- 1Department of Neurology, Behavioral Neurology Section, University of Colorado School of Medicine, Aurora, CO, United States
- 2University of Colorado Alzheimer’s and Cognition Center, Aurora, CO, United States
- 3University of Colorado Movement Disorders Center, Aurora, CO, United States
- 4Department of Psychology, University of Houston, Houston, TX, United States
- 5Department of Neurology, Movement Disorders Section, University of Colorado School of Medicine, Aurora, CO, United States
Introduction: Cognitive decline is common in Parkinson’s disease (PD). Calculating personalized risk of cognitive decline in PD would allow for appropriate counseling, early intervention with available treatments, and inclusion in disease-modifying trials.
Methods: Data were from the Parkinson’s Progression Markers Initiative de novo cohort. Baseline scores were calculated for Lifestyle for Brain Health (LIBRA) and the Montreal Parkinson Risk of Dementia Scale (MoPaRDS) per prior literature and preliminary Parkinson’s disease Risk Estimator for Decline In Cognition Tool (pPREDICT) by attributing a point for fourteen posited risk factors. Baseline and 5-year follow-up composite cognitive scores (CCSs) were calculated from a neuropsychological battery and used to define cognitive decliners (PD-decline) versus maintainers (PD-maintain).
Results: The PD-decline group (n = 44) had higher LIBRA (6.76 ± 0.57, p < 0.05), MoPaRDS (2.45 ± 1.41, p < 0.05) and pPREDICT (4.52 ± 1.66, p < 0.05) scores compared to the PD-maintain group (n = 263; LIBRA 4.98 ± 0.20, MoPaRDS 1.68 ± 1.16, pPREDICT 3.38 ± 1.69). Area-under-the-curve (AUC) for LIBRA was 0.64 (95% confidence interval [CI], 0.55–0.73), MoPaRDS was 0.66 (95% CI, 0.58–0.75) and for pPREDICT was 0.68 (95% CI, 0.61–0.76). In linear regression analyses, LIBRA (p < 0.05), MoPaRDS (p < 0.05) and pPREDICT (p < 0.05) predicted change in CCS. Only age stratified by sex (p < 0.05) contributed significantly to the model for LIBRA. Age and presence of hallucinations (p < 0.05) contributed significantly to the model for MoPaRDS. Male sex, older age, excessive daytime sleepiness, and moderate–severe motor symptoms (all p < 0.05) contributed significantly to the model for pPREDICT.
Conclusion: Although MoPaRDS is a PD-specific tool for predicting cognitive decline relying on only clinical features, it does not focus on potentially modifiable risk factors. LIBRA does focus on potentially modifiable risk factors and is associated with prediction of all-cause dementia in some populations, but pPREDICT potentially demonstrates improved performance in cognitive decline risk calculation in individuals with PD and may identify actionable risk factors. As pPREDICT incorporates multiple potentially modifiable risk factors that can be obtained easily in the clinical setting, it is a first step in developing an easily assessable tool for a personalized approach to reduce dementia risk in people with PD.
1. Introduction
About 10–20% of individuals newly diagnosed with Parkinson’s disease (PD) have cognitive changes (Aarsland et al., 2009; Weintraub et al., 2015) and cognitive decline is associated with high rates of conversion to dementia (Aarsland et al., 2003). Multiple tools have been developed to predict risk of cognitive decline in PD (Schrag et al., 2017; Dawson et al., 2018; Hogue et al., 2018; Gramotnev et al., 2019; Yousaf et al., 2019; Wilson et al., 2020), however these tools rely on testing not routinely performed outside of tertiary care or research settings (e.g., dopamine transporter (DaT) imaging (Schrag et al., 2017; Yousaf et al., 2019), formal olfactory testing (Schrag et al., 2017), cerebral spinal fluid (CSF) studies (Schrag et al., 2017; Gramotnev et al., 2019; Yousaf et al., 2019), and genetic testing (Gramotnev et al., 2019). There are tools using specific neuropsychological assessments (Hogue et al., 2018; Wilson et al., 2020); however, the selection of formal neuropsychological tests is not standardized nor are all formal neuropsychological tests available in all clinical settings. The Montreal Parkinson Risk of Dementia Scale (MoPaRDS) is an office-based tool for estimating risk of developing dementia in PD and therefore holds promise for scalability to a wider PD population (Dawson et al., 2018); however, MoPaRDS does not focus on potentially modifiable risk factors allowing for actionable lifestyle or treatment changes based on personalized risk assessment.
Indeed, although previous PD-specific predictor tools, including the MoPaRDS, provide information about the risk of cognitive decline (Schrag et al., 2017; Dawson et al., 2018; Hogue et al., 2018; Gramotnev et al., 2019; Yousaf et al., 2019; Wilson et al., 2020), none focus on modifiable risk factors. To our knowledge, there is currently no PD-specific predictor tool for cognitive decline that focuses on risk factors that have potential interventions for immediate action with lifestyle modifications or available symptom-based treatments. The Lifestyle for Brain Health (LIBRA) score focuses on modifiable, lifestyle-related risk factors associated with all-cause dementia identified through systematic literature review and Delphi consensus (Deckers et al., 2015; Vos et al., 2017; Schiepers et al., 2018). Components of the full LIBRA score can be easily assessed in routine clinical settings and include demographics and modifiable risk factors (Table 1; Deckers et al., 2015). To our knowledge, LIBRA has not been validated to assess risk of cognitive decline specifically in a population of individuals with PD.
The goal of this study was to explore development of a pragmatic tool to estimate personal risk of cognitive decline specifically for people with PD while focusing on modifiable factors. LIBRA was used as a starting point given its utility in all-cause dementia prediction, but additional risk factors easily obtained in routine clinical assessment without expensive or invasive testing or procedures were identified to explore a PD-specific tool. MoPaRDS was used as a PD-specific comparator tool given its utility in the clinical setting even outside of tertiary/quaternary care centers or in the research setting. The fourteen putative, dichotomous risk factors for the preliminary Parkinson’s disease Risk Estimator for Decline In Cognition Tool (pPREDICT) included demographic, clinical, PD-specific, and potentially reversible and/or treatable risk factors based on literature review (Table 1; Riggeal et al., 2007; Pilotto et al., 2016; Gottesman et al., 2017; Chahine et al., 2019; Kim et al., 2019; Paul et al., 2019; Baiano et al., 2020; Ben-Joseph et al., 2020; Reekes et al., 2020; Forbes et al., 2021; Guo et al., 2021; Nicoletti et al., 2021; Xie et al., 2021). There are no disease-modifying therapeutics to slow the cognitive decline associated with PD, therefore focusing on potentially reversible and/or treatable risks is of particular importance. Additionally, focusing on easily obtained clinical markers allows for greater accessibility to the wider PD population, which is of upmost importance given that many (40%) individuals with PD do not see a neurologist every year and even fewer (1.8%) are treated by movement disorder specialists (Pearson et al., 2023). A predictor tool focusing on lifestyle-related and/or treatable PD-specific risk factors opens the door for individualized treatment plans to slow or prevent cognitive decline in PD.
2. Methods
2.1. Study population and data handling
Data were obtained from the Parkinson’s Progression Markers Initiative (PPMI) database.1 For up-to-date information on the study, visit www.ppmi-info.org. Data were downloaded on September 2, 2019 with updated neuropsychological data downloaded June 7, 2021. The study was approved by the Colorado Multiple Institutional Review Board (exempt protocol #20–3173).
