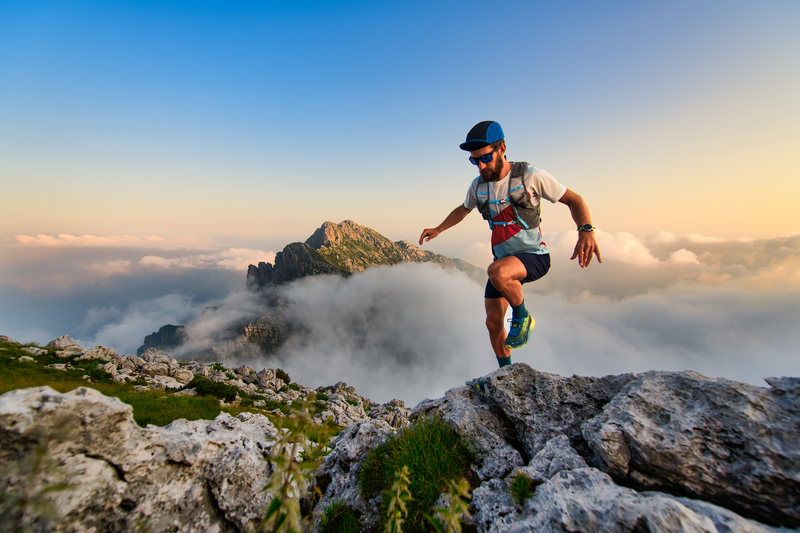
95% of researchers rate our articles as excellent or good
Learn more about the work of our research integrity team to safeguard the quality of each article we publish.
Find out more
CLINICAL TRIAL article
Front. Neurosci. , 16 November 2023
Sec. Translational Neuroscience
Volume 17 - 2023 | https://doi.org/10.3389/fnins.2023.1282496
This article is part of the Research Topic New Insights into Brain Imaging Methods for Rehabilitation of Brain Diseases View all 19 articles
Background: Previous studies showed that cerebral small vessel disease (cSVD) is a leading cause of cognitive decline in elderly people and the development of Alzheimer’s disease. Although brain structural changes of cSVD have been documented well, it remains unclear about the properties of brain intrinsic spontaneous activity in patients with cSVD.
Methods: We collected resting-state fMRI (rs-fMRI) and T1-weighted 3D high-resolution brain structural images from 41 cSVD patients and 32 healthy controls (HC). By estimating the amplitude of low-frequency fluctuation (ALFF) under three different frequency bands (typical band: 0.01–0.1 Hz; slow-4: 0.027–0.073 Hz; and slow-5: 0.01–0.027 Hz) in the whole-brain, we analyzed band-specific ALFF differences between the cSVD patients and controls.
Results: The cSVD patients showed uniformly lower ALFF than the healthy controls in the typical and slow-4 bands (pFWE < 0.05). In the typical band, cSVD patients showed lower ALFF involving voxels of the fusiform, hippocampus, inferior occipital cortex, middle occipital cortex, insula, inferior frontal cortex, rolandic operculum, and cerebellum compared with the controls. In the slow-4 band, cSVD patients showed lower ALFF involving voxels of the cerebellum, hippocampus, occipital, and fusiform compared with the controls. However, there is no significant between-group difference of ALFF in the slow-5 band. Moreover, we found significant “group × frequency” interactions in the left precuneus.
Conclusion: Our results suggested that brain intrinsic spontaneous activity of cSVD patients was abnormal and showed a frequency-specific characteristic. The ALFF in the slow-4 band may be more sensitive to detecting a malfunction in cSVD patients.
- cSVD patients showed uniformly lower ALFF than controls at typical frequency band and slow-4 band.
- At The typical frequency band (0.01–0.1 Hz), cSVD patients Had lower ALFF In The cerebellum, occipital cortex, and hippocampus compared To healthy controls.
Cerebral small vessel disease (cSVD) refers to an intracranial vascular disease that involves various pathological and neurological processes affecting brain blood vessels (Feng et al., 2021). The disease of cSVD exhibits a high prevalence, which largely exceeds that of large-vessel stroke (Dey et al., 2016). Up to 45% of dementia cases may be brought on by CSVD, which also causes 20% of all strokes worldwide, 25% of which are ischemic strokes (also known as lacunar strokes). It is responsible for roughly 20% of all strokes worldwide and 25% of ischemic strokes (also known as lacunar strokes), of which 20% result in disability for the patient (Pantoni, 2010). Moreover, cSVD is one of main factors leading to vascular cognitive decline and dementia (Cai et al., 2015) and contributing to the pathogenesis of Alzheimer’s disease (AD) (Liu et al., 2018; Kim et al., 2020). Recently, structural magnetic resonance imaging (MRI) techniques have been widely applied to identify brain structural neuroimaging markers associated with cSVD (Chen et al., 2019). Although originating from different pathogenesis, cSVD may commonly exhibit similar structural markers including small subcortical infarcts, vascular lacunes, vascular white-matter hyperintensity, cerebral microbleeds, perivascular space and brain atrophy (Staals et al., 2015; De Guio et al., 2016; Chen et al., 2019). In addition to tremendous endeavors made in the structural field, current studies pertaining to brain functional properties are progressively increasing.
The resting-state functional MRI (rs-fMRI) has been applied to investigate the relationship between cognitive impairment and brain functional activity using the amplitude of low-frequency fluctuation (ALFF) (Zang et al., 2007) in patients with cSVD (Zhou et al., 2020; Feng et al., 2021; Li et al., 2021; Mo et al., 2023). Low-frequency oscillation ranging from 0.01 to 0.1 Hz has been identified as a representative indicator of brain spontaneous activity (Zou et al., 2008; Zuo et al., 2010), and the ALFF, the square root of the power spectrum of the frequency range, is supposed to reflect brain regional spontaneous activity (Zang et al., 2007). Moreover, the regional ALFF changes may function as diagnostic biomarker for cSVD (Feng et al., 2021).
