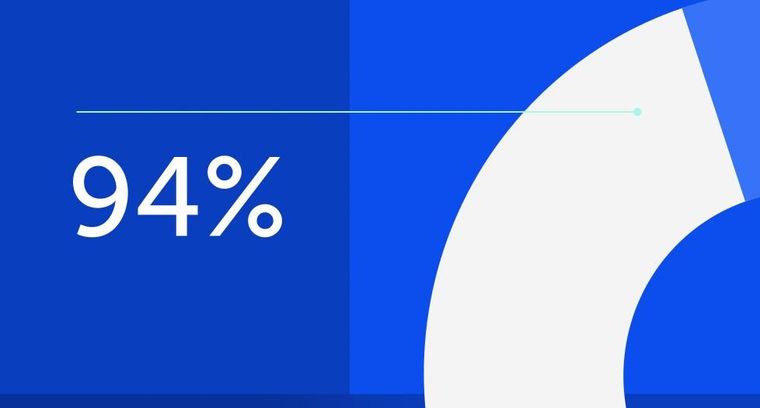
94% of researchers rate our articles as excellent or good
Learn more about the work of our research integrity team to safeguard the quality of each article we publish.
Find out more
REVIEW article
Front. Neurosci., 18 June 2024
Sec. Brain Imaging Methods
Volume 18 - 2024 | https://doi.org/10.3389/fnins.2024.1389651
This article is part of the Research TopicExploration of the Human Brain using Magnetic Resonance Imaging and Spectroscopy with Transcranial Direct Current StimulationView all 10 articles
Transcranial direct current stimulation (tDCS) has been studied extensively for its potential to enhance human cognitive functions in healthy individuals and to treat cognitive impairment in various clinical populations. However, little is known about how tDCS modulates the neural networks supporting cognition and the complex interplay with mediating factors that may explain the frequently observed variability of stimulation effects within and between studies. Moreover, research in this field has been characterized by substantial methodological variability, frequent lack of rigorous experimental control and small sample sizes, thereby limiting the generalizability of findings and translational potential of tDCS. The present manuscript aims to delineate how these important issues can be addressed within a neuroimaging context, to reveal the neural underpinnings, predictors and mediators of tDCS-induced behavioral modulation. We will focus on functional magnetic resonance imaging (fMRI), because it allows the investigation of tDCS effects with excellent spatial precision and sufficient temporal resolution across the entire brain. Moreover, high resolution structural imaging data can be acquired for precise localization of stimulation effects, verification of electrode positions on the scalp and realistic current modeling based on individual head and brain anatomy. However, the general principles outlined in this review will also be applicable to other imaging modalities. Following an introduction to the overall state-of-the-art in this field, we will discuss in more detail the underlying causes of variability in previous tDCS studies. Moreover, we will elaborate on design considerations for tDCS-fMRI studies, optimization of tDCS and imaging protocols and how to assure high-level experimental control. Two additional sections address the pressing need for more systematic investigation of tDCS effects across the healthy human lifespan and implications for tDCS studies in age-associated disease, and potential benefits of establishing large-scale, multidisciplinary consortia for more coordinated tDCS research in the future. We hope that this review will contribute to more coordinated, methodologically sound, transparent and reproducible research in this field. Ultimately, our aim is to facilitate a better understanding of the underlying mechanisms by which tDCS modulates human cognitive functions and more effective and individually tailored translational and clinical applications of this technique in the future.
Non-invasive brain stimulation (NIBS) techniques apply electric fields to the brain using currents injected via scalp electrodes (tES, transcranial electric simulation) or electromagnetic induction (TMS, transcranial magnetic stimulation). They aim to modulate the excitability of the human brain and induce neuroplasticity. NIBS has been ascribed great promise for allowing targeted modulation of specific brain regions or large-scale brain networks relevant for higher cognitive functions. In particular, transcranial direct current stimulation (tDCS) has recently sparked considerable scientific, clinical, and public interest (Dubljević et al., 2014; Riggall et al., 2015). Compared to other types of NIBS (e.g., transcranial magnetic stimulation, TMS), tDCS is relatively low-cost and easy to administer, has no significant adverse effects, and offers a relatively effective mode for placebo (sham) stimulation (Antal et al., 2017a).
The underlying neurophysiological mechanisms of tDCS have been studied extensively in the motor system by utilizing neuropharmacological interventions and TMS, to explore the modulation of cortico-cortical and cortico-spinal excitability (Cirillo et al., 2017). These studies have revealed that the applied current does not induce action potentials. Rather, it is suggested that tDCS transiently shifts the neuronal resting membrane potential toward either de- or hyperpolarization, resulting in enhanced or reduced neural excitability at the macroscale level with standard protocols (Sandrini et al., 2011; Stagg et al., 2018). Moreover, with regard to tDCS effects involving synaptic plasticity, animal and human studies have indicated that tDCS also introduces a secondary mechanism (in addition to alterations of the resting-membrane potential) that involves the induction of long-term potentiation and depression (LTP and LTD)-like processes (Stagg et al., 2018). Repeated stimulation sessions can enhance training-induced adaptive neuroplasticity and induce long-lasting behavioral improvements (Allman et al., 2016; Perceval et al., 2020).
TDCS has also been studied extensively with regard to its potential to enhance cognitive functions in healthy individuals and to treat cognitive impairment in various neurological and psychiatric diseases. However, recent reviews have noted substantial methodological variability, frequent lack of rigorous experimental control and overall, highly variable outcomes within and between studies (Galli et al., 2019; Lee et al., 2021; Lavezzi et al., 2022). Moreover, several recent registered reports have reported weak effects of tDCS, limited intra-individual reliability of tDCS responses or failed to replicate previous studies (Boayue et al., 2020; Alexandersen et al., 2022; Willmot et al., 2024). Nonetheless, a substantial body of research has demonstrated potential positive effects of tDCS on behavior and brain function, but the aforementioned issues also suggest that the current “state-of-the-art” may not yet be suited for translational applications. This would require systematic and coordinated evaluation of the parameter space, clarification of the underlying neural mechanisms, and likely individual adaptation of interventions based on this knowledge. Here, we review and critically discuss recent efforts that aim to address these important issues to improve the effectiveness of tDCS in experimental and clinical settings, as well as transparency and reproducibility of research outcomes in this field. We also aim to provide recommendations for future research investigating the neural mechanisms underlying tDCS effects on higher cognitive functions using neuroimaging technology (see Table 1 for a summary of the recommendations).
Approximately 80% of the published tDCS studies target the primary motor cortex (M1), and it is currently unclear if results from these studies generalize to other cortical regions and brain networks, in particular those enabling higher cognitive functions (Stagg et al., 2018). Moreover, while neurophysiological effects of tDCS on local cortical excitability in the motor system can be assessed directly via modulation of TMS-induced motor evoked potentials (MEPs), this approach cannot be used to quantify neural effects of tDCS on cognition. Contemporary systems neuroscience research has also highlighted that cognitive functions are enabled by large-scale functional brain networks relying on coordinated processing across various regions (Breakspear, 2017; Pang et al., 2023). To date, however, there is still relatively little knowledge about how tDCS impacts these complex human brain networks, which can be addressed by combining tDCS with modern brain imaging techniques.
In principle, tDCS can be combined with different functional imaging techniques, including electroencephalography (EEG, Polanía et al., 2011; Okazaki et al., 2023), magnetoencephalography (MEG, Jang et al., 2017; Matsushita et al., 2021), functional near-infrared spectroscopy (fNIRS, McKendrick et al., 2015; Dutta, 2021) or functional magnetic resonance imaging (fMRI, Esmaeilpour et al., 2020; Jamil et al., 2020). However, combining tDCS with imaging technology requires careful consideration of appropriate designs and also decisions about which approach is best suited to answer specific research questions.
For example, imaging can be conducted either sequentially (before and after tDCS) or concurrently with tDCS. Sequential imaging allows the investigation of potential after-effects of the stimulation on brain function (Keeser et al., 2011; Shahbabaie et al., 2018) or can be used to interrogate the neural consequences of behavioral add-on effects when tDCS is administered during multiday training sessions or therapeutic interventions (Allman et al., 2016; Antonenko et al., 2023). In contrast, concurrent imaging and tDCS allows investigation of immediate tDCS effects on brain function (Esmaeilpour et al., 2020).
With regard to imaging modalities, EEG and MEG allow mapping of brain dynamics with excellent temporal resolution, which renders them optimal for investigating modulation of fast neural oscillations (Rossi et al., 2022). MEG and fNIRS are most sensitive to modulation of cortical regions (Attal et al., 2012; Li et al., 2023), and therefore less suited to investigate potential subcortical stimulation effects. In this review, we will focus on fMRI, because it allows investigating tDCS effects on brain dynamics with high spatial precision and sufficient temporal resolution across the entire brain. In addition, high resolution structural imaging data can be acquired in the same imaging session, allowing precise localization of potential stimulation effects, verification of correct electrode positions on the scalp and realistic current modeling based on individual head and brain anatomy (Hunold et al., 2023). These advantages have turned fMRI into the most widely used imaging technique to investigate the neural mechanisms underlying tDCS (Esmaeilpour et al., 2020), including pioneering intrascanner work that demonstrated acute modulation of ongoing brain activity at the stimulation site and large-scale neural networks (Meinzer et al., 2013; Antonenko et al., 2017; Jamil et al., 2020).
Since the reintroduction of tDCS at the turn of the 20th century (Priori et al., 1998; Nitsche and Paulus, 2000), numerous publications have reported promising effects of tDCS on motor and cognitive functions in health and disease, but also substantial intra- and interindividual variability of stimulation effects. This has prompted increased interest in investigating the underlying sources of variable tDCS responses, that are thought to be multifactorial and can broadly be classified as participant- and stimulation-dependent factors (Fertonani and Miniussi, 2017). In addition, developing optimal designs for either tDCS and fMRI studies can be challenging in itself. This is further complicated when both techniques are combined and design optimization may require creative solutions and specialist input from different fields. This will be discussed in the following sections.
Participant-dependent factors include trait- and state-dependent characteristics of the participants, including baseline behavioral performance, microstructural, metabolic and functional brain network variations between participants or intraindividual differences in intrinsic brain states of each participant at different stimulation sessions (Hordacre et al., 2017; Aberra et al., 2018; Antonenko et al., 2019a). The importance to account for these factors has been emphasized by studies showing that the tDCS response can be associated with demographic, behavioral, or neurofunctional characteristics of participants, including sex, age, education levels, genetics, cultural background, baseline task performance and neural network organization (Kuo et al., 2006; Berryhill and Jones, 2012; Martin et al., 2017b, 2019b; Antonenko et al., 2018; Fridriksson et al., 2019; Perceval et al., 2020; Ghasemian-Shirvan et al., 2022). Moreover, electric field modeling studies that considered individual head and brain anatomy have demonstrated associations between regional electric field strength and modulation of behavior, neurophysiological parameters, fMRI-derived brain networks, regional cerebral blood flow and neurochemical parameters (Kim et al., 2014; Cabral-Calderin et al., 2016; Antonenko et al., 2017, 2023; Jamil et al., 2020). These studies have highlighted the contribution of various participant characteristics to variable tDCS responses that require more systematic investigation in the future.
