tongren Hospital, Capital Medical University, Beijing, China
- 2Beijing Key Laboratory of Fundamental Research on Biomechanics in Clinical Application, School of Biomedical Engineering, Capital Medical University, Beijing, China
- 3Beijing Institute of Otolaryngology, Otolaryngology-Head and Neck Surgery, Key Laboratory of Otolaryngology Head and Neck Surgery (Capital Medical University), Ministry of Education, Beijing Tongren Hospital, Capital Medical University, Beijing, China
- 4Sonova Shanghai Co., Ltd., Shanghai, China
- 5Department of Otolaryngology Head and Neck Surgery, Peking University People’s Hospital, Beijing, China
Introduction: Noise reduction (NR) algorithms have been integrated into modern digital hearing aids to reduce noise annoyance and enhance speech intelligibility. This study aimed to evaluate the influences of a novel hearing aid NR algorithm on individuals with severe-to-profound hearing loss.
Methods: Twenty-five participants with severe-to-profound bilateral sensorineural hearing loss underwent three tests (speech intelligibility, listening effort, and subjective sound quality in noise) to investigate the influences of NR. All three tests were performed under three NR strength levels (Off, Moderate, and Strong) for both speech in noise program (SpiN) and speech in loud noise program (SpiLN), comprising six different hearing aid conditions.
Results: NR activation significantly reduced listening effort. Subjective sound quality assessments also exhibited benefits of activated NR in terms of noise suppression, listening comfort, satisfaction, and speech clarity.
Discussion: Individuals with severe-to-profound hearing loss still experienced advantages from NR technology in both listening effort measure and subjective sound quality assessments. Importantly, these benefits did not adversely affect speech intelligibility.
Introduction
Severe-to-profound hearing loss patients (estimated at 87 million worldwide) may face challenges related to social participation, health complications, work or study limitations, and decline of overall life quality (Stevens et al., 2013). Several studies have found that adults with severe and profound hearing loss experienced higher levels of social isolation, anxiety, and depression compared to peers with better hearing (Grimby and Ringdahl, 2000; Hallam et al., 2006). Besides social support networks from friends and family, hearing healthcare plays a crucial role in the lives of hearing loss individuals, which provides support and effective communication tools to avoid poor health outcomes and social isolation (Carlsson et al., 2015).
If we adopt the definition of severe hearing loss as an average air-conduction threshold of above 60 dB HL across 0.25–4 kHz, the prevalence is approximately 2.5% of the population (Goman and Lin, 2016). Taking a more conservative approach by pushing up the cutoff value to 70 dB HL, the estimated prevalence decreases to 0.7% of the population (Turton and Smith, 2013; Carlsson et al., 2015). One study showed that out of 4,286 patients with severe-to-profound hearing loss, 1,323 (31%) used a unilateral hearing aid in either the right or left ear, 2,403 (58.5%) used bilateral hearing aids, totaling 3,726 (87%) participants using either unilateral or bilateral hearing aids (Turunen-Taheri et al., 2019).
The present study follows the guideline of WHO by defining severe hearing loss as an average air-conduction threshold of above 65 dB HL across 0.25–4 kHz (World Health Organization, 2021; Lin, 2024). Individuals with severe-to-profound hearing loss are often long-term, full-day users of hearing aids and heavily relying on them due to the extent of their hearing loss. Their amplification needs are unique: individuals in this group require a wide range of input levels to be audible, comfortable, and safe within their narrow residual hearing range (Convery and Keidser, 2011). Severe-to-profound hearing loss is one of the most challenging auditory problems, as affected individuals may struggle to communicate in daily life and may not hear or barely hear conversational-level speech without assistive hearing devices. Even with properly fitted hearing aids that can improve hearing, people with severe-to-profound hearing loss may still experience difficulties in understanding speech, especially in noisy environments (Flynn et al., 1998).
Hearing loss affects not only the audibility of sound but also the quality of sound perception (Moore, 1996). Among hearing aid users, one of the most common complaints is difficulty experienced when listening in noisy environments. To address this issue, noise reduction (NR) algorithms are integrated into modern digital hearing aids to alleviate noise annoyance and enhance speech intelligibility (Brons et al., 2013). These NR algorithms continuously analyze the input signal, estimate the signal-to-noise ratio (SNR), and selectively attenuate the gain in frequency regions dominated by noise to increase SNR. This improvement in SNR aims to facilitate better speech intelligibility in noise.
However, the efficacy of NR has yielded mixed results in studies that have utilized different variables, tools, and methodologies (Bentler et al., 2008; Brons et al., 2013, 2014, 2015; Ohlenforst et al., 2018; Wong et al., 2018). It’s worth noting that these studies primarily focused on participants with normal hearing (Brons et al., 2013), or those with mild to moderate hearing loss (Bentler et al., 2008; Brons et al., 2014, 2015; Ohlenforst et al., 2018; Wong et al., 2018). The impact of NR on individuals with severe-to-profound hearing loss remains unclear.
Many studies assessed the benefits of NR via speech intelligibility test. Results have exhibited wide variation, with some studies reporting no effect on speech intelligibility (Bentler et al., 2008; Brons et al., 2013, 2014; Desjardins and Doherty, 2014; Brons et al., 2015; Desjardins, 2016), but others showed significant improvement (Peeters et al., 2009; Wong et al., 2018). Besides, a subset of studies also raised concerns about potential adverse effects associated with NR processing on intelligibility (Nordrum et al., 2006; Bentler et al., 2008). These studies suggested that although background noise could be attenuated, there might also be unintended consequence of speech distortion. More aggressive signal processing might introduce more speech enhancement as well as more distortions (Loizou and Kim, 2011).
In summary, no consistent benefits of NR application in speech understanding are observed in literature so far. Recent studies have incorporated measures of listening effort, which appear to capture subtler NR influences that may be advantageous to listeners (Sarampalis et al., 2009; Brons et al., 2013; Desjardins and Doherty, 2014; Ohlenforst et al., 2017a,b, 2018). NR is designed to improve speech audibility and intelligibility in both quiet and noisy conditions, potentially reducing the listening effort.
Objective assessment of listening effort has involved physiological measurements, such as pupil response and electroencephalography (EEG; Neher et al., 2014; Ohlenforst et al., 2018; Fiedler et al., 2021). For instance, Wendt et al. (2017) investigated the effect of intelligibility level and NR schemes on listening effort as indicated by the peak pupil dilation (PPD) in a group of people with hearing impairment. The results indicated that processing effort and recognition performance were affected by both intelligibility level and NR scheme (NoNR vs. NR). Increased PPD was observed for SNR corresponding to the individual 50% correct (L50) compared to SNR corresponding to the individual 95% correct (L90), suggesting increased listening effort in L50. The application of an NR scheme resulted in reduced listening effort as indicated by smaller PPDs.
Subjective assessment of listening effort included self-reporting and questionnaire surveys, such as the Speech, Spatial and Qualities of Hearing Scale (SSQ; Gatehouse and Noble, 2004), categorical scales (Alhanbali et al., 2017; Ferschneider and Moulin, 2023), and behavioral tests like single-task or dual-task paradigms (Sarampalis et al., 2009; Neher et al., 2014; Degeest et al., 2021). Baer et al. (1993) suggested that the primary benefit of hearing aid NR might be a reduction in listening effort rather than a direct enhancement of speech clarity. Research has indicated that signal processing of modern hearing aids has the potential to reduce listening effort (Brons et al., 2014; Ohlenforst et al., 2017b; Wong et al., 2018).
Previous studies have assessed subjective listening effort under fixed conditions like SNRs (Zekveld et al., 2011; Ohlenforst et al., 2017b, 2018). However, recent research has indicated that listening effort may not correlate monotonically with task demands. Variations in effort follow an inverted U-shaped curve, suggesting that listeners may “give up” when challenged and expend less effort at lower SNRs (Ohlenforst et al., 2017a). Studies showed listeners with normal hearing and impairment hearing may allocate different auditory resources for speech understanding (Zekveld et al., 2011; Ohlenforst et al., 2017a,b).
Knowledge about the effects of NR influences on listening effort for individuals with severe-to-profound hearing loss remains limited. Unlike mild and moderate hearing loss, the abilities of individuals with severe-to-profound hearing loss do not show a clear correlation with the degree of hearing loss. In the case of severe hearing loss, speech recognition in quiet was associated with multiple factors, including the degree of hearing loss, spectral resolution and the presence of dead regions. However, for speech in noise, the variance in performance was only modestly explained by amount of hearing loss (Souza et al., 2018).
The purpose of the present study was to assess aided speech recognition, listening effort, and sound quality ratings in noise conditions with and without NR, implemented within a commercially available hearing aid for adults with severe-to-profound hearing loss.
Materials and methods
Participants
The sample size was computed based on the measure of subjective listening effort. Power analysis was performed using R software (version 4.3.1) based on the simulation of the data from five pilot participants. A linear mixed effects model was fitted to the data and the mixed power function from the mixedPower package (Kumle et al., 2021) was applied to estimate power for different sample size. The simulation was based on 1,000 runs with a varying sample size from 18 to 26. To ensure that a smaller significant effect size can also be detected, a new parameter SESOI (smallest effect size of interest) was added to the mixedPower function. The simulation results were based on the dataset as well as the defined estimates at the same time. Results suggested that a sample size of 20 subjects should be sufficient with a power over 0.8.
Twenty-five Mandarin-speaking adults (9 males and 16 females) aged between 26 and 77 years old (M = 46.5, SD = 18.4) were recruited. All participants were diagnosed with sloping severe-to-profound hearing loss. The average pure-tone audiometric thresholds between 500 and 4,000 Hz for both ears were above 65 dB HL. Figure 1 shows the group average pure-tone thresholds with standard deviation. All participants met the following inclusion criteria: (1) symmetric sloping sensorineural hearing loss (i.e., interaural difference ≤ 15 dB at all octave frequencies from 250 to 8,000 Hz) with air-bone gaps at each frequency ≤ 15 dB; (2) normal middle ear function as indicated by tympanometry and otoscopy examinations; (3) normal cognitive ability screening with MoCA-basic version of Chinses (passing score: 23/30; Chen et al., 2016). (4) At least 1 year hearing aid usage. (5) native Mandarin speakers in daily life. Among the 25 participants, 17 used Phonak brand hearing aids, 4 used Oticon, 2 used Resound, and 2 used Starkey.
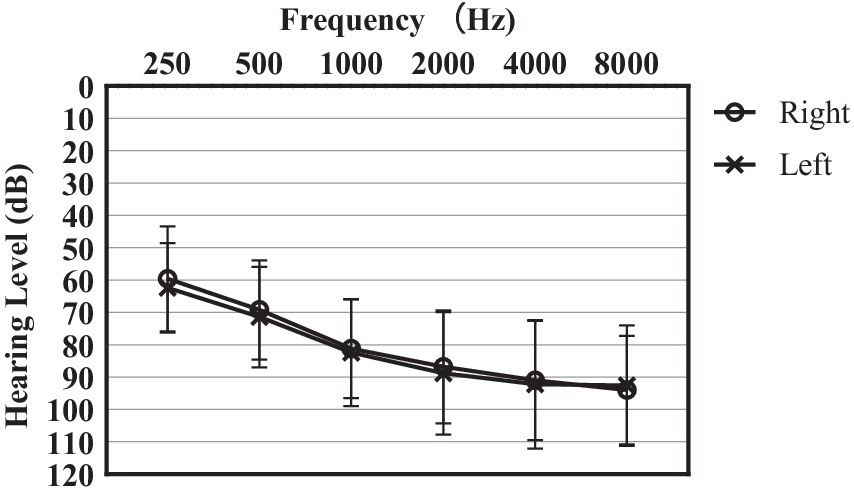
Figure 1. The average air-conduction hearing thresholds of study participants. Error bars show the standard deviations.