Given the fact that different oscillatory bands usually have different generation mechanisms and different physiological functions (Buzsáki and Draguhn, 2004). The detection of different brain tissues may be more sensitive under a specific frequency spectrum (Zuo et al., 2010; Qi et al., 2020; Sasai et al., 2021). Previous studies (Zuo et al., 2010; Qi et al., 2020) subdivided frequency spectrums of BOLD signals into several frequency bands such as the slow-6 (0.0052–0.01 Hz), slow-5 (0.01–0.027 Hz), slow-4 (0.027–0.073 Hz), slow−3 (0.073–0.198 Hz) and slow-2 (0.198–0.25 Hz) to compare frequency-specific ALFF values in different brain regions. For example, the basal ganglia, thalamus, and precuneus were found to show higher ALFF value in the slow-4 band than that in the slow-5 band (Zuo et al., 2010). Several studies showed a strong association between local brain abnormalities in psychiatric disorders and neural activity in specific frequency bands (slow-4 and slow-5 bands) (Wu et al., 2020; Liao et al., 2021; Ren et al., 2021). Wang et al. (2021) compared the difference in ALFF between AD and aMCI patients under three different frequency bands. They suggest that ALFF in the slow-5 band may be able to help identify severe AD and aMCI. So far, little is known about the functional brain activity of cSVD patients with cognitive impairment in response to different frequency bands. Therefore, we attempted to study the characteristic performance of ALFF values in patients with cognitive impairment at different frequency bands.
This study addressed this problem by examining frequency-dependent neural activity in cSVD patients during the resting state. It is the first study to undertake the spontaneous neural activity of specific frequency bands in cSVD. There are two primary aims of this study: 1. To investigate whether cSVD patients would show abnormal ALFF in regions associated with cognitive function; 2. To ascertain whether these abnormalities would be associated with specific frequency bands. The present study detected the brain regions with ALFF alterations (0.01–0.1 Hz) in cSVD patients contrasting with controls. Based on the specificity of sub-bands, we attempted to reveal the brain regions with abnormal ALFF in slow-4 (0.027–0.073 Hz) and slow-5 (0.01–0.027 Hz), separately.
We recruited 41 cSVD patients with right-handedness for this study from December 2016 to December 2018 from the inpatient and outpatient of the Neurology Department of Zhongshan TCM Hospital, Guangdong, China. Two experienced neurologists (XH, Huang; SQ, Ling) screened the participants based on the diagnostic criteria. In addition, we also recruited 32 sex-matched healthy participants as the healthy controls (HCs). This study was approved by the Institutional Review Board (IRB) of Zhongshan TCM Hospital (ClinicalTrials.gov identifier: 2016ZSZY-LLK-028). Written informed consent for each patient and the healthy participant was obtained before the study. Table 1 lists the clinical and demographic characteristics of the participants.
Table 1. Demography characteristics for the patients with cerebral small vessel disease (cSVD) and the healthy controls (HCs).
The cSVD patients were diagnosed according to the Neuroimaging Standards for Research into Small Vessel Disease (Wardlaw et al., 2013). Specifically, the diagnostic standard for imaging of cSVD included: (i) Recent small subcortical infarct: Axial views show an infarct diameter less than 20 mm, which can be larger than 20 mm in the coronal or sagittal view. (ii) Lacunes of presumed vascular origin: round or ovoid in shape, 3-15 mm in diameter, distributed in subcortical regions, filled with the same signals as cerebrospinal fluid (CSF). (iii) white matter hyperintensity (WMH) of presumed vascular origin: abnormal brain white matter (WM) signals, the range of lesions can vary in size, showing a high signal on the T2-weighted or T2-weighted FLAIR images. (iv) Perivascular space: the signal of perivascular space is the same as that of the CSF in all MRI sequences. The shape was linear when the image plane ran parallel to the blood vessels and round or oval when running perpendicular to the blood vessels, usually less than 3 mm in diameter. (v) Cerebral microbleeds: cerebral microbleeds are defined as the following changes in the images obtained with T2*-weighted gradient-echo sensitive to magnetizing effects, for instance, (1) small round or oval, clear boundary, homogeneity, lack of signal focus; (2) diameter in 2-5 mm, maximum 10 mm, and the lesion is surrounded by the brain parenchyma; (3) brain atrophy: reduced brain volume, but it was not associated with mainly specific focal lesions such as trauma and cerebral infarction.
The diagnostic criteria of vascular cognitive impairment (VCI) [37] indicate a continuum of clinical manifestations for cSVD patients. Mild VCI refers to impairment in at least one cognitive domain and mild to no impairment in instrumental activities of daily living (IADLs)/activities of daily living (ADLs) (independent of the motor/sensory sequelae of the vascular event). Major VCI refers to clinically significant deficits of sufficient severity in at least one cognitive domain (deficits may be present in multiple domains) and severe disruption to IADLs/ADLs (independent of the motor/sensory sequelae of the vascular event).
The inclusion criteria for the cSVD patients were as follows: (a) the patients aged in a range of 40–80 years old, (b) the patients or legal guardians agreed and signed informed consents, (c) the patient was confirmed to satisfy the diagnostic imaging criteria for cSVD and the diagnostic criteria for VCI, and (d) the patient was in mild to moderate cognitive impairment with a mini-mental state examination (MMSE) screening score in 9–24 points. Patients meeting all these criteria were included in our study. The exclusion criteria for the patients included: (a) the cognitive dysfunction caused by macrovascular and cardiogenic cerebral embolism confirmed by examination; (b) non-vascular causes of WM degeneration and pure AD; (c) those who have been confirmed to have brain tumors, brain trauma, cerebral parasitic diseases, encephalitis and other diseases that can cause cognitive impairment; (d) patients with severe speech, vision, hearing or mental disorders that affect cognitive testing and cognitive training; (e) patients suffered from depression and other neuropsychological disorders resulting in cognitive impairment; (f) patients have the history of alcohol and drug abuse; (g) patients combined with severe heart, liver, kidney endocrine system, and hematopoietic system diseases; and (h) participating in other clinical trials.
Each patient was requested to attend the clinical assessments, such as a medical history inquiry, a neurological examination, and a series of neuropsychological tests, which included the verbal fluency test (VFT), digit span test (DST), and digit symbol substitution test (DSST).
The VFT is a widely used neuropsychological scale mainly to measure cognitive, verbal, and executive functions (Vaucheret Paz et al., 2020). It consists of three subtests to detect semantic, speech, and motion fluency, respectively. (1) semantic subtest: ask the participants to say as many animals, vegetable, or fruit words as possible in one minute; (2) speech subtest: ask the participants to say as many words as possible, starting with “Fa” in one minute; (3) motion subtest: participants were asked to say as many words as possible in one minute about an event that could occur in a particular location (e.g., kitchen).