On the other hand, many stimulation-dependent factors, like the timing, intensity or duration of tDCS are determined by a given experiment. Yet, even minor modifications to experimental protocols can alter the outcomes (e.g., Gauvin et al., 2017), thereby contributing to differences between studies. However, many of these factors can also interact directly with participant characteristics. For example, while the intensity of the induced current was held constant in the majority of previous tDCS studies, it has been convincingly suggested that individual skull (Datta et al., 2009; Bikson et al., 2012; Hanley and Tales, 2019; Sun et al., 2021), or brain anatomy (Suh et al., 2012; Dahnke et al., 2013; Filmer et al., 2019), critically determine how much current reaches the target regions for tDCS, resulting in variable current dose in the target regions. Moreover, accurate positioning of the electrodes on the participants’ scalp, one of the most critical stimulation dependent factors, can be affected by experimenter error (i.e., electrode misplacement) or incremental drift over the course of the experiment, resulting in current flow variations between participants (Woods et al., 2016; Indahlastari et al., 2023). This issue might be even more relevant for focal tDCS set-ups, that constrain the current flow to circumscribed brain regions (Villamar et al., 2013; Gbadeyan et al., 2016b), because the regional specificity of the administered current renders these setups particularly vulnerable for deviations from intended electrode positions, resulting in reduced current dose in target regions for tDCS (Niemann et al., 2024).
Of note, complex intrascanner tDCS-fMRI studies that require participants to walk to and be positioned inside the scanner with electrodes attached, are at high risk for electrode displacement and this effect may vary depending on target sites (e.g., electrodes positioned underneath the cushions that are used to stabilize the head may be more likely to move). Specific problems pertaining to mis- and displacement of electrodes during tDCS-fMRI studies and subsequent verification of correct electrode positions are illustrated in Figure 1, along with suggestions how to minimize them. Therefore, future research in this field should routinely implement appropriate methods not only for improving electrode positioning prior to scanning (e.g., electrode placement guided by neuronavigation) (De Witte et al., 2018), but also implement methods to minimize electrode displacement and drift, verify electrode positions before and/or after functional imaging, and consider empirically determined actual electrode positions when dose–response relationships are investigated (Woods et al., 2015; Knotkova et al., 2019; Antonenko et al., 2019b; Albizu et al., 2023; Niemann et al., 2024).
Figure 1. Illustrates factors that can affect electrode position accuracy during concurrent fMRI-tDCS experiments and subsequent verification. Suggestions are based on our own experience during an ongoing multicenter, intrascanner tDCS-fMRI project (www.memoslap.de/). The upper panel shows the different phases of a concurrent tDCS-fMRI study and subsequent identification of electrode positions based on structural images of individual participants. Below, examples for potential sources of errors (middle panel) and possible solutions (bottom panel) are described. An example for a method to assure constant spacing of electrodes for a 3 × 1 focal montage that uses a 3D printed spacer can be found in Niemann et al. (2024). Because verification of electrode positions based on 3D structural images can be challenging, transformation into a 2D “pancake view” of the reconstructed scalp may be suited to facilitate identification of actual scalp positions of tDCS electrodes (De Munck et al., 2013; Fleury et al., 2019). Fully automated methods based on neural network models are currently being developed by our group.
Investigating and controlling for variability in tDCS-fMRI studies can be challenging because stimulation effects are not assessed by a direct marker of brain physiology (i.e., MEPs), but rely on proxy measures of brain function (i.e., variable behavioral performance in task-related fMRI and indirect measures of neural activity, like the blood oxygenation-dependent response). Behavioral performance parameters can be significantly influenced by numerous internal and external factors. For example, improvements in performance across repeated sessions have been linked to familiarization and training effects, or the development of cognitive strategies (Bell et al., 2018). These confounds are particularly relevant for cross-over designs frequently used in tDCS-fMRI studies and can result in reduced effect sizes, even when the stimulation conditions are appropriately balanced across participants (Falleti et al., 2006; Hausknecht et al., 2007; Bartels et al., 2010). While these confounds can be mitigated to some degree by choosing robust designs and implementation of parallel task versions, it is advisable to formally establish test–retest reliability of experimental paradigms in the specific target populations (e.g., young vs. older individuals, clinical populations of interest) prior to implementation in costly tDCS-fMRI studies and to consider the outcomes when interpreting effect sizes of behavioral and neural modulation.
Moreover, specific paradigms or populations are particularly challenging for tDCS-fMRI studies. For example, learning paradigms are typically associated with performance increases across time, therefore the number of correct responses and errors vary depending on learning stage. Inclusion of both response types in the analysis can be problematic, because the neural signatures differ (Postman-Caucheteux et al., 2010). Moreover, restricting the analysis to correct trials reduces statistical power to detect learning specific neural activation in early stages or in different tDCS conditions. One possible solution for this problem was suggested by Sliwinska et al. (2017), who used an associative picture-word learning paradigm and provided feedback about correct associations after each trial. This feedback-based design assured that “learning” was possible even following incorrect responses, thereby allowing to investigate a common neural process across all trials. Moreover, behavioral responses can be highly variable per se in certain populations, which can mask potential neural tDCS effects. This was addressed by Darkow and Flöel (2016), who investigated tDCS effects during a picture naming task in patients with chronic language impairment (aphasia). In this study, only object pictures that could be named consistently by the patients across several baseline naming assessments were used. This maximized the number of correct responses during a subsequent cross-over tDCS-fMRI study and allowed imaging of the neural effects of tDCS on residual language networks, independent of performance.
Another important aspect specific to imaging of tDCS effects pertains to the robustness of the imaging procedure itself, that can be affected by a number of different factors including physiological noise due to cardiac and respiratory cycles, head motion artifacts, magnetic field inhomogeneities, and fMRI signal drift. These factors can contribute to variability in cross-over and longitudinal tDCS-fMRI studies and need to be monitored and considered in data analysis (Esmaeilpour et al., 2020). However, certain aspects of tDCS-fMRI studies can also be addressed at the design level. For example, fMRI signal drift depends on gradual heating of the MRI scanner and can be controlled for to some extent by scanning participants at the same time of day or by “warming up” the scanner prior to each session. However, this does not address within-session effects, i.e., signal changes from the early to later stages of a paradigm. An elegant solution to this problem was suggested by Sliwinska et al. (2017) in their picture-word association learning paradigm. The authors grouped learning trials in a way that different stages of the learning process (i.e., low vs. higher proficiency) were achieved repeatedly across consecutive micro-blocks, thereby minimizing the effect of signal drift on fMRI activity for each learning stage. Notably, numerous other factors have been shown to reduce reliability in task-related imaging protocols, including participant characteristics (e.g., advanced age, clinical populations > young healthy participants), design (e.g., long > short retest intervals; event-related > block designs) or specifics of data analysis (e.g., univariate or region-of-interest > multivariate analyses, complex > simple contrasts; for review see Noble et al., 2021). Because these factors can also increase variability in tDCS-fMRI studies, they require careful consideration at the design stage, to minimize the risk of masking potential tDCS effects on behavior and brain functions.
Additional consideration pertains to imaging artifacts that are induced by conventional and focal set-ups. While image distortions are typically limited to the scalp and skull, signal-to-noise (SNR) reductions in the functional images, that are most pronounced underneath the location of the electrodes, have been reported (Saiote et al., 2013). SNR reductions are most pronounced for the comparison between images acquired with and without electrodes (Gbadeyan et al., 2016b), but occur to a lesser degree also for the comparison of active vs. sham conditions and may vary between brain regions (Antal et al., 2011; Holland et al., 2011). Therefore, careful quantification of potential imaging artifacts and SNR reductions associated with specific equipment and target regions is necessary, and the outcomes should be considered in the design of the study. For example, when effects of different active stimulation sites are of interest, the between site effect needs to be controlled by comparison with its own sham condition with a similar regional SNR profile.
In sum, investigating the variability underlying tDCS effects using fMRI requires careful consideration of generic issues relevant for each individual approach (e.g., robustness of designs, accurate placement of electrodes), but also those that are specific to their combined use (e.g., imaging artifacts induced by the tDCS set-up, drift of electrodes during imaging, design optimization for imaging and tDCS), which can be challenging. However, tDCS-fMRI approaches also offer unique opportunities to tease apart the contribution of participant-, stimulation- and design-dependent factors on the variability of tDCS effects (e.g., structural MRI allows to estimate effects of anatomical variability on current flow patterns; comparison of tDCS administered during task performance or rest investigate neural stimulation effects on a constrained vs. unconstrained set of brain regions), thereby contributing to the development of future individualized tDCS approaches with potential to enhance the effectiveness of this technique in experimental and translational human neuroscience.
The specific montages used to administer tDCS (e.g., conventional vs. focal set-ups, size and positioning of electrodes) affect the intensity and distribution of the induced current. Conventional set-ups use relatively large electrodes (e.g., 5 × 7 cm, up to 10 × 10 cm) that are typically attached over cortical regions in different hemispheres and induce a relatively wide-spread current flow affecting multiple neural networks. This lack of focality renders them less desirable for revealing regionally specific, causal brain-behavior relationships compared to focal montages. Those use smaller and often concentrically arranged electrodes in the same hemisphere to constrain the current to circumscribed brain regions (Kuo et al., 2013; Bortoletto et al., 2016). Notably, conventional setups may be more resilient to positioning errors and electrode drift compared to focal set-ups and electrode displacement has been shown to result in physiologically significant reductions in current dosage specifically within the immediate target regions (Niemann et al., 2024). This is particularly relevant for tDCS-fMRI studies, that are at high risk for electrode displacement, e.g., due to positioning of participants in the scanner after electrode attachment (see discussion above). Conventional set-ups may also have advantages in contexts where experimenter error is more likely to occur (e.g., routine clinical care, multicenter intervention studies) or specific clinical populations with variable lesion patterns and functional reorganization (Darkow et al., 2017). Therefore, the choice of montage in tDCS-fMRI studies strongly depends on the specific research question and population.
Furthermore, the majority of tDCS research has relied on the 10–20 (or 10–10) EEG system to identify target brain regions (Thair et al., 2017). This approach involves manual or automatic identification of anatomical markers (e.g., nasion, inion, preauricular points) and additional measurements (e.g., head circumference, calculation of intersections between landmarks) to determine the intended scalp positions of electrodes. Depending on which system is used, 25 or 74 reference points are available and placement is often guided by electrode caps (Tsuzuki et al., 2016). While this approach considers head size of individual participants to some degree, other properties of brain and skull morphology are neglected, resulting in a loss of precision (Herwig et al., 2003; De Witte et al., 2018). Structural MRI-guided neuro-navigation is a more individualized localization technique, which has mainly been used in experimental and clinical TMS studies, but more recently also for positioning of tDCS electrodes (De Witte et al., 2018; Lioumis and Rosanova, 2022). This approach requires a high-resolution structural T1-weighted image of individual participants that can be acquired prior to a tDCS-fMRI study. By co-registration of the structural image with a three-dimensional brain model and use of specific soft- and hardware for identifying the target brain regions, electrode positioning accuracy relative to individual brain anatomy can be improved (De Witte et al., 2018). Because of significant variations in brain anatomy and head shape, individualized neuronavigation-based electrode placement is currently the best option to improve positioning of electrodes at the intended scalp positions in tDCS-fMRI studies and scalp-based approaches are discouraged, especially for focal set-ups.
Individual differences in brain anatomy are crucial not only for precise placement of electrodes but also for optimizing the distribution of tDCS-induced electric fields within individual brains (Kim et al., 2014; Bikson et al., 2015; Antonenko et al., 2021). Previous studies have also highlighted the importance of investigating fundamental aspects of the induced electric field, such as current strength, focality, and its dependency on anatomical features of the head (Edwards et al., 2013; Opitz et al., 2015; Saturnino et al., 2015). In this context, computational models are frequently used to estimate the strength of the cortical electric field, since direct measurement in the human brain is not feasible except in highly selected patient populations (e.g., tumor resection, brain surgery for epilepsy treatment; e.g., Huang et al., 2017).