Hearing aids fitting
During the study, each participant wore a pair of Phonak (Stäfa, Switzerland) Naida Paradise 90 UP hearing aids programmed in Phonak Target fitting software (v. 7.1) with Noahlink Wireless. To ensure proper amplification of sound and to limit potential acoustic feedback, custom hard silicone ear molds were made, and the vent size was selected based on the recommendation of the fitting software. The feedback measurement was then performed using the “Real ear and feedback measurement” module in the Phonak Target fitting software to ensure that there was no feedback problem or gain limitation. The APD-Contrast (Adaptive Phonak Digital Contrast) 2.0 fitting prescription was chosen as the target. The initial gain level was set to 100%. Fine-tuning was accomplished by playing a practice list of CMNmatrix (Hu et al., 2018) sentences at 65 dBA SPL from a speaker 1 meter in front of the participant. If the loudness was not appropriate, the broadband frequency gain for the 65 dB SPL input level was adjusted until the participant was satisfied. If participants reported an occlusion problem, the “Occlusion Compensation” feature was turned on and adjusted according to their feedback. To avoid touching by mistake, the button function for volume adjustment was disabled. For tests with speech in noise (SpiN) program, three manual programs based on SpiN were created. These three manual programs differ only in NR strength, namely off, moderate (12) and strong (20) accordingly. For tests with speech in loud noise (SpiLN) program, the same three manual programs were created similarly. These two different base programs were tested in separate appointments. Except from NR setting, the other advanced features such as noise reduction, directionality, etc. were kept the same within each base program.
The NR function
The NR algorithm used in this study (Dynamic Noise Cancelation, DNC) is a spatial noise reduction feature that works in combination with a directional beamformer to improve the signal-to-noise ratio in challenging situations. It is microphone dependent and is only activated when the beamformer is fully activated and switches off as soon as the SNR reaches 18 dB and higher. NR can be adjusted from off to weak, moderate and strong levels in Phonak Target and in myPhonak APP. In Target, the NR appears on a 20-point slider: 0 is off, 1–9 is weak, 10–16 is moderate and 17–20 is strong. A stronger NR setting leads to higher attenuation of noise, which in turn provides a higher SNR benefit (Figure 2).
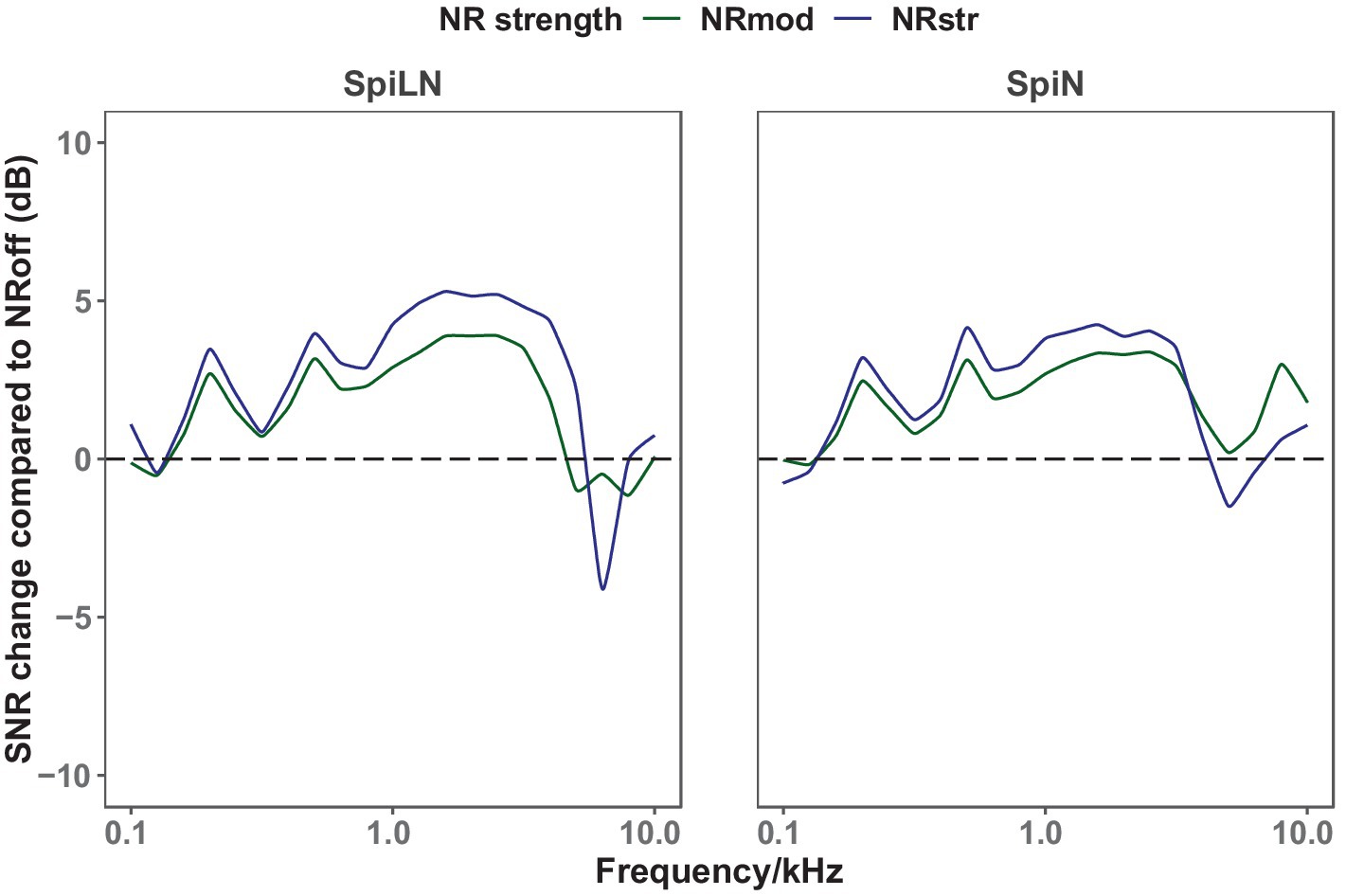
Figure 2. Increased SNR over frequency range of 0.1–10 kHz for NRmod and NRstr compared to NRoff. The measurement was performed using Hagerman and Olofsson (2004) phase invert method. The test setup was same as speech recognition test in Figure 3.
Perceptual tasks and outcome measures
Speech recognition in noise
The speech reception thresholds (SRTs) were measured adaptively by open-set Mandarin Chinese matrix (CMNmatrix) Sentence Test (Hu et al., 2018). The CMNmatrix sentence contains five words (name-verb-numeral-adjective-noun). There are 10 possible alternatives for each word category, which are randomly combined into syntactically fixed, grammatically correct but semantically unpredictable sentences. During the test, participants responded by repeating what they heard, and the investigator outside of the sound booth marked the correct answers. The SRT was defined as the signal-to-noise ratio at which a listener yielded 50% speech intelligibility.
Test setup of the sound field is shown in Figure 3. The target sentences were presented from 0° azimuth in front of the participant with competing noise from the remaining 11 speakers. Noise sources consisted of two parts. International Female Fluctuating Masker (IFFM) stimuli were used for speakers at 120° and 240° azimuth. Cafeteria noise (recorded in a restaurant during its peak hours with ZOOM H6 in Shanghai) that has been filtered to have the same long-term average spectrum as the speech signal were emitted from the remaining nine speakers. This was considered an ecologically valid noisy situation, which incorporated both stationary and modulated noise source. The level of each cafeteria noise source was approximately 56 dB A, resulting a total cafeteria noise level of 65 dB A. The level of each IFFM source was approximately 63 dB A, resulting a total IFFM level of 65 dB A. In this case, the overall competing noise level was set to 68 dB A. Against the fixed noise level, the sentence level was automatically adjusted according to the Oldenburg sentence test (OLSA) procedure (Brand and Kollmeier, 2002). The initial SNR level was 5 dB, i.e., the sentence level began at 73 dB A, and then was adaptively changed according to the correctness of the participants’ responses.
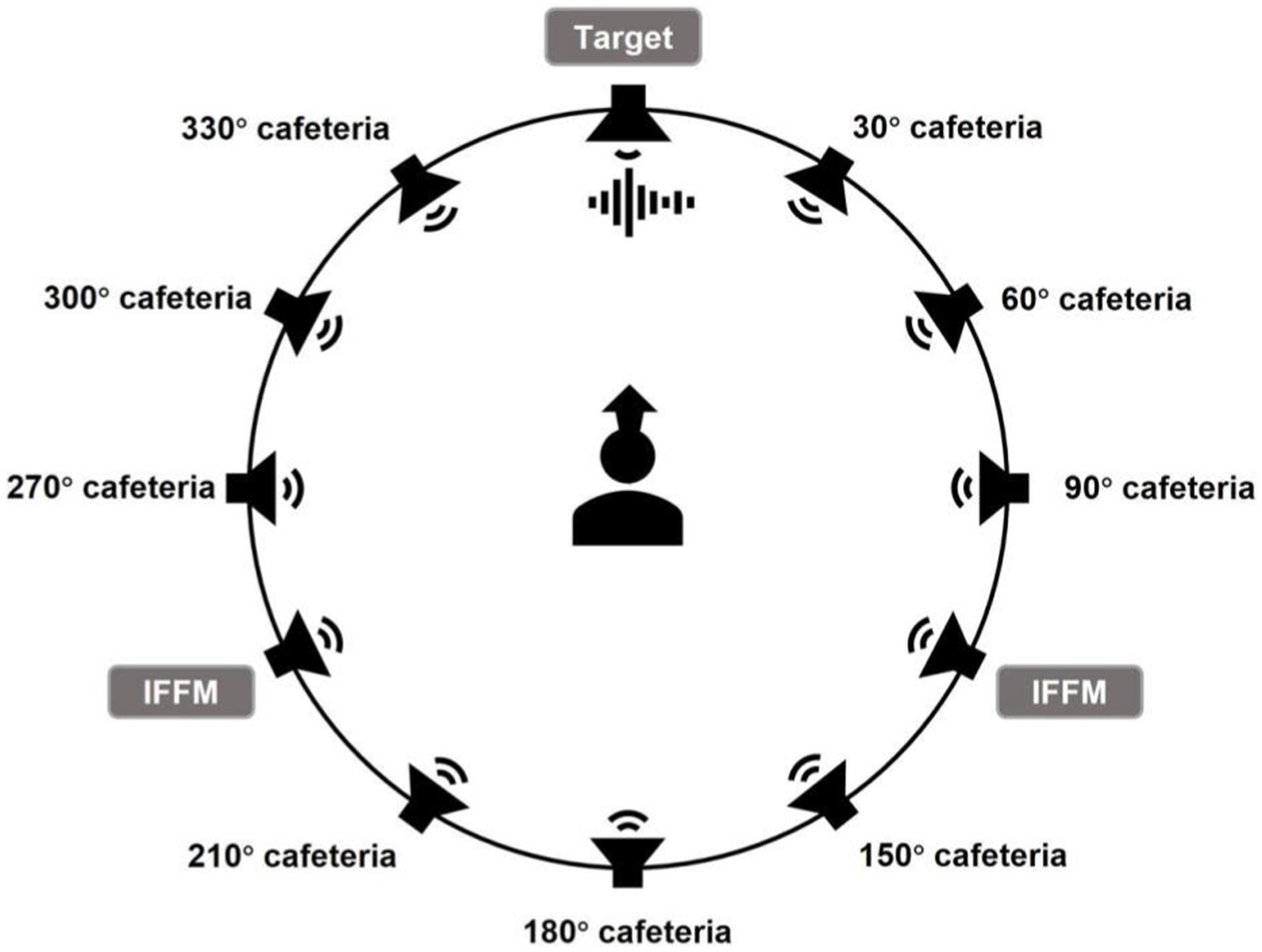
Figure 3. Diagram of speaker and stimuli configuration for ACALES and CMNmatrix sentence test; the radius of speaker circle is 1.4 m.
Before the test, participants were required to review all the test words printed out on a paper to ensure they were familiar with these words especially the names. Two practice lists were administered with NRoff to ensure that the participants fully understood the test procedure and to reduce the training effect. In the formal session, one sentence list was tested for each NR strength condition and the order of testing was randomized for three NR strengths.