The DST (Leung et al., 2011) consists of two parts, a digit forward and a digit backward. During the test process, the participants are asked to remember two numbers simultaneously, read by the researcher with the speed of one digit per second starting with the first set. If the participants pass the 2-digit number, then the 3-digit number is measured, and so on. If the participant does not pass the 8-digit number, the 8-digit number of the second set will be read, and when the participant passes the 8-digit number of the second set, the 9-digit number will be read. If the participant fails to pass the 8-digit number of the second set, his score will be “7-digit.”
The DSST was used to evaluate multiple aspects of cognitive function, such as executive function, processing speed, attention, and working memory (Baune et al., 2018). According to the diagram at the top of the scale, the participants filled in the matching numbers under each symbol in the table below as quickly as they could. Rating instructions are that the number of correct answers in 90 s is the final score, not including the numbers filled in during the practice. The number of correct answers will be counted as the total score.
All images were obtained on a GE 3T MRI scanner with an 8-channel phased-array head coil. The participant was requested to keep their eyes closed, relax but not fall asleep, and minimize head movement during the scanning. Functional images were collected with a gradient-echo echo-planar imaging (EPI) sequence with the following parameters: repetition time (TR) = 2,000 ms, echo time (TE) = 30 ms, flip angle (FA) = 90°, field of vision (FOV) = 240 mm × 240 mm, slice thickness = 3.5 mm, inter-slice gap = 0.7 mm, data matrix = 64 × 64, 33 interleaved axial slices coving the whole brain, and 240 volumes acquired in about 8 min. In addition, high-resolution brain structural images were acquired using a T1-weighed 3D BRAVO sequence with the following parameters: TR = 8.0 ms, TE = 3.0 ms, FA = 12°, data matrix = 256 × 256, FOV = 256 mm × 256 mm, slice thickness = 1 mm, and 188 sagittal slices covering the whole brain. The conventional T1-weighted and T2-weighted FLAIR images were acquired for clinical assessment. All MRI images for each participant were acquired in the same session.
The rs-fMRI data were preprocessed using the DPARSF toolbox1 based on Matlab2012a (Mathworks, Inc., Massachusetts). Before pre-processing the data, we visually inspected both brain functional and structural images and excluded the datasets with significant signal dropouts, distortion, and other quality problems. The procedure of pre-processing included: (1) removing the first 10 volumes to keep the magnetization equilibrium; (2) performing slice-timing and head-movement correction to remove effects caused by slice acquisition time differences and head movements; (3) conducting a linear co-registration between functional images and structural images for each participant; (4) regressed out signals of the WM and CSF, and head-movement parameters (Friston-24 model); (5) performed a non-linear transformation between structural images and template brain images of the Montreal Neurological Institute (MNI) space, and normalized functional images into the MNI space with 3 × 3 × 3 mm3 voxel size and smoothed with a Gaussian kernel of 5 mm full width at half maximum (FWHM), and (6) performed temporal band-pass filtering for the typical band (0.01–0.1 Hz), the slow-4 band (0.027–0.073 Hz), and the slow-5 band (0.01–0.027 Hz), respectively. In this study, the fMRI data for subjects with head motion displacement >2mm or rotation >2° in any axis (x, y, and z-axis) were discarded. In the calculation, we excluded the datasets for three participants because of their head-movement displacements exceeding 2 mm and the rotation exceeding 2°. A total of 41 cSVD patients and 32 HCs were finally included in the following analysis. There were also no significant group differences in the head motion between the two groups.
We first performed a voxel-wise Fast Fourier Transform (FFT) method for each participant to convert the filtered time series into the frequency domain to obtain the power spectrum. Since the power within a given frequency band is proportional to the square of the magnitude of that frequency component, we calculated the square root of the power spectrum within each frequency band and then averaged these square roots across three frequency bands: 0.01–0.1 Hz (typical band), 0.027–0.073 Hz (slow-4), and 0.01–0.027 Hz (slow-5) at each voxel. This averaged square root was taken as ALFF (Zang et al., 2007), which was assumed to reflect the absolute intensity of spontaneous brain activity.
A χ2-test was used to test between-group differences in sex. A t-test was used to test between-group differences in age. The Wilcoxon test was used to test the education level between groups. The statistical significance level was set at p < 0.05. Statistical analysis was conducted using SPSS (version 21.0).
The between-group difference in ALFF was conducted by PALM that is implemented in the DPARSF toolbox.2 In the calculations. A general linear model (GLM) was applied, and sex and age factors were regressed. A two-tailed non-parametric permutation test (5,000 times) was conducted to determine the differences between the two groups. For the multiple-comparison correction, we used cluster-forming threshold and family-wise error (FWE) methods. The significance level was set at p < 0.05 (cluster-forming threshold >2.3, voxel-wise FWE <0.05).
A mixed effect analysis was performed on the two groups and their ALFF on the slow-5 and slow-4 band, using a two-way repeated measures analysis of variance (ANOVA) to investigate the effects of group and frequency band with age, sex, and head motions (mean FD) as covariates. Group (cSVD and HCs) was used as a between-subjects factor and frequency band (slow-4 and slow-5) as a repeated measurements factor. In addition, post hoc tests were performed between slow-5 band and slow-4 bands on the cSVD patients within brain regions showing group and frequency band interactions (Gu et al., 2019; Wang Z. et al., 2020). In addition, we also took a threshold of 50 voxels to remove small clusters, which meant only a cluster size >50 voxels were reported. For the multiple-comparison correction, we used threshold free cluster enhancement (TFCE) and family-wise error (FWE) methods. The significance level was set at p < 0.01 (voxel-wise FWE < 0.01). The imaging results after the multiple-comparison correction were reported by the AAL atlas (Rolls et al., 2020).
To determine whether the ALFF value in each region varied with clinical measures, we performed the correlation analyses between the ALFF values for the significantly changed ALFF and each clinical variable (i.e., VFT, DSST, and DST). The threshold was set at ptwo-tailed < 0.05.
Table 1 lists the demographic information for both the cSVD and healthy controls, and clinical information for the cSVD group. No significant difference was found in sex between the two groups. The age and education level of the cSVD group were significantly higher than those of the healthy controls (p < 0.05).
Figure 1 displays clusters with significant between-group differences in ALFF. Compared with the controls, the cSVD patients showed significantly reduced ALFF in one cluster (cluster size = 3,019 voxels). The locations of the cluster are listed in Table 2. Specifically, the cSVD patients had significantly lower ALFF than the controls in the left fusiform gyrus/hippocampus/insula/inferior occipital gyrus/middle occipital gyrus/inferior frontal gyrus-medial orbital/rolandic operculum and the bilateral cerebellum.