Recently, individualized electric field calculations have allowed investigating correlations between the individually received physical stimulation dose and the physiological impact of tDCS (for review see Hunold et al., 2023), known as cortical dose–response relationship. Moreover, manufacturers of brain stimulation devices are increasingly interested in updating their devices’ capabilities to estimate the electric field via computer modeling techniques, which rely on electrode positioning and stimulation current intensity. For instance, Soterix HD-Explore1 is a commercial, stand-alone software that models the current flow using the finite element method to estimate the electric field distribution for complex tDCS set-ups. However, it is essential to note that computer simulations estimate the electric field based on assumptions about electrical conductivity of different tissue classes and that they depend on the anatomical accuracy of the (semi-)automatic tissue segmentations obtained from the MR images. Therefore, validation of these assumptions and assessment of the segmentation accuracy is critical to improve the accuracy of computational models, and simulation errors may obscure potential associations between estimated fields and the recorded behavioral or neural response. In this context, Magnetic Resonance Current Density Imaging (MRCDI) and Magnetic Resonance Electrical Impedance Tomography (MREIT) are emerging techniques to investigate tDCS-induced current flow conductivity of brain tissue during concurrent tDCS-MRI measurements, respectively (Göksu et al., 2018, 2019). These modalities have potential for validating tDCS electric field simulations and optimizing individualized current dose calculations in both healthy participants and patients.
Another issue that has recently gained substantial attention in tDCS studies is dose control. In this context it is important to note that even when the same current intensity is applied, participant-specific factors like skull and brain anatomy and others are major determinants of the actual current dose arriving in the target regions (Evans et al., 2020; Antonenko et al., 2021). This is illustrated in Figure 2. Hence, recent studies have used computational current modeling approaches to optimize stimulation intensity across participants. For example, Caulfield et al. (2020) acquired structural MRI data of individual participants to compute a “reverse-calculated tDCS dose” of tDCS applied at the scalp required to induce a uniform E-field (arbitrarily set at 1.00 V/m and not yet empirically tested in a prospective stimulation study) in a region-of-interest in the primary motor cortex. Notably, the minimum current intensity threshold for physiological modulation in different brain regions is unknown and may vary between stimulation sites and individuals. Moreover, increasing intensity does not necessarily increase neurophysiological effects and even changes in polarity have been observed. For example, while cathodal tDCS with 1 mA and 3 mA induced inhibitory effects, 2 mA may result in enhanced excitability (Batsikadze et al., 2013; Mosayebi Samani et al., 2019).
Figure 2. Computational models of tDCS-induced electrical current flow based on structural MRI data of three participants and a conventional montage targeting the left primary motor cortex (anode). Cathodes are positioned over the contralateral supraorbital region. Colors illustrate the distribution and intensity of the current (electric field strength, V/m). Only the left side of the brain is shown. (A) When individual head and brain anatomy is considered in computational models of current flow, the same intensity (i.e., tDCS administered with 2 mA) can result in highly variable current flow in individual participants. (B) Provides a simple example of dose control. Simulations were conducted with different intensities (i.e., 2.6, 2.0, and 1.5 mA) to minimize differences between participants.
Therefore, currently little is known about objective optimization criteria for field intensity (Lee et al., 2021). Moreover, reaching a pre-determined criterion may require intensities beyond accepted safety thresholds in individual participants (Antal et al., 2017a) and in the study above, the required current intensity across participants ranged from 3.75–9.74 mA (Caulfield et al., 2020). Therefore, alternative approaches are currently being developed that use a combination of individual electric field modeling and anatomical information to enhance regional precision (Rasmussen et al., 2021) or the development of prospective dosing strategies aimed at matching the average field dose in different target regions at the group level, while maintaining dose variability for each region to enable systematic tests of dose–response relationships (Saturnino et al., 2021). However, in order to be valuable, optimization approaches should be informed by multiple sources (e.g., underlying anatomy, computational estimation of field magnitude, focality or functional task-dependent activity for optimization of stimulation targets) rather than being unidimensional.
Achieving high-level experimental control is crucial not only to ensure reliable and valid results, but also to establish causal relationships in tDCS studies. In this context, we will discuss two major aspects relevant to tDCS-fMRI studies: (a) blinding of participants and research staff and (b) design-related issues pertaining the establishment of valid assumptions about the relationship between brain stimulation and behavioral and neural modulation. A separate section, we will discuss “control” from a broader perspective, i.e., in the context of open science practices and recent efforts to increase the validity, reproducibility and transparency of empirical research, which is highly relevant for tDCS-fMRI studies.
The majority of previous tDCS studies have relied on placebo (“sham”) tDCS conditions to control for placebo effects. Sham-tDCS typically involves gradually increasing the current to the target intensity (e.g., over 10 s), followed by an immediate or briefly delayed fade-out period, during which the current intensity is decreased to zero (Huey et al., 2007; Axelrod et al., 2015; Jaberzadeh et al., 2019). Sham-tDCS protocols intend to elicit a transient physical sensation on the scalp (e.g., tingling, itching) that closely resembles the sensation experienced during active stimulation, but without inducing physiologically relevant effects on brain function due to the short duration of the stimulation. Sham protocols have been suggested to allow for effective blinding of participants (Gandiga et al., 2006) without modulating corticospinal excitability, CSE (Dissanayaka et al., 2018). However, several recent studies have also questioned blinding efficacy of specific sham-tDCS protocols (O’Connell et al., 2012; Wallace et al., 2016; Greinacher et al., 2019; Turi et al., 2019). For instance, Greinacher et al. (2019) probed blinding integrity every 30 s during a low-intensity active or sham tDCS protocol (30 s ramp-up/down, 600 vs. 20 s active 1 mA M1-tDCS) and demonstrated that participants could identify active tDCS in approx. 60% of the probes with high confidence.
These findings have recently led to the development of novel sham protocols that minimize differences between active and sham conditions in tDCS studies. For example, Neri et al. (2020) introduced a new sham-tDCS approach for multi-electrode tDCS that used computational current modeling to optimize electrode positions during sham in a way that zero or very low magnitude electric fields are delivered to the brain, while medium to high intensity currents are maintained in at least some scalp electrodes. Notably, participant blinding for this new approach was superior compared a conventional bifocal montage and the desired blinding effect was achieved without eliciting a significant effect on CSE (Neri et al., 2020). These findings suggest that blinding efficacy reported for conventional sham protocols may need to be interpreted with caution (O’Connell et al., 2012) and alternative protocols that minimize differences between active and sham-tDCS may be more appropriate for achieving participant blinding.
In addition, rigorous staff blinding is crucial for preventing experimenter effects, such as the introduction of selection bias, observer bias, or inadvertent effects on experimental outcomes or during data analysis (Grimes and Schulz, 2002; Holman et al., 2015). Blinding of experimenters conducting the experiment and interacting with participants can be difficult with some commercially available stimulators (e.g., with indicator lights or sounds indicating on vs. off conditions), unless a two-experimenter approach is adopted: one administering the stimulation, while the second remains blinded while interacting with the participant (Reinhart et al., 2017). However, the majority of modern stimulators can now be equipped with advanced study modes, enabling easy customization for various stimulation conditions and parameters that can be triggered by pre-assigned codes. These new developments minimize the risk of unintentional unblinding of the experimenter and it is highly advisable to use such approaches in tDCS-fMRI studies. In addition, blinding of staff during data analysis is also advised and can be achieved by masking the stimulation conditions (i.e., by using participant codes that do not reveal active and control conditions).
The final issue pertains to how blinding is assessed. Here, a common practice involves a post-stimulation questionnaire, serving two key purposes: (a) directly valuating participants’ capacity to differentiate between stimulation conditions, which is frequently complemented by (b) self-reported assessment of potential side effects (e.g., tingling or burning, changes in mood or concentration levels) experienced during the stimulation (Ambrus et al., 2012; Turi et al., 2019). Although end-of-study questionnaires have been considered valid measures for evaluating the effectiveness of blinding (Antal et al., 2017b), a recent study by Turner et al. (2021) reported that the accuracy of end-of-study guesses was not more reliable than chance in predicting participants’ ability to distinguish between active or sham tDCS. Hence, it was suggested to incorporate additional online probe questions during the stimulation process for more accurate evaluation of blinding efficacy if possible. In any case, careful documentation of methods and results of participant and staff blinding is essential in all tDCS studies (Ekhtiari et al., 2022).
Another aspect particularly relevant for tDCS-fMRI studies pertains to establishing causal relationships between stimulation effects and behavioral and neural modulation. This cannot be achieved by comparing the effects of active vs. sham tDCS alone, because the latter only controls for potential placebo effects. In principle, stronger causal assumptions for the relevance of a given brain region to specific behavioral outcomes are potentially possible by additional direct comparison of anodal-excitation and cathodal-inhibition effects (AeCi). However, AeCi effects have rarely been demonstrated for cognitive tasks, mainly due to relatively weak or variable inhibitory effects of cathodal tDCS on cognition, which may be explained by redundancy within the neural networks supporting higher-order cognitive functions (Jacobson et al., 2012). Moreover, cathodal tDCS has also been shown to enhance performance during specific tasks, presumably by enhancing signal-to-noise during cognitive tasks (Antal et al., 2004).
However, several other approaches to achieve high-level experimental control in tDCS-fMRI studies are suitable and depend on the specific research question. For example, regional specificity of tDCS effects can be investigated by including one or more additional active control stimulation sites, specifically targeting cortical regions outside of the neural network(s) involved in processing of the task of interest. This approach not only allows to investigate unspecific (placebo) effects, but also the specificity of neural network modulation relative to the respective task. For example, Gbadeyan et al. (2016a) investigated behavioral effects of focal tDCS administered to either the left or right dorsolateral prefrontal cortex (dlPFC) and M1 during a visual Flanker task. Prefrontal active vs. sham tDCS improved adaptive cognitive control, thereby confirming involvement of both left and right dlPFC in this specific process. The absence of stimulation effects after left or right M1 tDCS demonstrated regional specificity. Notably, higher-order cognitive functions are often supported by multiple brain regions that are organized in partially overlapping neural networks. Therefore, selecting suitable and meaningful active control sites can be challenging.
Regional specificity can be complemented by the investigation of task specificity of tDCS effects. At the lowest level of control, the latter involves two or more different tasks, that are completed while the same cortical region is stimulated. This allows controlling for unspecific effects of the stimulation and demonstration that a given region or network is involved in task A, but not B. For instance, Martin et al. (2017a) demonstrated improved performance in a visual perspective taking task when focal anodal tDCS was administered over the dorsomedial frontal cortex (dmPFC). No significant change was observed in a source memory task with the same tDCS intervention, which illustrates a simple case of task-specificity of tDCS effects. Another highly specific aspect of task specificity pertains to activity selective stimulation effects (Bikson et al., 2013). This implies that even though several brain regions may be affected by the current, only those activated by a specific task are susceptible to the effects of tDCS. To the best of our knowledge, this assumption has not yet been tested with functional imaging. In principle, this could be investigated with a conventional montage that induces relevant current in neighboring brain areas that are differentially activated by two tasks. In this context, it would be predicted that the same montage preferentially modulates activity in the respective task-relevant regions and networks.
Moreover, one of the highest levels of experimental control at the design stage can be achieved by the combined investigation of regional and task specificity. This requires a minimum of two stimulation sites targeting processes or neural networks relevant for different tasks. For example, Martin et al. (2019a) investigated effects of focal tDCS administered to either the right TPJ or dmPFC on social cognition, including visual perspective taking tasks requiring line-of-sight and mental rotation judgments. Using this approach, the authors demonstrated a double dissociation of behavioral tDCS effects, indexed by specific facilitation of embodied mental rotation of the self into an alternate perspective by rTPJ tDCS, while dmPFC tDCS facilitated integration of social information relevant to self-directed processes.