Subjective listening effort
The listening effort test was administered using Adaptive Categorical Listening Effort Scaling (ACALES; Krueger et al., 2017) method. The test was performed using MATLAB software (MathWorks, Natick, MA) and was configured with female CMNmatrix sentences being played from 0° azimuth speaker and noise sources present from the remaining 11 speakers. The competing noise for ACALES was the same as that of the CMNmatrix sentence test (Figure 3). The level of the noise was held constant at 68 dBA and the level of the target speech was changed adaptively in order to achieve desired SNRs. For each trial, a group of three sentences was played. Participants were required to rate how much effort they need to follow the speaker at the current SNR by choosing from the 14 categories ranging from “effortless” to “extremely effortful” with one additional “only noise” option. If no response was made after 5 s, another group of sentences were played at the same SNR. For more details about the test structure please see Krueger et al. (2017). Participants responded by mouse clicking with a screen placed directly in front of them (see Figure 4 for the interface).
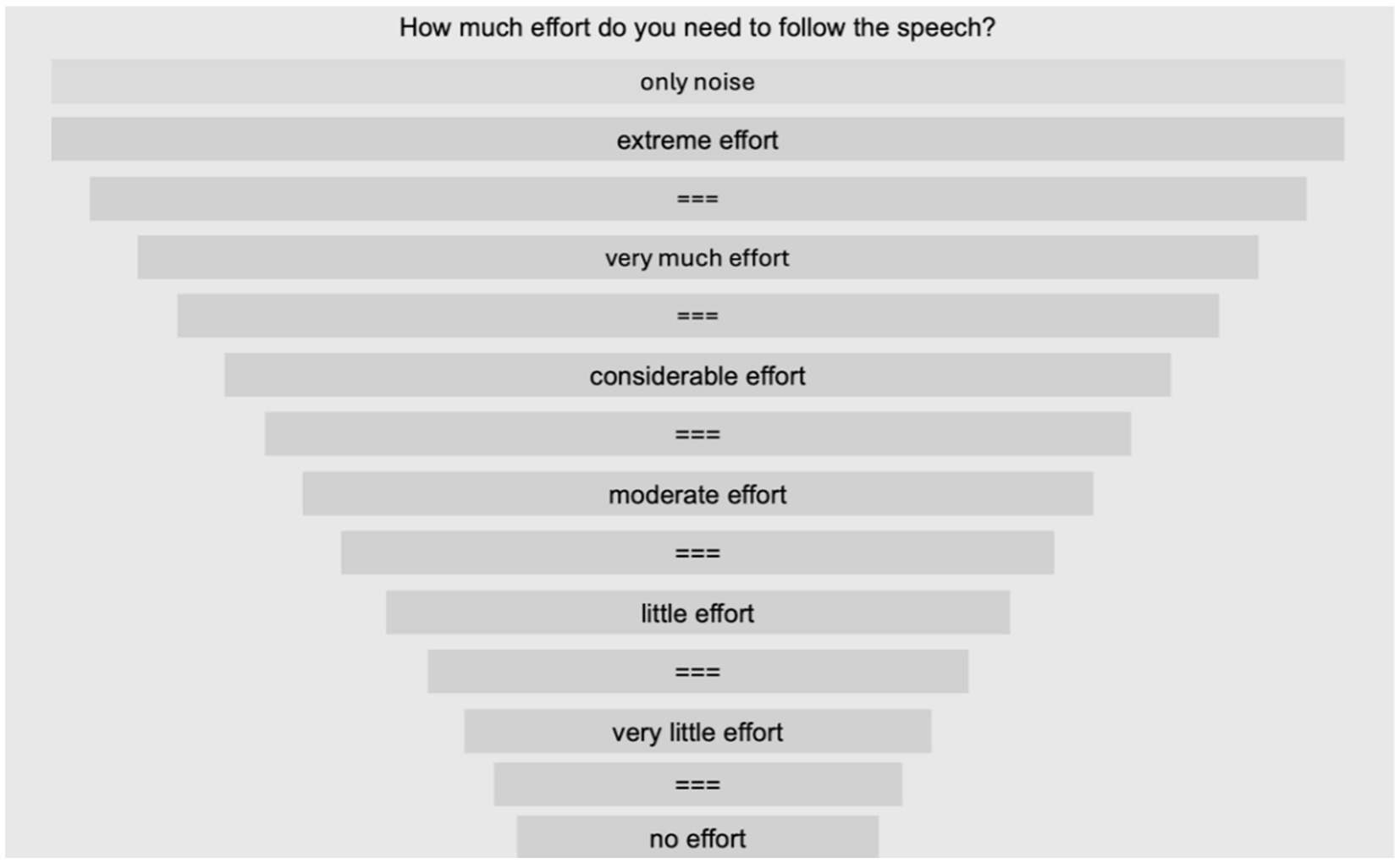
Figure 4. Response interface of ACALES test. See Appendix 1 for the Mandarin text.
Since the paradigm was less intuitive as the speech recognition test, adequate training was provided before the formal test. The practice consisted of a maximum of 20 trials presented at SNRs in the range from −25 to 30 dB. The custom MATLAB program presented the training results by plotting the selected listening effort category as function of SNRs in a scatterplot. If the dots were distributed randomly and not even a weak trend of increasing effort with decreasing SNRs was observed visually, the participant received re-instruction and a second practice test. NR settings for the practice test was randomly selected for each participant. For the formal test, the order of three NR strength settings was also randomized across participants.
Sound quality assessment
Sound quality was assessed by comparing the processing effect of three manual programs that differ only in NR strength in real time. A custom MATLAB program that can communicate with the fitting software Target was developed, in which three manual programs were represented by three buttons. When one button was clicked, the underlying HA program was activated by the fitting software. The test sound scene included a target speech presented from the front loudspeaker at 0° azimuth and cafeteria noise presented simultaneously from the remaining 11 loudspeaker (Figure 5). The speech signal at 66 dB A was a 15-s excerpt from a Chinese passage (The North Wind and the Sun) recorded by a female speaker. The combined level of 11 noise sources was 66 dB A, resulting in an SNR of 0 dB. Participants were seated at the center of the speaker circle with an LCD monitor placed in front of them. They were asked to rate the sound quality of the speech signal in dimensions of listening comfort, noise suppression, speech clarity, and overall satisfaction. For each attribute, there were three sliders that the participant can give the rating score for each of the three programs (see Figure 6 for the interface). The range of the slider was from 0 to 100 with five categorical labels at each 25 intervals. The categorical labels and corresponding number of ticks for each attribute are shown in Table 1. The participant was able to switch between three programs with different NR strengths (hidden by “A,” “B,” “C”) by clicking on the buttons at the bottom of the screen (Figure 6). At the top of the screen, the title of the test was shown to instruct participants which attribute to rate at the current trial. Before the formal test, a training session was provided to familiarize the participants with the method and the rating scale. In the formal test, both test and retest were included for each attribute.
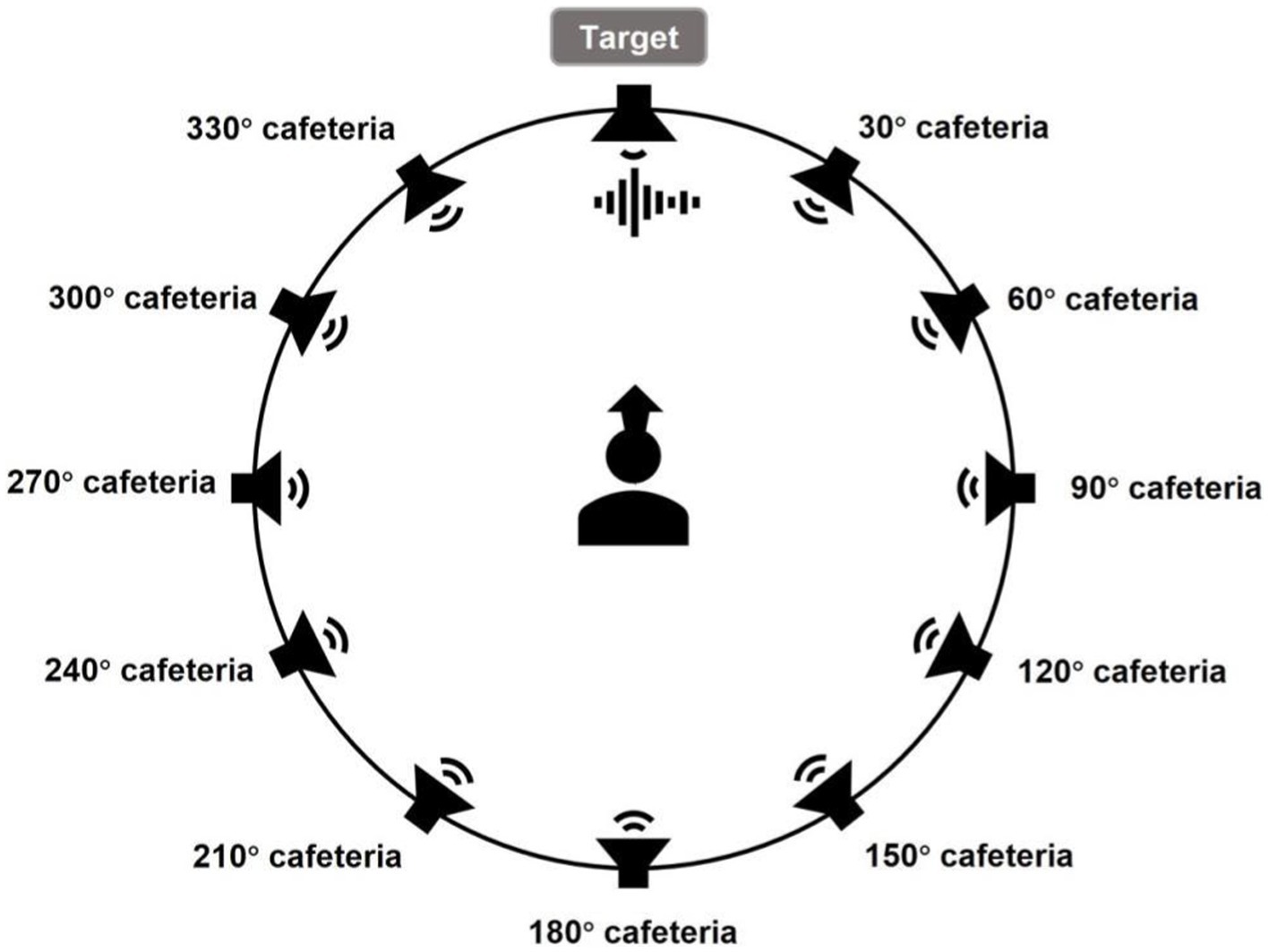
Figure 5. Diagram of speaker and stimuli configuration for subjective sound quality assessment; the radius of the speaker circle is 1.4 m.