Figure 1. The brain regions show a significant difference in the amplitude of low-frequency fluctuation (ALFF) between the cSVD patients and the healthy controls in the typical frequency band (p < 0.05, cluster-forming threshold and FWE corrected). We found that the cSVD showed lower ALFF values in the one cluster in the left cerebellum, left hippocampus/parahippocampal, and left inferior occipital gyrus (IOG) of the controls.
Table 2. Brain areas showing significant differences in ALFF values under the typical (0.01–0.1 Hz) and slow-4 bands (0.027–0.073 Hz) between cSVD patients and HCs.
Figure 2 shows the clusters with a significant difference in ALFF between the cSVD and controls for slow-4. For the slow-4, we found that the cSVD patients had significantly reduced ALFF in one cluster (voxel size = 1,383 voxels) in the left hemisphere, including the left cerebellum/hippocampus/fusiform gyrus/inferior occipital gyrus. As for the slow-5, no clusters showed a significant between-group difference in ALFF. The detailed information for the clusters is also listed in Table 2.
Figure 2. The brain regions showed a significant ALFF difference in the slow-4 band between the cSVD patients and the HCs (p < 0.05, cluster-forming threshold and FWE corrected). Hot (cold) colors represent higher (lower) ALFF in the HCs than in the cSVD patients.
We observed a significant interaction between the frequency band and group in the left calcarine, bilateral lingual, left cerebellum, bilateral precuneus, left cuneus, and right superior parietal gyrus (Table 3). Further post hoc tests showed significantly decreased ALFF values were identified in the left precuneus in the slow-4 band (Figures 3, 4).
Figure 3. The main effect for frequency band on ALFF. The color bar on the right indicates the statistical t-value. Blue cold colors represent lower ALFF. The results were obtained by two-way repeated-measures ANOVA (TFCE and FWE corrected, voxel p < 0.01). TFCE, Threshold Free Cluster Enhancement.
Figure 4. The interaction between frequency band and group on ALFF for cSVD patients. The results were obtained by a two-way repeated-measures ANOVA (p < 0.01, TFCE and FWE corrected) (A); and then a post-hoc pair t-test shown in the (B) (p < 0.025, TFCE and FWE corrected), compared to the slow-5 band, cold overlays indicate lower ALFF in cSVD patients in the slow-4 band. TFCE, Threshold Free Cluster Enhancement.
For each of the significant clusters, no significant correlation was found between ALFF values and any neuropsychological scales (p > 0.05).
This study identified the specific alteration pattern of ALFF in cSVD patients under different frequency bands through a voxel-based whole-brain analysis. We found that the cSVD patients showed significantly lower ALFF in the cerebellum, hippocampus, and occipital cortex than the controls in the typical band and the slow-4 band. Additionally, post hoc analysis indicated that cSVD was associated with a wide range of abnormalities in brain activity with related frequency bands.
We found that cSVD patients showed reduced ALFF in the cerebellum compared with the controls (Figure 1). Although previous studies had noted that the cerebellum is widely believed to be responsible for motor skills (Baune et al., 2018), coordination, and balance of visual-motor (Garfinkle et al., 2020), several studies (Koziol et al., 2014; Thomas et al., 2017; Seese, 2020) indicated that it is also involved in cognitive function and executive function. The decrease of microstructural integrity in the cerebellum of deep white matter hyperintensities patients was associated with dual-task gait speed. Dual-task gait speed was associated with three cognitive domains (global cognition, attention/processing speed, and executive function) (Ghanavati et al., 2018). Schaefer et al. (2014) applied rs-fMRI analysis methods (eigenvector centrality) in cSVD patients and showed reduced connectivity in frontoparietal networks, whereas connectivity increases in the cerebellum. Another important finding is a positive correlation between reaction time in the incongruent condition of the Stroop task with the eigenvector centralities of the cerebellar region. The functional connectome in cerebellar regions was increased while the functional connectome in frontoparietal cognitive networks was decreased; therefore, the author speculated that frontoparietal hypoconnectivity might be compensated by hyper-connectivity. For example, Li et al. (2018) found lower grey matter volume in the cerebellar in patients with early-onset AD than in healthy controls. Several studies (Li et al., 2013; Mascalchi et al., 2014) also found that MCI patients had reduced WM integrity. These studies of AD or MCI provided insight into MCI patients with brain structural or functional impairment in the cerebellum. Previous studies (Castellazzi et al., 2014) in MCI patients also reported that the FC of the cerebellum is lower than that in healthy controls and the cerebellar FC correlates positively with semantic fluency. Thus, we infer that the reduction of spontaneous activity in the cerebellum may be related to the cognitive dysfunction of cSVD patients.
This study also found aberrant spontaneous brain activity in the occipital cortex (Figure 1). In the pathological anatomy of cSVD, researchers found that cortical microinfarcts were presented more frequently in the parietal and occipital lobes, which were also related to the severity of cSVD (Ii et al., 2021). A comparison of our findings with those of other studies (De Marco et al., 2017; Marjańska et al., 2019) confirms dysfunction of the occipital lobe during AD and MCI. Gray matter volume of different brain regions was associated with cognitive ability in cSVD patients, and gray matter volume in the occipital thalamus was positively correlated with Montreal Cognitive Assessment scores (Wang Y. et al., 2020). Lambert et al. (2016) did longitudinal research into symptomatic cSVD to investigate. They showed that the rate of white matter hyperintensity progression is associated with increases in cortical gray matter atrophy rates in occipital lobes. Chen et al. (2020) found that the right inferior longitudinal fasciculus had a significantly negative correlation with total cognitive and episodic memory. It could be that the cSVD patients showed a relative decline of spontaneous brain activity in the occipital cortex, which might be part of the underlying brain function mechanism of cognitive dysfunction.
In addition, the current study also found abnormal spontaneous brain activity in the hippocampus of cSVD patients, suggesting that the occurrence of cognitive decline in cSVD may relate to the decreased activity in the hippocampus. The hippocampus is closely related to memory function and spatial navigational ability and plays a crucial role in all forms of declarative memory (including recognition) (Wixted and Squire, 2011). Jokinen et al. (2020) studied the relationship between grey matter volume and clinical symptoms of cSVD. They found a positive correlation between hippocampal volume and Vascular Dementia Assessment Scale–Cognitive Subscale, executive function, and memory. A study evaluated the influence of negative and positive cSVD-hippocampal subfield atrophy on episodic memory and frontal executive function (Wong et al., 2021). Hippocampal subfield atrophy worsened with increasing SVD severity, and atrophy in the subiculum, CA1, CA4, molecular layer, and dentate gyrus of the hippocampal were essential to poor episodic memory and frontal executive function. Xi et al. (2012) found that the low-frequency oscillation amplitudes of the right hippocampus, parahippocampal gyrus, left middle temporal gyrus and right ventromedial prefrontal cortex of MCI patients were reduced. The ALFF values of the hippocampus and parahippocampal gyrus had a positive correlation with the neuropsychological scale score. The hippocampal ALFF value of cSVD patients is abnormally decreased, and it is positively correlated with the MoCA score in our research. Many studies have affirmed the role of the hippocampus in the field of cognitive function, which may be a critical factor in the cognitive dysfunction of cSVD patients.