Participants from the de novo (i.e., recently diagnosed) cohort with PD containing baseline and 5-year follow-up neuropsychological testing scores were included in the final analysis (n = 307). Participants defined as PD without available DaT imaging and scans without evidence of dopaminergic deficit (SWEDD) were not included to reduce the heterogeneity of the participant sample. There were missing data for disease duration and race/ethnicity for two participants and therefore not included in the linear regression analyses for pPREDICT (n = 305).
2.2. Neuropsychological battery and composite cognitive score
Five neuropsychological measures spanning different cognitive domains were included in the analysis: (1) attention and working memory: WAIS-IV Letter Number Sequencing (LNS) (Wechsler, 1987), (2) executive function: Symbol Digit Modalities Test (SDMT) (Smith, 1973), (3) language: category/semantic (animal) fluency (SF) (Straus et al., 2006), (4) memory: Hopkins Verbal Learning Test, Delayed Recall (HVLT-DR) (Brandt, 1991), and (5) visuospatial function: Benton’s Judgment of Line Orientation (JLO) (Benton et al., 1978). Raw neuropsychological test scores for JLO, SDMT, and SF were converted to z-scores based on normative data drawn either from testing manuals (Smith, 1973; Benton et al., 1978) or additional studies (Tombaugh et al., 1999). For HVLT-DR and LNS, the PPMI-derived T-scores and scaled scores, respectively, were converted to z-scores. Baseline and 5-year follow-up composite cognitive scores (CCS) were calculated as a mean of the z-scores. CCS are routinely used in Alzheimer’s disease research (Langbaum et al., 2014; Burnham et al., 2015) and used in PD cognitive research (Wood et al., 2021).
The Montreal Cognitive Assessment (MoCA) is a sensitive screening tool for cognitive impairment in PD (Chou et al., 2010; Kim et al., 2019), however is not intended to replace formal neuropsychological testing. The MoCA is a screening tool that identifies individuals with PD at higher risk for cognitive impairment, but even with validated cut-off values does not perform perfectly with only 64% of individuals with PD correctly diagnosed on the cognitive spectrum (Hoops et al., 2009); therefore, it is not unreasonable to use as a risk factor for cognitive decline. The MoCA score was also not used for the outcome measure to determine cognitive decline (CCS), thereby avoiding circularity in the statistical analysis for MoPaRDS and pPREDICT. Additionally, MoCA is a cognitive screen that can easily be performed in multiple clinical settings (Nasreddine et al., 2005), therefore use is not restricted to tertiary/quaternary care centers or in the research setting making it ideal for the goal of wider access.
2.3. Cognitive trajectory classification
Cognitive decliners (PD-decline) were defined as ≥0.5 standard deviation (SD) CCS decline from baseline to 5-year follow-up and cognitive maintainers (PD-maintain) as <0.5 CCS decline. This cutoff was chosen given Movement Disorder Society Task Force Guidelines for diagnosing PD mild cognitive impairment (PD-MCI) based on significant decline on serial neuropsychological testing (Litvan et al., 2012); since PPMI is a highly educated cohort (Marek et al., 2018), significant decline over a 5-year period was defined as ≥0.5 SD CCS.
2.4. LIBRA scoring
For LIBRA score calculations, points were attributed to each risk factor as previously described (Vos et al., 2017; Table 1). Smoking status, alcohol use history, diet, and cognitively based activities are not reliably available through PPMI due to missingness of data, but there is precedence for omitting components of LIBRA due to absence of data in establish cohorts (Vos et al., 2017; van Middelaar et al., 2018). Risk factors from PPMI data requiring specific cutoff values for LIBRA were based on previous studies applying the LIBRA score (Table 1; Deckers et al., 2018; van Middelaar et al., 2018). We were unable to identify any prior publications using Physical Activity for the Elderly Scale (PASE) for calculating LIBRA. However, original Delphi consensus compared highest to lowest level of activity (Deckers et al., 2015); therefore, a cutoff for moderate-intense activity (i.e., PASE >90) was selected.
2.5. MoPaRDS scoring
For MoPaRDS score calculations, a point was added for each of the eight risk factors as previously described (Dawson et al., 2018; Table 1). As the PPMI database was used previously for MoPaRDS, the same cutoff values were used (Dawson et al., 2018).
2.6. pPREDICT scoring
For pPREDICT, dichotomous cutoff values for risk factors were chosen based on literature search (Table 1; Johns, 1991; Nasreddine et al., 2005; Dissanayaka et al., 2007; Julian, 2011; Chahine et al., 2013; Segura et al., 2014; Mak et al., 2015; Martinez-Martin et al., 2015; Santangelo et al., 2015; Gottesman et al., 2017; Pedersen et al., 2017; Peraza et al., 2017; Hong et al., 2018; Curcio et al., 2019; Li et al., 2019; Ben-Joseph et al., 2020; Reekes et al., 2020; Forbes et al., 2021). For total pPREDICT score calculations, the predicted dichotomous risk factors each represented a point toward fourteen possible total points. This point system is similar to other screening tools designed for easy use in the clinical setting, including the office-based MoPaRDS screen for cognitive decline in PD that showed no significant improvement in diagnostic accuracy with weighting (Dawson et al., 2018).
2.7. Statistical analysis
Clinical and demographic features of the PD-decline and PD-maintain groups were evaluated using descriptive statistics. Groupwise comparisons were made using either t-tests or Mann Whitney U tests as appropriate based on data skew. Sensitivity, specificity, and receiver operating curves (ROCs) were determined for group classification of PD-decline using LIBRA, MoPaRDS, and pPREDICT scores. ROC comparisons were made to test the differences in ROC area under the curves (AUCs) for LIBRA, MoPaRDS, and pPREDICT using chi-square difference test. Linear regression models were built with CCS as the continuous outcome, first with total LIBRA, MoPaRDS, or pPREDICT score as the predictors, then also with each of the individual risk factors as predictors. Statistical analyses were performed using the Stata 15 software (StataCorp. 2017. Stata Statistical Software: Release 15. College Station, TX: StataCorp LLC).
3. Results
Table 2 displays the characteristics of the de novo PPMI participants with PD included in the analysis. The PD-decline group (n = 44) was older (64.8 ± 9.1 versus 60.1 ± 9.8 years, p = 0.004) and with higher Unified Parkinson’s Disease Rating Scale (UPDRS) Pt. III motor score (22.4 ± 9.7 versus 19.5 ± 8.2, p = 0.04), Rapid Eye Movement (REM) Sleep Behavior Disorder Questionnaire (RSBDQ) (4.1 ± 3.0 versus 2.9 ± 2.4, p = 0.004), and Epworth Sleepiness Scale (ESS) (7.0 ± 3.8 versus 5.5 ± 3.1, p = 0.0003) scores compared to the PD-maintain group (n = 263). Additionally, the PD-decline group had higher LIBRA (6.76 ± 3.77, p = 0.0013), MoPaRDS (2.45 ± 1.41, p = 0.0001), and pPREDICT scores (4.52 ± 1.66, p < 0.001) compared to the PD-maintain group (LIBRA 4.98 ± 3.30, MoPaRDS 1.67 ± 1.16, pPREDICT 3.38 ± 1.69).
