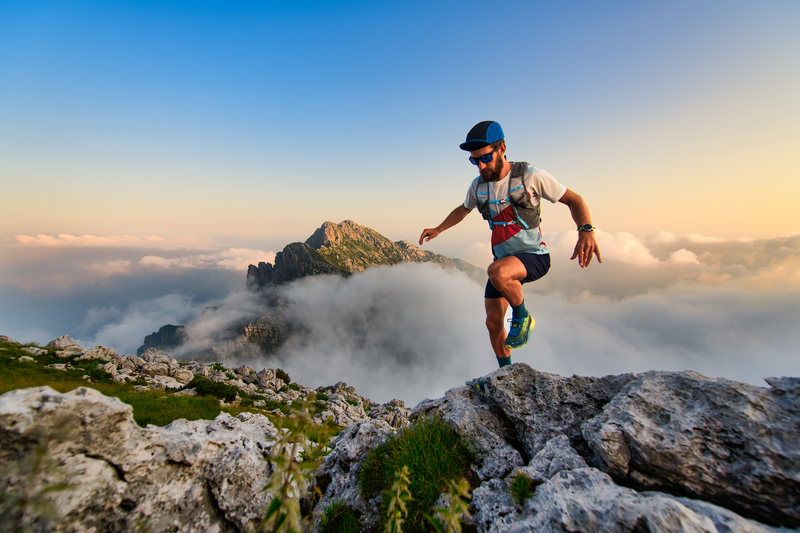
94% of researchers rate our articles as excellent or good
Learn more about the work of our research integrity team to safeguard the quality of each article we publish.
Find out more
ORIGINAL RESEARCH article
Front. Neurosci. , 09 February 2024
Sec. Neuroprosthetics
Volume 18 - 2024 | https://doi.org/10.3389/fnins.2024.1293120
This article is part of the Research Topic Trends in Digital Hearing Health and Computational Audiology View all 12 articles
Introduction: Underlying mechanisms of speech perception masked by background speakers, a common daily listening condition, are often investigated using various and lengthy psychophysical tests. The presence of a social agent, such as an interactive humanoid NAO robot, may help maintain engagement and attention. However, such robots potentially have limited sound quality or processing speed.
Methods: As a first step toward the use of NAO in psychophysical testing of speech- in-speech perception, we compared normal-hearing young adults’ performance when using the standard computer interface to that when using a NAO robot to introduce the test and present all corresponding stimuli. Target sentences were presented with colour and number keywords in the presence of competing masker speech at varying target-to-masker ratios. Sentences were produced by the same speaker, but voice differences between the target and masker were introduced using speech synthesis methods. To assess test performance, speech intelligibility and data collection duration were compared between the computer and NAO setups. Human-robot interaction was assessed using the Negative Attitude Toward Robot Scale (NARS) and quantification of behavioural cues (backchannels).
Results: Speech intelligibility results showed functional similarity between the computer and NAO setups. Data collection durations were longer when using NAO. NARS results showed participants had a relatively positive attitude toward “situations of interactions” with robots prior to the experiment, but otherwise showed neutral attitudes toward the “social influence” of and “emotions in interaction” with robots. The presence of more positive backchannels when using NAO suggest higher engagement with the robot in comparison to the computer.
Discussion: Overall, the study presents the potential of the NAO for presenting speech materials and collecting psychophysical measurements for speech-in-speech perception.
Daily life often presents us with situations in which sounds with overlapping properties originating from different sources compete for our attention. Perception of speech in background noise requires segregating target speech and interfering masker signals. Further, in the case of competing background speech (speech masking), listeners need to suppress the information provided by the masking speech, oftentimes resulting in informational/perceptual masking (Carhart et al., 1969; Pollack, 1975; Mattys et al., 2009). Speakers’ voice characteristics facilitate segregating target speech from masking speech (Abercrombie, 1982; Bregman, 1990). Fundamental frequency (F0), related to the pitch of a speaker’s voice (e.g., Fitch and Giedd, 1999), and vocal-tract length (VTL), related to the size and height of a speaker (e.g., Smith and Patterson, 2005), are two such speaker voice characteristics often used in differentiating voices and speakers (Skuk and Schweinberger, 2014; Gaudrain and Başkent, 2018). Normal-hearing listeners have been shown to be sensitive to small differences in F0 and VTL cues (Gaudrain and Başkent, 2018; El Boghdady et al., 2019; Nagels et al., 2020a; Koelewijn et al., 2021), and can make effective use of these differences to differentiate between target and masker speech (Darwin et al., 2003; Drullman and Bronkhorst, 2004; Vestergaard et al., 2009; Başkent and Gaudrain, 2016; El Boghdady et al., 2019; Nagels et al., 2021). In contrast, hard-of-hearing individuals who hear via the electric stimulation of a cochlear implant (CI), a sensorineural prosthesis for the hearing-impaired, struggle in such situations, and show less sensitivity to F0 and VTL cues (El Boghdady et al., 2019). This challenge could be due to the inherent spectrotemporal degradation of electric hearing [see Başkent et al. (2016) for more information on the workings of CIs] and thus, difficulty in perceiving various speaker voice cues (Gaudrain and Başkent, 2018; El Boghdady et al., 2019). Therefore, the investigation of these vocal cues through psychophysical testing, both in clinical and research settings, is important. On the other hand, evaluation of speech-in-speech perception requires the use of long and repetitive auditory psychophysical tests to ensure data reliability (Mühl et al., 2018; Smith et al., 2018; Humble et al., 2023). This can be a challenge for individuals being tested, especially for those with short or limited attention spans, such as young children (Hartley et al., 2000; Bess et al., 2020; Cervantes et al., 2023), or those with hearing loss, such as the elderly (Alhanbali et al., 2017). Therefore, any interface or setup that can improve engagement and focus may be helpful in collecting such data.
The use of a computer auditory psychophysics testing has led to methods that allow for better controlled experiments, more complex test designs, and the varying of more test parameters (Laneau et al., 2005). This has subsequently led to the use of desktop or laptop computers as typical test interfaces for auditory psychophysical tests (Marin-Campos et al., 2021; Zhao et al., 2022). When used as the test interfaces, the computer presents stimuli and collects responses. These capabilities have also expanded the potential use of computers for psychophysics testing outside of clinical or highly controlled environments (Gallun et al., 2018). Sometimes interfaces are modified to resemble a game-like format, especially for children (Moore et al., 2008; Kopelovich et al., 2010; Nagels et al., 2021; Harding et al., 2023). However, in a previous study by Looije et al. (2012), it was shown that during learning tasks, the use of a robot was better able to hold the attention of children in comparison to a computer interface. Furthermore, literature has shown that the physical presence of a social actor, both human and human-like, has a greater effect on engagement (Lee et al., 2006), in comparison to its virtual counterpart (Kidd and Breazeal, 2004; Kontogiorgos et al., 2021). This can be leveraged to motivate users to exert more effort during a given task (Bond, 1982; Song et al., 2021). This was also reported by Marge et al. (2022), who comment that a robot can be advantageous in motivating and engaging users. Therefore, it could be that the inclusion of an interactive robot, such as the NAO humanoid robot, could be used to further retain one’s attention, especially for psychophysical tests of speech-in-speech perception.
Over the last two decades humanoid robots have gained presence in a wide range of areas, including: high-risk environments (Sulistijono et al., 2010; Kaneko et al., 2019), entertainment (Fujita et al., 2003), home (Asfour et al., 2006), and healthcare (Ting et al., 2014; Choudhury et al., 2018; Saeedvand et al., 2019), to name only a few. Joseph et al. (2018) details more specifically how humanoid robots have been involved in healthcare applications, such as assisting tasks through social interactions (McGinn et al., 2014), telehealthcare (Douissard et al., 2019), and nurse assistive tasks (Hu et al., 2011). The use of social robotics has steadily increased in recent years to the point where they are no longer only being used as research tools, but being implemented in day-to-day life (Henschel et al., 2021). The robots from Aldebaran Robotics (NAO and Pepper) are the two most frequently recurring robots in the field of social robotics. Moreover, the use of both the NAO and Pepper robots has been suggested in the literature as a facilitating interface in testing procedures for hearing research. Uluer et al. (2023), for example, have explored using a Pepper robot to increase motivation during auditory tests with CI children. The NAO has frequently been used in healthcare contexts, as shown in a scoping review by Dawe et al. (2019). Due to the robot’s small size and its friendly and human-like appearance, the NAO has been used often in the investigation of child-robot interactions (Amirova et al., 2021). Polycarpou et al. (2016) used a NAO robot with seven CI children between the ages of 5–15 years to assess their speaking and listening skills through play. Although there have been other audiological studies utilising robot interactions, to the best of our knowledge, the evaluation or analysis of the human-robot interaction (HRI) has been limited and has predominantly focussed on task performance.