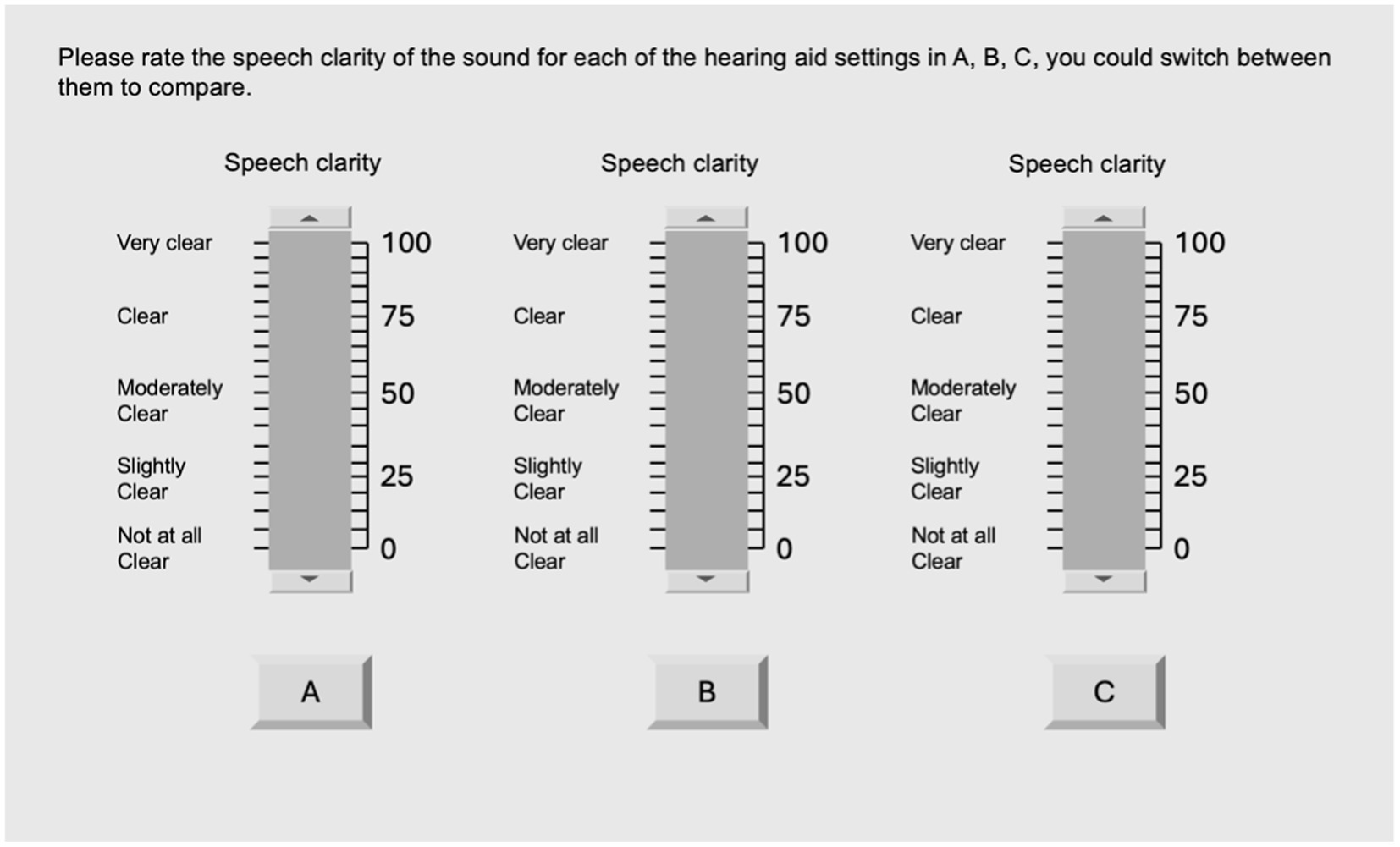
Figure 6. Participant’s screen for the attribute of speech clarity as an example. See Appendix 2 for the Mandarin text.
Procedures
All participants were tested at three separate sessions to complete all the measures within a 2-week period. The interval between each visit was 3 to 4 days. Tests were conducted in a sound booth with background noise below 30 dB A in Beijing Tongren Hospital. Twelve speakers (Audioengine 2+) were arranged in a circle with a radius of 1.4 m. The touchscreen monitor (Philips) used in listening effort and sound quality rating to present response interface was 50 cm long and 30 cm wide.
In the first session, after obtaining the informed consent from participants, otoscopy, pure tone audiometry (both air conduction and bone conduction) and tympanometry were implemented to check hearing condition, followed by MoCA screening. Individual ear impression was taken at last. In the second visit, speech recognition in noise test, subjective listening effort rating and sound quality assessment were conducted for three manual programs of different NR strengths (NRoff, NRmod, NRstr) with SpiN as the base program. In the third visit, all the tests and settings were kept the same as those in the second visit, except that SpiLN was used as the base program to create manual programs. In these two sessions, the order of three outcome measures was fixed, but the order of NR strength conditions was randomized within each measure. Break was scheduled between tests or whenever required by the participants.
Statistical analysis
The above three outcomes were measured repeatedly with three NR strengths under each of the two HA programs (SpiN and SpiLN). For all three measures, data of SpiN and SpiLN was analyzed separately. The individual rated listening effort was fitted as a two-slope function of the SNR using the BX fitting method (Oetting et al., 2014) which was recommended by Krueger et al. (2017). The data points of the rating category “only noise” were excluded. The SNR values at “moderate effort” (7 ESCU-Effort Scaling Category Units) for each participant and condition were extracted. A repeated measures analysis of variance (rmANOVA) with SNRs at “moderate effort” as the dependent variable and NR strength condition as the independent variable was performed to see if SNRs required to reach “medium effort” were different between NR strength conditions. Data of speech recognition in noise test was analyzed by the repeated measures one-way ANOVA with SRT50 as the dependent variable and NR strength conditions as the independent variable. For sound quality assessment, rating scores were averaged across the test and retest for analysis. Repeated measures one-way ANOVA with rating scores as the dependent variable and NR strength conditions as the independent variable was performed for each of the four attributes. Friedman’s test was used instead if the normal distribution assumption was not met. Statistical analyses were conducted using R software (version 4.3.2; R Core Team, 2021) with “bruceR” package (Bao, 2023) for ANOVA and “ggplot2” package (Wickham, 2016) for graphs.
Results
Speech intelligibility
Figure 7 shows boxplots of SRTs of three NR strength levels for the SpiLN and for SpiN programs. The mean SRTs of −3.0, −3.7, and −3.5 dB were obtained in the three NR conditions for the SpiLN program, respectively. Great variability in results was observed, with SDs of 5.5 dB, 5.1 dB, and 4.9 dB, respectively, in the three NR conditions. The median SRTs of −2.8, −2.9, and −3.0 dB, obtained in the three NR conditions for condition of SpiN, respectively. SRT50s were compared between three NR levels (NRoff, NRmod, NRstr) in two programs (SpiN or SpiLN) separately. SRTs in each test condition of SpiLN program were normally distributed as verified by the Shapiro–Wilk test. There is no significant effect of noise reduction (NR), F(2, 42) = 1.133, p = 0.332. For SpiN program, SRTs in NRmod were not normally distributed. Therefore, a non-parametric test Friedman Test was applied, which also indicated no significant effect of NR, χ2(2) = 3.7, df = 2, p = 0.157.
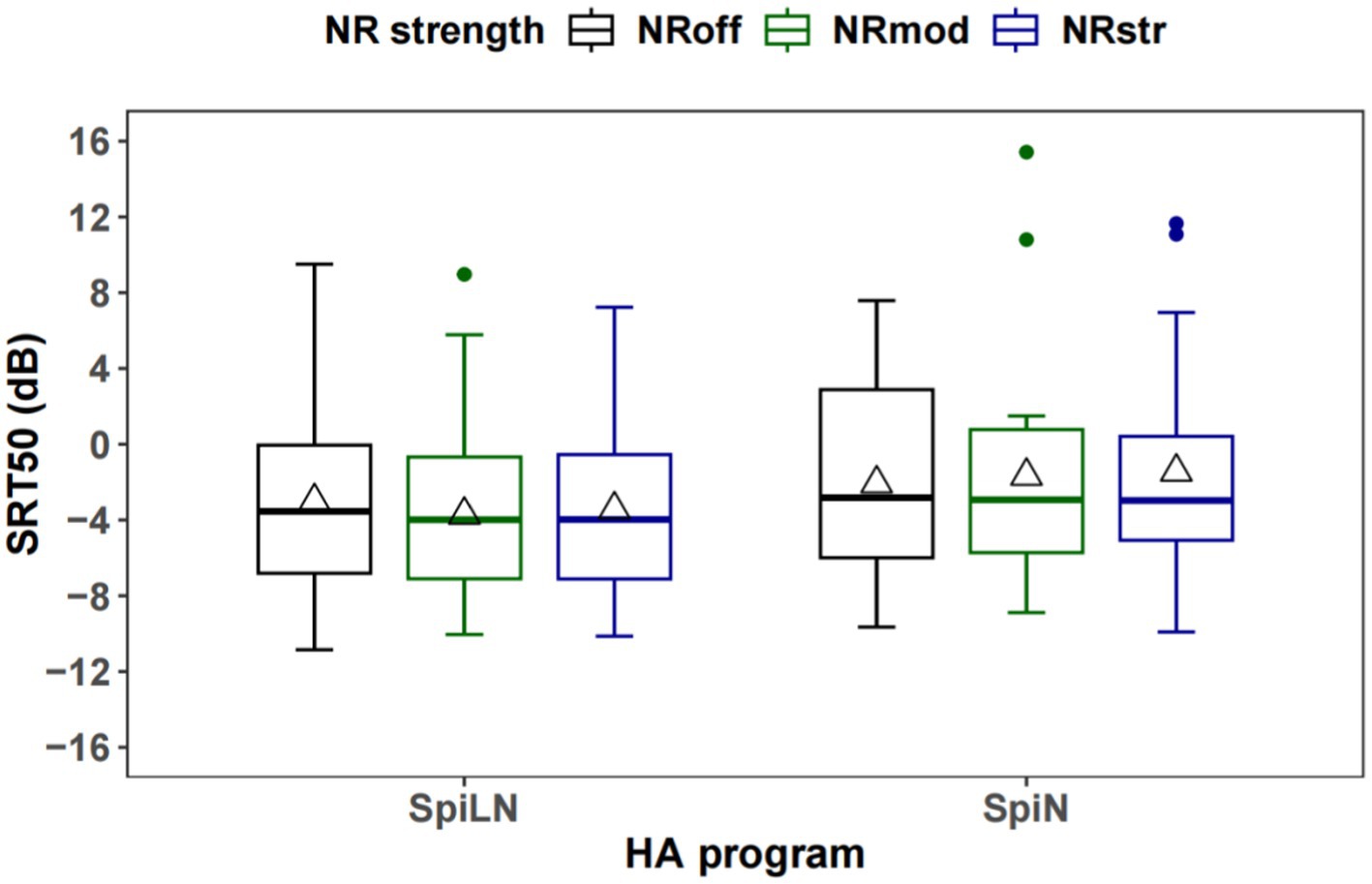
Figure 7. Boxplots of SRTs of three NR strength levels for both SpiLN and SpiN programs. The triangle sign represents the mean. The horizontal line within each box indicates the median. Lines extending from the top and bottom of each box indicate the highest and lowest values within 1.5 times the interquartile range. NR, Noise Reduction; SpiN, speech in noise program; SpiLN, speech in loud noise program.
Listening effort
Figures 8, 9 show estimated two-slope functions of listening effort for each individual and the group mean of the results for three NR strengths within the SpiN program an SpiLN program, respectively. The mean listening effort function was calculated by averaging the SNRs for each rating category over all participants. In both HA programs, there is a large amount of variation in the participant’s individual listening effort function. For the group mean function, the perceived listening effort decreased with increasing SNR. Across the entire range of listening effort scaling, the NRoff required higher SNR values to achieve similar effort ratings compared to the NR activate conditions (NRmod and NRstr). Such advantages of NR in SpiN program appeared to be more pronounced roughly between “little effort” (5 ESCU) to “medium effort” (7 ESCU), but more discrete for “no effort” (1 ESCU) and “extreme effort” (13 ESCU). As for SpiLN program, NR activated conditions (NRmod and NRstr) provided more advantages roughly from “medium effort” (7 ESCU) to “considerable effort” (9 ESCU). In terms of quantitative analysis, in Figure 10, the SNR values at 7 ESCU were extracted because it was the midpoint of the smooth area of the two-slope listening effort function. For SpiN program, the mean SNR at 7 ESCU was 0 dB for the NRoff, −1.3 dB for the NRmod, and −1.1 dB for the NRstr with standard deviations of 3.3, 3.1, and 3.3 dB, respectively. There is a significant effect of noise reduction (NR) settings. Specifically, the difference from NRoff was significant for NRmod [t(22) = −3.032, p = 0.018] but not for NRstr [t(22) = −2.23, p = 0.11]. No significant difference was found between NRmod and NRstr [t(22) = 0.36, p = 1]. In the SpiLN program, the mean SNR at 7 ESCU was −1.1 dB for NRoff, −3.3 dB for NRmod, and −3 dB for NRstr, with standard deviations of 4.0 dB, 3.8 dB, and 4.2 dB, respectively. Significant differences from NRoff were observed with both NRmod [t(24) = −6.17, p < 0.001] and NRstr [t(24) = −6.08, p < 0.001]. No significant difference was found between NRmod and NRstr [t(24) = 1.03, p = 0.944].
