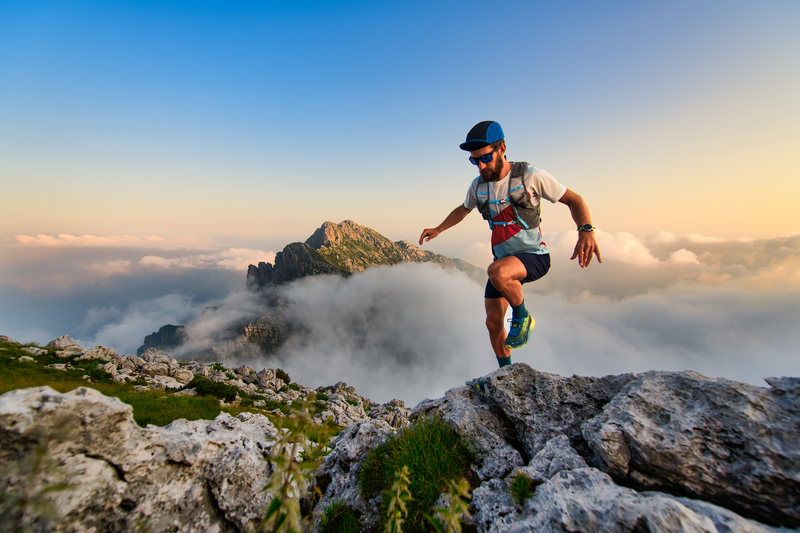
94% of researchers rate our articles as excellent or good
Learn more about the work of our research integrity team to safeguard the quality of each article we publish.
Find out more
SYSTEMATIC REVIEW article
Front. Neurosci. , 22 August 2023
Sec. Translational Neuroscience
Volume 17 - 2023 | https://doi.org/10.3389/fnins.2023.1252651
This article is part of the Research Topic Use of Computerized Gait Analysis in Neurological Pathologies: Volume II View all 4 articles
Background: With the aging of the global population, Spinal injuries are often prone to occur and affect human health. The development of technology has put robots on the stage to assist in the treatment of spinal injuries.
Methods: A comprehensive literature search were carried out in multiple databases, including PubMed, Medline (Ovid), Web of Science, Cochrane, Embase, Scopus, CKNI, Wang fang, VIP database, Sino Med, Clinical Trails until 20th, June, 2023 to collect effect of robot-assisted gait training for patients with spinal cord injury patients. Primary outcome includes any changes of gait distance and gait speed. Secondary outcomes include any changes in functions (Such as TUG, Leg strength, 10 MWT) and any advent events. Data were extracted from two independent individuals and Cochrane Risk of Bias tool version 2.0 was assessed for the included studies. Systematic review and meta-analysis were performed by RevMan 5.3 software.
Results: 11 studies were included in meta-analysis. The result showed that gait distance [WMD = 16.05, 95% CI (−15.73, 47.83), I2 = 69%], gait speed (RAGT vs. regular treatment) [WMD = 0.01, 95% CI (−0.04, 0.05), I2 = 43%], gait speed (RAGT vs. no intervention) [WMD = 0.07, 95% CI (0.01, 0.12), I2 = 0%], leg strength [WMD = 0.59, 95% CI (−1.22, 2.40), I2 = 29%], TUG [WMD = 9.25, 95% CI (2.76, 15.73), I2 = 74%], 10 MWT [WMD = 0.01, 95% CI (−0.15, 0.16), I2 = 0%], and 6 MWT [WMD = 1.79, 95% CI (−21.32, 24.90), I2 = 0%].
Conclusion: Robot-assisted gait training seems to be helpful for patients with spinal cord to improve TUG. It may not affect gait distance, gait speed, leg strength, 10 MWT, and 6 MWT.