The ROC-AUC for distinguishing PD-decline versus PD-maintain was 0.64 (95% CI, 0.55–0.73) for LIBRA, 0.66 (95% CI, 0.58–0.75) for MoPaRDS, and 0.68 (95% CI, 0.61–0.76) for pPREDICT (Figure 1A). LIBRA score ≥ 4.2, chosen based on previous cutoff value for high risk (Vos et al., 2017), conferred 68.2% sensitivity and 49.4% specificity for identifying PD-decline which correctly classified 52.1% with a positive likelihood ratio (LR+) of 1.35 and negative LR (LR-) of 0.64. MoPaRDS score ≥ 4, chosen based on previous cutoff value (Dawson et al., 2018), conferred 25.0% sensitivity and 92.8% specificity for distinguishing PD-decline from PD-maintain which correctly classified 83.1% with a LR+ of 3.46 and LR- of 0.81. A pPREDICT cutoff of ≥4, chosen to maximize sensitivity without compromising sensitivity (Figure 1B), conferred 77.2% sensitivity and 54.3% specificity for identifying PD-decline which correctly classified 57.7% with a LR+ of 1.69 and LR- of 0.42. Comparing the ROC-AUC for LIBRA, MoPaRDS, and pPREDICT revealed no significant differences (chi-squared = 0.95, p = 0.62).
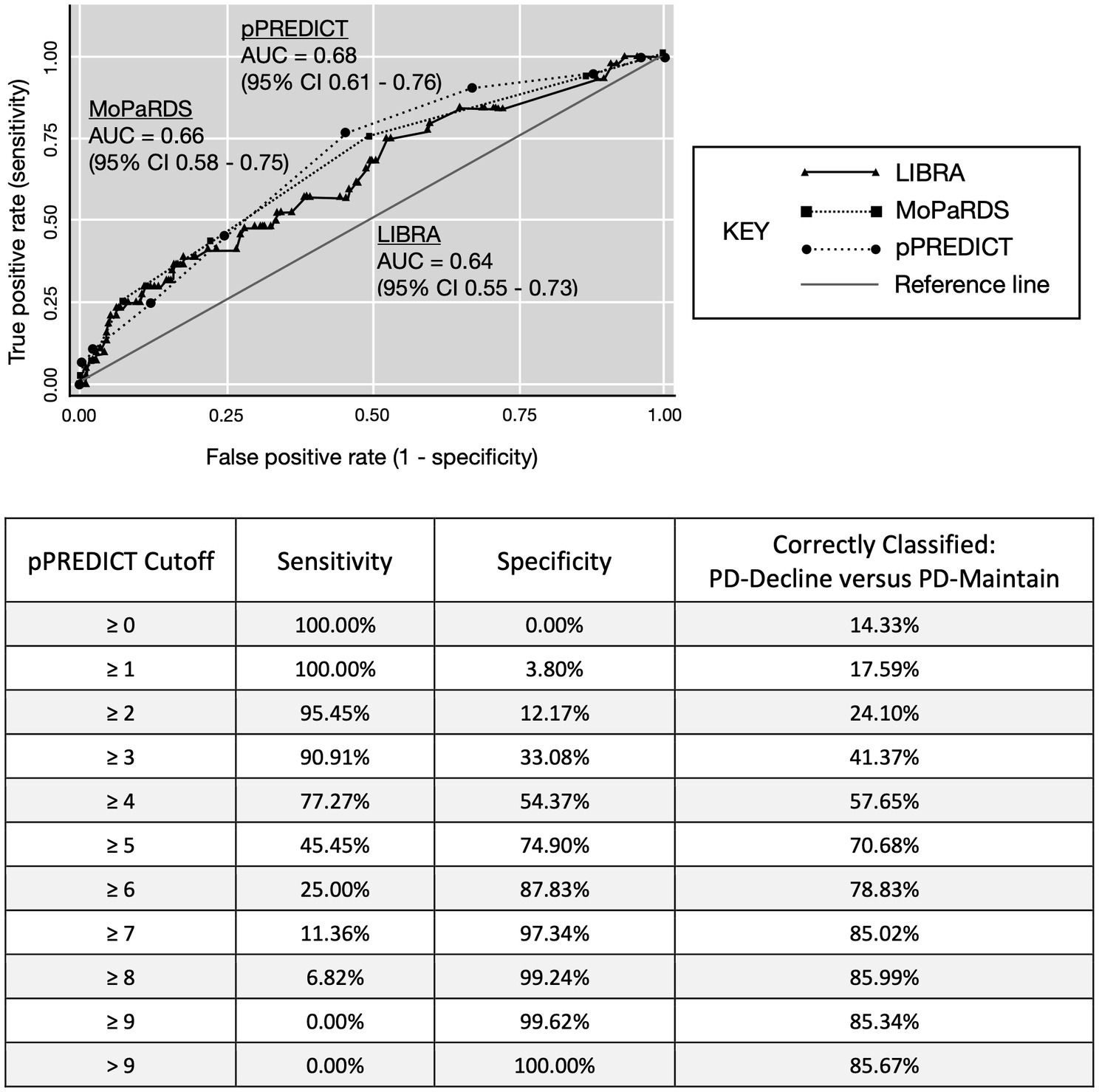
Figure 1. ROC-AUC curve for LIBRA and pPREDICT. AUC, area under the curve; LIBRA, Lifestyle for Brain Health; pPREDICT, preliminary Parkinson’s disease Risk Estimator for Decline In Cognition Tool. MoPaRDS, Montreal Parkinson Risk of Dementia Scale.
In linear regression analyses with change in CCS as the continuous dependent variable, total LIBRA (n = 307, p = 0.020), MoPaRDS (n = 307, p < 0.001), and pPREDICT (n = 305, p < 0.001) scores were associated with change in CCS. In a linear regression analysis with the nine LIBRA risk factors that were included as independent variables, the R-squared value was 0.063. The LIBRA risk factor that contributed to the model was age stratified by sex (coefficient −0.044, 95% CI -0.070 – −0.019; p = 0.001; Table 3). In a linear regression analysis with the eight MoPaRDS risk factors that were included as independent variables, the R-squared value was 0.13. For MoPaRDS, age older than 70 years (coefficient − 0.26, 95% CI -0.41 – −0.099; p = 0.001) and report of hallucinations (coefficient −0.90, 95% CI -1.3 – −0.53; p < 0.001) contributed to the model (Table 3). In a linear regression analysis with the fourteen pPREDICT risk factors as independent variables, the R-squared value was 0.15. For pPREDICT, male sex (coefficient 0.15, 95% CI 0.0028–0.30; p = 0.046), older age (coefficient −0.25, 95% CI −0.39 – −0.12; p < 0.001), excessive daytime sleepiness (coefficient −0.47, 95% CI −0.71 – −0.23; p < 0.001), and moderate–severe motor symptoms (coefficient −0.30, 95% CI −0.52 – −0.072; p = 0.010) contributed to the model (Table 3).