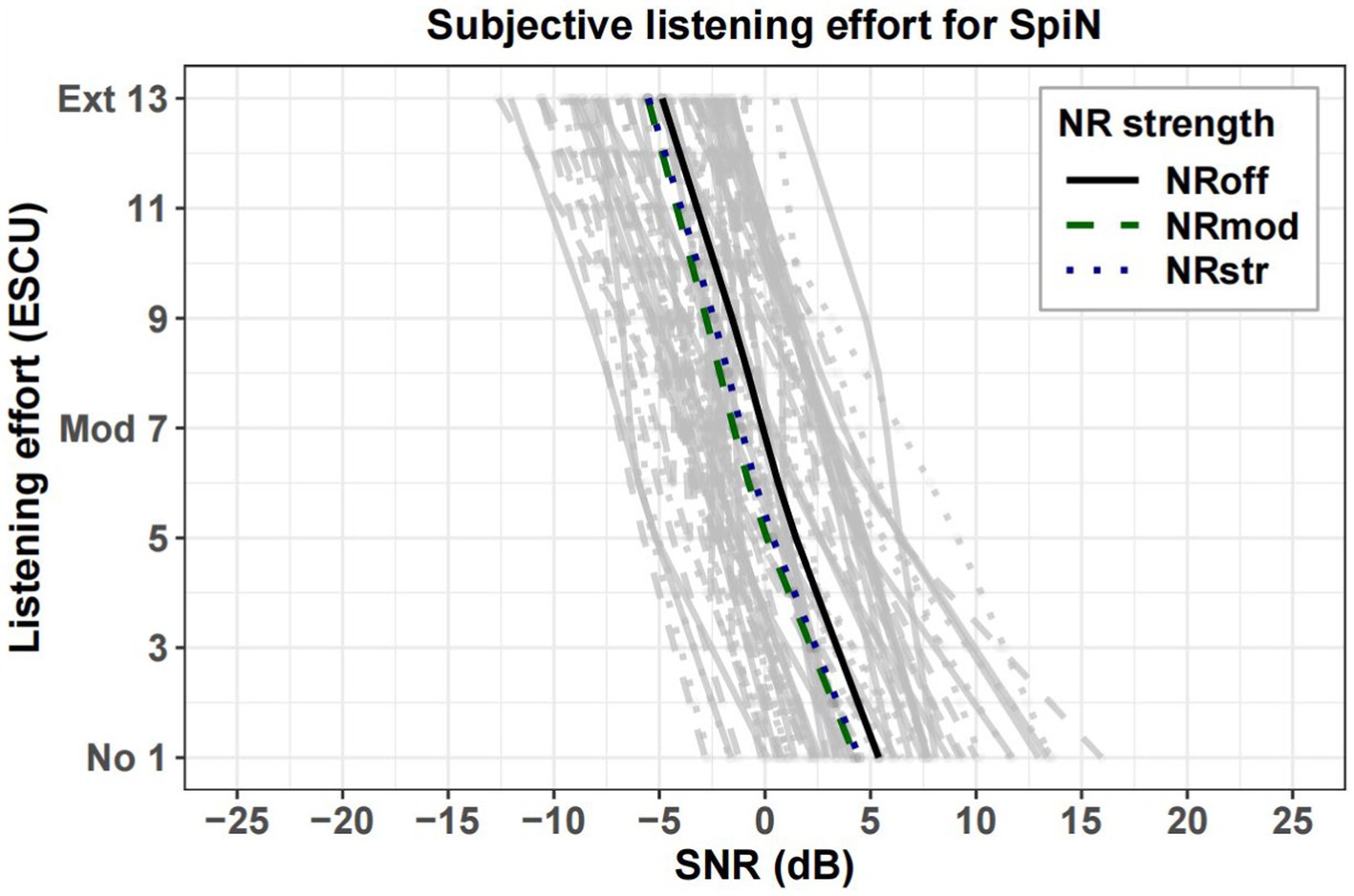
Figure 8. Individual estimated two slope functions for listening effort of each participant (gray lines) and mean estimated two slope functions (highlighted) for NRoff (solid line), NRmod (dashed line), and NRstr (dotted line) in SpiN program. The rating category “no effort” corresponds to 1 ESCU, “medium effort” to 7 ESCU, and “extreme effort” to 13 ESCU. NR, noise reduction; ESCU, Effort Scaling Category Units.
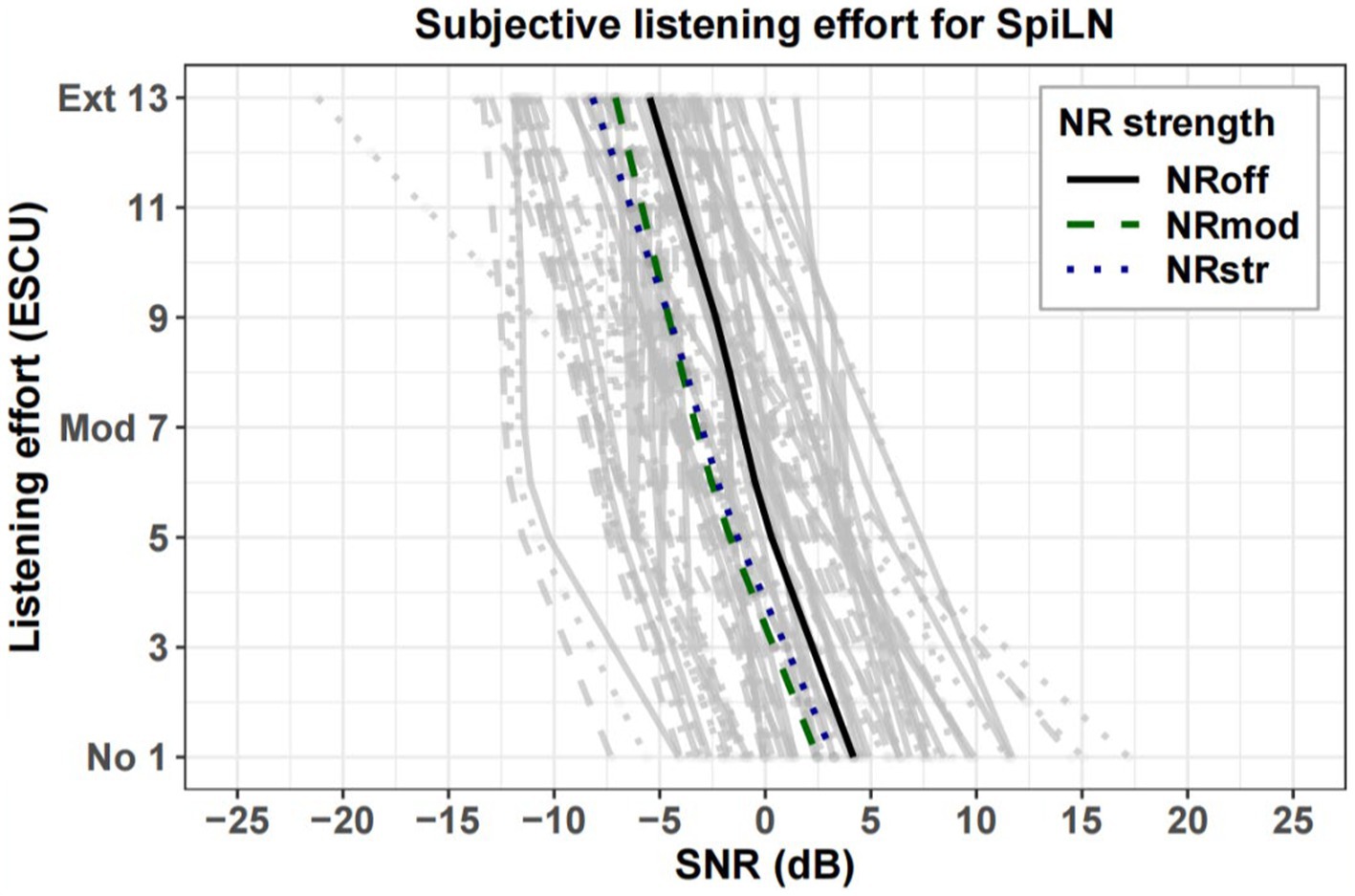
Figure 9. Individual estimated two slope functions for listening effort of each participant (gray lines) and mean estimated two slope functions (highlighted) for NRoff (solid line), NRmod (dashed line), and NRstr (dotted line) in SpiLN program. The rating category “no effort” corresponds to 1 ESCU, “medium effort” to 7 ESCU, and “extreme effort” to 13 ESCU. NR, noise reduction; ESCU, Effort Scaling Category Units.
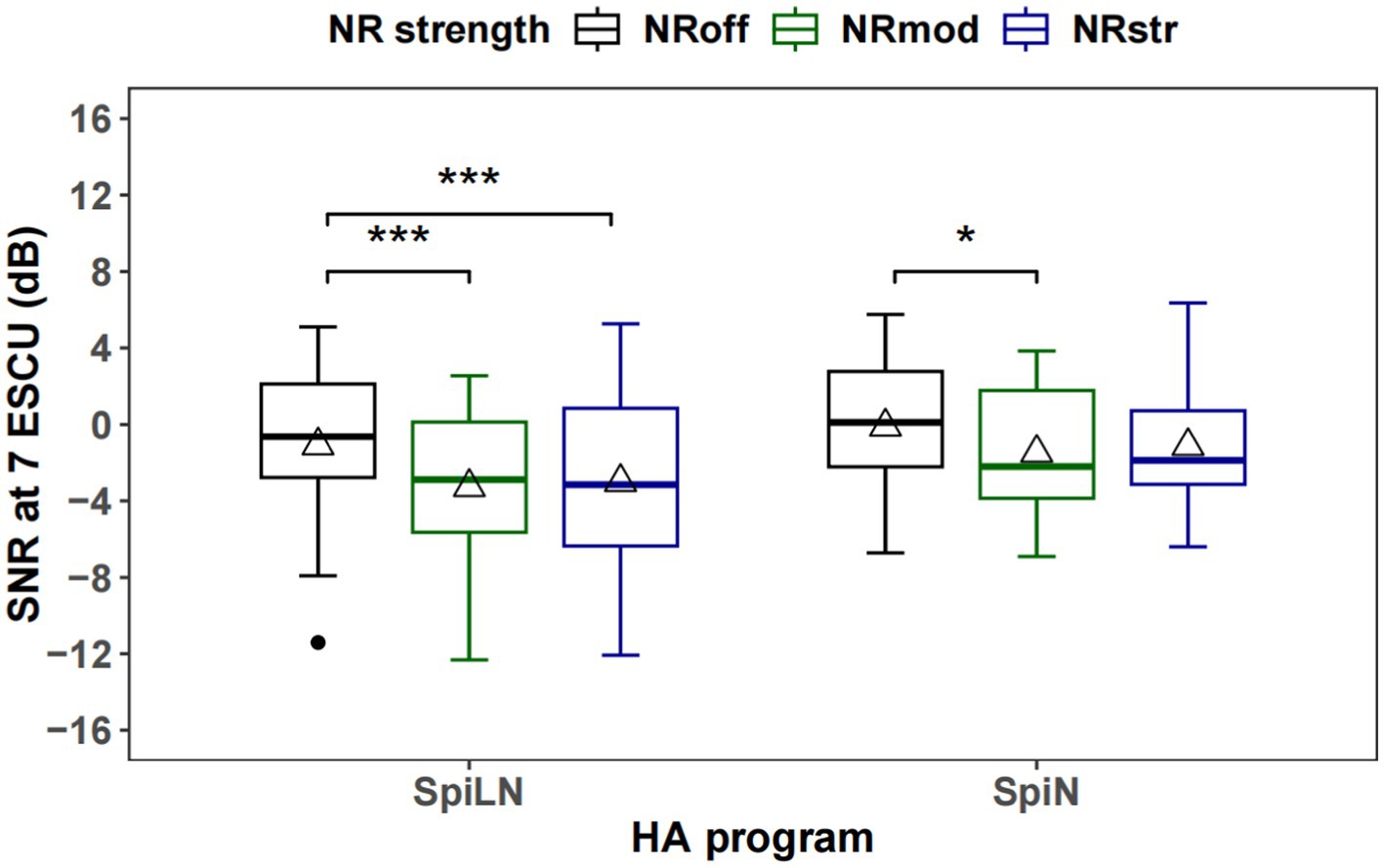
Figure 10. Boxplots of SNR at 7 ESCU, which represents the medium effort rating point in the listening effort function in dB SNR, are presented. The triangle marker indicates the mean, and the horizontal line shows the median. The width of the box depicts the interquartile range, and lines extending from the box represent values within 1.5 times the interquartile range. ***p < 0.001, *p < 0.05.