Finally, analysis of specificity is not limited to tasks and regions, but also applies to the timing of the stimulation relative to a given task (temporal specificity). The majority of previous studies have employed single tasks and investigated behavioral effects of tDCS administered at different time points (i.e., prior to, during or after the task). These studies have highlighted that maximal tDCS effects may be achieved with varying timing across different functional domains, including visuomotor and visuospatial skills (Reis et al., 2015; Oldrati et al., 2018), motor network modulation (Calzolari et al., 2023) and language processing (Cao and Liu, 2018). Moreover, timing specific neurophysiological or behavioral modulation have been reported in different populations (i.e., young vs. older adults, for review see Perceval et al., 2016). Hence, these factors also need to be considered in the design phase of future tDCS-fMRI studies, e.g., by establishing optimal stimulation time windows in prior behavioral studies.
From a broader perspective, high-level control also includes the promotion of open and transparent research practices (Munro and Prendergast, 2019). For example, fMRI data analysis is a complex process that can be accomplished using a variety of platforms and analytical approaches that frequently comprise custom code. This was highlighted by Botvinik-Nezer et al. (2020), who demonstrated that of 70 labs that were asked to analyze the same fMRI dataset, all used different workflows. In the context of the ongoing replication crisis in science (Open Science Collaboration, 2015), appropriate documentation of data analysis procedures, code, and optimally pre-registration of analytical steps is highly desirable when investigating tDCS effects using imaging. This will facilitate the interpretation of the results and enhance the validity of research in this field (Esmaeilpour et al., 2020). Transparency, reproducibility and availability of data and analytical approaches can be further enhanced by adhering to relevant guidelines for data analysis and sharing (e.g., Gorgolewski and Poldrack, 2016; Nichols et al., 2017) and the FAIR (Findable, Accessible, Interoperable, Reusable) guiding principles for scientific data management and stewardship (Wilkinson et al., 2016).
Another important issue pertains to publication bias favoring positive outcomes, either because researchers do not attempt to disseminate negative results, or are discouraged by publishers to submit even valid negative findings. A particularly powerful approach to prevent publication bias are registered reports, where a study is accepted for publication based on its merit to answer a specific research question, irrespective of the eventual outcomes (that will be published alongside the protocol after study completion). Unlike pre-registration, the methodology and hypotheses of the planned study undergoes peer review, which helps to prevent publication of negative results based on methodological flaws (Chambers and Tzavella, 2021). Pre-registered reports are particularly relevant in the context of recent meta-analyses and systematic reviews of tDCS effects on human cognition (Galli et al., 2019; Yan et al., 2020; Figeys et al., 2021; Majdi et al., 2022), that have discussed not only limited reproducibility and small sample sizes in this field, but also the risk of p-hacking or HARKing (i.e., Hypothesizing After the Results are Known). Pre-registrated reports effectively address these biases and also provide a robust foundation for hypothesis-driven research and confirmatory replication. Notably, several hundred of journals now offer this option, including high profile neuroscience and neuroimaging outlets that are of interest for tDCS-fMRI studies (e.g., Nature Communications, NeuroImage, Cortex). Nevertheless, we would also like to emphasize the importance of data-driven and exploratory analyses. This particularly relevant in a relatively novel and evolving fields of science like tDCS-fMRI and the multitude of parameters that can influence stimulation success. In this context, clearly stated exploratory analyses can generate new hypotheses and serve as starting points for subsequent confirmatory studies.
Translational tDCS research that aims at counteracting age-associated decline or impairment of cognitive functions has yielded promising but mixed results so far (e.g., Perceval et al., 2016). For example, studies that directly compared tDCS effects in young and older adults have demonstrated larger behavioral effects in younger (Ross et al., 2011; Manenti et al., 2013), while others revealed larger effects in older adults (Zimerman et al., 2014; Cespón et al., 2017; Perceval et al., 2020). Moreover, while some studies have suggested that tDCS can improve (impaired) performance in older adults to the level of younger adults (Meinzer et al., 2013), others found detrimental effects in older adults when using the same montage that improved behavioral functions in young adults (Boggio et al., 2010; Fertonani et al., 2014). These findings are not surprising, because the neural substrates that support cognition and motor function in young and older adults can differ substantially and therefore, positive results obtained in tDCS studies involving young individuals cannot automatically be translated to older populations (Perceval et al., 2016). This needs to be investigated more systematically in the future across functional domains and the entire human lifespan.
Furthermore, neural aging, which for most cognitive domains becomes apparent by the end of the third decade of life (e.g., Hedden and Gabrieli, 2004), is not a uniform process and the degree of functional and structural brain reorganization is influenced by a number of intra-individual, environmental and life-style factors (Gutchess, 2014). In addition, age-related changes in brain structure, cerebrospinal fluid/brain ratio or skull thickness may affect the degree or distribution of the induced current itself (Opitz et al., 2015; Indahlastari et al., 2020; Antonenko et al., 2021). These aging-related factors may explain differences in stimulation response between young and older individuals, but also variability of stimulation effects within older population. To date, however, it has not yet been systematically investigated how potential differences in current flow due to brain atrophy or other factors, reported to significantly impact current flow based on simulation studies (Indahlastari et al., 2020), and interactions with age-associated functional network reorganization, affect behavioral and neural tDCS effects. TDCS-fMRI approaches are particularly suited to investigate these important issues, because they can provide information on both baseline neural network organization and functional changes due to the stimulation. Moreover, structural imaging data can be acquired in the same session and subsequently be used for individualized current modeling. Consideration of these variables in data analysis has great potential to reveal the underlying mechanisms and predictors of stimulation response across the human lifespan.
Finally, many neurological conditions (e.g., stroke-induced motor or cognitive impairment, dementia and precursors) primarily occur in elderly individuals, thereby a pathological process is superimposed on “normal” age-associated structural and functional brain reorganization. Hence, a better understanding of how these age-associated brain changes interact with tDCS also has direct implications for enhancing the clinical application of this technique in the future (Crosson et al., 2015).
Developing research consortia has yielded unprecedented insights and facilitated discovery research in many fields of basic and translational neuroscience (e.g., Human Brain Project2; ENIGMA3), by strengthening of research capacity through pooling of resources and expertise and generating standardized outcomes and solutions for a common set of questions (Tagoe et al., 2019).
To date, however, a significant proportion of tDCS studies have been limited by small sample sizes and highly variable methodological approaches (Minarik et al., 2016; Hiew et al., 2022). Given the vast parameter space of tDCS experiments (e.g., montage, current intensity, target region, polarity, control condition; Sergiou et al., 2020; Kurtin et al., 2021), there are rarely comparable studies with similar protocols, precluding definitive conclusions regarding the effects of tDCS on cognition or establishing optimal protocols for specific research questions. Consortia can effectively address these issues by facilitating participant recruitment from multiple contributors using coordinated methodology and outcome measures. The increased diversity of the sample can in turn increase the generalizability of research findings and concurrent recruitment of participants expedites data acquisition, thereby accelerating the research process. This is currently being addressed for the first time by a recently funded project in Germany, that employs highly coordinated tDCS-fMRI and computational approaches to systematically investigate the underlying neural mechanisms and predictors of tDCS effects on learning and memory across different functional domains and the human lifespan. This consortium will also play an important educational role by providing training opportunities for junior scientists and researchers through high-quality brain stimulation workshops and conferences, to foster knowledge exchange, skill development, and networking. Notably, a similar global approach has been initiated by the International Network of Neuroimaging Neuromodulation (INNN), a group comprising experts and early-mid career researchers, that conducts regular workshops and education seminars that are publicly available via a YouTube channel.4
Furthermore, consortia play a vital role in monitoring and standardizing the execution and reporting of tDCS interventions. Importantly, combining tDCS with fMRI requires specific equipment, poses multiple technological challenges (e.g., safety assurance and management of potential imaging artifacts introduced by the equipment). Consequently, research consortia should aim to bring together expertise not only from brain stimulation, but also invite collaborators from additional relevant fields with specific expertise in neurophysics, engineering, neuroimaging methodology and data analysis and also data management, to further strengthen this field. These approaches will be highly relevant, because development of large-scale coordinated tDCS-fMRI datasets will require advanced and automated analytical procedures like machine learning and other data-driven approaches. For example, machine learning algorithms have shown great promise in predicting tDCS response in small scale studies based on a variety of factors, including current intensity and direction (e.g., Albizu et al., 2020). Adapting and fine-tuning these methods for the use in large-scale samples will likely be the next frontier in increasing our understanding of the neural mechanisms and predictors of tDCS response.
Finally, the lack of sufficient methodological and procedural information frequently hinders reproducibility and further advances in this field (Esmaeilpour et al., 2020). This was recently addressed by an international consortium (The International Network of Transcranial Electrical Stimulation-fMRI, tES-fMRI) by establishing a consensus-based standard for reporting essential details in concurrent tES-fMRI studies (Ekhtiari et al., 2022). The checklist comprises 17 items across three broad categories, namely technological factors (e.g., details of equipment, electrode positioning), safety and noise tests (e.g., reporting of incidents, noise quantification) and methodological factors (e.g., reporting of set-up schematics or the tES-fMRI timing). These critical elements represent suggestions for the minimum required information to ensure reproducibility and to enhance the technical and scientific quality and interpretability of future concurrent fMRI-tDCS studies. Importantly, the checklist is suited to facilitate development of similar guidelines for other imaging modalities.
To date, there is limited knowledge on how tDCS modulates the complex neural networks supporting higher human cognitive functions in health and disease. Combining neuroimaging technology with tDCS has great potential to reveal the neural mechanisms and predictors underlying behavioral modulation and to identify sources of variability in stimulation response. The present manuscript aimed to discuss the underlying causes of variability in tDCS studies, elaborate on design-related considerations for tDCS-fMRI research, optimization of tDCS and imaging protocols and how to assure high-level experimental control at the level of individual experiments and from a meta-perspective. We also addressed variable tDCS effects across the healthy human lifespan, implications for tDCS studies in age-associated disease, and potential benefits of establishing large-scale, multidisciplinary consortia for more coordinated tDCS research in the future.
We hope that this manuscript will contribute to more coordinated, methodologically sound, transparent and reproducible research in this field, thereby fostering a better understanding of the underlying mechanisms by which tDCS modulates human cognitive functions and ultimately more effective and individually tailored translational and clinical applications of this technique in the future. Ultimately, this will yield information if and how tDCS can modulate human brain functions in a meaningful way.
MM: Conceptualization, Writing – original draft, Writing – review & editing, Investigation, Methodology, Supervision, Funding acquisition, Resources. AS: Conceptualization, Investigation, Methodology, Supervision, Writing – original draft, Writing – review & editing, Visualization. DA: Writing – review & editing. FB: Writing – review & editing. RF: Writing – review & editing. GH: Writing – review & editing. MAN: Writing – review & editing. S-CL: Writing – review & editing. AT: Writing – review & editing. DT: Writing – review & editing. MA: Writing – original draft, Writing – review & editing. HK: Writing – review & editing. LC: Writing – review & editing. GB: Writing – review & editing. MG: Writing – review & editing. TC: Writing – review & editing. DH: Writing – review & editing. ST: Writing – review & editing. FS: Writing – review & editing. YS: Writing – review & editing. AK: Writing – review & editing. MB: Writing – review & editing. AF: Writing – review & editing, Funding acquisition, Supervision, Writing – original draft. DW: Writing – review & editing. SR: Writing – review & editing. FN: Writing – review & editing.