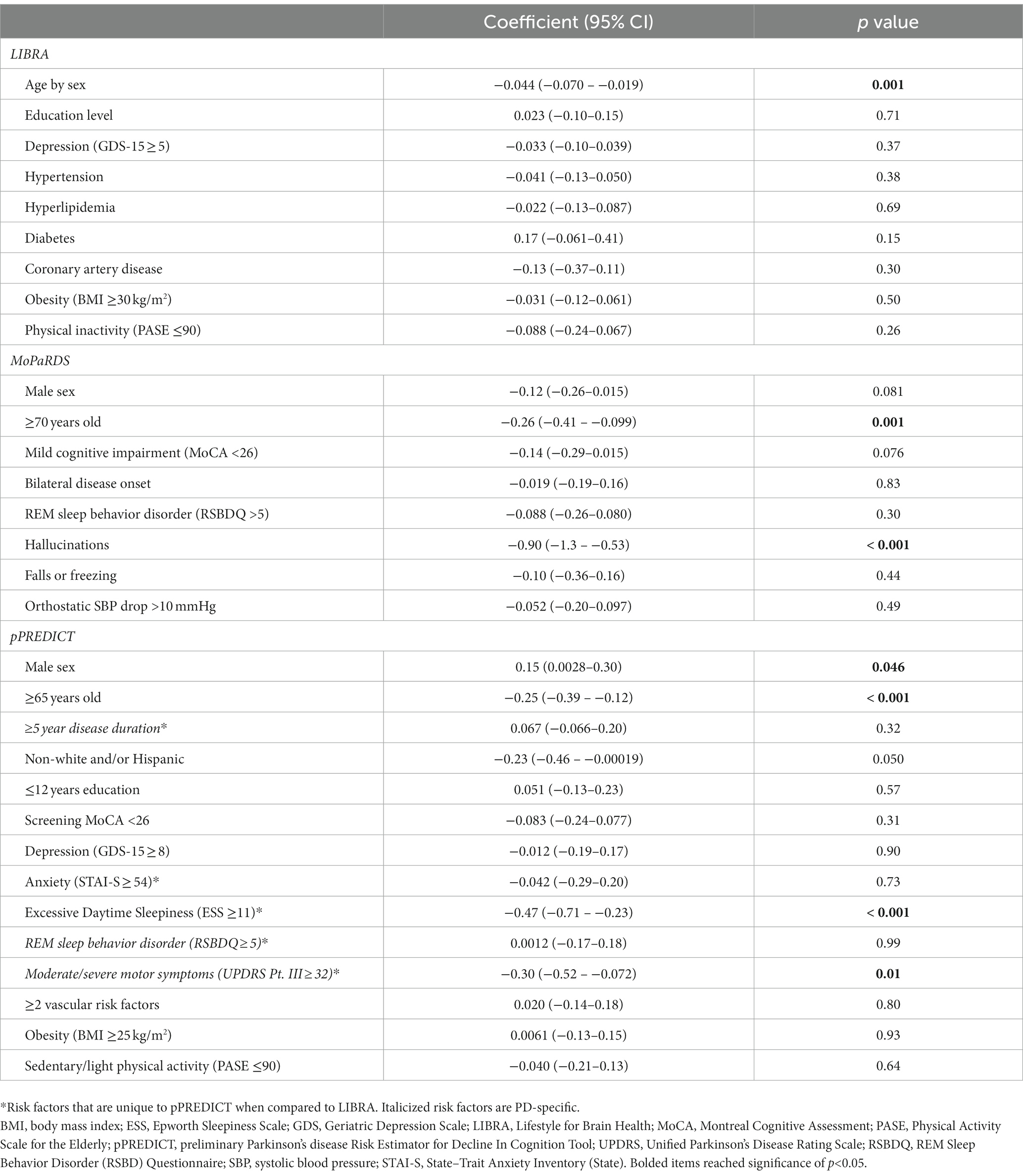
Table 3. Linear regression analyses of the association between baseline risk factors for LIBRA and pPREDICT with change in CCS over 5 years.
4. Conclusion
There is currently no disease-modifying therapy available for cognitive impairment in PD (Sun and Armstrong, 2021). Potentially modifiable risk factors are associated with 40% of all-cause dementia cases worldwide (Livingston et al., 2020); therefore, lifestyle modifications may potentially impact cognitive outcomes in PD. For example, there is evidence that aerobic exercise improves cognition in PD-MCI (Sun and Armstrong, 2021). The goal of this study was to adapt or explore development of a PD-specific predictor tool focusing on modifiable and/or treatable risk factors while being user-friendly and easily applied in a variety of clinical settings. Given that many individuals with PD do not receive care from movement disorder specialists – and even often do not have regular appointments with a neurologist (Pearson et al., 2023) – the development of a simple tool to help guide personalize discussions about cognitive decline risk is of significant importance. Such a predictor tool would provide personalized recommendations for specific individuals with PD rather than relying on general recommendations for the masses.
LIBRA was an ideal starting point as a tool developed for all-cause dementia focusing on modifiable lifestyle factors (Deckers et al., 2015). LIBRA was associated with change in CCS over 5 years in this PD population. Importantly, the only LIBRA risk factor that contributed to the model was non-modifiable age stratified by sex. Of note, LIBRA scoring applies higher risk to female sex for every age > 65 years, whereas male sex has higher risk of cognitive decline in PD. MoPaRDS is a PD-specific tool developed for use in clinical settings (Dawson et al., 2018; Reekes et al., 2020), therefore is also an important tool for comparison given that it holds promise for scalability to a wider PD population. MoPaRDS was also associated with change in CCS over 5 years; however, only non-modifiable older age and report of hallucinations contributed to the linear regression model. The presence of hallucinations alone is associated with an increased risk of mortality in individuals with PD (Isaacson and Citrome, 2022). Hallucinations are a treatable symptom in PD, however antipsychotics can worsen motor symptoms and increase the mortality in individuals with dementia. Therefore, unmodified LIBRA and MoPaRDS risk tools may both identify at-risk individuals with PD, but neither highlight actionable lifestyle modifications or treatable symptoms to potentially mitigate this risk.
pPREDICT may provide more PD-specific information on the risk of cognitive decline compared to LIBRA while also focusing on potentially modifiable risk factors unlike the PD-specific MoPaRDS. Although the ROC-AUC were not significantly different between LIBRA, MoPaRDS, and pPREDICT, pPREDICT has the potential for being more PD-specific while also focusing on potentially modifiable riss and/or treatable symptoms. Not only was the total pPREDICT score associated with change in CCS from baseline to 5-year follow-up in this PD population, but multiple individual risk factors contributed including male sex, older age, excessive daytime sleepiness, and moderate–severe motor symptoms. Therefore, potentially treatable risk factors contributed to the pPREDICT model, which could represent actionable targets for personalized risk reduction.
There are multiple advantages to pPREDICT. It uses demographic and clinical information that can be collected without additional invasive and/or expensive diagnostic testing. It also does not rely on specific neuropsychological tests rather uses a cognitive screen (i.e., MoCA) that can be performed in a variety of clinical settings where individuals with PD regularly receive care. Therefore, while the performance of previously reported cognitive decline predictor tools are superior in their respective cohorts (Schrag et al., 2017; Dawson et al., 2018; Gramotnev et al., 2019), pPREDICT holds potential for wider applicability outside of tertiary/quaternary care centers or in the research setting. Unlike other office-based tools for predicting PD-associated cognitive decline including the MoPaRDS, it focuses on potentially reversible/modifiable risk factors. Additionally, the simplicity of using dichotomized risk factors may allow for application to clinical settings that use alternative questionnaires. For example, although PPMI used the Geriatric Depression Scale Short Form (GDS-15) for depression assessment, there are other depression scales with validated cutoff values in PD (i.e., Hospital Anxiety and Depression Scale (Mondolo et al., 2006)) that could potentially be used with pPREDICT; however, pPREDICT needs to be validated in cohorts using alternative scales to determine applicability outside of the PPMI-chosen questionnaires.