User engagement (Kont and Alimardani, 2020) is one of the most frequently used metrics in human-robot interaction (HRI) analysis as it provides a measure of interaction quality, and thus one’s perception toward an interface. One’s own perception toward a robot is often performed using self-assessments, such as the Negative Attitude toward Robots Scale [NARS; (Nomura et al., 2004)]. The NARS is used to determine the attitudes one has toward communication with robots in daily life and is divided into three components: subordinate scale 1 (S1), negative attitudes toward situations and interactions with robots; S2, negative attitudes toward social influence of robots; and S3, negative attitudes toward emotions in interactions with robots. In addition to self-assessments, much can be gleaned regarding the perception toward a robot as well as user engagement through the analysis of behavioural cues using video recordings. Verbal or gestural behavioural cues, known as backchannels and defined as cues directed back to a conversation initiator to convey understanding or comprehension, and a desire for the interaction to continue (Rich et al., 2010), have also been suggested as measures to evaluate user engagement (Türker et al., 2017).
In this study, we aim to expand the use of a NAO robot in psychophysical evaluations of speech-in-speech perception. Combined with its speech-based mode of communication, the NAO robot could be a relatively low-cost tool for auditory perception evaluation. In both research and clinical contexts, such an implementation could potentially provide participants with an interactive testing interface, possibly helping with engagement and enjoyment during experiments and diagnostic measurements (Henkemans et al., 2017). On the other hand, a number of factors related to the hardware and software of the robot could potentially affect auditory testing. For example, the internal soundcard and speaker combination may not be able to produce sound stimuli of sufficient quality for all psychophysical measurements (Okuno et al., 2002), such as stimuli measured close to hearing thresholds. Non-experimental artefacts such as the noise of the fans or actuators in the robot could add unintentional background noise to the stimuli (Frid et al., 2018). Although the robot could potentially offer beneficial engagement during psychophysical tests, the different test setup with the NAO may impact the quality of the test results. Therefore, we first need to investigate how comparable the results are when conducting a psychophysics test using a robot to those when using the standard computer setup, while also evaluating the engagement factor via HRI analysis.
The present experiment is part of a large project, Perception of Indexical Cues in Kids and Adults (PICKA), and expands on previous work conducted using the same NAO robot for other psychophysical tests (Meyer et al., 2023). The purpose of the PICKA project is to investigate the perception of voice and speech in varying populations, such as normal-hearing and hard-of-hearing adults and children with varying degrees and types of hearing loss and hearing devices, and in varying languages, such as English, Dutch, and Turkish.
In the present study, the PICKA speech-in-speech perception test was used. The speech-in-speech perception test evaluates speech intelligibility of sentences presented in competing speech, using an adapted version of the coordinate response measure (CRM, Bolia et al., 2000; Brungart, 2001; Hazan et al., 2009; Welch et al., 2015). The test was performed via the computer [identical to that reported in Nagels et al. (2021)] as well as with a NAO humanoid robot named “Sam,” chosen to represent a gender-neutral name. The computer and Sam versions of the test differ slightly in their implementation, much of which was done intentionally. The implementation differences are further explained in the sections below.
To compare the test performance with the robot to both the standard computer setup and to previous relevant work, we have collected both auditory speech intelligibility scores and data collection duration. To quantify the human-robot interaction (HRI), we have collected data in the form of a questionnaire, the Negative Attitude Toward Robots Scale (NARS), a common HRI metric (Nomura et al., 2004), and behavioural cues exhibited during the experiment to explore engagement related factors.
Twenty-nine (aged 19–36; 23.46 ± 4.40 years) individuals took part in the study. Two participants did not meet the inclusion criteria for normal hearing, and therefore data for the speech-in-speech perception test was analysed from 27 participants (aged 19–36; 23.23 ± 4.43 years). However, all 29 participants were included in the analysis of the HRI as there was no inclusion criteria for this component of the study. Sample size was determined based on a rule of thumb for human-robot interaction studies in which it is recommended that a minimum of 25 participants are included per tested condition (Bartneck, 2020), and an extra four participants to account for potential drop-outs. All participants reported English as either native or additional language and completed at least high school education. A pure-tone audiogram was conducted to confirm normal hearing (NH). Hearing thresholds >20 dB HL (Hearing Level) at any of the audiometric octave frequencies (between 250 Hz and 8 kHz) qualified for exclusion. The study was conducted according to the guidelines of the Declaration of Helsinki, and the PICKA project protocol was approved by the Medical Ethical Committee (METc) at UMCG (METc 2018/427, ABR nr NL66549.042.18). Written informed consent was obtained prior to the start of the experiment. The participants were compensated €8/hr for their participation.
The CRM sentence stimuli used were in English, introduced by Hazan et al. (2009), Messaoud-Galusi et al. (2011), and Welch et al. (2015), and similar in structure to the Dutch sentences used by Nagels et al. (2021). The 48 English sentences contained a carrier phrase with a call sign (“dog” or “cat”), one colour keyword (selected from six colours: red, green, pink, white, black, and blue, all monosyllabic), and one number keyword (selected from eight numbers between 1 and 9, excluding disyllabic seven); e.g., Show the dog where the pink (colour) five (number) is. The same 48 sentences were used to create all stimuli for the present test. Each of the stimuli sets (Dutch and English) of the PICKA test battery were generated by a female speaker with a reference F0 of 242 Hz.
Target and masker sentences were originally produced by the same speaker. Speech-in-speech conditions were implemented by combining target and masker speech with two manipulations: (1) the target-to-masker ratios (TMRs) were varied, and (2) the voice cues F0 and VTL of the masker speech varied to introduce a voice difference between the target and masker speech [see El Boghdady et al. (2019) and Nagels et al. (2021) for a detailed explanation on the influence of TMR and voice cues on speech-in-speech perception]. For TMRs, expressed in dB, three conditions were used (−6 dB, 0 dB, + 6 dB). F0 and VTL voice cues were expressed in semitones (st.), an intuitive frequency increment unit used in music and expressed as 1/12th of an octave. Four different voice conditions were used: (1) the same voice parameters as the target speech, but with resynthesis to account for synthesis artefacts (ΔF0: 0 st., ΔVTL: 0.0 st.); (2 and 3) a difference of either −12 st. in F0 or + 3.8 st in VTL (ΔF0: −12 st., ΔVTL: 0.0 st.; ΔF0: 0 st., ΔVTL: + 3.8 st.); and (4) a difference of −12 st in F0 and + 3.8 st. in VTL (ΔF0: −12 st., ΔVTL: + 3.8 st.). This resulted in 12 experimental conditions (three TMRs x four voice conditions). An additional condition with no manipulations (no TMR, no voice condition) was included as a baseline condition for a check of the experiment paradigm, but not included in data analyses. Each condition was tested with 7 trials (i.e., 7 target sentences), resulting in a total of 84 experimental trials + 7 baseline trials = 91 trials in the experimental corpus, all tested within one block.
For familiarisation of the test, a small corpus of training stimuli was created with nine F0 and VTL combinations: ΔF0 = −12 st., ΔVTL = 0.0 st.; ΔF0 = −12 st., ΔVTL = + 1.9 st.; ΔF0 = −12 st., ΔVTL = + 3.8 st.; ΔF0 = −6 st., ΔVTL = 0.0 st.; ΔF0 = −6 st., ΔVTL = + 1.9 st.; ΔF0 = −6 st., ΔVTL = + 3.8 st.; ΔF0 = 0 st., ΔVTL = 0.0 st.; ΔF0 = 0 st., ΔVTL = + 1.9 st.; ΔF0 = 0 st., ΔVTL = + 3.8 st. The first two trials had a TMR of 0 dB and the remaining trials a TMR of + 6 dB. Of the nine training stimuli, four were randomly selected for the training phase of the test.
For each trial, a target sentence was randomly selected from the 48 sentences with the “dog” call sign, and the masker speech was prepared from 48 sentences with the “cat” call sign. For the masker speech, random sentences were selected while avoiding sentences with the same number and colour keywords as the target sentence. From these sentences, 150–300 ms segments were randomly selected, applying 50 ms raised cosine ramps to prevent spectral splatter, and concatenating these segments to produce the masker speech. The masker speech started 750 ms before the target sentence onset and continued for 250 ms after the target sentence offset.
The HRI was evaluated via the NARS questionnaire and behavioural data captured in video recordings of the experiment. The NARS is presented as a five-point Likert scale (1: strongly disagree—5: strongly agree), used to grade each item, and the higher the score, the more negative an attitude one has toward robots. Total scores for each of the NARS subscales are obtained by totalling the grades of each subscale (S1, S2, S3). Therefore, minimum and maximum scores are 6 and 30 for S1, 5 and 25 for S2, and 3 and 15 for S3. For the video recordings, we analysed behaviours that could be used to indicate engagement (backchannels). “Smiling” and “laughing” (Türker et al., 2017) are two behaviours which can be considered positive backchannels and therefore positive engagement. To characterise negative backchannels, “frowning,” and “grimacing” were used as opposites to smiling and laughing.