Different neurophysiological mechanisms have different physiological functions, including different oscillatory bands (Buzsáki and Draguhn, 2004). Previous studies had explored the differences in ALFF between brain areas in the slow-4 and slow-5 bands (Xiao et al., 2018; Wang Z. et al., 2020). Neural oscillations of different frequencies in the human brain may be sensitive to activities in different regions and can reflect different physiological functions of brain activities (Knyazev, 2007). Low-frequency oscillations are related to long-distance connections and the integration of large neuronal systems. In contrast, the high-frequency oscillations synchronization constitutes a functionally important neuronal peak time relationship in brain activity (Arnulfo et al., 2020). Connectivity between distal cortical regions is a valuable but expensive feature of cortical tissue and exists mainly between heterotypic (Oligschläger et al., 2017). It is generally believed that the slow-5 band is the main contributor of resting-state fluctuations in healthy humans (Zuo et al., 2010), and is more sensitive in the cortex (such as the temporal and Rolandic lobes). Our results are dissimilar to previous rs-fMRI studies in AD, showing more varied areas in the slow-5 band than the slow-4 band (Yang et al., 2020). Liu et al. (2014) also found that several temporal regions were more significant in the slow-5 band than the slow-4 band in AD patients. Our results are similar to previous studies in patients with cognitive impairment, which suggested that most cortical areas had more robust low-frequency oscillations and higher functional activities in the slow-5 band.
We observed a significant group × frequency interaction effect on the ALFF value in the left precuneus. These findings showed that the ALFF changes in CSVD patients with cognitive dysfunction are related to frequency bands, and the neurophysiological mechanism is unclear. Thus, we conclude that certain pathologic conditions in the precuneus may increase the influence of specific frequency bands. However, studies have found that precuneus plays a central role in many highly integrated tasks, including visual space imagery, situational memory extraction, and self-processing operations (Cavanna and Trimble, 2006). Hebscher et al. (2020) have also confirmed that the precuneus was closely related to human autobiographical memory. Luo et al. (2020) studied the functional connection between the precuneus and the cortical using resting-state fMRI and found that the human precuneus can be subdivided into six symmetrical and connected parcels. The precuneus has four sub-regions and acts as a mediator in the interaction of the default mode, multi-faceted attention, and frontoparietal control network. Precuneus and posterior cingulate cortex together belong to the posterior default mode network (DMN). The DMN is a specific neuronal circuitry to global brain function. Damage to the DMN often occurs in diseases such as Alzheimer’s disease, Parkinson’s disease, Epilepsy and attention deficit hyperactivity disorder (ADHD) (Mohan et al., 2016). A meta-analysis compared amnestic mild cognitive impairment patients (aMCI) with healthy control suggested that aMCI is associated with widespread aberrant regional spontaneous brain activity, predominantly involving the default mode, salience, and visual networks. In contrast, the increased severity of cognitive impairment in aMCI patients was associated with more significant decreases in ALFFs in the cuneus/precuneus cortices (Pan et al., 2017).
These findings indicated that frequency-specific ALFF could supplement important information for disease diagnosis as well as supply a new perspective for our understanding of the pathology of CSVD.
This study has several limitations. First, the number of samples is not large enough, and age differences across cSVD patients or between patients and healthy control groups were not well addressed. Second, one of limitations of the present study is the 8-channel phased-array head coil employed for data acquisition, which represents the minimal number of channels. Using an advanced scanning sequence may need to be considered in future studies, which may potentially enhance qualities of scanned images. Third, we did not classify the sub-type of cSVD although there are many types of imaging performance and various pathophysiology of cSVD. Future study needs to recruit more patients and distinguish between different imaging findings to evaluate the degree of impairment of brain function in different types to guide the treatment. Fourth, the current study used the rs-fMRI only. It would be better to use multi-modal neuroimaging data, such as arterial spin labeling (ASL) and diffusion tensor imaging (DTI). The combination of multi-modal neuroimaging and neuropsychological scales might help us understand cognitive dysfunction progression in cSVD more thoroughly. Finally, the healthy controls included in our study were different in age from the CSVD group. Because age is a high-risk factor for the development of cerebral small vessel disease, we had great difficulty in finding age-matched healthy controls.
This study analyzed spontaneous brain activity in cSVD patients and showed abnormal ALFF in distinct brain regions in patients compared with controls. These abnormal brain regions were found in the cerebellum, occipital, and hippocampus in cSVD patients. These findings could reflect the pathogenesis of specific clinical manifestations of cSVD, especially cognitive decline.
The original contributions presented in the study are included in the article/supplementary materials, further inquiries can be directed to the corresponding authors.
The studies involving humans were approved by the Institutional Review Board of Zhongshan TCM Hospital (ClinicalTrials.gov identifier: 2016ZSZY-LLK-028). The studies were conducted in accordance with the local legislation and institutional requirements. The participants provided their written informed consent to participate in this study.
SC: Data curation, Writing – original draft. RH: Methodology, Writing – original draft. MZ: Writing – original draft. XH: Writing – review & editing. SLing: Writing – review & editing. SLiu: Resources, Writing – review & editing. NY: Methodology, Writing – review & editing.
The author(s) declare that no financial support was received for the research, authorship, and/or publication of this article.
This study was approved by the Institutional Review Board of Zhongshan TCM Hospital (ClinicalTrials.gov identifier: 2016ZSZY-LLK-028).
The authors declare that the research was conducted in the absence of any commercial or financial relationships that could be construed as a potential conflict of interest.
All claims expressed in this article are solely those of the authors and do not necessarily represent those of their affiliated organizations, or those of the publisher, the editors and the reviewers. Any product that may be evaluated in this article, or claim that may be made by its manufacturer, is not guaranteed or endorsed by the publisher.
cSVD, Cerebral small vessel disease; AD, Alzheimer’s disease; MCI, Mild cognitive impairment; ALFF, Amplitude of low-frequency fluctuation; ReHo, Regional homogeneity; WMH, White matter hyperintensity; LA, Lacune; CMB, Cerebral microbleed; VFT, Verbal fluency test; DST, Digit span test; DSST, Digit symbol substitution test.