Excessive daytime sleepiness was significantly associated with cognitive decline in the pPREDICT model. Excessive daytime sleepiness has been suggested as a marker of more severe disease in PD, however there are also potential treatable causes of excessive daytime sleepiness in individuals with PD including unrecognized/undiagnosed sleep apnea or more broadly sleep disordered breathing, insomnia, or uncontrolled restless leg syndrome symptoms (Zuzuarregui and During, 2020). Excessive daytime sleepiness can be treated with light therapy (Zuzuarregui and During, 2020), although meta-analysis has not shown benefit for excessive daytime sleepiness, depressive symptoms, or motor symptoms (Huang et al., 2021). Additionally, the potential longer-term benefits on cognitive outcomes of treating excessive daytime sleepiness in PD are currently unknown, however an area of interest given that it may be a potentially modifiable risk. At this time, it is not clear whether excessive daytime sleepiness is a sign of more severe or progressive disease – therefore associated with cognitive impairment – but given that excessive daytime sleepiness may have potential treatable causes with cognitive impacts, it is important to be aware of the potential role in individualized risk reduction.
Moderate to severe motor symptoms was also significantly associated with cognitive decline in the pPREDICT model. Although this association is likely to be multifactorial including a relationship with disease severity, a potential modifiable/treatable mechanism is physical activity. A questionnaire to assess for physical activity (i.e., the Physical Activity Scale for the Elderly [PASE]) was a measurement added to PPMI after the trial started (Mantri et al., 2018), therefore values for scoring pPREDICT were not limited to baseline assessments for all the participants creating some variability. Additionally, there is evidence of individuals with PD over-reporting activity as compared to objective measurements (Mantri et al., 2019). Despite the hurdles of studying physical activity in large cohort studies, there is evidence supporting physical activity for improved motor outcomes (Ernst et al., 2023), as well as evidence for exercise improving cognition outcomes in PD (Sun and Armstrong, 2021). There may be variable impact on specific cognitive domains with differential responses for specific modalities (Sun and Armstrong, 2021), however the longer-term benefits on cognitive outcomes is an area of active research. Physical activity is a modifiable risk for all-cause dementia (Livingston et al., 2020), therefore is important to highlight in individuals with PD especially with increased risk of cognitive and motor decline.
The other potentially modifiable and/or treatable risk factors in pPREDICT that did not significantly contribute to the model included REM sleep behavior disorder (RSBD), depression, anxiety, obesity, and multiple vascular risk factors. One potential reason for RSBD not significantly contributing to the model for MoPaRDS or pPREDICT was using clinical history via the RSBDQ rather than confirmed diagnosis by polysomnography. REM sleep behavior disorder in PD is associated with worse cognitive performance (Maggi et al., 2021), therefore there is interest in targeting RSBD for improvement of cognitive outcomes in PD and in idiopathic cases. Depression and anxiety are both common non-motor symptoms associated with PD, however the screens (i.e., GDS-15, State-Trait Anxiety Inventory [STAI]) may be capturing other symptoms in PD such as apathy and fatigue (i.e., GDS-15) (Lopez et al., 2018) and autonomic dysfunction (i.e., STAI) (Wang et al., 2023). As a result, it is possible these screens are not sensitive enough for depression or anxiety to provide the necessary signal for pPREDICT. Higher body mass index (BMI) at baseline has previously been shown to be associated with cognitive decline in the PPMI cohort per sequential MoCA assessments (Forbes et al., 2021), however higher BMI did not significantly contribute to the pPREDICT model perhaps as a result of using CCS as the outcome. Additionally, vascular risk factors in the setting of PD are associated with performance on specific cognitive domains (Pilotto et al., 2016), therefore using CCS may have masked any contributions to the pPREDICT model.
The dataset and approach used to develop pPREDICT have several limitations. The PPMI cohort is highly educated compared to the general population as is not uncommon for clinical trials, so it needs to be validated in other cohorts including those with lower educational status to determine the applicability to other populations. Additionally, cognitive decline in PD takes years to develop, so the relatively short follow-up of 5 years may be too short. The predicted conversion rate from PD-MCI to PDD is about 10% per year (Sun and Armstrong, 2021) and nearly half of individuals with PD and normal cognition develop cognitive impairment within 6 years (Pigott et al., 2015). Additionally, the follow-up duration for pPREDICT is longer (Schrag et al., 2017; Hogue et al., 2018; Yousaf et al., 2019; Wilson et al., 2020) or on par (Dawson et al., 2018; Gramotnev et al., 2019) with prior cognitive decline predictor tools. This initial pPREDICT does not correct for absent neuropsychological data at 5-year follow-up, which may be concentrated for individuals experiencing decline thereby producing bias in the data. A future approach to correct for missingness by imputing data based on the trajectory of available follow-up will clarify whether the model improves. Lastly, using change in CCS as the outcome measure of cognitive decline may mask decline isolated to specific cognitive domains that may be more sensitive to PD-specific cognitive changes. Investigating the risk factors associated with decline in specific neuropsychological tests will be informative in the future.
This is a first step in developing a pragmatic and practical tool to predict cognitive decline in PD focusing on potentially modifiable risk factors. Further refinement of the tool aimed at improving performance while maintaining simplicity is the next step. Once a refined tool is developed, external validation will be important to determine wider applicability and scalability. In the future, this tool may allow for personalized counseling on actionable lifestyle modifications and other disease-specific treatments to reduce risk of cognitive decline in individuals with PD.
Data availability statement
Publicly available datasets were analyzed in this study. This data can be found here: https://www.ppmi-info.org/.
Ethics statement
The studies involving humans were approved by Colorado Multiple Institutional Review Board. The studies were conducted in accordance with the local legislation and institutional requirements. Written informed consent for participation was not required from the participants or the participants’ legal guardians/next of kin in accordance with the national legislation and institutional requirements.
Author contributions
TC: Conceptualization, Data curation, Funding acquisition, Investigation, Methodology, Project administration, Writing – original draft, Writing – review & editing. LM: Methodology, Supervision, Writing – review & editing. SH: Conceptualization, Formal analysis, Methodology, Supervision, Writing – review & editing.
Funding
The author(s) declare financial support was received for the research, authorship, and/or publication of this article. This work was supported by the University of Colorado Movement Disorders Center Pilot Grant (2020). PPMI – a public-private partnership – is funded by the Michael J. Fox Foundation for Parkinson’s Research funding partners 4D Pharma, Abbvie, Acurex Therapeutics, Allergan, Amathus Therapeutics, ASAP, Avid Radiopharmaceuticals, Bial Biotech, Biogen, BioLegend, Bristol-Myers Squibb, BlueRock Therapeutics, Calico, Celgene, Dacapo Brain Science, Denali, The Edmond J. Safra Foundaiton, GE Healthcare, Genentech, GlaxoSmithKline, Golub Capital, Handl Therapeutics, Insitro, Janssen Neuroscience, Lilly, Lundbeck, Merck, Meso Scale Discovery, Neurocrine Biosciences, Pfizer, Piramal, Prevail, Roche, Sanofi Genzyme, Servier, Takeda, Teva, UCB, Verily, and Voyager Therapeutics.