As mentioned previously, the paradigm of the speech-in-speech perception test is based on the CRM, which has been used extensively in the literature (Hazan et al., 2009; Welch et al., 2015; Semeraro et al., 2017; Nagels et al., 2021). In the standard version of the test, to log responses, participants make use of a coloured and numbered matrix representing all possible response combinations (Figure 1). Although other tests of the PICKA battery have been modified to resemble game-like interfaces (Nagels et al., 2020a,b; Meyer et al., 2023), the speech-in-speech perception test has not been similarly modified to remain consistent with literature and allow for comparison to previously reported data.
Figure 1. The standard computer user interface, showing the speech-in-speech perception coordinate response measure (CRM) test matrix as presented on the screen. Each item in the matrix represents a possible response option, corresponding to the target sentence. Bar at the top of the image depicts progress indicating how many stimuli are remaining in either the training or data collection phases. The matrix and image are published under the CC BY 4.0 licence (https://creativecommons.org/licenses/by/4.0/).
The speech-in-speech perception test was run using MATLAB 2019b (MATLAB, 2019) on an HP Notebook (Intel Core i5 7th gen) running Ubuntu 16.04. The user interface with the standard numbered matrix (Figure 1) was used, similar to Nagels et al. (2021). There are two deviations from the aforementioned study: English vs. Dutch stimuli, and use of high-quality headphones vs. internal soundcard and stereo speakers. We made use of the computer’s loudspeakers in this study to present a more comparable test setup with the NAO, on which there is no audio connection for headphones.
A NAO V5 H25 humanoid robot developed by Aldebaran Robotics (Sam) was used as an auditory interface to introduce the speech-in-speech perception test and present all corresponding stimuli. The PICKA Matlab scripts were rewritten into Python, which allowed all tests and stimuli to be stored and run directly on Sam. Housed in Sam is an Atom Z530 1.6 GHz CPU processor, 1 Gb RAM, and a total of 11 tactile sensors (three on the head, three on each hand, one bumper on each foot), two cameras and four ultrasound sensors (Figure 2A). The software locally installed on the NAO robot is the NAOqi OS, an operating system based on Gentoo Linux specifically created for NAO by the developers. A cross-platform NAOqi SDK (software development kit) framework is installed onto a computer, which can then be used to control and communicate with the robot. The NAO SDKs available are Python (Van Rossum and Drake, 2009), C + + (Stroustrup, 2000), and Java (Arnold et al., 2005). NAO has 25 degrees of freedom and is able to perform movements and actions resembling that of a human.
Figure 2. Panel (A) The robot auditory interface, NAO V5 H25 humanoid robot from Aldebaran Robotics. Panel (B) Webpage displayed on the Samsung Tablet to input participant details and begin one of the four PICKA psychophysics tests. Participant details included: participant ID, the phase of the test (either training or data collection), and the language of the test (either English or Dutch). Test buttons from left to right are for starting the different PICKA tests: voice cue sensitivity, voice gender categorization, voice emotion identification, and speech-in-speech perception (the focus of the present experiment), respectively. The cartoon illustrations were made by Jop Luberti for the purpose of the PICKA project. This image is published under the CC BY 4.0 licence (https://creativecommons.org/licenses/by/4.0/).
To improve the useability of running the PICKA tests through Sam, a simple website was designed for the researcher conducting any of the PICKA tests and hosted on Sam. Through this website, displayed on a Samsung Galaxy Tablet A, relevant participant information (e.g., participant ID and language) could be entered and the relevant PICKA auditory test could be initiated (Figure 2B). Stimuli were presented through the onboard soundcard, and the internal stereo loudspeakers located in Sam’s head. The same tablet depicted a scaled down (approximately by a factor of 1.8) version of the aforementioned standard computer matrix for participants to log their responses (Figure 1). Henceforth, the robot and tablet are referred to as the “robot setup” and “auditory interface” refers to the robot only, as the tablet is considered a response logging interface.
The computer and the NAO inherently differ in their abilities to reproduce sounds due to the different hardware. To measure the output of the speakers, a noise signal that was spectrally shaped to match the averaged spectrum of the test stimuli was used. On both the computer and Sam, the noise was presented and measurements were recorded in third-octaves using a Knowles Electronics Mannequin for Acoustic Research (KEMAR, GRAS, Holte, Denmark) head assembly and a Svantek sound level metre (Svan 979). Measurements were conducted in a sound treated room, identical to that used for experimentation. The KEMAR was placed approximately one metre away from the auditory interface, similar to how a participant would be seated during the experiment. Replicating the experimental setup, the sounds were played on both interfaces at the calibrated level of 65 dB SPL (Figure 3). To further compare these signals, the digitally extracted levels from the original noise signal used for calibration have also been included in Figure 3 (blue line) to depict its spectral shape.
Figure 3. Comparison of auditory interface speaker comparison. Yellow and red lines show the levels of the noise signal when presented at the calibrated 65 dB SPL for the computer and Sam, respectively. Each point represents the total power within a third-octave band. The blue line is the digitally extracted levels from the noise signal and shifted to the ideal presentation of 65 dB SPL.
Figure 3 shows that both the computer and Sam have relatively low level outputs below 250 Hz, compared to the original sound. Furthermore, the computer shows lower levels than Sam at frequencies below 800 Hz. To maintain the overall level of 65 dB SPL, this lack of low frequencies is then compensated above 800 Hz in the computer. These level differences will affect the perceived loudness and timbre of the sounds, and could also potentially affect audibility of lower harmonics in the speech stimuli.
Participants were seated at a desk with either the computer or Sam and the tablet placed in front of them on the desk in an unoccupied and quiet room. Participants were seated approximately one metre from the auditory interface; however, this varied as participants moved to interact with Sam or the computer. The unused setup was removed from the desk and placed outside the participants’ line of sight. To capture the behavioural HRI data, two video cameras were placed to the side and in front of the participant to capture their body positioning and facial expressions, respectively.
Prior to their experimental session, participants were requested to complete the NARS questionnaire online. The order of the setups with which participants started the test was randomised. The speech-in-speech perception test consisted of two phases: a training phase and a data collection phase. The task was the same for both training and data collection. Participants were instructed that they would hear a coherent target sentence with the call sign “dog” that contained both a colour and a number (such as “Show the dog where the red four is.”) in the presence of a speech masker to replicate a speech-in-speech listening scenario. Participants were also told that the speech masker might be louder, quieter, or have the same volume as the target, or be absent. Participants were instructed to log the heard colour and number combination on the provided colour-number matrix either by clicking with the connected mouse when using the computer or by touching the tablet screen.
Once the participant started the training phase and prior to the presentation of the first training trial, all stimuli for both the training and experimental corpora were processed with all TMR and voice conditions, and the splicing and resynthesis of speech maskers were randomised per participant. The training phase presented participants with four trials to familiarise themselves with the procedure of the test, but the participant responses were not taken into account for scoring purposes. Once confirmed by the researcher that the participant understood the test, the data collection phase started, consisting of a single block of all 91 trials (84 experimental + 7 baseline) with all sentences presented in a random order. Each logged response was then recorded as either correct or incorrect. Responses were only considered as correct when both the colour and number combination were correct. Participants performed the speech-in-speech perception test twice, once on each auditory interface with a break in-between, in a single session lasting approximately 40 min. Following the completion of the first iteration of the test on either the computer or Sam, participants were offered a break by the researcher before being seated again at the same desk with the next setup placed upon the desk.
When using the computer, participants were presented with the start screen of the test. Once “start” was clicked, the test immediately began with the training phase. Once completed, participants would again be presented with the start screen, which would initiate the data collection phase. No positive feedback was presented to participants; however, negative feedback was presented in the form of the correct colour-number pair briefly being outlined in green before continuing with the next trial. During the data collection phase, at predefined points, breaks would be offered to participants. A pop-up window would inform participants that they could take a break should they wish, and the test would resume when the pop-up window was dismissed.
When using Sam, the robot first introduced itself to the participant before explaining how the test would be carried out. Similar to the computer, first a training phase was presented to participants to familiarise themselves with the robot and the test procedure. Sam informed participants when the training phase was completed and waited for the participant to touch the top of its head to continue to the data collection phase. To maintain motivation and encouragement during the test, both positive (head nod) and negative (head shake) feedback were presented to participants throughout the training and data collection phases, as well as visual feedback to signal when a response could be logged (eyes turning green), and when the response was successfully logged (eyes return to default white). During the data collection phase, at the same predefined points as with the computer, a break was offered to participants. Sam would verbally ask the participant if they wanted to take a break, to which the participant could then verbally reply with either “yes” or “no,” If the participant decided to take a break, Sam would ask a follow-up question if they would like to stand up and join in a stretch routine. Again, the participants could respond verbally with either “yes” or “no,” If answered with “yes,” Sam would stand and perform a short stretch routine. If answered with “no,” Sam would stay in a seated position for 10 s before asking if the participant was ready to continue, again awaiting a verbal response. If “yes,” Sam continued the experiment. If answered with “no,” Sam would allow for another 10 s break before continuing the test. Once all trials were completed, Sam informed participants that they reached the end of the test and thanked them for their participation.