The author(s) declare that financial support was received for the research, authorship, and/or publication of this article. This research was funded by the German Research Foundation (project grants: ME 3161/3-1; FL 379/26-1; CRC INST 276/741-2 and 292/155-1, Research Unit 5429/1 (467143400), FL 379/34-1, FL 379/35-1, Fl 379/37-1, Fl 379/22-1, Fl 379/26-1, ME 3161/5-1, ME 3161/6-1, AN 1103/5-1, TH 1330/6-1, TH 1330/7-1, NI 683/17-1, HA 6314/10-1, TI 239/23-1, BL 977/4-1, LI 879/24-1). AT was supported by the Lundbeck foundation (grant R313-2019-622). GH was supported by Lise Meitner Excellence funding from the Max Planck Society, and by the European Research Council (ERC-2021-COG 101043747).
MAN is in the scientific advisory board of Neuroelectrics and Précis’s.
The remaining authors declare that the research was conducted in the absence of any commercial or financial relationships that could be construed as a potential conflict of interest.
The reviewer BL declared a past co-authorship with the authors AF and MAN to the handling editor.
All claims expressed in this article are solely those of the authors and do not necessarily represent those of their affiliated organizations, or those of the publisher, the editors and the reviewers. Any product that may be evaluated in this article, or claim that may be made by its manufacturer, is not guaranteed or endorsed by the publisher.
1. ^https://soterixmedical.com/research/software/hd-explore
2. ^https://www.humanbrainproject.eu/en/
Aberra, A. S., Peterchev, A. V., and Grill, W. M. (2018). Biophysically realistic neuron models for simulation of cortical stimulation. J. Neural Eng. 15:066023. doi: 10.1088/1741-2552/aadbb1
Albizu, A., Fang, R., Indahlastari, A., O’Shea, A., Stolte, S. E., See, K. B., et al. (2020). Machine learning and individual variability in electric field characteristics predict tDCS treatment response. Brain Stimul. 13, 1753–1764. doi: 10.1016/j.brs.2020.10.001
Albizu, A., Indahlastari, A., Huang, Z., Waner, J., Stolte, S. E., Fang, R., et al. (2023). Machine-learning defined precision tDCS for improving cognitive function. Brain Stimul. 16, 969–974. doi: 10.1016/j.brs.2023.05.020
Alexandersen, A., Csifcsák, G., Groot, J., and Mittner, M. (2022). The effect of transcranial direct current stimulation on the interplay between executive control, behavioral variability and mind wandering: a registered report. Neuroimage: Reports 2:100109. doi: 10.1016/j.ynirp.2022.100109
Allman, C., Amadi, U., Winkler, A. M., Wilkins, L., Filippini, N., Kischka, U., et al. (2016). Ipsilesional anodal tDCS enhances the functional benefits of rehabilitation in patients after stroke. Sci. Transl. Med. 8:330re1. doi: 10.1126/scitranslmed.aad5651
Ambrus, G. G., Al-Moyed, H., Chaieb, L., Sarp, L., Antal, A., and Paulus, W. (2012). The fade-in – short stimulation – fade out approach to sham tDCS – reliable at 1 mA for naïve and experienced subjects, but not investigators. Brain Stimul. 5, 499–504. doi: 10.1016/j.brs.2011.12.001
Antal, A., Alekseichuk, I., Bikson, M., Brockmöller, J., Brunoni, A. R., Chen, R., et al. (2017). Low intensity transcranial electric stimulation: safety, ethical, legal regulatory and application guidelines. Clin. Neurophysiol. 128, 1774–1809. doi: 10.1016/j.clinph.2017.06.001
Antal, A., Nitsche, M. A., Kruse, W., Kincses, T. Z., Hoffmann, K.-P., and Paulus, W. (2004). Direct current stimulation over V5 enhances Visuomotor coordination by improving motion perception in humans. J. Cogn. Neurosci. 16, 521–527. doi: 10.1162/089892904323057263
Antal, A., Polania, R., Schmidt-Samoa, C., Dechent, P., and Paulus, W. (2011). Transcranial direct current stimulation over the primary motor cortex during fMRI. NeuroImage 55, 590–596. doi: 10.1016/j.neuroimage.2010.11.085
Antonenko, D., Fromm, A. E., Thams, F., Grittner, U., Meinzer, M., and Flöel, A. (2023). Microstructural and functional plasticity following repeated brain stimulation during cognitive training in older adults. Nat. Commun. 14:3184. doi: 10.1038/s41467-023-38910-x
Antonenko, D., Grittner, U., Saturnino, G., Nierhaus, T., Thielscher, A., and Flöel, A. (2021). Inter-individual and age-dependent variability in simulated electric fields induced by conventional transcranial electrical stimulation. NeuroImage 224:117413. doi: 10.1016/j.neuroimage.2020.117413
Antonenko, D., Hayek, D., Netzband, J., Grittner, U., and Flöel, A. (2019a). tDCS-induced episodic memory enhancement and its association with functional network coupling in older adults. Sci. Rep. 9:2273. doi: 10.1038/s41598-019-38630-7
Antonenko, D., Nierhaus, T., Meinzer, M., Prehn, K., Thielscher, A., Ittermann, B., et al. (2018). Age-dependent effects of brain stimulation on network centrality. NeuroImage 176, 71–82. doi: 10.1016/j.neuroimage.2018.04.038
Antonenko, D., Schubert, F., Bohm, F., Ittermann, B., Aydin, S., Hayek, D., et al. (2017). tDCS-induced modulation of GABA levels and resting-state functional connectivity in older adults. J. Neurosci. 37, 4065–4073. doi: 10.1523/JNEUROSCI.0079-17.2017
Antonenko, D., Thielscher, A., Saturnino, G. B., Aydin, S., Ittermann, B., Grittner, U., et al. (2019b). Towards precise brain stimulation: is electric field simulation related to neuromodulation? Brain Stimul. 12, 1159–1168. doi: 10.1016/j.brs.2019.03.072
Attal, Y., Maess, B., Friederici, A., and David, O. (2012). Head models and dynamic causal modeling of subcortical activity using magnetoencephalographic/electroencephalographic data. Rev. Neurosci. 23, 85–95. doi: 10.1515/rns.2011.056
Axelrod, V., Rees, G., Lavidor, M., and Bar, M. (2015). Increasing propensity to mind-wander with transcranial direct current stimulation. Proc. Natl. Acad. Sci. USA 112, 3314–3319. doi: 10.1073/pnas.1421435112
Bartels, C., Wegrzyn, M., Wiedl, A., Ackermann, V., and Ehrenreich, H. (2010). Practice effects in healthy adults: a longitudinal study on frequent repetitive cognitive testing. BMC Neurosci. 11:118. doi: 10.1186/1471-2202-11-118
Batsikadze, G., Moliadze, V., Paulus, W., Kuo, M. -F., and Nitsche, M. A. (2013). Partially non-linear stimulation intensity-dependent effects of direct current stimulation on motor cortex excitability in humans. J. Physiol. 591, 1987–2000. doi: 10.1113/jphysiol.2012.249730
Bell, L., Lamport, D. J., Field, D. T., Butler, L. T., and Williams, C. M. (2018). Practice effects in nutrition intervention studies with repeated cognitive testing. NHA 4, 309–322. doi: 10.3233/NHA-170038
Berryhill, M. E., and Jones, K. T. (2012). tDCS selectively improves working memory in older adults with more education. Neurosci. Lett. 521, 148–151. doi: 10.1016/j.neulet.2012.05.074
Bikson, M., Name, A., and Rahman, A. (2013). Origins of specificity during tDCS: anatomical, activity-selective, and input-bias mechanisms. Front. Hum. Neurosci. 7:688. doi: 10.3389/fnhum.2013.00688
Bikson, M., Rahman, A., and Datta, A. (2012). Computational models of transcranial direct current stimulation. Clin. EEG Neurosci. 43, 176–183. doi: 10.1177/1550059412445138
Bikson, M., Truong, D. Q., Mourdoukoutas, A. P., Aboseria, M., Khadka, N., Adair, D., et al. (2015). Modeling sequence and quasi-uniform assumption in computational neurostimulation. Prog. Brain Res. 222, 1–23. doi: 10.1016/bs.pbr.2015.08.005
Boayue, N. M., Csifcsák, G., Aslaksen, P., Turi, Z., Antal, A., Groot, J., et al. (2020). Increasing propensity to mind-wander by transcranial direct current stimulation? A registered report. Eur. J. Neurosci. 51, 755–780. doi: 10.1111/ejn.14347
Boggio, P. S., Campanhã, C., Valasek, C. A., Fecteau, S., Pascual-Leone, A., and Fregni, F. (2010). Modulation of decision-making in a gambling task in older adults with transcranial direct current stimulation. Eur. J. Neurosci. 31, 593–597. doi: 10.1111/j.1460-9568.2010.07080.x
Bortoletto, M., Rodella, C., Salvador, R., Miranda, P. C., and Miniussi, C. (2016). Reduced current spread by concentric electrodes in transcranial electrical stimulation (tES). Brain Stimul. 9, 525–528. doi: 10.1016/j.brs.2016.03.001
Botvinik-Nezer, R., Holzmeister, F., Camerer, C. F., Dreber, A., Huber, J., Johannesson, M., et al. (2020). Variability in the analysis of a single neuroimaging dataset by many teams. Nature 582, 84–88. doi: 10.1038/s41586-020-2314-9
Breakspear, M. (2017). Dynamic models of large-scale brain activity. Nat. Neurosci. 20, 340–352. doi: 10.1038/nn.4497
Cabral-Calderin, Y., Anne Weinrich, C., Schmidt-Samoa, C., Poland, E., Dechent, P., Bähr, M., et al. (2016). Transcranial alternating current stimulation affects the BOLD signal in a frequency and task-dependent manner. Hum. Brain Mapp. 37, 94–121. doi: 10.1002/hbm.23016
Calzolari, S., Jalali, R., and Fernández-Espejo, D. (2023). Characterising stationary and dynamic effective connectivity changes in the motor network during and after tDCS. NeuroImage 269:119915. doi: 10.1016/j.neuroimage.2023.119915
Cao, J., and Liu, H. (2018). Modulating the resting-state functional connectivity patterns of language processing areas in the human brain with anodal transcranial direct current stimulation applied over the Broca’s area. Neurophoton 5:1. doi: 10.1117/1.NPh.5.2.025002
Caulfield, K. A., Badran, B. W., Li, X., Bikson, M., and George, M. S. (2020). Can transcranial electrical stimulation motor threshold estimate individualized tDCS doses over the prefrontal cortex? Evidence from reverse-calculation electric field modeling. Brain Stimul. 13, 1150–1152. doi: 10.1016/j.brs.2020.05.012
Cespón, J., Rodella, C., Rossini, P. M., Miniussi, C., and Pellicciari, M. C. (2017). Anodal transcranial direct current stimulation promotes frontal compensatory mechanisms in healthy elderly subjects. Front. Aging Neurosci. 9:420. doi: 10.3389/fnagi.2017.00420
Chambers, C. D., and Tzavella, L. (2021). The past, present and future of registered reports. Nat. Hum. Behav. 6, 29–42. doi: 10.1038/s41562-021-01193-7
Cirillo, G., Di Pino, G., Capone, F., Ranieri, F., Florio, L., Todisco, V., et al. (2017). Neurobiological after-effects of non-invasive brain stimulation. Brain Stimul. 10, 1–18. doi: 10.1016/j.brs.2016.11.009
Crosson, B., McGregor, K. M., Nocera, J. R., Drucker, J. H., Tran, S. M., and Butler, A. J. (2015). The relevance of aging-related changes in brain function to rehabilitation in aging-related disease. Front. Hum. Neurosci. 9:307. doi: 10.3389/fnhum.2015.00307
Dahnke, R., Yotter, R. A., and Gaser, C. (2013). Cortical thickness and central surface estimation. NeuroImage 65, 336–348. doi: 10.1016/j.neuroimage.2012.09.050