Sound quality assessment
Figure 11 plots the average sound quality ratings from the 24 participants in the three NR strengths. One participant’s data was missing. For the rating of noise suppression in the SpiLN program, the analysis yielded significant main effect of NR [F(1.461, 33.594) = 18.43, p < 0.001]. The participants rated noise suppression significantly higher with NRstr than with NRoff [t(23) = 4.78, p < 0.001] and with NRmod [t(23) = 2.95, p < 0.05]; NRmod was rated significantly higher than NRoff [t(23) = 4.39, p < 0.001]. For SpiN program, the main effect of NR was significant [F(1.344, 30.908) = 18.57, p < 0.001]. The participants rated noise suppression significantly higher with NRstr [t(23) = 4.45, p < 0.001] and NRmod [t(23) = 5.19, p < 0.001] than with NRoff.
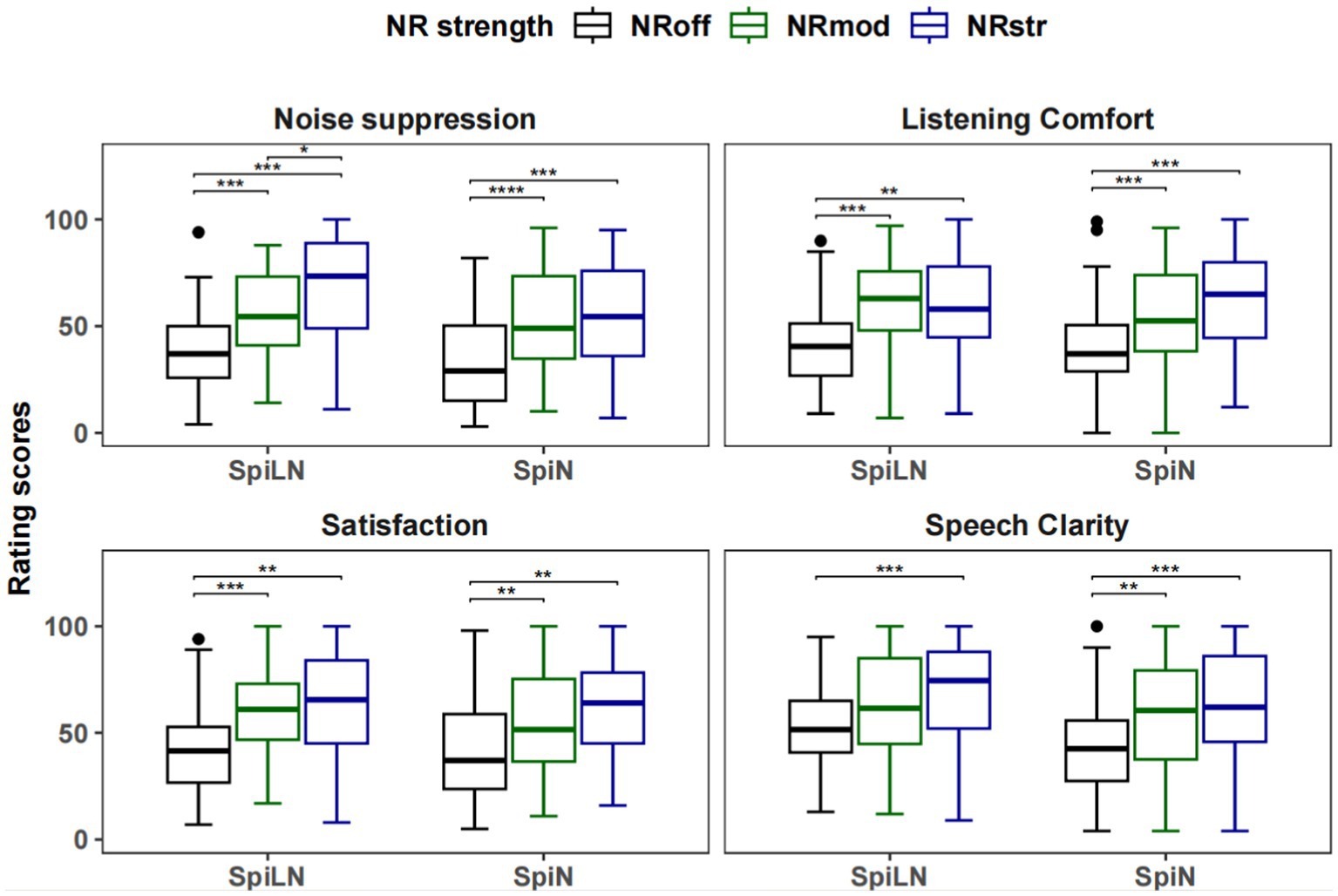
Figure 11. Boxplots of sound quality ratings for four attributes. The median is shown by the horizontal line at the center of each box. The width of the box represents the interquartile range, while lines extending from the top and bottom edges of the box indicate the highest and lowest values within 1.5 times the interquartile range. ****p < 0.0001, ***p < 0.001, **p < 0.01, *p < 0.05.
In terms of listening comfort and satisfaction ratings, the main effect of NR was significant for both the SpiLN program [Listening comfort: F(2, 46) = 11.35, p < 0.001; Satisfaction: F(1.308, 30.094) = 12.87, p < 0.001] and the SpiN program [Listening comfort: F(2, 46) = 16.56, p < 0.001; Satisfaction: χ2(2) = 17.58, p < 0.001]. For SpiLN program, participants rated significantly higher listening comfort and satisfaction with NRstr [Listening comfort: t(23) = 3.35, p < 0.01; Satisfaction: t(23) = 3.45, p < 0.01] and with NRmod [Listening comfort: t(23) = 4.38, p < 0.001; Satisfaction: t(23) = 4.80, p < 0.001] than with NRoff. For SpiN program, both sound attributes were rated significantly higher with NRstr [Listening comfort: t(23) = 4.81, p < 0.001; Satisfaction: Z = 37, p < 0.01] and NRmod [Listening comfort: t(23) = 4.41, p < 0.001; Satisfaction: Z = 50, p < 0.01] than with NRoff.
For clarity ratings, the analysis revealed a significant effect of NR for both the SpiLN program [F(2, 46) = 9.09, p < 0.001] and the SpiN program [F(2, 46) = 15.36, p < 0.001]. Participants rated significantly higher speech clarity with NRstr than with NRoff for both the SpiLN program [t(23) = 4.26, p < 0.001] and the SpiN program [t(23) = 4.68, p < 0.001]. The speech clarity was rated significantly higher with NRmod than with NRoff only in the SpiN program [t(23) = 3.84, p < 0.01].
Discussion
The primary objective of this study was to examine the perceptual outcome of individuals with severe-to-profound hearing loss in response to noise reduction technology. Our assessments encompassed domains related to speech recognition, listening effort, and subjective sound quality. Our research findings indicate that individuals with severe-to-profound hearing loss derived specific benefits from the implementation of noise reduction technologies. These benefits included reduced listening effort and improved subjective sound quality, as observed within the methodological framework of our study. It is important to note, however, that these advantages did not extend to improvements in speech intelligibility.
Speech intelligibility
No significant difference in speech intelligibility was observed between HA settings with and without NR. While the NR algorithm utilized in this study did not lead to improved speech recognition, it is noteworthy that it did not negatively affect performance, which has also been observed with certain other NR algorithms (Ricketts and Hornsby, 2005). This is consistent with our hypothesis that speech recognition in noise may not be improved by NR processing, but neither is degraded. Previous research has suggested that speech degradation caused by high levels of noise or distorted speech cues could lead to delays in lexical processing (Ben-David et al., 2011; McMurray et al., 2017). On the one hand, the application of NR processing could attenuate a certain amount of background noise, but on the other hand it would introduce some distortion of speech cues (Kates, 2008). If the level of speech cue distortion is high enough to counteract the effect of noise reduction, listeners may not benefit from NR due to the increased cognitive workload in speech processing (Winn et al., 2015; Ward et al., 2017).
For individuals with mild to moderate hearing loss, several studies have reported improvements in speech intelligibility with NR algorithms (Peeters et al., 2009; Ohlenforst et al., 2018; Wong et al., 2018). Ohlenforst et al. (2018) found that sentence recognition performance was significantly better when NR was enabled than when it was not. The study by Wong et al. (2018) revealed that when NR was activated, listeners demonstrated improved speech perception abilities and reported reduced noise annoyance, resulting in improved speech clarity. These findings have been attributed in part to predictable relationships between degree of impairment and auditory-cognitive abilities in individuals with mild to moderate hearing loss (Humes, 2007; Souza et al., 2007). These associations diminished for listeners with more severe hearing loss, whose abilities became more variable and unpredictable.
In this study, NR settings on the hearing aids, being new settings for the participants, might have been perceived as unfamiliar input. This unfamiliarity could lead to an increased reliance on working memory, as participants needed to utilize more cognitive resources to resolve the unfamiliar stimuli (Souza et al., 2015; Ronnberg et al., 2019, 2022). The speech tests employed in this study included cafeteria noise and the International Female Fluctuating Masker (IFFM), incorporating both energetic and informational maskers. These maskers pose distinct challenges: energetic maskers distract, while informational maskers impact more significantly as they engage semantic long-term memory (SLTM; Sorqvist and Ronnberg, 2012). In situations involving informational maskers, working memory capacity (WMC) is a crucial predictor of performance. The findings suggest that high dependency on working memory was not mitigated by prolonged use of hearing aids; a decade of hearing aid use does not diminish this reliance, especially with four-talker (4 T) maskers (Ng and Ronnberg, 2020). Additionally, the study’s speech recognition materials were akin to Hagerman matrix sentences. Ronnberg et al. (2016) found that working memory had a stronger correlation with Hagerman matrix sentences than with HINT sentences, which are driven by everyday contexts. This underscores the significant role of working memory in complex listening environments. Hearing aid users with severe-to-profound hearing loss often exhibited constraints in working memory capacity, which might impair their capability to process and decode speech signals following NR processing. Although NR technology effectively suppressed background noise, this suppression might not adequately compensate for the challenges encountered by these individuals in processing speech information and memory tasks. Consequently, focusing on traditional speech recognition tests might not fully capture the overall improvements in communication achieved through NR technology. Additionally, these tests might lack sensitivity to the enhancements in NR’s top-down processing. Therefore, it was essential to supplement these assessments with subjective evaluations and measures of listening effort to comprehensively evaluate the influence of NR.
Listening effort
This study investigated the effect of NR schemes on listening effort as indicated by a subjective method in a group of people with sever-to-profound hearing impairment. Our results suggested that the NR processing reduced the listening effort required in noisy conditions for individuals with severe-to-profound hearing loss. Participants preferred either NRmod or NRstr over NRoff, were consistent with previous work demonstrating a reduction in listening effort but no improvement in speech intelligibility when enabling NR (Desjardins and Doherty, 2014; Ohlenforst et al., 2018). In addition, several other studies had shown that participants experienced reduced listening effort when using NR in noisy environments (Sarampalis et al., 2009; Brons et al., 2014; Wong et al., 2018). Despite variations in specific details, overall, these findings confirmed that listening effort evaluations could sensitively capture listeners’ perceived effort.