Spinal cord injury is a serious neurological injury, the annual global incidence is estimated to be between 250,000 and 500,000 cases (Mekki et al., 2018), usually caused by car accidents, falls, sports accidents, or other external factors. This type of injury can lead to lower limb paralysis or loss of function, which has a significant impact on the quality of life and independence of patients. Previously, spinal cord injury meant being confined to a wheelchair and accompanied by lifelong medical complications. Doctors have very limited treatment options (McDonald and Sadowsky, 2002). Nevertheless, as technology continues to advance, individuals with incomplete para−/tetraplegia can regain the ability (neuroplasticity) to relearn crucial daily activities and reintegrate into the workforce (Dietz and Fouad, 2014). Neuroplasticity refers to the ability of the nervous system to reorganize and adapt in response to changes in demands and environments. This phenomenon occurs during skill acquisition, following nervous system damage, and as a result of sensory deprivation (Bavelier and Neville, 2002; Hötting and Röder, 2013). Neuroplasticity can be promoted through exercise training with the help of the need and/or through electrical stimulation techniques (Alashram et al., 2021). This kind of training helps the existence of physiological Proprioception input of the spinal cord, and the addition of rehabilitation technology (Dietz and Fouad, 2014).
The utilization of neuro-rehabilitation robots in rehabilitation has demonstrated promising clinical outcomes (Hu et al., 2015; Qian et al., 2017; Rong et al., 2017; Demofonti et al., 2021; Selph et al., 2021). Robot-assisted gait training has been shown to enhance neuroplasticity, whereby injured nerves gradually regain their functionality through repetitive training and stimulation (Kuwahara et al., 2022). Secondly, this type of training can enhance the patient’s muscle strength and stability, improve the efficiency and safety of walking (Mıdık et al., 2020). Furthermore, robot-assisted gait training can offer patients confidence and motivation during the initial stages of recovery, thereby reducing feelings of anxiety and depression (Yang et al., 2022). Robot assisted gait training refers to the use of advanced robot technology, combined with the principles of physical therapy, to provide gait training and rehabilitation treatment for patients with spinal cord injury through robot equipment (Calabrò et al., 2021). This training method can provide accurate control and support for patients, helping them recover walking function (Picelli et al., 2021). For example, wearable Exoskeleton or robot walker can provide additional support and stability to assist patients in walking (Swinnen et al., 2010) These devices are usually equipped with sensors and motors that can be adjusted according to the needs and abilities of patients. Through repeated training and gradually increasing challenges, patients can gradually improve muscle strength, balance, and walking coordination (Stampacchia et al., 2022).
However, the effect of robot-assisted gait training for patients with spinal cord injury is still unclear. Therefore, we conduct a systematic review and meta-analysis to assess the effect and safety of robot-assisted gait training for patients with spinal cord injury patients.
Literature searches were conducted in the database of PubMed, Medline (Ovid), Web of Science, Cochrane, Embase, Scopus, CKNI, Wang fang, VIP database, Sino Med, Clinical Trails until 20th, June, 2023. The search strategy of Medline (Ovid) is shown as following (Table 1).
A. Randomized controlled trials (RCTs);
B. Inclusion of people with spinal cord injury; and
C. Patients using robot-assisted gait training as the main treatment or robot-assisted gait training with regular treatment comparing with regular treatment is also acceptable.
A. There is no single variable;
B. observational studies; and
C. lack of sufficient information on baseline
Any changes of gait distance and gait speed.
Any changes in functions (Such as TUG, Leg strength, 10 MWT).
Any advent events.
Two independent reviewers (LB and XB) extracted data using a standardized form that included study demographics, baseline characteristics, study design, intervention methods, outcome measures, and results. Any disagreements were resolved through discussion, and a third review author was consulted if necessary (Sally Green, 2011).