Conflict of interest
LM has received research grant support from the Alzheimer’s Association and the National Institutes of Health. SH has received research grant support from the National Institutes of Health and the Michael J. Fox Foundation for Parkinson’s Research, and honoraria from the Parkinson Association of the Rockies and American Academy of Neurology. The Michael J. Fox Foundation for Parkinson’s Research, the Parkinson Association of the Rockies, the American Academy of Neurology, National Institutes of Health, and the Alzheimer’s Association had no involvement in the study.
The remaining author declares that the research was conducted in the absence of any commercial or financial relationships that could be construed as a potential conflict of interest.
Publisher’s note
All claims expressed in this article are solely those of the authors and do not necessarily represent those of their affiliated organizations, or those of the publisher, the editors and the reviewers. Any product that may be evaluated in this article, or claim that may be made by its manufacturer, is not guaranteed or endorsed by the publisher.
Footnotes
References
Aarsland, D., Andersen, K., Larsen, J. P., Lolk, A., and Kragh-Sorensen, P. (2003). Prevalence and characteristics of dementia in Parkinson disease: an 8-year prospective study. Arch. Neurol. 60, 387–392. doi: 10.1001/archneur.60.3.387
Aarsland, D., Bronnick, K., Larsen, J. P., Tysnes, O. B., and Alves, G., Norwegian Park West Study G (2009). Cognitive impairment in incident, untreated Parkinson disease: the Norwegian ParkWest study. Neurology 72, 1121–1126. doi: 10.1212/01.wnl.0000338632.00552.cb
Baiano, C., Barone, P., Trojano, L., and Santangelo, G. (2020). Prevalence and clinical aspects of mild cognitive impairment in Parkinson's disease: a meta-analysis. Mov. Disord. 35, 45–54. doi: 10.1002/mds.27902
Ben-Joseph, A., Marshall, C. R., Lees, A. J., and Noyce, A. J. (2020). Ethnic variation in the manifestation of Parkinson's disease: a narrative review. J. Parkinsons Dis. 10, 31–45. doi: 10.3233/JPD-191763
Benton, A. L., Varney, N. R., and Hamsher, K. D. (1978). Visuospatial judgment. A clinical test. Arch Neurol. 35, 364–367. doi: 10.1001/archneur.1978.00500300038006
Brandt, J. (1991). The Hopkins verbal learning test: development of a new memory test with six equivalent forms. Clin. Neuropsychol. 5, 125–142. doi: 10.1080/13854049108403297
Burnham, S. C., Raghavan, N., Wilson, W., Baker, D., Ropacki, M. T., Novak, G., et al. (2015). Novel statistically-derived composite measures for assessing the efficacy of disease-modifying therapies in prodromal Alzheimer's disease trials: an AIBL study. J. Alzheimers Dis. 46, 1079–1089. doi: 10.3233/JAD-143015
Chahine, L. M., Daley, J., Horn, S., Colcher, A., Hurtig, H., Cantor, C., et al. (2013). Questionnaire-based diagnosis of REM sleep behavior disorder in Parkinson's disease. Mov. Disord. 28, 1146–1149. doi: 10.1002/mds.25438
Chahine, L. M., Dos Santos, C., Fullard, M., Scordia, C., Weintraub, D., Erus, G., et al. (2019). Modifiable vascular risk factors, white matter disease and cognition in early Parkinson's disease. Eur. J. Neurol. 26, 246–e18. doi: 10.1111/ene.13797
Chou, K. L., Amick, M. M., Brandt, J., Camicioli, R., Frei, K., Gitelman, D., et al. (2010). A recommended scale for cognitive screening in clinical trials of Parkinson's disease. Mov. Disord. 25, 2501–2507. doi: 10.1002/mds.23362
Curcio, F., Liguori, I., Cellulare, M., Sasso, G., Della-Morte, D., Gargiulo, G., et al. (2019). Physical activity scale for the elderly (PASE) score is related to sarcopenia in noninstitutionalized older adults. J. Geriatr. Phys. Ther. 42, 130–135. doi: 10.1519/JPT.0000000000000139
Dawson, B. K., Fereshtehnejad, S. M., Anang, J. B. M., Nomura, T., Rios-Romenets, S., Nakashima, K., et al. (2018). Office-based screening for dementia in Parkinson disease: the Montreal Parkinson risk of dementia scale in 4 longitudinal cohorts. JAMA Neurol. 75, 704–710. doi: 10.1001/jamaneurol.2018.0254
Deckers, K., Kohler, S., van Boxtel, M., Verhey, F., Brayne, C., and Fleming, J. (2018). Lack of associations between modifiable risk factors and dementia in the very old: findings from the Cambridge City over-75s cohort study. Aging Ment. Health 22, 1272–1278. doi: 10.1080/13607863.2017.1280767
Deckers, K., van Boxtel, M. P., Schiepers, O. J., de Vugt, M., Muñoz Sánchez, J. L., Anstey, K. J., et al. (2015). Target risk factors for dementia prevention: a systematic review and Delphi consensus study on the evidence from observational studies. Int. J. Geriatr. Psychiatry 30, 234–246. doi: 10.1002/gps.4245
Dissanayaka, N. N., Sellbach, A., Matheson, S., Marsh, R., Silburn, P. A., O'Sullivan, J. D., et al. (2007). Validity of Hamilton depression inventory in Parkinson's disease. Mov. Disord. 22, 399–403. doi: 10.1002/mds.21309
Ernst, M., Folkerts, A. K., Gollan, R., Lieker, E., Caro-Valenzuela, J., Adams, A., et al. (2023). Physical exercise for people with Parkinson's disease: a systematic review and network meta-analysis. Cochrane Database Syst. Rev. 1:CD013856. doi: 10.1002/14651858.CD013856.pub2
Forbes, E., Tropea, T. F., Mantri, S., Xie, S. X., and Morley, J. F. (2021). Modifiable comorbidities associated with cognitive decline in Parkinson's disease. Mov. Disord. Clin. Pract. 8, 254–263. doi: 10.1002/mdc3.13143
Gottesman, R. F., Schneider, A. L., Zhou, Y., Coresh, J., Green, E., Gupta, N., et al. (2017). Association between midlife vascular risk factors and estimated brain amyloid deposition. JAMA 317, 1443–1450. doi: 10.1001/jama.2017.3090
Gramotnev, G., Gramotnev, D. K., and Gramotnev, A. (2019). Parkinson's disease prognostic scores for progression of cognitive decline. Sci. Rep. 9:17485. doi: 10.1038/s41598-019-54029-w
Guo, Y., Liu, F. T., Hou, X. H., Li, J. Q., Cao, X. P., Tan, L., et al. (2021). Predictors of cognitive impairment in Parkinson's disease: a systematic review and meta-analysis of prospective cohort studies. J. Neurol. 268, 2713–2722. doi: 10.1007/s00415-020-09757-9