Kestens et al. (2021) undertook a systematic review to investigate how intersubject differences in cognition could influence the aided benefit for speech understanding and listening effort with bilateral digital hearing aids. The research results demonstrated that the effects of noise reduction technology varied among hearing aid users with different cognitive abilities. For instance, the study conducted by Desjardins and Doherty (2014) did not find a significant relationship between working memory and listening effort when using noise reduction technology. In contrast, Wendt et al. (2017) found that users with better working memory capacities experienced less objectively measured listening effort compared to HA users with less memory capacity when utilizing the noise reduction feature. Furthermore, users with slower processing speeds appeared to benefit more from noise reduction technology in terms of listening effort, indicating that they relied more on hearing aids when dealing with complex listening situations (Desjardins and Doherty, 2014). Although noise reduction technology is expected to theoretically help reduce listening effort, the existing research findings remains inconsistent. This variability in outcomes could be due to the different measurement methods used in various studies, the differences in cognitive abilities among participants, and variations in hearing aid technologies.
Researches suggested that NR might not necessarily enhance speech clarity, but rather it could reduce cognitive effort, a benefit that becomes particularly apparent in listening effort (Sarampalis et al., 2009; Desjardins and Doherty, 2014; Wong et al., 2018). In the patient-centered model, testing for listening effort is crucial. The goal of rehabilitation is to reduce the degree of barriers, activity limitations, and participation restrictions for hearing aid users (World Health Organization, 2001). Subjective listening effort seemed to be related to perceived hearing impairment (Alhanbali et al., 2018), and subjective listening effort could have a negative impact on the quality of life (Pichora-Fuller et al., 2016).
Sound quality assessment
Overall, the activation of NR provided clear advantages compared to its deactivation across several dimensions, including enhanced noise tolerance, improved listening comfort, increased satisfaction, and better perceived speech signal quality. These trends showed a more favorable and comfortable auditory experiences when noise was selectively attenuated and fine-tuned by NR algorithms.
The findings of our study were consistent with observations that a majority of individuals with hearing impairments tended to employ NR features in their hearing aids (Souza et al., 2018). Clearly, with noise reduction activated, speech and noise that had undergone noise reduction processing became more comfortable for listeners due to the reduction in noise levels. Overall, these subjective assessments of NR evaluation demonstrated positive effects in terms of noise acceptance, user preferences, and enhanced listening comfort. Numerous studies have previously indicated that noise reduction had a positive impact on noise perception and preferences. Studies utilizing the Abbreviated Profile of Hearing Aid Benefit (APHAB; Cox and Alexander, 1995) have consistently shown that noise reduction has a notable impact on reducing aversion to noise (Boymans and Dreschler, 2000; Palmer et al., 2006). Brons et al. (2013) demonstrated that activating noise reduction reduced annoyance caused by noise, although it found no impact on speech naturalness through paired comparison testing. Palmer et al. (2006) discovered that when NR was activated, the annoyance ratings of hearing-impaired participants closely resembled those of individuals with normal hearing. These findings support that noise reduction has the potential to enhance listening comfort and reduce noise-related annoyance. In this study, participants reported improved speech clarity when NR was activated. This apparent discrepancy between objective speech intelligibility and subjective speech clarity ratings may stem from the different conditions under which each was tested. Specifically, speech intelligibility was assessed using an adaptive procedure that varied the signal-to-noise ratio (SNR) within a negative range. It is documented that NR algorithms are more effective at positive SNRs than at negative ones, which could influence these outcomes (Fredelake et al., 2012; Smeds et al., 2015). Furthermore, the benefits in terms of listening ease and comfort found in this study did not coincide with any reduction in speech intelligibility.
Conclusion
This study highlights that that individuals with severe-to-profound hearing loss can still derive significant benefits from noise reduction technology. These benefits are evidenced by reduced listening effort and improved subjective sound quality ratings. Importantly, these advantages were achieved without compromising speech intelligibility. While the efficacy of the specific NR was confirmed in this study, its clinical relevance, particularly its potential to predict real-world benefits, should be further explored in future research. It’s crucial to note that the findings of this study may not be directly applicable to hearing aids featuring different NR characteristics or to diverse acoustic environments not encompassed within this study. Furthermore, it is essential to assess the benefits of NR when combined with other adaptive features, such as beamformer.
Data availability statement
The raw data supporting the conclusions of this article will be made available by the authors, without undue reservation.
Ethics statement
The studies involving humans were approved by the Institutional Review Board at the Beijing Tongren Hospital. The studies were conducted in accordance with the local legislation and institutional requirements. The participants provided their written informed consent to participate in this study.
Author contributions
RD: Conceptualization, Data curation, Formal analysis, Funding acquisition, Investigation, Methodology, Project administration, Supervision, Validation, Writing – original draft, Writing – review & editing. PL: Data curation, Investigation, Resources, Supervision, Validation, Writing – review & editing. XT: Conceptualization, Formal analysis, Methodology, Project administration, Supervision, Validation, Visualization, Writing – original draft, Writing – review & editing. YW: Data curation, Investigation, Supervision, Validation, Writing – review & editing. YC: Data curation, Investigation, Supervision, Writing – review & editing. JZ: Conceptualization, Data curation, Methodology, Validation, Visualization, Writing – original draft, Writing – review & editing. LY: Conceptualization, Methodology, Resources, Writing – review & editing. SZ: Supervision, Validation, Writing – review & editing. JG: Conceptualization, Methodology, Supervision, Validation, Writing – review & editing. SW: Conceptualization, Funding acquisition, Methodology, Project administration, Resources, Supervision, Validation, Writing – review & editing.
Funding
The author(s) declare that financial support was received for the research, authorship, and/or publication of this article. This work was funded in part by grants from the National Key Research and Development Program of China (2023YFC3604200-project 2023YFC3604203) and High-Level Public Health Technical Talent Training Plan (Discipline Backbone-02-42).
Acknowledgments
We would like to express our sincere gratitude to Professor Haiyun Li for his invaluable guidance and meticulous revisions throughout the preparation of this manuscript. His insights and suggestions were crucial in shaping the final version of this paper. The authors thank the participants in the study for volunteering their time. The authors also thank Phonak for lending the hearing aids used in this study to the laboratory.
Conflict of interest
XT, JZ, and JG were employed by Sonova Shanghai Co., Ltd.
The remaining authors declare that the research was conducted in the absence of any commercial or financial relationships that could be construed as a potential conflict of interest.
Publisher’s note
All claims expressed in this article are solely those of the authors and do not necessarily represent those of their affiliated organizations, or those of the publisher, the editors and the reviewers. Any product that may be evaluated in this article, or claim that may be made by its manufacturer, is not guaranteed or endorsed by the publisher.
Supplementary material
The Supplementary material for this article can be found online at: https://www.frontiersin.org/articles/10.3389/fnins.2024.1407775/full#supplementary-material
References
Alhanbali, S., Dawes, P., Lloyd, S., and Munro, K. J. (2017). Self-reported listening-related effort and fatigue in hearing-impaired adults. Ear Hear. 38, e39–e48. doi: 10.1097/AUD.0000000000000361
Alhanbali, S., Dawes, P., Lloyd, S., and Munro, K. J. (2018). Hearing handicap and speech recognition correlate with self-reported listening effort and fatigue. Ear Hear. 39, 470–474. doi: 10.1097/AUD.0000000000000515
Baer, T., Moore, B. C., and Gatehouse, S. (1993). Spectral contrast enhancement of speech in noise for listeners with sensorineural hearing impairment: effects on intelligibility, quality, and response times. J. Rehabil. Res. Dev. 30, 49–72.
Bao, H.-W.-S. (2023). bruceR: broadly useful convenient and efficient R functions (version 2023.9) [computer software]. Available at: https://CRAN.R-project.org/package=bruceR
Ben-David, B. M., Chambers, C. G., Daneman, M., Pichora-Fuller, M. K., Reingold, E. M., and Schneider, B. A. (2011). Effects of aging and noise on real-time spoken word recognition: evidence from eye movements. J. Speech Lang. Hear. Res. 54, 243–262. doi: 10.1044/1092-4388(2010/09-0233)
Bentler, R., Wu, Y. H., Kettel, J., and Hurtig, R. (2008). Digital noise reduction: outcomes from laboratory and field studies. Int. J. Audiol. 47, 447–460. doi: 10.1080/14992020802033091
Boymans, M., and Dreschler, W. A. (2000). Field trials using a digital hearing aid with active noise reduction and dual-microphone directionality. Audiology 39, 260–268. doi: 10.3109/00206090009073090
Brand, T., and Kollmeier, B. (2002). Efficient adaptive procedures for threshold and concurrent slope estimates for psychophysics and speech intelligibility tests. J. Acoust. Soc. Am. 111, 2801–2810. doi: 10.1121/1.1479152
Brons, I., Houben, R., and Dreschler, W. A. (2013). Perceptual effects of noise reduction with respect to personal preference, speech intelligibility, and listening effort. Ear Hear. 34, 29–41. doi: 10.1097/AUD.0b013e31825f299f
Brons, I., Houben, R., and Dreschler, W. A. (2014). Effects of noise reduction on speech intelligibility, perceived listening effort, and personal preference in hearing-impaired listeners. Trends Hear 18:924. doi: 10.1177/2331216514553924
Brons, I., Houben, R., and Dreschler, W. A. (2015). Acoustical and perceptual comparison of noise reduction and compression in hearing aids. J. Speech Lang. Hear. Res. 58, 1363–1376. doi: 10.1044/2015_JSLHR-H-14-0347
Carlsson, P. I., Hjaldahl, J., Magnuson, A., Ternevall, E., Edén, M., Skagerstrand, Å., et al. (2015). Severe to profound hearing impairment: quality of life, psychosocial consequences and audiological rehabilitation. Disabil. Rehabil. 37, 1849–1856. doi: 10.3109/09638288.2014.982833
Chen, K. L., Xu, Y., Chu, A. Q., Ding, D., Liang, X. N., Nasreddine, Z. S., et al. (2016). Validation of the Chinese version of Montreal cognitive assessment basic for screening mild cognitive impairment. J. Am. Geriatr. Soc. 64, e285–e290. doi: 10.1111/jgs.14530
Convery, E., and Keidser, G. (2011). Transitioning hearing aid users with severe and profound loss to a new gain/frequency response: benefit, perception, and acceptance. J. Am. Acad. Audiol. 22, 168–180. doi: 10.3766/jaaa.22.3.5
Cox, R. M., and Alexander, G. C. (1995). The abbreviated profile of hearing aid benefit. Ear Hear. 16, 176–186. doi: 10.1097/00003446-199504000-00005
Degeest, S., Corthals, P., and Keppler, H. (2021). A Dutch version of a dual-task paradigm for measuring listening effort: a pilot study regarding its short-term test-retest reliability. B-ENT 17, 135–144. doi: 10.5152/B-ENT.2021.20160
Desjardins, J. L. (2016). The effects of hearing aid directional microphone and noise reduction processing on listening effort in older adults with hearing loss. J. Am. Acad. Audiol. 27, 29–41. doi: 10.3766/jaaa.15030
Desjardins, J. L., and Doherty, K. A. (2014). The effect of hearing aid noise reduction on listening effort in hearing-impaired adults. Ear Hear. 35, 600–610. doi: 10.1097/AUD.0000000000000028
Ferschneider, M., and Moulin, A. (2023). Listening effort in quiet and Noisy environments in the daily life of adults with hearing aids: an extended version of the effort assessment scale (EEAS). Trends Hear 27:23312165231176320. doi: 10.1177/23312165231176320
Fiedler, L., Seifi Ala, T., Graversen, C., Alickovic, E., Lunner, T., and Wendt, D. (2021). Hearing aid noise reduction lowers the sustained listening effort during continuous speech in noise-a combined Pupillometry and EEG study. Ear Hear. 42, 1590–1601. doi: 10.1097/AUD.0000000000001050
Flynn, M. C., Dowell, R. C., and Clark, G. M. (1998). Aided speech recognition abilities of adults with a severe or severe-to-profound hearing loss. J. Speech Lang. Hear. Res. 41, 285–299. doi: 10.1044/jslhr.4102.285
Fredelake, S., Holube, I., Schlueter, A., and Hansen, M. (2012). Measurement and prediction of the acceptable noise level for single-microphone noise reduction algorithms. Int. J. Audiol. 51, 299–308. doi: 10.3109/14992027.2011.645075
Gatehouse, S., and Noble, W. (2004). The speech, spatial and qualities of hearing scale (SSQ). Int. J. Audiol. 43, 85–99. doi: 10.1080/14992020400050014
Goman, A. M., and Lin, F. R. (2016). Prevalence of hearing loss by severity in the United States. Am. J. Public Health 106, 1820–1822. doi: 10.2105/AJPH.2016.303299
Grimby, A., and Ringdahl, A. (2000). Does having a job improve the quality of life among post-lingually deafened Swedish adults with severe-profound hearing impairment? Br. J. Audiol. 34, 187–195. doi: 10.3109/03005364000000128
Hagerman, B., and Olofsson, A. (2004). A method to measure the effect of noise reduction algorithms using simultaneous speech and noise. Act Acust United Acust 90, 356–361.