Two authors evaluated the risk of bias in the included study using the Cochrane Handbook for Systematic Reviews of Interventions Version 6.0 (updated July 2019) risk of bias assessment tool. Any discrepancies were resolved through consensus. The assessment tool evaluated seven items, including random sequence generation, assignment concealment, blinding of participants and personnel, blinding of outcome assessment, incomplete outcome data, selective reporting, and other bias. The items were categorized as green, yellow, and red colors and “+,” “−,” “?,” indicating “low,” “high,” and “unclear” risk of bias.
The RevMan 5.3 software was utilized to conduct funnel plots for the assessment of publication bias pertaining to the primary outcome measures.
The Review Manager software (RevMan version 5.3, Cochrane Collaboration, Oxford, UK) was utilized to conduct statistical analyses. The effect quantity used to combine continuous variables in the study was Weighted Mean Difference (WMD) and 95% CI.
Heterogeneity between trial results was tested using p value and I2 statistic. In cases where more than two articles were included, heterogeneity was assessed. If the I2 > 50%, the random effect model was applied based on Clinical heterogeneity. To evaluate the source of heterogeneity, subgroup, sensitivity analysis, and funnel chart were employed. The statistical calculation process was carried out using RevMan5.3 software.
We searched 8 databases including PubMed, Medline (Ovid), Web of Science, Cochrane, Embase, Scopus, CKNI, Wang fang, VIP database, Sino Med and Clinical Trails until 20th, June, 2023. 1,520 Records identified through database searching and 12 records identified through other sources. 528 records are collected after duplicates removed. 22 articles are assessed for eligibility and 11studies are finally involved in meta-analysis (Figure 1).
11 studies characteristics information are collected in Table 2. The difference are discussed by the third author or the whole group.
All inclueded are low risk of selection bias. Due to using different rehabilitation methods, all the studies are high of performance bias and detection bias. All studies are low risk of attritions bias and reporting bias. Some studies are unclear of other bias such are lost of follow-up (See Figure 2).
4 studies mentioned gait distance. The forest plot weight mean difference WMD = 16.05, 95% CI (−15.73, 47.83), I2 = 69%. The funnel plot shows that asymmetric. It may have publication bias. Sensitivity analysis is conducted and show that values included in the literature are all within a reasonable range (Figure 3).
8 studies mentioned gait speed. Subgroud group analysis are used to distinguish different variables. The forest plot weight mean difference (RAGT vs. regular treatment) WMD = 0.01, 95% CI (−0.04, 0.05), I2 = 43%. The forest plot weight mean difference (RAGT vs. no intervention) WMD = 0.07, 95% CI (0.01, 0.12), I2 = 0%. The funnel plot shows that asymmetric. It may have publication bias. Sensitivity analysis is conducted and show that values included in the literature are all within a reasonable range (Figure 4).
6 studies mentioned leg strength. The forest plot weight mean difference WMD = 0.59, 95% CI (−1.22, 2.40), I2 = 29%. The funnel plot shows that asymmetric. It may have publication bias. Sensitivity analysis is conducted and show that values included in the literature are all within a reasonable range (Figure 5).
3 studies mentioned TUG. The forest plot weight mean difference WMD = 9.25, 95% CI (2.76, 15.73), I2 = 74%. The funnel plot shows that asymmetric. It may have publication bias. Sensitivity analysis is conducted and show that values included in the literature are all within a reasonable range (Figure 6).
2 studies mentioned 10 MWT. The forest plot weight mean difference WMD = 0.01, 95% CI (−0.15, 0.16), I2 = 0%. The funnel plot shows that asymmetric. It may have publication bias (Figure 7).
2 studies mentioned 6 MWT. The forest plot weight mean difference WMD = 1.79, 95% CI (−21.32, 24.90), I2 = 0%. The funnel plot shows that asymmetric. It may have publication bias (Figure 8).
No studies have reported the occurrence of adverse events.