Test performance was quantified by speech intelligibility scores (percentage correct) and data collection duration (minutes) with the computer and Sam setups. Intelligibility scores were calculated by averaging the recorded correct responses across all presented test trials per TMR and voice condition per participant. Data collection durations were calculated from when the first trial was presented until the response of the last trial was logged. Therefore, neither the interactions with Sam in the beginning and end of the test were taken into account, nor the duration of the training phases.
A classical repeated-measures ANOVA (RMANOVA) with three-repeated factors was performed for the intelligibility: the auditory interface with which the test was performed (computer or Sam), the four voice conditions applied to the masker voice (ΔF0: 0 st., ΔVTL: 0 st.; ΔF0: −12 st., ΔVTL: 0 st.; ΔF0: 0 st., ΔVTL: + 3.8 st.; ΔF0: −12 st., ΔVTL: + 3.8 st.), and the three TMR conditions (−6 dB, 0 dB, + 6 dB), resulting in a 2 × 4 × 3 repeated-measures design. When RMANOVA tests violated sphericity, Greenhouse-Geisser corrections were applied (pgg). Evaluation of data collection phase duration was performed using paired t-tests.
As the purpose of this study is to present a potential alternative auditory interface to the computer, we aim to look for evidence that both setups (using the computer and Sam) are comparable in their data collection. Therefore, for robustness, we also conducted a Bayesian RMANOVA using the same three-repeated factors as a conclusion of similarity cannot be obtained with classical (frequentist) inference. Bayesian inferential methods focus solely on the observed data, and not on hypothetical datasets as with classical methods. Therefore, they can provide an alternative interpretation of the data, the amount of evidence, based on the observed data, that can be attributed to the presence or absence of an effect [for more detailed explanations see (Wagenmakers et al., 2018)]. The output of Bayesian inferential methods is the Bayes factor (BF) and can be denoted in one of two ways: BF01 where 0 < BF < 1 shows increasing evidence for the null hypothesis as the BF approaches 0, and BF > 1 shows increasing evidence for the alternative hypothesis as the BF approaches infinity; and BF10, which is the inverse of BF01; i.e., 0 < BF < 1 shows evidence for the alternative hypothesis, and BF > 1 shows evidence for the null hypothesis. The two notation methods can be used interchangeably for easier interpretation depending on the inference to be made. Since the intended focus of the inference of this study is evidence for the null hypothesis, the BF10 notation is used. The degree of evidence is given by different thresholds of the BF: anecdotal, 0.33 < BF < 1 or 1 < BF < 3; medium, 0.1 < BF < 0.33 or 3 < BF < 10; strong, 0.03 < BF < 0.1 or 10 < BF < 30.
Analysis of the NARS was performed using one sample t-tests were performed for each subscale to determine if the results were significantly different from the expected means (18, 15, and 9 for S1, S2, and S3, respectively), which would indicate neutrality toward interactions with robots, and thus an unbiased sample.
To analyse the behavioural data from the video recordings, two independent coders viewed the recordings and logged the frequency of displayed behaviours using the behavioural analysis software BORIS (Friard and Gamba, 2016). Total duration of raw video footage was approximately 23 h 57 min. To reduce the workload of coders, video recordings were post-processed and segments of different phases of the test were extracted. Segments were pseudo randomised and concatenated, resulting in approximately 8 h 23 min of footage to be coded. Due to the repetitive nature of the test, these segments would provide “snapshots” during the different phases. Segments were created as follows: 35 s from the introduction when using Sam (introduction in its entirety); 30 s from the training phase for both the computer and Sam; 2 min from the beginning, 1 min from the middle, and 2 min from the end of the data collection phase for both the computer and Sam; 7 s from the break during the data collection phase in the case where the total duration was less than 10, or 45 s if the break was up to a minute. Engagement was assessed using the frequency of backchannels recorded by the two coders and were compared both within and between coders. Within coder comparisons were performed using Student t-tests. Reliability between coders was evaluated using intraclass correlation [ICC; (Bartko, 1966)] based on the frequency of exhibited behaviours during each of the concatenated video segments. An ICC analysis is often used for ordinal, interval, or ratio data (Hallgren, 2012). Because the frequency of behaviours is analysed per interval of the full video recording, as well as all subjects are observed by multiple coders, this makes an ICC appropriate.
The baseline speech intelligibility scores with no speech masker showed good consistency of the experimental paradigm: 99.0% on average when using the computer, and 99.5% on average when using Sam. Figure 4 shows the intelligibility scores per TMR and voice condition across all participants. Table 1 shows the results of both the classical and Bayesian RMANOVAs performed across both setups, three TMRs and four voice conditions. Results of the classical RMANOVA showed no significant difference between participants’ intelligibility scores when using the computer or Sam [F(1, 36) = 1.090, p = 0.306, np2 = 0.040], no significant interaction between the auditory interface and the TMR [Fgg(1.490, 38.746) = 0.065, pgg = 0.888, np2 = 0.003], no significant interaction between the auditory interface and the voice condition [Fgg(2.353, 61.182) = 0.673, p = 0.537, np2 = 0.025], and no significant interaction between all three factors [F(3.643, 94.730) = 0.587, p = 0.657, np2 = 0.022].
Figure 4. Boxplots depicting the range, quartiles, and median percent correct scores of the speech-in-speech perception test, shown for each talker-to-masker ratio (TMR, rows from top to bottom) and voice condition (columns from left to right) for the computer and Sam setups (yellow and red filled boxes, respectively), and in comparison to data reported by Nagels et al. (2021; empty boxes).
Bayesian RMANOVA showed moderate evidence that the auditory interface on which the test was performed did not affect the results of the speech-in-speech perception test (BF10 = 0.185), strong evidence of no interaction between the auditory interface and the TMR (BF10 = 0.081), strong evidence of no interaction between the auditory interface and the voice condition (BF10 = 0.060), and strong evidence of no interaction between all three factors (BF10 = 0.039).
Figure 5 shows the duration of the speech-in-speech perception test when performed using each auditory interface, and in comparison, to previous data reported by Nagels et al. (2021). The average duration of the data collection phase was 9 ± 1 min on the computer and 15 ± 5.1 min on Sam. However, we observed that three outlier participants took substantially longer to complete the data collection phase when using Sam. Removing these outliers resulted in an average duration of 13 ± 1 min. The removal of the outliers showed that they had a significant effect on the total duration of the data collection phase [t(45) = −12.22, p < 0.001].
Figure 5. Duration to complete the data collection phase of the speech-in-speech perception test on the computer and Sam setups (following the removal of three outliers), and in comparison to data reported by Nagels et al. (2021).
Average scores for the subscales were 14.8 ± 3.74, 15.8 ± 2.17, and 8.5 ± 1.91 out of possible totals 30, 25, and 15 for S1, S2, and S3, respectively. One sample t-tests for each subscale showed only a statistically significant difference to the expected mean for S1 [t(19) = −3.83, p < 0.01], and non-significant differences for S2 and S3. The results are summarised in Table 2 below.
Table 2. Results of the one sample t-tests comparing each of the NARS subscales to their respective expected means (indicating neutrality).
Behavioural coding results (Figure 6) showed on average (after pooling all backchannels) more frequent “frowning” when using the computer, although not statistically significant [t(1.493) = 0.721, p > 0.05], and significantly more frequent “smiling” when using Sam [t(1) = −13, p < 0.05]. “Grimacing” and “laughing” showed near identical frequencies between the two auditory interfaces. Intraclass correlation showed poor absolute agreement between coders for the behaviours “frowning” [ICC(2, k) = 0.175] and “laughing” [ICC(2,k) = −0.375], and high correlation for the behaviours “grimacing” [ICC(2, k) = 0.671] and “smiling” [ICC(2, k) = 0.697].
Figure 6. Coded backchannel frequencies by coder 1 (C01) and coder 2 (C02) when using both the computer and Sam.
The aim of the present study was to evaluate Sam as an alternative auditory interface for the testing of speech-in-speech perception. To explore this, we compared the test performance data (both percent correct scores of intelligibility and data collection phase duration) obtained from normal-hearing young adults for the speech-in-speech perception test when using the proposed robot setup, to data when using the standard computer setup, as well as to previous studies using similar methods. Due to the inherent repetition of the speech-in-speech perception test, we propose Sam to offer an engaging experience for participants when conducting such a psychophysical test. Although there have been other studies in which psychophysical tests have been gamified to offer more engagement (Moore et al., 2008; Nagels et al., 2021; Harding et al., 2023), there may be certain tests for which gamification may not be appropriate, either to be consistent with literature, or gamification may result in an overcomplication (e.g., Hanus and Fox, 2015) of the test, having instead the opposite effect. In such cases, it may be beneficial to incorporate a social agent to facilitate engagement, not only due to its presence, but also playing an active role in the procedure. To explore this, we have also evaluated engagement with the two setups using an HRI questionnaire and analyses of behavioural data from video recordings.