Arnulfo, G., Wang, S. H., Myrov, V., Toselli, B., Hirvonen, J., Fato, M. M., et al. (2020). Long-range phase synchronization of high-frequency oscillations in human cortex. Nat. Commun. 11:5363. doi: 10.1038/s41467-020-18975-8
Baune, B. T., Brignone, M., and Larsen, K. G. (2018). A network meta-analysis comparing effects of various antidepressant classes on the digit symbol substitution test (DSST) as a measure of cognitive dysfunction in patients with major depressive disorder. Int. J. Neuropsychopharmacol. 21, 97–107. doi: 10.1093/ijnp/pyx070
Buzsáki, G., and Draguhn, A. (2004). Neuronal oscillations in cortical networks. Science 304, 1926–1929. doi: 10.1126/science.1099745
Cai, Z., Wang, C., He, W., Tu, H., Tang, Z., Xiao, M., et al. (2015). Cerebral small vessel disease and Alzheimer’s disease. Clin. Interv. Aging 10, 1695–1704. doi: 10.2147/CIA.S90871
Castellazzi, G., Palesi, F., Casali, S., Vitali, P., Sinforiani, E., Wheeler-Kingshott, C. A., et al. (2014). A comprehensive assessment of resting state networks: bidirectional modification of functional integrity in cerebro-cerebellar networks in dementia. Front. Neurosci. 8:223. doi: 10.3389/fnins.2014.00223
Cavanna, A. E., and Trimble, M. R. (2006). The precuneus: a review of its functional anatomy and behavioural correlates. Brain 129, 564–583. doi: 10.1093/brain/awl004
Chen, H. F., Huang, L. L., Li, H. Y., Qian, Y., Yang, D., Qing, Z., et al. (2020). Microstructural disruption of the right inferior fronto-occipital and inferior longitudinal fasciculus contributes to WMH-related cognitive impairment. CNS Neurosci. Ther. 26, 576–588. doi: 10.1111/cns.13283
Chen, X., Wang, J., Shan, Y., Cai, W., Liu, S., Hu, M., et al. (2019). Cerebral small vessel disease: neuroimaging markers and clinical implication. J. Neurol. 266, 2347–2362. doi: 10.1007/s00415-018-9077-3
De Guio, F., Jouvent, E., Biessels, G. J., Black, S. E., Brayne, C., Chen, C., et al. (2016). Reproducibility and variability of quantitative magnetic resonance imaging markers in cerebral small vessel disease. J. Cereb. Blood Flow Metab. 36, 1319–1337. doi: 10.1177/0271678X16647396
De Marco, M., Duzzi, D., Meneghello, F., and Venneri, A. (2017). Cognitive efficiency in Alzheimer’s disease is associated with increased occipital connectivity. J. Alzheimers Dis. 57, 541–556. doi: 10.3233/jad-161164
Dey, A. K., Stamenova, V., Turner, G., Black, S. E., and Levine, B. (2016). Pathoconnectomics of cognitive impairment in small vessel disease: a systematic review. Alzheimers Dement. 12, 831–845. doi: 10.1016/j.jalz.2016.01.007
Feng, M., Wen, H., Xin, H., Zhang, N., Liang, C., and Guo, L. (2021). Altered spontaneous brain activity related to neurologic dysfunction in patients with cerebral small vessel disease. Front. Aging Neurosci. 13:731585. doi: 10.3389/fnagi.2021.731585
Garfinkle, J., Guo, T., Synnes, A., Chau, V., Branson, H. M., Ufkes, S., et al. (2020). Location and size of preterm cerebellar hemorrhage and childhood development. Ann. Neurol. 88, 1095–1108. doi: 10.1002/ana.25899
Ghanavati, T., Smitt, M. S., Lord, S. R., Sachdev, P., Wen, W., Kochan, N. A., et al. (2018). Deep white matter hyperintensities, microstructural integrity and dual task walking in older people. Brain Imaging Behav. 12, 1488–1496. doi: 10.1007/s11682-017-9787-7
Gu, L., Hong, S., Jiang, J., Liu, J., Cao, X., Huang, Q., et al. (2019). Bidirectional alterations in ALFF across slow-5 and slow-4 frequencies in the brains of postherpetic neuralgia patients. J. Pain Res. 12, 39–47. doi: 10.2147/jpr.S179077
Hebscher, M., Ibrahim, C., and Gilboa, A. (2020). Precuneus stimulation alters the neural dynamics of autobiographical memory retrieval. NeuroImage 210:116575. doi: 10.1016/j.neuroimage.2020.116575
Ii, Y., Ishikawa, H., Shindo, A., Matsuyama, H., Matsuura, K., Matsuda, K., et al. (2021). Association between cortical microinfarcts and total small vessel disease burden in cerebral amyloid angiopathy on 3-tesla magnetic resonance imaging. Eur. J. Neurol. 28, 794–799. doi: 10.1111/ene.14610
Jokinen, H., Koikkalainen, J., Laakso, H. M., Melkas, S., Nieminen, T., Brander, A., et al. (2020). Global burden of small vessel disease-related brain changes on MRI predicts cognitive and functional decline. Stroke 51, 170–178. doi: 10.1161/strokeaha.119.026170
Kim, H. W., Hong, J., and Jeon, J. C. (2020). Cerebral small vessel disease and Alzheimer’s disease: A Review. Review 11:927. doi: 10.3389/fneur.2020.00927
Knyazev, G. G. (2007). Motivation, emotion, and their inhibitory control mirrored in brain oscillations. Neurosci. Biobehav. Rev. 31, 377–395. doi: 10.1016/j.neubiorev.2006.10.004
Koziol, L. F., Budding, D., Andreasen, N., D’Arrigo, S., Bulgheroni, S., Imamizu, H., et al. (2014). Consensus paper: the cerebellum’s role in movement and cognition. Cerebellum 13, 151–177. doi: 10.1007/s12311-013-0511-x
Lambert, C., Benjamin, P., Zeestraten, E., Lawrence, A. J., Barrick, T. R., and Markus, H. S. (2016). Longitudinal patterns of leukoaraiosis and brain atrophy in symptomatic small vessel disease. Brain 139, 1136–1151. doi: 10.1093/brain/aww009
Leung, J. L., Lee, G. T., Lam, Y. H., Chan, R. C., and Wu, J. Y. (2011). The use of the digit span test in screening for cognitive impairment in acute medical inpatients. Int. Psychogeriatr. 23, 1569–1574. doi: 10.1017/s1041610211000792