Darkow, R., and Flöel, A. (2016). Aphasie: evidenzbasierte Therapieansätze. Nervenarzt 87, 1051–1056. doi: 10.1007/s00115-016-0213-y
Darkow, R., Martin, A., Würtz, A., Flöel, A., and Meinzer, M. (2017). Transcranial direct current stimulation effects on neural processing in post-stroke aphasia. Hum. Brain Mapp. 38, 1518–1531. doi: 10.1002/hbm.23469
Datta, A., Bansal, V., Diaz, J., Patel, J., Reato, D., and Bikson, M. (2009). Gyri-precise head model of transcranial direct current stimulation: improved spatial focality using a ring electrode versus conventional rectangular pad. Brain Stimul. 2, 201–207.e1. doi: 10.1016/j.brs.2009.03.005
De Munck, J. C., Van Houdt, P. J., Gonçalves, S. I., Van Wegen, E., and Ossenblok, P. P. W. (2013). Novel artefact removal algorithms for co-registered EEG/fMRI based on selective averaging and subtraction. NeuroImage 64, 407–415. doi: 10.1016/j.neuroimage.2012.09.022
De Witte, S., Klooster, D., Dedoncker, J., Duprat, R., Remue, J., and Baeken, C. (2018). Left prefrontal neuronavigated electrode localization in tDCS: 10–20 EEG system versus MRI-guided neuronavigation. Psychiatry Res. Neuroimaging 274, 1–6. doi: 10.1016/j.pscychresns.2018.02.001
Dissanayaka, T. D., Zoghi, M., Farrell, M., Egan, G. F., and Jaberzadeh, S. (2018). Sham transcranial electrical stimulation and its effects on corticospinal excitability: a systematic review and meta-analysis. Rev. Neurosci. 29, 223–232. doi: 10.1515/revneuro-2017-0026
Dubljević, V., Saigle, V., and Racine, E. (2014). The rising tide of tDCS in the media and academic literature. Neuron 82, 731–736. doi: 10.1016/j.neuron.2014.05.003
Dutta, A. (2021). Simultaneous functional near-infrared spectroscopy (fNIRS) and electroencephalogram (EEG) to elucidate neurovascular modulation by transcranial electrical stimulation (tES). Brain Stimul. 14, 1093–1094. doi: 10.1016/j.brs.2021.07.019
Edwards, D., Cortes, M., Datta, A., Minhas, P., Wassermann, E. M., and Bikson, M. (2013). Physiological and modeling evidence for focal transcranial electrical brain stimulation in humans: a basis for high-definition tDCS. NeuroImage 74, 266–275. doi: 10.1016/j.neuroimage.2013.01.042
Ekhtiari, H., Ghobadi-Azbari, P., Thielscher, A., Antal, A., Li, L. M., Shereen, A. D., et al. (2022). A checklist for assessing the methodological quality of concurrent tES-fMRI studies (ContES checklist): a consensus study and statement. Nat. Protoc. 17, 596–617. doi: 10.1038/s41596-021-00664-5
Esmaeilpour, Z., Shereen, A. D., Ghobadi-Azbari, P., Datta, A., Woods, A. J., Ironside, M., et al. (2020). Methodology for tDCS integration with fMRI. Hum. Brain Mapp. 41, 1950–1967. doi: 10.1002/hbm.24908
Evans, C., Bachmann, C., Lee, J. S. A., Gregoriou, E., Ward, N., and Bestmann, S. (2020). Dose-controlled tDCS reduces electric field intensity variability at a cortical target site. Brain Stimul. 13, 125–136. doi: 10.1016/j.brs.2019.10.004
Falleti, M. G., Maruff, P., Collie, A., and Darby, D. G. (2006). Practice effects associated with the repeated assessment of cognitive function using the CogState battery at 10-minute, one week and one month test-retest intervals. J. Clin. Exp. Neuropsychol. 28, 1095–1112. doi: 10.1080/13803390500205718
Fertonani, A., Brambilla, M., Cotelli, M., and Miniussi, C. (2014). The timing of cognitive plasticity in physiological aging: a tDCS study of naming. Front. Aging Neurosci. 6:131. doi: 10.3389/fnagi.2014.00131
Fertonani, A., and Miniussi, C. (2017). Transcranial electrical stimulation: what we know and do not know about mechanisms. Neuroscientist 23, 109–123. doi: 10.1177/1073858416631966
Figeys, M., Zeeman, M., and Kim, E. S. (2021). Effects of transcranial direct current stimulation (tDCS) on cognitive performance and cerebral oxygen hemodynamics: a systematic review. Front. Hum. Neurosci. 15:623315. doi: 10.3389/fnhum.2021.623315
Filmer, H. L., Ehrhardt, S. E., Shaw, T. B., Mattingley, J. B., and Dux, P. E. (2019). The efficacy of transcranial direct current stimulation to prefrontal areas is related to underlying cortical morphology. NeuroImage 196, 41–48. doi: 10.1016/j.neuroimage.2019.04.026
Fleury, M., Barillot, C., Mano, M., Bannier, E., and Maurel, P. (2019). Automated electrodes detection during simultaneous EEG/fMRI. Front. ICT 5:31. doi: 10.3389/fict.2018.00031
Fridriksson, J., Basilakos, A., Stark, B. C., Rorden, C., Elm, J., Gottfried, M., et al. (2019). Transcranial direct current stimulation to treat aphasia: longitudinal analysis of a randomized controlled trial. Brain Stimul. 12, 190–191. doi: 10.1016/j.brs.2018.09.016
Galli, G., Vadillo, M. A., Sirota, M., Feurra, M., and Medvedeva, A. (2019). A systematic review and meta-analysis of the effects of transcranial direct current stimulation (tDCS) on episodic memory. Brain Stimul. 12, 231–241. doi: 10.1016/j.brs.2018.11.008
Gandiga, P. C., Hummel, F. C., and Cohen, L. G. (2006). Transcranial DC stimulation (tDCS): a tool for double-blind sham-controlled clinical studies in brain stimulation. Clin. Neurophysiol. 117, 845–850. doi: 10.1016/j.clinph.2005.12.003
Gauvin, H. S., Meinzer, M., and De Zubicaray, G. I. (2017). tDCS effects on word production: limited by design? Comment on Westwood et al. (2017). Cortex 96, 137–142. doi: 10.1016/j.cortex.2017.06.017
Gbadeyan, O., McMahon, K., Steinhauser, M., and Meinzer, M. (2016a). Stimulation of dorsolateral prefrontal cortex enhances adaptive cognitive control: a high-definition transcranial direct current stimulation study. J. Neurosci. 36, 12530–12536. doi: 10.1523/JNEUROSCI.2450-16.2016
Gbadeyan, O., Steinhauser, M., McMahon, K., and Meinzer, M. (2016b). Safety, tolerability, blinding efficacy and Behavioural effects of a novel MRI-compatible, high-definition tDCS set-up. Brain Stimul. 9, 545–552. doi: 10.1016/j.brs.2016.03.018
Ghasemian-Shirvan, E., Mosayebi-Samani, M., Farnad, L., Kuo, M.-F., Meesen, R. L. J., and Nitsche, M. A. (2022). Age-dependent non-linear neuroplastic effects of cathodal tDCS in the elderly population: a titration study. Brain Stimul. 15, 296–305. doi: 10.1016/j.brs.2022.01.011
Göksu, C., Hanson, L. G., Siebner, H. R., Ehses, P., Scheffler, K., and Thielscher, A. (2018). Human in-vivo brain magnetic resonance current density imaging (MRCDI). NeuroImage 171, 26–39. doi: 10.1016/j.neuroimage.2017.12.075
Göksu, C., Scheffler, K., Siebner, H. R., Thielscher, A., and Hanson, L. G. (2019). The stray magnetic fields in magnetic resonance current density imaging (MRCDI). Phys. Med. 59, 142–150. doi: 10.1016/j.ejmp.2019.02.022
Gorgolewski, K. J., and Poldrack, R. A. (2016). A practical guide for improving transparency and reproducibility in neuroimaging research. PLoS Biol. 14:e1002506. doi: 10.1371/journal.pbio.1002506
Greinacher, R., Buhôt, L., Möller, L., and Learmonth, G. (2019). The time course of ineffective sham-blinding during low-intensity (1 mA) transcranial direct current stimulation. Eur. J. Neurosci. 50, 3380–3388. doi: 10.1111/ejn.14497
Grimes, D. A., and Schulz, K. F. (2002). An overview of clinical research: the lay of the land. Lancet 359, 57–61. doi: 10.1016/S0140-6736(02)07283-5
Gutchess, A. (2014). Plasticity of the aging brain: new directions in cognitive neuroscience. Science 346, 579–582. doi: 10.1126/science.1254604
Hanley, C. J., and Tales, A. (2019). Anodal tDCS improves attentional control in older adults. Exp. Gerontol. 115, 88–95. doi: 10.1016/j.exger.2018.11.019
Hausknecht, J. P., Halpert, J. A., Di Paolo, N. T., and Moriarty Gerrard, M. O. (2007). Retesting in selection: a meta-analysis of coaching and practice effects for tests of cognitive ability. J. Appl. Psychol. 92, 373–385. doi: 10.1037/0021-9010.92.2.373
Hedden, T., and Gabrieli, J. D. E. (2004). Insights into the ageing mind: a view from cognitive neuroscience. Nat. Rev. Neurosci. 5, 87–96. doi: 10.1038/nrn1323
Herwig, U., Satrapi, P., and Schönfeldt-Lecuona, C. (2003). Using the international 10-20 EEG system for positioning of transcranial magnetic stimulation. Brain Topogr. 16, 95–99. doi: 10.1023/B:BRAT.0000006333.93597.9d
Hiew, S., Nguemeni, C., and Zeller, D. (2022). Efficacy of transcranial direct current stimulation in people with multiple sclerosis: a review. Eur. J. Neurol. 29, 648–664. doi: 10.1111/ene.15163
Holland, R., Leff, A. P., Josephs, O., Galea, J. M., Desikan, M., Price, C. J., et al. (2011). Speech facilitation by left inferior frontal cortex stimulation. Curr. Biol. 21, 1403–1407. doi: 10.1016/j.cub.2011.07.021
Holman, L., Head, M. L., Lanfear, R., and Jennions, M. D. (2015). Evidence of experimental Bias in the life sciences: why we need blind data recording. PLoS Biol. 13:e1002190. doi: 10.1371/journal.pbio.1002190
Hordacre, B., Moezzi, B., Goldsworthy, M. R., Rogasch, N. C., Graetz, L. J., and Ridding, M. C. (2017). Resting state functional connectivity measures correlate with the response to anodal transcranial direct current stimulation. Eur. J. Neurosci. 45, 837–845. doi: 10.1111/ejn.13508
Huang, A. Y., Yu, D., Davis, L. K., Sul, J. H., Tsetsos, F., Ramensky, V., et al. (2017). Rare copy number variants in NRXN1 and CNTN6 increase risk for Tourette syndrome. Neuron 94, 1101–1111.e7. doi: 10.1016/j.neuron.2017.06.010
Huey, E. D., Probasco, J. C., Moll, J., Stocking, J., Ko, M.-H., Grafman, J., et al. (2007). No effect of DC brain polarization on verbal fluency in patients with advanced frontotemporal dementia. Clin. Neurophysiol. 118, 1417–1418. doi: 10.1016/j.clinph.2007.02.026
Hunold, A., Haueisen, J., Nees, F., and Moliadze, V. (2023). Review of individualized current flow modeling studies for transcranial electrical stimulation. J. Neurosci. Res. 101, 405–423. doi: 10.1002/jnr.25154
Indahlastari, A., Albizu, A., O’Shea, A., Forbes, M. A., Nissim, N. R., Kraft, J. N., et al. (2020). Modeling transcranial electrical stimulation in the aging brain. Brain Stimul. 13, 664–674. doi: 10.1016/j.brs.2020.02.007
Indahlastari, A., Dunn, A. L., Pedersen, S., Kraft, J. N., Someya, S., Albizu, A., et al. (2023). The importance of accurately representing electrode position in transcranial direct current stimulation computational models. Brain Stimul. 16, 930–932. doi: 10.1016/j.brs.2023.05.010
Jaberzadeh, S., Martin, D., Knotkova, H., and Woods, A. J. (2019). “Methodological considerations for selection of transcranial direct current stimulation approach, protocols and devices” in Practical guide to transcranial direct current stimulation. eds. H. Knotkova, M. A. Nitsche, M. Bikson, and A. J. Woods (Cham: Springer International Publishing), 199–223.