Results of the classical RMANOVA showed no significant difference between the percent correct scores obtained when using the computer or Sam. In addition, there was no significant interaction between the auditory interface and TMR, auditory interface and voice condition, or a combination of auditory interface, TMR and voice condition. Results of the Bayesian RMANOVA reflected the results of the classical RMANOVA, showing strong evidence in support of the two auditory interfaces being functionally identical. Visual inspection of Figure 4 also shows that the spread of the data between the TMRs and voice conditions are identical between the two auditory interfaces, and in comparison, to data reported by Nagels et al. (2021). It is also illustrated that most incorrect answers were given when the TMR was −6 dB, and a clear ceiling effect was observed at the TMR of + 6 dB. The relatively higher percent correct scores for all conditions in the data reported by Nagels et al. (2021) could be due to several reasons. One possibility is that in their study the participants used high-quality headphones instead of the built-in loudspeakers of the computer. In addition, their stimuli were Dutch, whereas the stimuli presented to participants in the present study were English. Although Nagels et al. (2021) used Dutch stimuli, their population consisted of native Dutch-speaking participants. In the present study, participants reported English as either their native or an additional language. Therefore, the lower intelligibility scores seen in the present study in comparison to those reported by Nagels et al. (2021) may be due to a non-native effect. It is not expected that the structure of the CRM sentences would affect the intelligibility of the sentences since the paradigm of the sentence structure is intended to work across languages, as suggested by Brungart (2001). However, the English stimuli were presented by a British English speaker. This may have also affected the intelligibility of the target sentences in the presence of the masker sentences, especially in the −6 dB TMR condition, for the non-native English-speaking participants who may be more acquainted with US English, for example.
While we attempted to replicate the test procedure of Nagels et al. (2021) as closely as possible, as has been detailed above, there were some differences in the implementation of the test between the computer and Sam. Some of these implementation differences were necessary to perform a fairer comparison between the computer and Sam, but others were related to the interaction between the participant and Sam. These differences may have inadvertently introduced differences in the overall percent correct scores, resulting in the lower intelligibility scores.
In the present study, between the computer and Sam, stimuli, language, and target and masker speaker were kept consistent. Several factors were postulated to potentially limit the usability of the robot, such as the soundcard, speaker quality, processing speed, and non-experimental artefacts. An analysis of the speaker quality of the two auditory interfaces showed that there was a reduced quality of the computer in comparison to Sam, especially at lower frequency ranges. However, the consistent scores of the speech-in-speech perception test show that despite these limitations and the implementation differences between the computer and Sam, both setups were capable of presenting and collecting comparable test data. In addition, both the computer and Sam showed similar patterns in test results for the different TMR and voice conditions to those reported in literature. Therefore, the comparable results between the computer and Sam, and previously reported data, indicate that Sam can be used as an effective auditory interface for the speech-in-speech perception test with a normal-hearing population.
The duration to complete the data collection phase of the speech-in-speech perception test was longer when using Sam in comparison to when using the computer; however, this increased duration did not seem to affect the performance of Sam’s setup for collecting comparable intelligibility scores. The three outliers removed from the data collection duration were the first three participants with whom this test was conducted. During the experimental procedure with these participants, it was discovered that the pauses between stimuli were increasing. This was determined to be due to how response data was saved during the test; with each response given, the size of the save file increased, resulting in a longer duration to open and write to the file. Upon discovering this response saving issue, the test code was amended to save the results to a smaller file format during the test and subsequently saving the full results once the test was completed, thus rectifying the duration problem.
However, it can still be seen in Figure 5 that, even without the outliers, the duration of the data collection phase when using Sam was much longer than that when using the computer. We have considered several factors that could contribute to this difference. Potential delays due to online stimulus preparation were ruled out, since the stimulus corpus was created prior to the training phase. Further investigation into the data collection phase durations per participant showed that on average, there were 6 s between the logging of one response and the logging of the next response when using the computer. With Sam, however, this was on average 9 s. Closer analysis of this 3 s difference showed that this occurs due to the feedback presented to participants following their response logging (head nod or head shake). Subsequent to the completion of data collection, separate measurements were taken by timing the duration of the head movements of Sam. On average, when a correct response was given, timings showed that it took 2.5 s for Sam to nod its head and then present the next stimulus. When an incorrect response was given, this time was on average 3.2 s for Sam to shake its head before presenting the next stimulus. Bootstrap simulations using the mean accuracy as the probability of a correct or incorrect response (and thus a head nod or head shake) for the various tested conditions showed that on average, the movement of Sam’s head added 3.9 min ± 2 s over the 91 trials. No positive feedback and brief negative feedback (outlining of the correct response) was presented to participants when using the computer. The inclusion of positive and negative feedback when using Sam, although different to the computer implementation, was done to increase the social presence of the robot (Akalin et al., 2019).
As mentioned previously, engagement during repetitive auditory tasks can be challenging, especially for certain populations, and to address this challenge we propose the use of a humanoid NAO robot. The use of such an interface for these tasks, at its core, relies on interactions, consisting of both social and physical components, between humans and the robot. The NARS questionnaire we used was developed as a measure of one’s attitudes toward communication robots in daily life (Nomura et al., 2004). The NARS is further broken down into three subscales to identify the attitudes of individuals toward social interactions with robots where the higher the score, the more an individual has negative attitudes toward those situations. The subscales are: S1, negative attitudes toward situations and interactions with robots; S2, negative attitudes toward social influence of robots; and S3, negative attitudes toward emotions in interactions with robots. Performing such a questionnaire prior to any interaction involving a robot allows it to be used as a cross-reference to explain any potential skewing of subsequently collected HRI data following the interaction. Results of the NARS subscales showed that only S1 was statistically different from the expected mean. The non-significant results of subscales S2 and S3 indicate that participants had neutral attitudes towards the social influence of robots and emotions in interactions with robots, respectively. However, the lower average S1 score indicates that participants had overall a relatively positive attitude toward situations of interactions with robots prior to their interaction with Sam. This is also reflected in the behavioural backchannels, coded from the video recordings. These showed more frequent smiling when using Sam in comparison to the computer, indicating both a state of comfort and engagement with Sam. This is contrasted by the more frequent frowning (although not significant, can be seen visually in Figure 6) when using the computer, which could indicate either a state of confusion (Rozin and Cohen, 2003) or contemplation (Keltner and Cordaro, 2017). Due to the nature of the speech-in-speech perception test and its fluctuating difficulty (especially when the TMR is −6 dB and where the target and masker speech did not differ in voice cues, the most difficult listening conditions tested), the more likely interpretation of the frowning is contemplation as participants focus harder in the more difficult voice conditions. Although this appears to be more frequent with the computer, this is not necessarily to say that the computer requires more focus. With both setups, this directed focus may subsequently lead to mental fatigue during the task (Boksem and Tops, 2008). However, the results of the speech-in-speech perception test show that this increased directed focus does not affect the outcome of speech intelligibility between the computer and Sam.
Our overall results show that the NAO robot shows promise to be used as an auditory interface for speech-in-speech testing. This finding is in line with and adds to our previous work (Meyer et al., 2023), which evaluated the test performance from two other PICKA tests (voice cue sensitivity and voice gender categorization). Voice cue sensitivity test measures the smallest difference between two voice cues a listener can hear. The linguistic content seems to have little effect on the voice cue perception (Koelewijn et al., 2021), and the perceived voice could be biassed by the perceived gender of the robot (Seaborn et al., 2022). Speech-in-speech perception relies not only on processing voice and speech cues, but also on modulating attention and inhibition to separate target speech from masker speech, and further use of cognitive and linguistic mechanisms to decode the lexical content. It is not clear if a voice bias due to perceived robot gender would affect the speech intelligibility scores (Ellis et al., 1996). Despite such differing natures of these tests, our findings were consistent, and both showed comparable test performance with both setups.
In comparing the test performance between the two setups, the only significant difference between the computer and Sam was the increased duration of the speech-in-speech perception test when using Sam. Although this is predominantly due to the presentation of positive and negative feedback to participants following the logged responses, we believe that it is an important component in establishing and maintaining the social presence of Sam. Therefore, instead of attempting to decrease the overall duration of the speech-in-speech perception test on Sam by removing the visual feedback, the social interaction with Sam could be improved. This way, we accept the longer duration with the inclusion of the feedback but provide the participant with a more natural interaction when performing the test. One such way this can be accomplished is by removing the use of the Samsung Galaxy tablet, which pulls the attention away from Sam with every response and replacing it with speech recognition on Sam. This would maintain the interaction with Sam both by not forcibly moving the participants’ attention between Sam and the tablet, but also by engaging in more natural speech communication with Sam. The use of automatic speech recognition (ASR) for response logging has been explored in another study from our lab by Araiza-Illan et al. (in press) with the use of Kaldi (Povey et al., 2011), an open-source speech recognition toolkit. The ASR was used to automatically score participant’s spoken responses during a speech audiometry test. Their results show the robustness of the ASR when decoding speech from normal-hearing adults, offering a natural alternative for participants to give their responses throughout the test. Therefore, an ASR system, such as Kaldi, could be coupled with Sam, enhancing its social presence and overall interface functionality.