Li, H., Jia, X., Li, Y., Jia, X., and Yang, Q. (2021). Aberrant amplitude of Low-frequency fluctuation and degree centrality within the default mode network in patients with vascular mild cognitive impairment. Brain Sci. 11:1534. doi: 10.3390/brainsci11111534
Li, H., Liang, Y., Chen, K., Li, X., Shu, N., Zhang, Z., et al. (2013). Different patterns of white matter disruption among amnestic mild cognitive impairment subtypes: relationship with neuropsychological performance. J. Alzheimers Dis. 36, 365–376. doi: 10.3233/jad-122023
Li, K. C., Luo, X., Zeng, Q. Z., Xu, X. J., Huang, P. Y., Shen, Z. J., et al. (2018). Distinct patterns of interhemispheric connectivity in patients with early- and late-onset Alzheimer’s disease. Front. Aging Neurosci. 10:261. doi: 10.3389/fnagi.2018.00261
Liao, H., Yi, J., Cai, S., Shen, Q., Liu, Q., Zhang, L., et al. (2021). Changes in degree centrality of network nodes in different frequency bands in Parkinson’s disease with depression and without depression. Front. Neurosci. 15:638554. doi: 10.3389/fnins.2021.638554
Liu, Y., Braidy, N., Poljak, A., Chan, D. K. Y., and Sachdev, P. (2018). Cerebral small vessel disease and the risk of Alzheimer’s disease: a systematic review. Ageing Res. Rev. 47, 41–48. doi: 10.1016/j.arr.2018.06.002
Liu, X., Wang, S., Zhang, X., Wang, Z., Tian, X., and He, Y. (2014). Abnormal amplitude of low-frequency fluctuations of intrinsic brain activity in Alzheimer’s disease. J. Alzheimers Dis. 40, 387–397. doi: 10.3233/jad-131322
Luo, Z., Zeng, L. L., Qin, J., Hou, C., Shen, H., and Hu, D. (2020). Functional Parcellation of human brain Precuneus using density-based clustering. Cereb. Cortex 30, 269–282. doi: 10.1093/cercor/bhz086
Marjańska, M., McCarten, J. R., Hodges, J. S., Hemmy, L. S., and Terpstra, M. (2019). Distinctive neurochemistry in Alzheimer’s disease via 7 T in vivo magnetic resonance spectroscopy. J. Alzheimers Dis. 68, 559–569. doi: 10.3233/jad-180861
Mascalchi, M., Ginestroni, A., Toschi, N., Poggesi, A., Cecchi, P., Salvadori, E., et al. (2014). The burden of microstructural damage modulates cortical activation in elderly subjects with MCI and leuko-araiosis. A DTI and fMRI study. Hum. Brain Mapp. 35, 819–830. doi: 10.1002/hbm.22216
Mo, Y., Mao, C., Yang, D., Ke, Z., Huang, L., Yang, Z., et al. (2023). Altered neuroimaging patterns of cerebellum and cognition underlying the gait and balance dysfunction in cerebral small vessel disease. Front. Aging Neurosci. 15:1117973. doi: 10.3389/fnagi.2023.1117973
Mohan, A., Roberto, A. J., Mohan, A., Lorenzo, A., Jones, K., Carney, M. J., et al. (2016). The significance of the default mode network (DMN) in neurological and neuropsychiatric disorders: a review. Yale J. Biol. Med. 89, 49–57.
Oligschläger, S., Huntenburg, J. M., Golchert, J., Lauckner, M. E., Bonnen, T., and Margulies, D. S. (2017). Gradients of connectivity distance are anchored in primary cortex. Brain Struct. Funct. 222, 2173–2182. doi: 10.1007/s00429-016-1333-7
Pan, P., Zhu, L., Yu, T., Shi, H., Zhang, B., Qin, R., et al. (2017). Aberrant spontaneous low-frequency brain activity in amnestic mild cognitive impairment: a meta-analysis of resting-state fMRI studies. Ageing Res. Rev. 35, 12–21. doi: 10.1016/j.arr.2016.12.001
Pantoni, L. (2010). Cerebral small vessel disease: from pathogenesis and clinical characteristics to therapeutic challenges. Lancet Neurol. 9, 689–701. doi: 10.1016/s1474-4422(10)70104-6
Qi, R., Luo, Y., Zhang, L., Weng, Y., Surento, W., Jahanshad, N., et al. (2020). FKBP5 haplotypes and PTSD modulate the resting-state brain activity in Han Chinese adults who lost their only child. Transl. Psychiatry 10:91. doi: 10.1038/s41398-020-0770-5
Ren, F., Ma, W., Zong, W., Li, N., Li, X., Li, F., et al. (2021). Brain frequency-specific changes in the spontaneous neural activity are associated with cognitive impairment in patients with Presbycusis. Front. Aging Neurosci. 13:649874. doi: 10.3389/fnagi.2021.649874
Rolls, E. T., Huang, C.-C., Lin, C.-P., Feng, J., and Joliot, M. (2020). Automated anatomical labelling atlas 3. NeuroImage 206:116189. doi: 10.1016/j.neuroimage.2019.116189
Sasai, S., Koike, T., Sugawara, S. K., Hamano, Y. H., Sumiya, M., Okazaki, S., et al. (2021). Frequency-specific task modulation of human brain functional networks: a fast fMRI study. NeuroImage 224:117375. doi: 10.1016/j.neuroimage.2020.117375
Schaefer, A., Quinque, E. M., Kipping, J. A., Arélin, K., Roggenhofer, E., Frisch, S., et al. (2014). Early small vessel disease affects frontoparietal and cerebellar hubs in close correlation with clinical symptoms--a resting-state fMRI study. J. Cereb. Blood Flow Metab. 34, 1091–1095. doi: 10.1038/jcbfm.2014.70
Seese, R. R. (2020). Working memory impairments in cerebellar disorders of childhood. Pediatr. Neurol. 107, 16–23. doi: 10.1016/j.pediatrneurol.2020.02.005
Staals, J., Booth, T., Morris, Z., Bastin, M. E., Gow, A. J., Corley, J., et al. (2015). Total MRI load of cerebral small vessel disease and cognitive ability in older people. Neurobiol. Aging 36, 2806–2811. doi: 10.1016/j.neurobiolaging.2015.06.024
Thomas, A. R., Lacadie, C., Vohr, B., Ment, L. R., and Scheinost, D. (2017). Fine motor skill mediates visual memory ability with microstructural neuro-correlates in cerebellar peduncles in prematurely born adolescents. Cereb. Cortex 27, 322–329. doi: 10.1093/cercor/bhw415