Jacobson, L., Koslowsky, M., and Lavidor, M. (2012). tDCS polarity effects in motor and cognitive domains: a meta-analytical review. Exp. Brain Res. 216, 1–10. doi: 10.1007/s00221-011-2891-9
Jamil, A., Batsikadze, G., Kuo, H., Meesen, R. L. J., Dechent, P., Paulus, W., et al. (2020). Current intensity- and polarity-specific online and aftereffects of transcranial direct current stimulation: an fMRI study. Hum. Brain Mapp. 41, 1644–1666. doi: 10.1002/hbm.24901
Jang, S., Kim, D., Cho, H., Kwon, M., and Jun, S. C. (2017). Assessing stimulation effects induced by tDCS using MEG. Brain Stimul. 10:526. doi: 10.1016/j.brs.2017.01.536
Keeser, D., Meindl, T., Bor, J., Palm, U., Pogarell, O., Mulert, C., et al. (2011). Prefrontal transcranial direct current stimulation changes connectivity of resting-state networks during fMRI. J. Neurosci. 31, 15284–15293. doi: 10.1523/JNEUROSCI.0542-11.2011
Kim, J.-H., Kim, D.-W., Chang, W. H., Kim, Y.-H., Kim, K., and Im, C.-H. (2014). Inconsistent outcomes of transcranial direct current stimulation may originate from anatomical differences among individuals: electric field simulation using individual MRI data. Neurosci. Lett. 564, 6–10. doi: 10.1016/j.neulet.2014.01.054
Knotkova, H., Riggs, A., Berisha, D., Borges, H., Bernstein, H., Patel, V., et al. (2019). Automatic M1-SO montage headgear for transcranial direct current stimulation (TDCS) suitable for home and high-throughput in-clinic applications. Neuromodulation 22, 904–910. doi: 10.1111/ner.12786
Kuo, H.-I., Bikson, M., Datta, A., Minhas, P., Paulus, W., Kuo, M.-F., et al. (2013). Comparing cortical plasticity induced by conventional and high-definition 4 × 1 ring tDCS: a neurophysiological study. Brain Stimul. 6, 644–648. doi: 10.1016/j.brs.2012.09.010
Kuo, M.-F., Paulus, W., and Nitsche, M. A. (2006). Sex differences in cortical neuroplasticity in humans. Neuroreport 17, 1703–1707. doi: 10.1097/01.wnr.0000239955.68319.c2
Kurtin, D. L., Violante, I. R., Zimmerman, K., Leech, R., Hampshire, A., Patel, M. C., et al. (2021). Investigating the interaction between white matter and brain state on tDCS-induced changes in brain network activity. Brain Stimul. 14, 1261–1270. doi: 10.1016/j.brs.2021.08.004
Lavezzi, G. D., Sanz Galan, S., Andersen, H., Tomer, D., and Cacciamani, L. (2022). The effects of tDCS on object perception: a systematic review and meta-analysis. Behav. Brain Res. 430:113927. doi: 10.1016/j.bbr.2022.113927
Lee, J.-H., Jeun, Y.-J., Park, H. Y., and Jung, Y.-J. (2021). Effect of transcranial direct current stimulation combined with rehabilitation on arm and hand function in stroke patients: a systematic review and Meta-analysis. Healthcare 9:1705. doi: 10.3390/healthcare9121705
Li, R., Hosseini, H., Saggar, M., Balters, S. C., and Reiss, A. L. (2023). Current opinions on the present and future use of functional near-infrared spectroscopy in psychiatry. Neurophoton 10:013505. doi: 10.1117/1.NPh.10.1.013505
Lioumis, P., and Rosanova, M. (2022). The role of neuronavigation in TMS–EEG studies: current applications and future perspectives. J. Neurosci. Methods 380:109677. doi: 10.1016/j.jneumeth.2022.109677
Majdi, A., Van Boekholdt, L., Sadigh-Eteghad, S., and Mc Laughlin, M. (2022). A systematic review and meta-analysis of transcranial direct-current stimulation effects on cognitive function in patients with Alzheimer’s disease. Mol. Psychiatry 27, 2000–2009. doi: 10.1038/s41380-022-01444-7
Manenti, R., Brambilla, M., Petesi, M., Ferrari, C., and Cotelli, M. (2013). Enhancing verbal episodic memory in older and young subjects after non-invasive brain stimulation. Front. Aging Neurosci. 5:49. doi: 10.3389/fnagi.2013.00049
Martin, A. K., Dzafic, I., Ramdave, S., and Meinzer, M. (2017a). Causal evidence for task-specific involvement of the dorsomedial prefrontal cortex in human social cognition. Soc. Cogn. Affect. Neurosci. 12, 1209–1218. doi: 10.1093/scan/nsx063
Martin, A. K., Huang, J., Hunold, A., and Meinzer, M. (2017b). Sex mediates the effects of high-definition transcranial direct current stimulation on “mind-Reading”. Neuroscience 366, 84–94. doi: 10.1016/j.neuroscience.2017.10.005
Martin, A. K., Huang, J., Hunold, A., and Meinzer, M. (2019a). Dissociable roles within the social brain for self–other processing: a HD-tDCS study. Cereb. Cortex 29, 3642–3654. doi: 10.1093/cercor/bhy238
Martin, A. K., Su, P., and Meinzer, M. (2019b). Common and unique effects of HD-tDCS to the social brain across cultural groups. Neuropsychologia 133:107170. doi: 10.1016/j.neuropsychologia.2019.107170
Matsushita, R., Puschmann, S., Baillet, S., and Zatorre, R. J. (2021). Inhibitory effect of tDCS on auditory evoked response: simultaneous MEG-tDCS reveals causal role of right auditory cortex in pitch learning. NeuroImage 233:117915. doi: 10.1016/j.neuroimage.2021.117915
McKendrick, R., Parasuraman, R., and Ayaz, H. (2015). Wearable functional near infrared spectroscopy (fNIRS) and transcranial direct current stimulation (tDCS): expanding vistas for neurocognitive augmentation. Front. Syst. Neurosci. 9:27. doi: 10.3389/fnsys.2015.00027
Meinzer, M., Lindenberg, R., Antonenko, D., Flaisch, T., and Floel, A. (2013). Anodal transcranial direct current stimulation temporarily reverses age-associated cognitive decline and functional brain activity changes. J. Neurosci. 33, 12470–12478. doi: 10.1523/JNEUROSCI.5743-12.2013
Minarik, T., Berger, B., Althaus, L., Bader, V., Biebl, B., Brotzeller, F., et al. (2016). The importance of sample size for reproducibility of tDCS effects. Front. Hum. Neurosci. 10:453. doi: 10.3389/fnhum.2016.00453
Mosayebi Samani, M., Agboada, D., Jamil, A., Kuo, M.-F., and Nitsche, M. A. (2019). Titrating the neuroplastic effects of cathodal transcranial direct current stimulation (tDCS) over the primary motor cortex. Cortex 119, 350–361. doi: 10.1016/j.cortex.2019.04.016
Munro, K. J., and Prendergast, G. (2019). Encouraging pre-registration of research studies. Int. J. Audiol. 58, 123–124. doi: 10.1080/14992027.2019.1574405
Neri, F., Mencarelli, L., Menardi, A., Giovannelli, F., Rossi, S., Sprugnoli, G., et al. (2020). A novel tDCS sham approach based on model-driven controlled shunting. Brain Stimul. 13, 507–516. doi: 10.1016/j.brs.2019.11.004
Nichols, T. E., Das, S., Eickhoff, S. B., Evans, A. C., Glatard, T., Hanke, M., et al. (2017). Best practices in data analysis and sharing in neuroimaging using MRI. Nat. Neurosci. 20, 299–303. doi: 10.1038/nn.4500
Niemann, F., Riemann, S., Hubert, A.-K., Antonenko, D., Thielscher, A., Martin, A. K., et al. (2024). Electrode positioning errors reduce current dose for focal tDCS set-ups: evidence from individualized electric field mapping. Clin. Neurophysiol. 162, 201–209. doi: 10.1016/j.clinph.2024.03.031
Nitsche, M. A., and Paulus, W. (2000). Excitability changes induced in the human motor cortex by weak transcranial direct current stimulation. J. Physiol. 527, 633–639. doi: 10.1111/j.1469-7793.2000.t01-1-00633.x
Noble, S., Scheinost, D., and Constable, R. T. (2021). A guide to the measurement and interpretation of fMRI test-retest reliability. Curr. Opin. Behav. Sci. 40, 27–32. doi: 10.1016/j.cobeha.2020.12.012
O’Connell, N. E., Cossar, J., Marston, L., Wand, B. M., Bunce, D., Moseley, G. L., et al. (2012). Rethinking clinical trials of transcranial direct current stimulation: participant and Assessor blinding is inadequate at intensities of 2mA. PLoS One 7:e47514. doi: 10.1371/journal.pone.0047514
Okazaki, Y., Izumiya, M., Hagihara, M., and Kitajo, K. (2023). An HD-tDCS–EEG study to reveal current polarity-dependent effects on attentional fluctuations. Brain Stimul. 16:381. doi: 10.1016/j.brs.2023.01.759
Oldrati, V., Colombo, B., and Antonietti, A. (2018). Combination of a short cognitive training and tDCS to enhance visuospatial skills: a comparison between online and offline neuromodulation. Brain Res. 1678, 32–39. doi: 10.1016/j.brainres.2017.10.002
Open Science Collaboration (2015). Estimating the reproducibility of psychological science. Science 349:aac4716. doi: 10.1126/science.aac4716
Opitz, A., Paulus, W., Will, S., Antunes, A., and Thielscher, A. (2015). Determinants of the electric field during transcranial direct current stimulation. NeuroImage 109, 140–150. doi: 10.1016/j.neuroimage.2015.01.033
Pang, J. C., Aquino, K. M., Oldehinkel, M., Robinson, P. A., Fulcher, B. D., Breakspear, M., et al. (2023). Geometric constraints on human brain function. Nature 618, 566–574. doi: 10.1038/s41586-023-06098-1
Perceval, G., Flöel, A., and Meinzer, M. (2016). Can transcranial direct current stimulation counteract age-associated functional impairment? Neurosci. Biobehav. Rev. 65, 157–172. doi: 10.1016/j.neubiorev.2016.03.028
Perceval, G., Martin, A. K., Copland, D. A., Laine, M., and Meinzer, M. (2020). Multisession transcranial direct current stimulation facilitates verbal learning and memory consolidation in young and older adults. Brain Lang. 205:104788. doi: 10.1016/j.bandl.2020.104788
Polanía, R., Nitsche, M. A., and Paulus, W. (2011). Modulating functional connectivity patterns and topological functional organization of the human brain with transcranial direct current stimulation. Hum. Brain Mapp. 32, 1236–1249. doi: 10.1002/hbm.21104
Postman-Caucheteux, W. A., Birn, R. M., Pursley, R. H., Butman, J. A., Solomon, J. M., Picchioni, D., et al. (2010). Single-trial fMRI shows Contralesional activity linked to overt naming errors in chronic aphasic patients. J. Cogn. Neurosci. 22, 1299–1318. doi: 10.1162/jocn.2009.21261
Priori, A., Berardelli, A., Rona, S., Accornero, N., and Manfredi, M. (1998). Polarization of the human motor cortex through the scalp. Neuroreport 9, 2257–2260. doi: 10.1097/00001756-199807130-00020
Rasmussen, I. D., Boayue, N. M., Mittner, M., Bystad, M., Grønli, O. K., Vangberg, T. R., et al. (2021). High-definition transcranial direct current stimulation improves delayed memory in Alzheimer’s disease patients: a pilot study using computational modeling to optimize electrode position. JAD 83, 753–769. doi: 10.3233/JAD-210378
Reinhart, R. M. G., Cosman, J. D., Fukuda, K., and Woodman, G. F. (2017). Using transcranial direct-current stimulation (tDCS) to understand cognitive processing. Atten. Percept. Psychophys. 79, 3–23. doi: 10.3758/s13414-016-1224-2
Reis, J., Fischer, J. T., Prichard, G., Weiller, C., Cohen, L. G., and Fritsch, B. (2015). Time- but not sleep-dependent consolidation of tDCS-enhanced Visuomotor skills. Cereb. Cortex 25, 109–117. doi: 10.1093/cercor/bht208
Riggall, K., Forlini, C., Carter, A., Hall, W., Weier, M., Partridge, B., et al. (2015). Researchers’ perspectives on scientific and ethical issues with transcranial direct current stimulation: an international survey. Sci. Rep. 5:10618. doi: 10.1038/srep10618
Ross, L. A., McCoy, D., Coslett, H. B., Olson, I. R., and Wolk, D. A. (2011). Improved proper Name recall in aging after electrical stimulation of the anterior temporal lobes. Front. Aging Neurosci. 3:16. doi: 10.3389/fnagi.2011.00016
Rossi, S., Santarnecchi, E., and Feurra, M. (2022). “Noninvasive brain stimulation and brain oscillations” in Handbook of Clinical Neurology (United States: Elsevier), 239–247.