Hogue, O., Fernandez, H. H., and Floden, D. P. (2018). Predicting early cognitive decline in newly-diagnosed Parkinson's patients: a practical model. Parkinsonism Relat. Disord. 56, 70–75. doi: 10.1016/j.parkreldis.2018.06.031
Hong, J. Y., Lee, Y., Sunwoo, M. K., Sohn, Y. H., and Lee, P. H. (2018). Subjective cognitive complaints and objective cognitive impairment in Parkinson's disease. J. Clin. Neurol. 14, 16–21. doi: 10.3988/jcn.2018.14.1.16
Hoops, S., Nazem, S., Siderowf, A. D., Duda, J. E., Xie, S. X., Stern, M. B., et al. (2009). Validity of the MoCA and MMSE in the detection of MCI and dementia in Parkinson disease. Neurology 73, 1738–1745. doi: 10.1212/WNL.0b013e3181c34b47
Huang, H. T., Huang, T. W., and Hong, C. T. (2021). Bright light therapy for Parkinson disease: a literature review and Meta-analysis of randomized controlled trials. Biology (Basel). 10:1205. doi: 10.3390/biology10111205
Isaacson, S. H., and Citrome, L. (2022). Hallucinations and delusions associated with Parkinson's disease psychosis: safety of current treatments and future directions. Expert Opin. Drug Saf. 21, 873–879. doi: 10.1080/14740338.2022.2069240
Johns, M. W. (1991). A new method for measuring daytime sleepiness: the Epworth sleepiness scale. Sleep 14, 540–545. doi: 10.1093/sleep/14.6.540
Julian, L. J. (2011). Measures of anxiety: state-trait anxiety inventory (STAI), Beck anxiety inventory (BAI), and hospital anxiety and depression scale-anxiety (HADS-A). Arthritis Care Res (Hoboken). 63, S467–S472. doi: 10.1002/acr.20561
Kim, H. M., Nazor, C., Zabetian, C. P., Quinn, J. F., Chung, K. A., Hiller, A. L., et al. (2019). Prediction of cognitive progression in Parkinson's disease using three cognitive screening measures. Clin. Park. Relat. Disord. 1, 91–97. doi: 10.1016/j.prdoa.2019.08.006
Langbaum, J. B., Hendrix, S. B., Ayutyanont, N., Chen, K., Fleisher, A. S., Shah, R. C., et al. (2014). An empirically derived composite cognitive test score with improved power to track and evaluate treatments for preclinical Alzheimer's disease. Alzheimers Dement. 10, 666–674. doi: 10.1016/j.jalz.2014.02.002
Li, M. G., Chen, Y. Y., Chen, Z. Y., Feng, J., Liu, M. Y., Lou, X., et al. (2019). Altered functional connectivity of the marginal division in Parkinson's disease with mild cognitive impairment: a pilot resting-state fMRI study. J. Magn. Reson. Imaging 50, 183–192. doi: 10.1002/jmri.26548
Litvan, I., Goldman, J. G., Tröster, A. I., Schmand, B. A., Weintraub, D., Petersen, R. C., et al. (2012). Diagnostic criteria for mild cognitive impairment in Parkinson's disease: movement disorder society task force guidelines. Mov. Disord. 27, 349–356. doi: 10.1002/mds.24893
Livingston, G., Huntley, J., Sommerlad, A., Ames, D., Ballard, C., Banerjee, S., et al. (2020). Dementia prevention, intervention, and care: 2020 report of the Lancet Commission. Lancet 396, 413–446. doi: 10.1016/s0140-6736(20)30367-6
Lopez, F. V., Split, M., Filoteo, J. V., Litvan, I., Moore, R. C., Pirogovsky-Turk, E., et al. (2018). Does the geriatric depression scale measure depression in Parkinson's disease? Int. J. Geriatr. Psychiatry 33, 1662–1670. doi: 10.1002/gps.4970
Maggi, G., Trojano, L., Barone, P., and Santangelo, G. (2021). Sleep disorders and cognitive dysfunctions in Parkinson's disease: a meta-analytic study. Neuropsychol. Rev. 31, 643–682. doi: 10.1007/s11065-020-09473-1
Mak, E., Su, L., Williams, G. B., Firbank, M. J., Lawson, R. A., Yarnall, A. J., et al. (2015). Baseline and longitudinal grey matter changes in newly diagnosed Parkinson's disease: ICICLE-PD study. Brain 138, 2974–2986. doi: 10.1093/brain/awv211
Mantri, S., Fullard, M. E., Duda, J. E., and Morley, J. F. (2018). Physical activity in early Parkinson disease. J. Parkinsons Dis. 8, 107–111. doi: 10.3233/JPD-171218
Mantri, S., Wood, S., Duda, J. E., and Morley, J. F. (2019). Comparing self-reported and objective monitoring of physical activity in Parkinson disease. Parkinsonism Relat. Disord. 67, 56–59. doi: 10.1016/j.parkreldis.2019.09.004
Marek, K., Chowdhury, S., Siderowf, A., Lasch, S., Coffey, C. S., Caspell-Garcia, C., et al. (2018). The Parkinson's progression markers initiative (PPMI) – establishing a PD biomarker cohort. Ann. Clin. Transl. Neurol. 5, 1460–1477. doi: 10.1002/acn3.644
Martinez-Martin, P., Rodriguez-Blazquez, C., Mario, A., Arakaki, T., Arillo, V. C., Chaná, P., et al. (2015). Parkinson's disease severity levels and MDS-unified Parkinson's disease rating scale. Parkinsonism Relat. Disord. 21, 50–54. doi: 10.1016/j.parkreldis.2014.10.026
Mondolo, F., Jahanshahi, M., Grana, A., Biasutti, E., Cacciatori, E., and Di Benedetto, P. (2006). The validity of the hospital anxiety and depression scale and the geriatric depression scale in Parkinson's disease. Behav. Neurol. 17, 109–115. doi: 10.1155/2006/136945
Nasreddine, Z. S., Phillips, N. A., Bédirian, V., Charbonneau, S., Whitehead, V., Collin, I., et al. (2005). The Montreal cognitive assessment, MoCA: a brief screening tool for mild cognitive impairment. J. Am. Geriatr. Soc. 53, 695–699. doi: 10.1111/j.1532-5415.2005.53221.x
Nicoletti, A., Luca, A., Baschi, R., Cicero, C. E., Mostile, G., Davì, M., et al. (2021). Vascular risk factors, white matter lesions and cognitive impairment in Parkinson's disease: the PACOS longitudinal study. J. Neurol. 268, 549–558. doi: 10.1007/s00415-020-10189-8
Paul, K. C., Chuang, Y. H., Shih, I. F., Keener, A., Bordelon, Y., Bronstein, J. M., et al. (2019). The association between lifestyle factors and Parkinson's disease progression and mortality. Mov. Disord. 34, 58–66. doi: 10.1002/mds.27577
Pearson, C., Hartzman, A., Munevar, D., Feeney, M., Dolhun, R., Todaro, V., et al. (2023). Care access and utilization among medicare beneficiaries living with Parkinson's disease. NPJ Parkinsons Dis. 9:108. doi: 10.1038/s41531-023-00523-y
Pedersen, K. F., Larsen, J. P., Tysnes, O. B., and Alves, G. (2017). Natural course of mild cognitive impairment in Parkinson disease: a 5-year population-based study. Neurology 88, 767–774. doi: 10.1212/WNL.0000000000003634