Hallam, R., Ashton, P., Sherbourne, K., and Gailey, L. (2006). Acquired profound hearing loss: mental health and other characteristics of a large sample. Int. J. Audiol. 45, 715–723. doi: 10.1080/14992020600957335
Hu, H., Xi, X., Wong, L. L. N., Hochmuth, S., Warzybok, A., and Kollmeier, B. (2018). Construction and evaluation of the mandarin Chinese matrix (CMNmatrix) sentence test for the assessment of speech recognition in noise. Int. J. Audiol. 57, 838–850. doi: 10.1080/14992027.2018.1483083
Humes, L. E. (2007). The contributions of audibility and cognitive factors to the benefit provided by amplified speech to older adults. J. Am. Acad. Audiol. 18, 590–603. doi: 10.3766/jaaa.18.7.6
Kestens, K., Degeest, S., and Keppler, H. (2021). The effect of cognition on the aided benefit in terms of speech understanding and listening effort obtained with digital hearing aids: a systematic review. Am. J. Audiol. 30, 190–210. doi: 10.1044/2020_AJA-20-00019
Krueger, M., Schulte, M., Brand, T., and Holube, I. (2017). Development of an adaptive scaling method for subjective listening effort. J. Acoust. Soc. Am. 141:4680. doi: 10.1121/1.4986938
Kumle, L., Võ, M. L.-H., and Draschkow, D. (2021). Estimating power in (generalized) linear mixed models: An open introduction and tutorial in R. Behav. Res. Methods. 53, 2528–2543. doi: 10.3758/s13428-021-01546-0
Lin, F. R. (2024). Age-related hearing loss. N. Engl. J. Med. 390, 1505–1512. doi: 10.1056/NEJMcp2306778
Loizou, P. C., and Kim, G. (2011). Reasons why current speech-enhancement algorithms do not improve speech intelligibility and suggested solutions. IEEE Trans. Audio Speech Lang. Process. 19, 47–56. doi: 10.1109/TASL.2010.2045180
McMurray, B., Farris-Trimble, A., and Rigler, H. (2017). Waiting for lexical access: Cochlear implants or severely degraded input lead listeners to process speech less incrementally. Cognition 169, 147–164. doi: 10.1016/j.cognition.2017.08.013
Moore, B. C. (1996). Perceptual consequences of cochlear hearing loss and their implications for the design of hearing aids. Ear Hear. 17, 133–161. doi: 10.1097/00003446-199604000-00007
Neher, T., Grimm, G., and Hohmann, V. (2014). Perceptual consequences of different signal changes due to binaural noise reduction: do hearing loss and working memory capacity play a role? Ear Hear. 35, e213–e227. doi: 10.1097/AUD.0000000000000054
Ng, E. H. N., and Ronnberg, J. (2020). Hearing aid experience and background noise affect the robust relationship between working memory and speech recognition in noise. Int. J. Audiol. 59, 208–218. doi: 10.1080/14992027.2019.1677951
Nordrum, S., Erler, S., Garstecki, D., and Dhar, S. (2006). Comparison of performance on the hearing in noise test using directional microphones and digital noise reduction algorithms. Am. J. Audiol. 15, 81–91. doi: 10.1044/1059-0889(2006/010)
Oetting, D., Brand, T., and Ewert, S. D. (2014). Optimized loudness-function estimation for categorical loudness scaling data. Hear. Res. 316, 16–27. doi: 10.1016/j.heares.2014.07.003
Ohlenforst, B., Wendt, D., Kramer, S. E., Naylor, G., Zekveld, A. A., and Lunner, T. (2018). Impact of SNR, masker type and noise reduction processing on sentence recognition performance and listening effort as indicated by the pupil dilation response. Hear. Res. 365, 90–99. doi: 10.1016/j.heares.2018.05.003
Ohlenforst, B., Zekveld, A. A., Jansma, E. P., Wang, Y., Naylor, G., Lorens, A., et al. (2017a). Effects of hearing impairment and hearing aid amplification on listening effort: a systematic review. Ear Hear. 38, 267–281. doi: 10.1097/AUD.0000000000000396
Ohlenforst, B., Zekveld, A. A., Lunner, T., Wendt, D., Naylor, G., Wang, Y., et al. (2017b). Impact of stimulus-related factors and hearing impairment on listening effort as indicated by pupil dilation. Hear. Res. 351, 68–79. doi: 10.1016/j.heares.2017.05.012
Palmer, C. V., Bentler, R., and Mueller, H. G. (2006). Amplification with digital noise reduction and the perception of annoying and aversive sounds. Trends Amplif. 10, 95–104. doi: 10.1177/1084713806289554
Peeters, H., Kuk, F., Lau, C. C., and Keenan, D. (2009). Subjective and objective evaluation of noise management algorithms. J. Am. Acad. Audiol. 20, 89–98. doi: 10.3766/jaaa.20.2.2
Pichora-Fuller, M. K., Kramer, S. E., Eckert, M. A., Edwards, B., Hornsby, B. W., Humes, L. E., et al. (2016). Hearing impairment and cognitive energy: the framework for understanding effortful listening (FUEL). Ear Hear. 37, 5S–27S. doi: 10.1097/AUD.0000000000000312
R Core Team (2021). R: A language and environment for statistical computing. Vienna, Austria: R Foundation for Statistical Computing.
Ricketts, T. A., and Hornsby, B. W. (2005). Sound quality measures for speech in noise through a commercial hearing aid implementing digital noise reduction. J. Am. Acad. Audiol. 16, 270–277. doi: 10.3766/jaaa.16.5.2
Ronnberg, J., Holmer, E., and Rudner, M. (2019). Cognitive hearing science and ease of language understanding. Int. J. Audiol. 58, 247–261. doi: 10.1080/14992027.2018.1551631
Ronnberg, J., Lunner, T., Ng, E. H., Lidestam, B., Zekveld, A. A., Sorqvist, P., et al. (2016). Hearing impairment, cognition and speech understanding: exploratory factor analyses of a comprehensive test battery for a group of hearing aid users, the n200 study. Int. J. Audiol. 55, 623–642. doi: 10.1080/14992027.2016.1219775
Ronnberg, J., Signoret, C., Andin, J., and Holmer, E. (2022). The cognitive hearing science perspective on perceiving, understanding, and remembering language: the ELU model. Front. Psychol. 13:967260. doi: 10.3389/fpsyg.2022.967260
Sarampalis, A., Kalluri, S., Edwards, B., and Hafter, E. (2009). Objective measures of listening effort: effects of background noise and noise reduction. J. Speech Lang. Hear. Res. 52, 1230–1240. doi: 10.1044/1092-4388(2009/08-0111)
Smeds, K., Wolters, F., and Rung, M. (2015). Estimation of signal-to-noise ratios in realistic sound scenarios. J. Am. Acad. Audiol. 26, 183–196. doi: 10.3766/jaaa.26.2.7
Sorqvist, P., and Ronnberg, J. (2012). Episodic long-term memory of spoken discourse masked by speech: what is the role for working memory capacity? J. Speech Lang. Hear. Res. 55, 210–218. doi: 10.1044/1092-4388(2011/10-0353)
Souza, P., Arehart, K., and Neher, T. (2015). Working memory and hearing aid processing: literature findings, future directions, and clinical applications. Front. Psychol. 6:1894. doi: 10.3389/fpsyg.2015.01894
Souza, P. E., Boike, K. T., Witherell, K., and Tremblay, K. (2007). Prediction of speech recognition from audibility in older listeners with hearing loss: effects of age, amplification, and background noise. J. Am. Acad. Audiol. 18, 54–65. doi: 10.3766/jaaa.18.1.5
Souza, P., Hoover, E., Blackburn, M., and Gallun, F. (2018). The characteristics of adults with severe hearing loss. J. Am. Acad. Audiol. 29, 764–779. doi: 10.3766/jaaa.17050
Stevens, G., Flaxman, S., Brunskill, E., Mascarenhas, M., Mathers, C. D., and Finucane, M. (2013). Global and regional hearing impairment prevalence: an analysis of 42 studies in 29 countries. Eur. J. Public Health 23, 146–152. doi: 10.1093/eurpub/ckr176
Turton, L., and Smith, P. (2013). Prevalence & characteristics of severe and profound hearing loss in adults in a UK National Health Service clinic. Int. J. Audiol. 52, 92–97. doi: 10.3109/14992027.2012.735376
Turunen-Taheri, S., Carlsson, P. I., Johnson, A. C., and Hellstrom, S. (2019). Severe-to-profound hearing impairment: demographic data, gender differences and benefits of audiological rehabilitation. Disabil. Rehabil. 41, 2766–2774. doi: 10.1080/09638288.2018.1477208
Ward, K. M., Shen, J., Souza, P. E., and Grieco-Calub, T. M. (2017). Age-related differences in listening effort during degraded speech recognition. Ear Hear. 38, 74–84. doi: 10.1097/AUD.0000000000000355
Wendt, D., Hietkamp, R. K., and Lunner, T. (2017). Impact of noise and noise reduction on processing effort: a Pupillometry study. Ear Hear. 38, 690–700. doi: 10.1097/AUD.0000000000000454
Winn, M. B., Edwards, J. R., and Litovsky, R. Y. (2015). The impact of auditory spectral resolution on listening effort revealed by pupil dilation. Ear Hear. 36, e153–e165. doi: 10.1097/AUD.0000000000000145
Wong, L. L. N., Chen, Y., Wang, Q., and Kuehnel, V. (2018). Efficacy of a hearing aid noise reduction function. Trends Hear 22:2331216518782839. doi: 10.1177/2331216518782839
World Health Organization (2001). International classification of functioning, disability and health: ICF. Geneva: World Health Organization.
World Health Organization (2021). World report on hearing. Available at: https://www.who.int/publications/i/item/9789240020481.
Keywords: severe-to-profound hearing loss, hearing aids, noise reduction, intelligibility, listening effort
Citation: Dong R, Liu P, Tian X, Wang Y, Chen Y, Zhang J, Yang L, Zhao S, Guan J and Wang S (2024) Influences of noise reduction on speech intelligibility, listening effort, and sound quality among adults with severe to profound hearing loss. Front. Neurosci. 18:1407775. doi: 10.3389/fnins.2024.1407775
Edited by:
Hannah Keppler, Ghent University, BelgiumReviewed by:
Jerker Rönnberg, Linköping University, SwedenMengfan Wu, University of Nottingham, United Kingdom
Copyright © 2024 Dong, Liu, Tian, Wang, Chen, Zhang, Yang, Zhao, Guan and Wang. This is an open-access article distributed under the terms of the Creative Commons Attribution License (CC BY). The use, distribution or reproduction in other forums is permitted, provided the original author(s) and the copyright owner(s) are credited and that the original publication in this journal is cited, in accordance with accepted academic practice. No use, distribution or reproduction is permitted which does not comply with these terms.
*Correspondence: Shuo Wang, c2hhbm5vbndzaEBhbGl5dW4uY29t