Literature has shown that the gamification of tests can also have beneficial effects on attention and engagement (Moore et al., 2008; Kopelovich et al., 2010; Harding et al., 2023). Although the speech-in-speech perception test has been suggested above to not be appropriate for gamification, it may indeed be interesting to explore how an intentional gamification of the test compares to the data collected here. This applies both to how speech intelligibility may be affected by such a gamification, but also how engagement may differ in comparison to Sam, especially after the implementation of speech recognition and removal of the tablet.
Both the present study and our previous work show the potential use of a NAO humanoid for speech-in-speech perception (present study) and voice manipulation perception (previous work) assessments by taking advantage of the robot’s speech-related features. Furthermore, since current technical limitations are expected to be improved in the future, the proposed setup with the NAO provides exciting application possibilities in research and clinical applications.
The datasets presented in this study can be found in online repositories. The names of the repository/repositories and accession number(s) can be found below: https://doi.org/10.34894/IAGXVF.
The studies involving humans were approved by METc (Medical Ethical Committee) at UMCG (METc 2018/427, ABR nr NL66549.042.18). The studies were conducted in accordance with the local legislation and institutional requirements. The participants provided their written informed consent to participate in this study.
LM: Conceptualization, Data curation, Formal Analysis, Funding acquisition, Investigation, Methodology, Software, Visualization, Writing – original draft, Writing – review and editing. GA-I: Conceptualization, Investigation, Methodology, Project administration, Supervision, Visualization, Writing – review and editing. LR: Conceptualization, Methodology, Supervision, Visualization, Writing – review and editing. EG: Investigation, Resources, Software, Visualization, Writing – review and editing. DB: Conceptualization, Funding acquisition, Project administration, Resources, Supervision, Writing – review and editing.
The author(s) declare financial support was received for the research, authorship, and/or publication of this article. This study was supported by the VICI grant 918-17-603 from Netherlands Organization for Scientific Research (NWO) and Netherlands Organization for Health Research and Development (ZonMw). Further support was provided by the WJ Kolff Institute for Biomedical Engineering and Material Sciences, University of Groningen, the Heinsius Houbolt Foundation and the Rosalind Franklin Fellowship from University Medical Center Groningen, University of Groningen.
We thank Josephine Marriage and Debi Vickers for sharing the English CRM stimuli, Paolo Toffanin, Iris van Bommel, Evelien Birza, Jacqueline Libert and Jop Luberti for their contribution to the development of the PICKA test battery, as well as Tord Helliesen and Conor Durkin for coding the video footage. The study was conducted in the framework of the LabEx CeLyA (ANR-10-LABX-0060/ANR-11-IDEX-0007) operated by the French ANR and is also part of the research program of the UMCG Otorhinolaryngology Department: Healthy Aging and Communication.
The authors declare that the research was conducted in the absence of any commercial or financial relationships that could be construed as a potential conflict of interest.
All claims expressed in this article are solely those of the authors and do not necessarily represent those of their affiliated organizations, or those of the publisher, the editors and the reviewers. Any product that may be evaluated in this article, or claim that may be made by its manufacturer, is not guaranteed or endorsed by the publisher.
Akalin, N., Kristoffersson, A., and Loutfi, A. (2019). The influence of feedback type in robot-assisted training. Mult. Technol. Interact. 3:67. doi: 10.3390/mti3040067
Alhanbali, S., Dawes, P., Lloyd, S., and Munro, K. J. (2017). Self-reported listening-related effort and fatigue in hearing-impaired adults. Ear Hear. 38, e39–e48. doi: 10.1097/AUD.0000000000000361
Amirova, A., Rakhymbayeva, N., Yadollahi, E., Sandygulova, A., and Johal, W. (2021). 10 years of human-NAO interaction research: A scoping review. Front. Robot. AI 8:744526. doi: 10.3389/frobt.2021.744526
Araiza-Illan, G., Meyer, L., Truong, K., and Baskent, D. (in press). Automated speech audiometry: Can it work using open-source pre-trained Kaldi-NL automatic speech recognition?
Arnold, K., Gosling, J., and Holmes, D. (2005). The java programming language. Boston, MA: Addison-Wesley Professional.
Asfour, T., Regenstein, K., Azad, P., Schroder, J., Bierbaum, A., Vahrenkamp, N., et al. (2006). “ARMAR-III: An integrated humanoid platform for sensory-motor control,” in 6th IEEE-RAS international conference on humanoid robots, Genova, 169–175. doi: 10.1109/ICHR.2006.321380
Bartko, J. J. (1966). The intraclass correlation coefficient as a measure of reliability. Psychol. Rep. 19, 3–11. doi: 10.2466/pr0.1966.19.1.3
Bartneck, C. (2020). Human–robot interaction: An introduction. Cambridge, MA: Cambridge University Press, doi: 10.1017/9781108676649
Başkent, D., and Gaudrain, E. (2016). Musician advantage for speech-on-speech perception. J. Acoust. Soc. Am. 139, EL51–EL56. doi: 10.1121/1.4942628
Başkent, D., Gaudrain, E., Tamati, T., and Wagner, A. (2016). “Perception and psychoacoustics of speech in cochlear implant users,” in Scientific foundations of audiology: perspectives from physics, biology, modeling, and medicine, eds. A. T. Cacace, E. de Kleine, A. G. Holt, and P. van Dijk (San Diego, CA: Plural Publishing, Inc).
Bess, F. H., Davis, H., Camarata, S., and Hornsby, B. W. Y. (2020). Listening-related fatigue in children with unilateral hearing loss. Lang. Speech Hear. Serv. Sch. 51, 84–97. doi: 10.1044/2019_LSHSS-OCHL-19-0017
Boksem, M. A. S., and Tops, M. (2008). Mental fatigue: Costs and benefits. Brain Res. Rev. 59, 125–139. doi: 10.1016/j.brainresrev.2008.07.001
Bolia, R. S., Nelson, W. T., Ericson, M. A., and Simpson, B. D. (2000). A speech corpus for multitalker communications research. J. Acoust. Soc. Am. 107, 1065–1066. doi: 10.1121/1.428288
Bond, C. F. (1982). Social facilitation: A self-presentational view. J. Person. Soc. Psychol. 42, 1042–1050. doi: 10.1037/0022-3514.42.6.1042
Bregman, A. S. (1990). Auditory scene analysis: The perceptual organization of Sound. Cambridge, MA: The MIT Press, doi: 10.7551/mitpress/1486.001.0001
Brungart, D. S. (2001). Informational and energetic masking effects in the perception of two simultaneous talkers. J. Acoust. Soc. Am. 109, 1101–1109. doi: 10.1121/1.1345696
Carhart, R., Tillman, T. W., and Greetis, E. S. (1969). Perceptual masking in multiple sound backgrounds. J. Acoust. Soc. Am. 45, 694–703. doi: 10.1121/1.1911445
Cervantes, J.-A., López, S., Cervantes, S., Hernández, A., and Duarte, H. (2023). Social robots and brain–computer interface video games for dealing with attention deficit hyperactivity disorder: A systematic review. Brain Sci. 13:1172. doi: 10.3390/brainsci13081172
Choudhury, A., Li, H., Greene, C., and Perumalla, S. (2018). Humanoid robot-application and influence. Arch. Clin. Biomed. Res. 2, 198–227. doi: 10.26502/acbr.50170059
Darwin, C. J., Brungart, D. S., and Simpson, B. D. (2003). Effects of fundamental frequency and vocal-tract length changes on attention to one of two simultaneous talkers. J. Acoust. Soc. Am. 114, 2913–2922. doi: 10.1121/1.1616924
Dawe, J., Sutherland, C., Barco, A., and Broadbent, E. (2019). Can social robots help children in healthcare contexts? A scoping review. BMJ Paediatr. Open 3:e000371. doi: 10.1136/bmjpo-2018-000371
Douissard, J., Hagen, M. E., and Morel, P. (2019). “The da Vinci Surgical System,” in Bariatric robotic surgery: A comprehensive guide, eds C. E. Domene, K. C. Kim, R. V. Puy, and P. Volpe (Berlin: Springer International Publishing), 13–27. doi: 10.1007/978-3-030-17223-7_3
Drullman, R., and Bronkhorst, A. W. (2004). Speech perception and talker segregation: Effects of level, pitch, and tactile support with multiple simultaneous talkers. J. Acoust. Soc. Am. 116, 3090–3098. doi: 10.1121/1.1802535