Peraza, L. R., Nesbitt, D., Lawson, R. A., Duncan, G. W., Yarnall, A. J., Khoo, T. K., et al. (2017). Intra-and inter-network functional alterations in Parkinson's disease with mild cognitive impairment. Hum. Brain Mapp. 38, 1702–1715. doi: 10.1002/hbm.23499
Pigott, K., Rick, J., Xie, S. X., Hurtig, H., Chen-Plotkin, A., Duda, J. E., et al. (2015). Longitudinal study of normal cognition in Parkinson disease. Neurology 85, 1276–1282. doi: 10.1212/WNL.0000000000002001
Pilotto, A., Turrone, R., Liepelt-Scarfone, I., Bianchi, M., Poli, L., Borroni, B., et al. (2016). Vascular risk factors and cognition in Parkinson's disease. J. Alzheimers Dis. 51, 563–570. doi: 10.3233/JAD-150610
Reekes, T. H., Higginson, C. I., Ledbetter, C. R., Sathivadivel, N., Zweig, R. M., and Disbrow, E. A. (2020). Sex specific cognitive differences in Parkinson disease. NPJ Parkinsons Dis. 6:7. doi: 10.1038/s41531-020-0109-1
Riggeal, B. D., Crucian, G. P., Seignourel, P., Jacobson, C. E., Okun, M. S., Rodriguez Rl,, et al. (2007). Cognitive decline tracks motor progression and not disease duration in Parkinson patients. Neuropsychiatr. Dis. Treat. 3, 955–958. doi: 10.2147/ndt.s2237
Santangelo, G., Vitale, C., Trojano, L., Picillo, M., Moccia, M., Pisano, G., et al. (2015). Relationship between apathy and cognitive dysfunctions in de novo untreated Parkinson's disease: a prospective longitudinal study. Eur. J. Neurol. 22, 253–260. doi: 10.1111/ene.12467
Schiepers, O. J. G., Kohler, S., Deckers, K., Irving, K., O'Donnell, C. A., van den Akker, M., et al. (2018). Lifestyle for Brain Health (LIBRA): a new model for dementia prevention. Int. J. Geriatr. Psychiatry 33, 167–175. doi: 10.1002/gps.4700
Schrag, A., Siddiqui, U. F., Anastasiou, Z., Weintraub, D., and Schott, J. M. (2017). Clinical variables and biomarkers in prediction of cognitive impairment in patients with newly diagnosed Parkinson's disease: a cohort study. Lancet Neurol. 16, 66–75. doi: 10.1016/s1474-4422(16)30328-3
Segura, B., Baggio, H. C., Marti, M. J., Valldeoriola, F., Compta, Y., Garcia-Diaz, A. I., et al. (2014). Cortical thinning associated with mild cognitive impairment in Parkinson's disease. Mov. Disord. 29, 1495–1503. doi: 10.1002/mds.25982
Straus, E., Sherman, E., and Spreen, O. A compendium of neuropsychological tests: administration, norms, and commentary. New York, NY Oxford University Press (2006).
Sun, C., and Armstrong, M. J. (2021). Treatment of Parkinson's disease with cognitive impairment: current approaches and future directions. Behav. Sci. 11:54 doi: 10.3390/bs11040054
Tombaugh, T. N., Kozak, J., and Rees, L. (1999). Normative data stratified by age and education for two measures of verbal fluency: FAS and animal naming. Arch. Clin. Neuropsychol. 14, 167–177.
van Middelaar, T., Hoevenaar-Blom, M. P., van Gool, W. A., Moll van Charante, E. P., van Dalen, J. W., Deckers, K., et al. (2018). Modifiable dementia risk score to study heterogeneity in treatment effect of a dementia prevention trial: a post hoc analysis in the pre DIVA trial using the LIBRA index. Alzheimers Res. Ther. 10:62. doi: 10.1186/s13195-018-0389-4
Vos, S. J. B., van Boxtel, M. P. J., Schiepers, O. J. G., Deckers, K., de Vugt, M., Carrière, I., et al. (2017). Modifiable risk factors for prevention of dementia in midlife, late life and the oldest-old: validation of the LIBRA index. J. Alzheimers Dis. 58, 537–547. doi: 10.3233/JAD-161208
Wang, H., Zhao, Y., and Schrag, A. (2023). Development of anxiety in early Parkinson's disease: a clinical and biomarker study. Eur. J. Neurol. 30, 2661–2668. doi: 10.1111/ene.15890
Wechsler, D. Wechsler memory scale—Revised manual. San Antonio, TX Psychological Corporation (1987).
Weintraub, D., Simuni, T., Caspell-Garcia, C., Coffey, C., Lasch, S., Siderowf, A., et al. (2015). Cognitive performance and neuropsychiatric symptoms in early, untreated Parkinson's disease. Mov. Disord. 30, 919–927. doi: 10.1002/mds.26170
Wilson, H., Pagano, G., Yousaf, T., Polychronis, S., de Micco, R., Giordano, B., et al. (2020). Predict cognitive decline with clinical markers in Parkinson's disease (PRECODE-1). J. Neural Transm. (Vienna) 127, 51–59. doi: 10.1007/s00702-019-02125-6
Wood, K. H., Memon, A. A., Memon, R. A., Joop, A., Pilkington, J., Catiul, C., et al. (2021). Slow wave sleep and EEG Delta spectral power are associated with cognitive function in Parkinson's disease. J. Parkinsons Dis. 11, 703–714. doi: 10.3233/JPD-202215
Xie, D., Shen, Q., Zhou, J., and Xu, Y. (2021). Non-motor symptoms are associated with REM sleep behavior disorder in Parkinson's disease: a systematic review and meta-analysis. Neurol. Sci. 42, 47–60. doi: 10.1007/s10072-020-04769-9
Yousaf, T., Pagano, G., Niccolini, F., and Politis, M. (2019). Predicting cognitive decline with non-clinical markers in Parkinson's disease (PRECODE-2). J. Neurol. 266, 1203–1210. doi: 10.1007/s00415-019-09250-y
Keywords: Parkinson disease, cognitive dysfunction, dementia, modifiable risk factors, predictor tool
Citation: Carlisle TC, Medina LD and Holden SK (2023) Original research: initial development of a pragmatic tool to estimate cognitive decline risk focusing on potentially modifiable factors in Parkinson’s disease. Front. Neurosci. 17:1278817. doi: 10.3389/fnins.2023.1278817
Edited by:
Suman Chowdhury, Rutgers, The State University of New Jersey, United StatesReviewed by:
Duncan Street, University of Birmingham, United KingdomVishal Singh, Rutgers University–Newark, United States
Hareram Birla, Rutgers, The State University of New Jersey, United States
Copyright © 2023 Carlisle, Medina and Holden. This is an open-access article distributed under the terms of the Creative Commons Attribution License (CC BY). The use, distribution or reproduction in other forums is permitted, provided the original author(s) and the copyright owner(s) are credited and that the original publication in this journal is cited, in accordance with accepted academic practice. No use, distribution or reproduction is permitted which does not comply with these terms.
*Correspondence: Tara C. Carlisle, Tara.Carlisle@cuanschutz.edu