Vaucheret Paz, E., Puga, C., Ekonen, C., Pintos, P., Lascombes, I., De Vita, S., et al. (2020). Verbal fluency test in children with neurodevelopmental disorders. J. Neurosci. Rural Pract. 11, 95–99. doi: 10.1055/s-0039-3400347
Wang, L., Feng, Q., Wang, M., Zhu, T., Yu, E., Niu, J., et al. (2021). An effective brain imaging biomarker for AD and aMCI: ALFF in Slow-5 frequency band. Curr. Alzheimer Res. 18, 45–55. doi: 10.2174/1567205018666210324130502
Wang, Z., Liu, Y., Ruan, X., Li, Y., Li, E., Zhang, G., et al. (2020). Aberrant amplitude of Low-frequency fluctuations in different frequency bands in patients with Parkinson’s disease. Front. Aging Neurosci. 12:576682. doi: 10.3389/fnagi.2020.576682
Wang, Y., Yang, Y., Wang, T., Nie, S., Yin, H., and Liu, J. (2020). Correlation between white matter Hyperintensities related gray matter volume and cognition in cerebral small vessel disease. J. Stroke Cerebrovasc. Dis. 29:105275. doi: 10.1016/j.jstrokecerebrovasdis.2020.105275
Wardlaw, J. M., Smith, E. E., Biessels, G. J., Cordonnier, C., Fazekas, F., Frayne, R., et al. (2013). Neuroimaging standards for research into small vessel disease and its contribution to ageing and neurodegeneration. Lancet Neurol. 12, 822–838. doi: 10.1016/S1474-4422(13)70124-8
Wixted, J. T., and Squire, L. R. (2011). The medial temporal lobe and the attributes of memory. Trends Cogn. Sci. 15, 210–217. doi: 10.1016/j.tics.2011.03.005
Wong, F. C. C., Yatawara, C., Low, A., Foo, H., Wong, B. Y. X., Lim, L., et al. (2021). Cerebral small vessel disease influences hippocampal subfield atrophy in mild cognitive impairment. Transl. Stroke Res. 12, 284–292. doi: 10.1007/s12975-020-00847-4
Wu, Z., Luo, Q., Wu, H., Wu, Z., Zheng, Y., Yang, Y., et al. (2020). Amplitude of Low-frequency oscillations in major depressive disorder with childhood trauma. Front. Psych. 11:596337. doi: 10.3389/fpsyt.2020.596337
Xi, Q., Zhao, X., Wang, P., Guo, Q., Jiang, H., Cao, X., et al. (2012). Spontaneous brain activity in mild cognitive impairment revealed by amplitude of low-frequency fluctuation analysis: a resting-state fMRI study. Radiol. Med. 117, 865–871. doi: 10.1007/s11547-011-0780-8
Xiao, F., Wang, T., Gao, L., Fang, J., Sun, Z., Xu, H., et al. (2018). Frequency-dependent changes of the resting BOLD signals predicts cognitive deficits in asymptomatic carotid artery stenosis. Front. Neurosci. 12:416. doi: 10.3389/fnins.2018.00416
Yang, L., Yan, Y., Li, Y., Hu, X., Lu, J., Chan, P., et al. (2020). Frequency-dependent changes in fractional amplitude of low-frequency oscillations in Alzheimer’s disease: a resting-state fMRI study. Brain Imaging Behav. 14, 2187–2201. doi: 10.1007/s11682-019-00169-6
Zang, Y. F., He, Y., Zhu, C. Z., Cao, Q. J., Sui, M. Q., Liang, M., et al. (2007). Altered baseline brain activity in children with ADHD revealed by resting-state functional MRI. Brain Dev. 29, 83–91. doi: 10.1016/j.braindev.2006.07.002
Zhou, X., Zhang, C., Li, L., Zhang, Y., Zhang, W., Yin, W., et al. (2020). Altered brain function in cerebral small vessel disease patients with gait disorders: a resting-state functional MRI study. Front. Aging Neurosci. 12:234. doi: 10.3389/fnagi.2020.00234
Zou, Q.-H., Zhu, C.-Z., Yang, Y., Zuo, X.-N., Long, X.-Y., Cao, Q.-J., et al. (2008). An improved approach to detection of amplitude of low-frequency fluctuation (ALFF) for resting-state fMRI: fractional ALFF. J. Neurosci. Methods 172, 137–141. doi: 10.1016/j.jneumeth.2008.04.012
Keywords: spontaneous brain activity, amplitude of low-frequency fluctuation, cerebral small vessel disease, mild cognitive impairment, white matter hyperintensity, lacune, cerebral microbleed
Citation: Chen S, Huang R, Zhang M, Huang X, Ling S, Liu S and Yang N (2023) Altered brain spontaneous activity in patients with cerebral small vessel disease using the amplitude of low-frequency fluctuation of different frequency bands. Front. Neurosci. 17:1282496. doi: 10.3389/fnins.2023.1282496
Received: 24 August 2023; Accepted: 16 October 2023;
Published: 16 November 2023.
Edited by:
Feng Zhang, Third Hospital of Hebei Medical University, ChinaReviewed by:
Daniel Tozer, University of Cambridge, United KingdomCopyright © 2023 Chen, Huang, Zhang, Huang, Ling, Liu and Yang. This is an open-access article distributed under the terms of the Creative Commons Attribution License (CC BY). The use, distribution or reproduction in other forums is permitted, provided the original author(s) and the copyright owner(s) are credited and that the original publication in this journal is cited, in accordance with accepted academic practice. No use, distribution or reproduction is permitted which does not comply with these terms.
*Correspondence: Nan Yang, c3JzeWFuZ0AxMjYuY29t; Shuxue Liu, bGl1c18yQDEyNi5jb20=
Disclaimer: All claims expressed in this article are solely those of the authors and do not necessarily represent those of their affiliated organizations, or those of the publisher, the editors and the reviewers. Any product that may be evaluated in this article or claim that may be made by its manufacturer is not guaranteed or endorsed by the publisher.
Research integrity at Frontiers
Learn more about the work of our research integrity team to safeguard the quality of each article we publish.