Saiote, C., Turi, Z., Paulus, W., and Antal, A. (2013). Combining functional magnetic resonance imaging with transcranial electrical stimulation. Front. Hum. Neurosci. 7:435. doi: 10.3389/fnhum.2013.00435
Sandrini, M., Umiltà, C., and Rusconi, E. (2011). The use of transcranial magnetic stimulation in cognitive neuroscience: a new synthesis of methodological issues. Neurosci. Biobehav. Rev. 35, 516–536. doi: 10.1016/j.neubiorev.2010.06.005
Saturnino, G. B., Antunes, A., and Thielscher, A. (2015). On the importance of electrode parameters for shaping electric field patterns generated by tDCS. NeuroImage 120, 25–35. doi: 10.1016/j.neuroimage.2015.06.067
Saturnino, G. B., Madsen, K. H., and Thielscher, A. (2021). Optimizing the electric field strength in multiple targets for multichannel transcranial electric stimulation. J. Neural Eng. 18:014001. doi: 10.1088/1741-2552/abca15
Sergiou, C. S., Santarnecchi, E., Franken, I. H. A., and Van Dongen, J. D. M. (2020). The effectiveness of transcranial direct current stimulation as an intervention to improve empathic abilities and reduce violent behavior: a literature review. Aggress. Violent Behav. 55:101463. doi: 10.1016/j.avb.2020.101463
Shahbabaie, A., Ebrahimpoor, M., Hariri, A., Nitsche, M. A., Hatami, J., Fatemizadeh, E., et al. (2018). Transcranial DC stimulation modifies functional connectivity of large-scale brain networks in abstinent methamphetamine users. Brain Behav. 8:e00922. doi: 10.1002/brb3.922
Sliwinska, M. W., Violante, I. R., Wise, R. J. S., Leech, R., Devlin, J. T., Geranmayeh, F., et al. (2017). Stimulating multiple-demand cortex enhances vocabulary learning. J. Neurosci. 37, 7606–7618. doi: 10.1523/JNEUROSCI.3857-16.2017
Stagg, C. J., Antal, A., and Nitsche, M. A. (2018). Physiology of transcranial direct current stimulation. J. ECT 34, 144–152. doi: 10.1097/YCT.0000000000000510
Suh, H. S., Lee, W. H., and Kim, T.-S. (2012). Influence of anisotropic conductivity in the skull and white matter on transcranial direct current stimulation via an anatomically realistic finite element head model. Phys. Med. Biol. 57, 6961–6980. doi: 10.1088/0031-9155/57/21/6961
Sun, W., Wang, H., Zhang, J., Yan, T., and Pei, G. (2021). Multi-layer skull modeling and importance for tDCS simulation. In Proceedings of the 2021 International Conference on Bioinformatics and Intelligent Computing, (Harbin: ACM), 250–256.
Tagoe, N., Molyneux, S., Pulford, J., Murunga, V. I., and Kinyanjui, S. (2019). Managing health research capacity strengthening consortia: a systematised review of the published literature. BMJ Glob. Health 4:e001318. doi: 10.1136/bmjgh-2018-001318
Thair, H., Holloway, A. L., Newport, R., and Smith, A. D. (2017). Transcranial direct current stimulation (tDCS): a Beginner’s guide for design and implementation. Front. Neurosci. 11:641. doi: 10.3389/fnins.2017.00641
Tsuzuki, D., Watanabe, H., Dan, I., and Taga, G. (2016). MinR 10/20 system: quantitative and reproducible cranial landmark setting method for MRI based on minimum initial reference points. J. Neurosci. Methods 264, 86–93. doi: 10.1016/j.jneumeth.2016.02.024
Turi, Z., Csifcsák, G., Boayue, N. M., Aslaksen, P., Antal, A., Paulus, W., et al. (2019). Blinding is compromised for transcranial direct current stimulation at 1 mA for 20 min in young healthy adults. Eur. J. Neurosci. 50, 3261–3268. doi: 10.1111/ejn.14403
Turner, C., Jackson, C., and Learmonth, G. (2021). Is the “end-of-study guess” a valid measure of sham blinding during transcranial direct current stimulation? Eur. J. Neurosci. 53, 1592–1604. doi: 10.1111/ejn.15018
Villamar, M. F., Volz, M. S., Bikson, M., Datta, A., DaSilva, A. F., and Fregni, F. (2013). Technique and considerations in the use of 4x1 ring high-definition transcranial direct current stimulation (HD-tDCS). JoVE 50309:e50309. doi: 10.3791/50309
Wallace, D., Cooper, N. R., Paulmann, S., Fitzgerald, P. B., and Russo, R. (2016). Perceived comfort and blinding efficacy in randomised sham-controlled transcranial direct current stimulation (tDCS) trials at 2 mA in young and older healthy adults. PLoS One 11:e0149703. doi: 10.1371/journal.pone.0149703
Wilkinson, M. D., Dumontier, M., Aalbersberg, I. J., Appleton, G., Axton, M., Baak, A., et al. (2016). The FAIR guiding principles for scientific data management and stewardship. Sci. Data 3:160018. doi: 10.1038/sdata.2016.18
Willmot, N., Leow, L.-A., Filmer, H. L., and Dux, P. E. (2024). Exploring the intra-individual reliability of tDCS: a registered report. Cortex 173, 61–79. doi: 10.1016/j.cortex.2023.12.015
Woods, A. J., Antal, A., Bikson, M., Boggio, P. S., Brunoni, A. R., Celnik, P., et al. (2016). A technical guide to tDCS, and related non-invasive brain stimulation tools. Clin. Neurophysiol. 127, 1031–1048. doi: 10.1016/j.clinph.2015.11.012
Woods, A. J., Bryant, V., Sacchetti, D., Gervits, F., and Hamilton, R. (2015). Effects of electrode drift in transcranial direct current stimulation. Brain Stimul. 8, 515–519. doi: 10.1016/j.brs.2014.12.007
Yan, R., Zhang, X., Li, Y., Hou, J., Chen, H., and Liu, H. (2020). Effect of transcranial direct-current stimulation on cognitive function in stroke patients: a systematic review and meta-analysis. PLoS One 15:e0233903. doi: 10.1371/journal.pone.0233903
Keywords: tES, tDCS-fMRI, cognition, variability, experimental control, lifespan, design optimization, consortia
Citation: Meinzer M, Shahbabaie A, Antonenko D, Blankenburg F, Fischer R, Hartwigsen G, Nitsche MA, Li S-C, Thielscher A, Timmann D, Waltemath D, Abdelmotaleb M, Kocataş H, Caisachana Guevara LM, Batsikadze G, Grundei M, Cunha T, Hayek D, Turker S, Schlitt F, Shi Y, Khan A, Burke M, Riemann S, Niemann F and Flöel A (2024) Investigating the neural mechanisms of transcranial direct current stimulation effects on human cognition: current issues and potential solutions. Front. Neurosci. 18:1389651. doi: 10.3389/fnins.2024.1389651
Received: 21 February 2024; Accepted: 15 May 2024;
Published: 18 June 2024.
Edited by:
Chang-Hoon Choi, Helmholtz Association of German Research Centres (HZ), GermanyReviewed by:
Satoshi Tanaka, Hamamatsu University School of Medicine, JapanCopyright © 2024 Meinzer, Shahbabaie, Antonenko, Blankenburg, Fischer, Hartwigsen, Nitsche, Li, Thielscher, Timmann, Waltemath, Abdelmotaleb, Kocataş, Caisachana Guevara, Batsikadze, Grundei, Cunha, Hayek, Turker, Schlitt, Shi, Khan, Burke, Riemann, Niemann and Flöel. This is an open-access article distributed under the terms of the Creative Commons Attribution License (CC BY). The use, distribution or reproduction in other forums is permitted, provided the original author(s) and the copyright owner(s) are credited and that the original publication in this journal is cited, in accordance with accepted academic practice. No use, distribution or reproduction is permitted which does not comply with these terms.
*Correspondence: Marcus Meinzer, bWFyY3VzLm1laW56ZXJAbWVkLnVuaS1ncmVpZnN3YWxkLmRl; Alireza Shahbabaie, QWxpcmV6YS5TaGFoYmFiYWllQG1lZC51bmktZ3JlaWZzd2FsZC5kZQ==
†These authors have contributed equally to this work
Disclaimer: All claims expressed in this article are solely those of the authors and do not necessarily represent those of their affiliated organizations, or those of the publisher, the editors and the reviewers. Any product that may be evaluated in this article or claim that may be made by its manufacturer is not guaranteed or endorsed by the publisher.
Research integrity at Frontiers
Learn more about the work of our research integrity team to safeguard the quality of each article we publish.