El Boghdady, N., Gaudrain, E., and Başkent, D. (2019). Does good perception of vocal characteristics relate to better speech-on-speech intelligibility for cochlear implant users? J. Acoust. Soc. Am. 145, 417–439. doi: 10.1121/1.5087693
Ellis, L., Reynolds, L., Fucci, D., and Benjamin, B. (1996). Effects of gender on listeners’ judgments of speech intelligibility. Percept. Mot. Skills 83(3 Pt 1), 771–775. doi: 10.2466/pms.1996.83.3.771
Fitch, W. T., and Giedd, J. (1999). Morphology and development of the human vocal tract: A study using magnetic resonance imaging. J. Acoust. Soc. Am. 106(3 Pt 1), 1511–1522. doi: 10.1121/1.427148
Friard, O., and Gamba, M. (2016). BORIS: A Free, versatile open-source event-logging software for video/audio coding and live observations. Methods Ecol. Evolut. 7, 1325–1330. doi: 10.1111/2041-210X.12584
Frid, E., Bresin, R., and Alexanderson, S. (2018). Perception of Mechanical Sounds Inherent to Expressive Gestures of a NAO Robot - Implications for Movement Sonification of Humanoids. Proceedings of the Sound and Music Computing Conference 2018 (SMC2018), Limassol. doi: 10.5281/zenodo.1422499
Fujita, M., Kuroki, Y., Ishida, T., and Doi, T. T. (2003). “A small humanoid robot SDR-4X for entertainment applications,” in Proceedings IEEE/ASME international conference on advanced intelligent mechatronics (AIM 2003), Vol. 2, Kobe, 938–943. doi: 10.1109/AIM.2003.1225468
Gallun, F. J., Seitz, A., Eddins, D. A., Molis, M. R., Stavropoulos, T., Jakien, K. M., et al. (2018). “Development and validation of portable automated rapid testing (PART) measures for auditory research,” in Proceedings of meetings on acoustics. Acoustical society of America, Vol. 33, Boston, MA, 050002. doi: 10.1121/2.0000878
Gaudrain, E., and Başkent, D. (2018). Discrimination of voice pitch and vocal-tract length in cochlear implant users. Ear Hear. 39, 226–237. doi: 10.1097/AUD.0000000000000480
Hallgren, K. A. (2012). Computing inter-rater reliability for observational data: An overview and tutorial. Tutorials Quant. Methods Psychol. 8, 23–34. doi: 10.20982/tqmp.08.1.p023
Hanus, M. D., and Fox, J. (2015). Assessing the effects of gamification in the classroom: A longitudinal study on intrinsic motivation, social comparison, satisfaction, effort, and academic performance. Comput. Educ. 80, 152–161. doi: 10.1016/j.compedu.2014.08.019
Harding, E. E., Gaudrain, E., Hrycyk, I. J., Harris, R. L., Tillmann, B., Maat, B., et al. (2023). Musical emotion categorization with vocoders of varying temporal and spectral content. Trends Hear. 27:23312165221141142. doi: 10.1177/23312165221141142
Hartley, D. E., Wright, B. A., Hogan, S. C., and Moore, D. R. (2000). Age-related improvements in auditory backward and simultaneous masking in 6- to 10-year-old children. J. Speech Lang. Hear. Res. 43, 1402–1415. doi: 10.1044/jslhr.4306.1402
Hazan, V., Messaoud-Galusi, S., Rosen, S., Nouwens, S., and Shakespeare, B. (2009). Speech perception abilities of adults with dyslexia: Is there any evidence for a true deficit? J. Speech Langu. Hear. Res. 52, 1510–1529. doi: 10.1044/1092-4388(2009/08-0220)
Henkemans, O. A. B., Bierman, B. P. B., Janssen, J., Looije, R., Neerincx, M. A., van Dooren, M. M. M., et al. (2017). Design and evaluation of a personal robot playing a self-management education game with children with diabetes type. Int. J. Hum. Comput. Stud. 106, 63–76. doi: 10.1016/j.ijhcs.2017.06.001
Henschel, A., Laban, G., and Cross, E. S. (2021). What makes a robot social? A review of social robots from science fiction to a home or hospital near you. Curr. Robot. Rep. 2, 9–19. doi: 10.1007/s43154-020-00035-0
Hu, J., Edsinger, A., Lim, Y.-J., Donaldson, N., Solano, M., Solochek, A., et al. (2011). “An advanced medical robotic system augmenting healthcare capabilities – Robotic nursing assistant,” in IEEE International conference on robotics and automation, Shanghai, 6264–6269. doi: 10.1109/ICRA.2011.5980213
Humble, D., Schweinberger, S. R., Mayer, A., Jesgarzewsky, T. L., Dobel, C., and Zäske, R. (2023). The Jena Voice Learning and memory test (JVLMT): A standardized tool for assessing the ability to learn and recognize voices. Behav. Res. Methods 55, 1352–1371. doi: 10.3758/s13428-022-01818-3
Joseph, A., Christian, B., Abiodun, A. A., and Oyawale, F. (2018). A review on humanoid robotics in healthcare. MATEC Web Confer. 153:02004. doi: 10.1051/matecconf/201815302004
Kaneko, K., Kaminaga, H., Sakaguchi, T., Kajita, S., Morisawa, M., Kumagai, I., et al. (2019). Humanoid robot HRP-5P: An electrically actuated humanoid robot with high-power and wide-range joints. IEEE Robot. Automat. Lett. 4, 1431–1438. doi: 10.1109/LRA.2019.2896465
Keltner, D., and Cordaro, D. T. (2017). “Understanding multimodal emotional expressions: Recent advances in basic emotion theory,” in The science of facial expression, eds J. A. Russell and J. M. F. Dols (Oxford: Oxford University Press), doi: 10.1093/acprof:oso/9780190613501.003.0004
Kidd, C. D., and Breazeal, C. (2004). “Effect of a robot on user perceptions,” in Conference on intelligent robots and systems (IROS), Vol. 4, ed. R. S. J. International Piscataway, NJ: IEEE Publications, 3559–3564. doi: 10.1109/IROS.2004.1389967
Koelewijn, T., Gaudrain, E., Tamati, T., and Baskent, D. (2021). The effects of lexical content, acoustic and linguistic variability, and vocoding on voice cue perception. J. Acoust. Soc. Am. 150, 1620–1634. doi: 10.1121/10.0005938
Kont, M., and Alimardani, M. (2020). “Engagement and mind perception within human–robot interaction: A comparison between elderly and young adults,” in Social robotics. Lecture notes in computer science, Vol. 12483, ed. A. R. Wagner (Berlin: Springer International Publishing), 344–356. doi: 10.1007/978-3-030-62056-1_29
Kontogiorgos, D., Pereira, A., and Gustafson, J. (2021). Grounding behaviours with conversational interfaces: Effects of embodiment and failures. J. Mult. User Interf. 15, 239–254. doi: 10.1007/s12193-021-00366-y
Kopelovich, J. C., Eisen, M. D., and Franck, K. H. (2010). Frequency and electrode discrimination in children with cochlear implants. Hear. Res. 268, 105–113. doi: 10.1016/j.heares.2010.05.006
Laneau, J., Boets, B., Moonen, M., van Wieringen, A., and Wouters, J. (2005). A flexible auditory research platform using acoustic or electric stimuli for adults and young children. J. Neurosci. Methods 142, 131–136. doi: 10.1016/j.jneumeth.2004.08.015
Lee, K. M., Peng, W., Jin, S.-A., and Yan, C. (2006). Can robots manifest personality: An empirical test of personality recognition, social responses, and social presence in human–robot interaction. J. Commun. 56, 754–772. doi: 10.1111/j.1460-2466.2006.00318.x
Looije, R., van der Zalm, A., Neerincx, M. A., and Beun, R.-J. (2012). “Help, I Need Some Body the effect of embodiment on playful Learning. In IEEE RO-MAN,” in The 21st IEEE international symposium on robot and human interactive communication, 2012, Piscataway, NJ: IEEE Publications, 718–724. doi: 10.1109/ROMAN.2012.6343836
Marge, M., Espy-Wilson, C., Ward, N. G., Alwan, A., Artzi, Y., Bansal, M., et al. (2022). Spoken language interaction with robots: Recommendations for future research. Comput. Speech Lang. 71:101255. doi: 10.1016/j.csl.2021.101255
Marin-Campos, R., Dalmau, J., Compte, A., and Linares, D. (2021). StimuliApp: Psychophysical tests on mobile devices. Behav. Res. Methods 53, 1301–1307. doi: 10.3758/s13428-020-01491-4
Mattys, S. L., Brooks, J., and Cooke, M. (2009). Recognizing speech under a processing load: Dissociating energetic from informational factors. Cogn. Psychol. 59, 203–243. doi: 10.1016/j.cogpsych.2009.04.001
McGinn, C., Cullinan, M., Holland, D., and Kelly, K. (2014). “Towards the design of a new humanoid robot for domestic applications,” in IEEE international conference on technologies for practical robot applications (TePRA), Woburn, MA, 1–6. doi: 10.1109/TePRA.2014.6869155
Messaoud-Galusi, S., Hazan, V., and Rosen, S. (2011). Investigating speech perception in children with dyslexia: Is there evidence of a consistent deficit in individuals? J. Speech Lang. Hear. Res. 54, 1682–1701. doi: 10.1044/1092-4388(2011/09-0261)
Meyer, L., Rachman, L., Araiza-Illan, G., Gaudrain, E., and Başkent, D. (2023). Use of a humanoid robot for auditory psychophysical testing. PLoS One 18:e0294328. doi: 10.1371/journal.pone.0294328
Moore, D. R., Ferguson, M. A., Halliday, L. F., and Riley, A. (2008). Frequency discrimination in children: Perception. Learning and attention. Hear. Res. 238, 147–154. doi: 10.1016/j.heares.2007.11.013
Mühl, C., Sheil, O., Jarutytė, L., and Bestelmeyer, P. E. G. (2018). The Bangor voice matching test: A standardized test for the assessment of voice perception ability. Behav. Res. Methods 50, 2184–2192. doi: 10.3758/s13428-017-0985-4
Nagels, L., Gaudrain, E., Vickers, D., Hendriks, P., and Başkent, D. (2020a). Development of voice perception is dissociated across gender cues in school-age children. Sci. Rep. 10:5074. doi: 10.1038/s41598-020-61732-6
Nagels, L., Gaudrain, E., Vickers, D., Matos Lopes, M. M., Hendriks, P., and Başkent, D. (2020b). Development of vocal emotion recognition in school-age children: The EmoHI test for hearing-impaired populations. PeerJ 8:e8773. doi: 10.7717/peerj.8773
Nagels, L., Gaudrain, E., Vickers, D., Hendriks, P., and Baskent, D. (2021). School-age children benefit from voice gender cue differences for the perception of speech in competing speech. J. Acoust. Soc. Am. 149, 3328–3344. doi: 10.1121/10.0004791
Nomura, T., Kanda, T., Suzuki, T., and Kato, K. (2004). “Psychology in human–robot communication: An attempt through investigation of negative attitudes and anxiety toward robots,” in RO-MAN 2004. 13th IEEE international workshop on robot and human interactive communication (IEEE Catalog No.04TH8759), Kurashiki, 35–40. doi: 10.1109/ROMAN.2004.1374726
Okuno, H., Nakadai, K., and Kitano, H. (2002). “Social interaction of humanoid robot based on audio-visual tracking,” in Developments in applied artificial intelligence. IEA/AIE 2002. Lecture notes in computer science, Vol. 2358, eds T. Hendtlass and M. Ali (Berlin: Springer), 140–173. doi: 10.1007/3-540-48035-8_70
Pollack, I. (1975). Auditory informational masking. J. Acoust. Soc. Am. 57, (Suppl. 1):S5. doi: 10.1121/1.1995329
Polycarpou, P., Andreeva, A., Ioannou, A., and Zaphiris, P. (2016). “Don’t read my lips: Assessing listening and speaking skills through play with a humanoid robot,” in HCI international 2016 – Posters’ extended abstracts, ed. C. Stephanidis Berlin: Springer International Publishing, 255–260. doi: 10.1007/978-3-319-40542-1_41
Povey, D., Ghoshal, A., Boulianne, G., Burget, L., Glembek, O., Goel, N., et al. (2011). “The Kaldi speech recognition toolkit,” in In Hilton Waikoloa village IEEE 2011 workshop on automatic speech recognition and understanding, Piscataway, NJ: IEEE Publications Signal Processing Society.
Rich, C., Ponsler, B., Holroyd, A., and Sidner, C. L. (2010). “Recognizing engagement in human–robot interaction,” in In Proceeding of the 5th ACM/IEEE international conference on human-robot interaction—HRI 2010, Vol. 375, Osaka: ACM Press, doi: 10.1109/HRI.2010.5453163
Rozin, P., and Cohen, A. B. (2003). High frequency of facial expressions corresponding to confusion, concentration, and worry in an analysis of naturally occurring facial expressions of Americans. Emotion 3, 68–75. doi: 10.1037/1528-3542.3.1.68
Saeedvand, S., Jafari, M., Aghdasi, H. S., and Baltes, J. (2019). A comprehensive survey on humanoid robot development. Knowl. Eng. Rev. 34:e20. doi: 10.1017/S0269888919000158
Seaborn, K., Miyake, N. P., Pennefather, P., and Otake-Matsuura, M. (2022). Voice in human–agent interaction: A survey. ACM Comput. Surveys 54, 1–43. doi: 10.1145/3386867
Semeraro, H. D., Rowan, D., van Besouw, R. M., and Allsopp, A. A. (2017). Development and evaluation of the British English coordinate response measure speech-in-noise test as an occupational hearing assessment tool. Int. J. Audiol. 56, 749–758. doi: 10.1080/14992027.2017.1317370
Skuk, V. G., and Schweinberger, S. R. (2014). Influences of fundamental frequency, formant frequencies, aperiodicity, and spectrum level on the perception of voice gender. J. Speech Lang. Hear. Res. 57, 285–296. doi: 10.1044/1092-4388(2013/12-0314)
Smith, D. R. R., and Patterson, R. D. (2005). The interaction of glottal-pulse rate and vocal-tract length in judgements of speaker size, sex, and age. J. Acoust. Soc. Am. 118, 3177–3186. doi: 10.1121/1.2047107
Smith, M. L., Cesana, M. L., Farran, E. K., Karmiloff-Smith, A., and Ewing, L. (2018). A “spoon full of sugar” helps the medicine go down: How a participant friendly version of a psychophysics task significantly improves task engagement, Performance and data quality in a typical adult sample. Behav. Res. Methods 50, 1011–1019. doi: 10.3758/s13428-017-0922-6
Song, H., Barakova, E. I., Markopoulos, P., and Ham, J. (2021). Personalizing HRI in musical instrument practicing: The influence of robot roles (evaluative versus nonevaluative) on the Child’s motivation for children in different learning stages. Front. Robot. AI 8:699524. doi: 10.3389/frobt.2021.699524
Sulistijono, I. A., Setiaji, O., Salfikar, I., and Kubota, N. (2010). “Fuzzy walking and turning tap movement for humanoid soccer robot EFuRIO,” in International conference on fuzzy systems, (Barcelona), 1–6. doi: 10.1109/FUZZY.2010.5584423
Ting, C., Yeo, W.-H. Y., King, Y.-J., Chuah, Y.-D., Lee, J.-V., and Khaw, W.-B. (2014). Humanoid robot: A review of the architecture, applications and future trend. Res. J. Appl. Sci. Eng. Technol. 7, 1364–1369. doi: 10.19026/rjaset.7.402
Türker, B. B., Buçinca, Z., Erzin, E., Yemez, Y., and Sezgin, M. (2017). “Analysis of Engagement and User Experience with a Laughter Responsive Social Robot,” in Interspeech, Stockholm: ISCA, 844–848. doi: 10.21437/Interspeech.2017-1395
Uluer, P., Kose, H., Gumuslu, E., and Barkana, D. E. (2023). Experience with an affective robot assistant for children with hearing disabilities. Int. J. Soc. Robot. 15, 643–660. doi: 10.1007/s12369-021-00830-5
Vestergaard, M. D., Fyson, N. R. C., and Patterson, R. D. (2009). The interaction of vocal characteristics and audibility in the recognition of concurrent Syllablesa. J. Acoust. Soc. Am. 125, 1114–1124. doi: 10.1121/1.3050321
Wagenmakers, E.-J., Marsman, M., Jamil, T., Ly, A., Verhagen, J., Love, J., et al. (2018). Bayesian inference for psychology. Part I: Theoretical advantages and practical ramifications’. Psychon. Bull. Rev. 25, 35–57. doi: 10.3758/s13423-017-1343-3
Welch, G. F., Saunders, J., Edwards, S., Palmer, Z., Himonides, E., Knight, J., et al. (2015). Using singing to nurture children’s hearing? A pilot study. Cochlear Implants Int. 16, (Suppl. 3), S63–S70. doi: 10.1179/1467010015Z.000000000276
Keywords: speech perception, psychophysics testing, speech masking, NAO robot, human robot interaction
Citation: Meyer L, Araiza-Illan G, Rachman L, Gaudrain E and Başkent D (2024) Evaluating speech-in-speech perception via a humanoid robot. Front. Neurosci. 18:1293120. doi: 10.3389/fnins.2024.1293120
Received: 12 September 2023; Accepted: 15 January 2024;
Published: 09 February 2024.
Edited by:
Karina De Sousa, University of Pretoria, South AfricaReviewed by:
Sam Denys, KU Leuven, BelgiumCopyright © 2024 Meyer, Araiza-Illan, Rachman, Gaudrain and Başkent. This is an open-access article distributed under the terms of the Creative Commons Attribution License (CC BY). The use, distribution or reproduction in other forums is permitted, provided the original author(s) and the copyright owner(s) are credited and that the original publication in this journal is cited, in accordance with accepted academic practice. No use, distribution or reproduction is permitted which does not comply with these terms.
*Correspondence: Luke Meyer, bC5tZXllckBydWcubmw=
Disclaimer: All claims expressed in this article are solely those of the authors and do not necessarily represent those of their affiliated organizations, or those of the publisher, the editors and the reviewers. Any product that may be evaluated in this article or claim that may be made by its manufacturer is not guaranteed or endorsed by the publisher.
Research integrity at Frontiers
Learn more about the work of our research integrity team to safeguard the quality of each article we publish.