1,520 records were found by searching the database, and 11 studies were finally selected for the meta-analysis. All studies included were deemed to have low risk of selection bias. However, due to the use of various rehabilitation methods, physicians inevitably know the differences in intervention methods used. All studies were found to have a high risk of performance bias and detection bias. Additionally, all studies were found to have a low risk of attrition bias and reporting bias. However, some studies had unclear risks of other biases, such as lost follow-up. The result showed that gait distance [WMD = 16.05, 95% CI (−15.73, 47.83), I2 = 69%], gait speed (RAGT vs. regular treatment) [WMD = 0.01, 95% CI (−0.04, 0.05), I2 = 43%], gait speed (RAGT vs. no intervention) [WMD = 0.07, 95% CI (0.01, 0.12), I2 = 0%], leg strength [WMD = 0.59, 95% CI (−1.22, 2.40), I2 = 29%], TUG [WMD = 9.25, 95% CI (2.76, 15.73), I2 = 74%], 10 MWT [WMD = 0.01, 95% CI (−0.15, 0.16), I2 = 0%], and 6 MWT [WMD = 1.79, 95% CI (−21.32, 24.90), I2 = 0%]. Robot-assisted gait training appears to be beneficial in enhancing TUG for patients with spinal cord injuries. However, it may not have an impact on gait distance, gait speed, leg strength, 10 MWT, and 6 MWT. The quality of the evidence level is not high because the included articles have high bias of risks.
According to the current results, the lack of significant aid from machine-assisted rehabilitation can be attributed to several factors (Bowman et al., 2021) Firstly, the current technology for machine-assisted rehabilitation is not advanced enough to fully replace human rehabilitation (Hayes et al., 2018). The equipment’s functions and applicability are limited, making it difficult to cater to the rehabilitation needs of all patients. Secondly, each person’s physical state and rehabilitation requirements are unique, and machine-assisted rehabilitation equipment cannot provide customized plans tailored to individual circumstances (Aguirre-Güemez et al., 2019). Thirdly, professional medical personnel are required to provide guidance and supervision for machine-assisted rehabilitation, and a lack of professional guidance can result in inadequate outcomes (Haji Hassani et al., 2022). Finally, long-term monitoring and evaluation are necessary for the rehabilitation effect of machine-assisted rehabilitation equipment, and the absence of such monitoring can lead to less significant rehabilitation outcomes (Alen et al., 2014).
Although in current research, the effectiveness of robot assisted rehabilitation therapy is not significant. There is still room for research in its development. With the continuous development of technology, the application of robots in the medical field is becoming increasingly extensive. In rehabilitation therapy, robots can provide accurate and precise motion control to assist patients in their recovery training. Robot-assisted rehabilitation therapy has advantages such as providing personalized treatment, enhancing patient engagement, improving treatment outcomes, and reducing the burden on healthcare professionals, making it an important trend in the field of rehabilitation therapy.
Our research has several strengths. While previous Cochrane Reviews have shown that the effect of spinal cord injury, they did not analyze the impact on spinal cord injury (Boldt et al., 2014). Additionally, other meta-analyses have been limited by their use of English-language studies only. In contrast, our systematic review includes studies in Chinese, making it a more comprehensive and up-to-date analysis of the role of robot-assisted gait training for patients with spinal cord injury. However, our study also has some limitations. Firstly, most of the studies we included did not use blinding methods and had a high risk of bias. Secondly, we were unable to assess the specific type of usually PT used in each study due to a lack of randomized controlled trials.
This study has important implications for future research in the field of clinical rehabilitation. To further advance our understanding of the benefits of Tai Chi, it is recommended that future studies include longer-term follow-up periods, as well as more randomized controlled trials and mechanism research. Additionally, many of the studies included in our analysis either inadequately reported or did not clearly report important methodological details such as randomization/allocation concealment and blinding methods. To improve the quality of reporting in future trials, we recommend that researchers adhere to the Consolidated Standards of Reporting Trials (CONSORT) statement (Schulz et al., 2010).
The raw data supporting the conclusions of this article will be made available by the authors, without undue reservation.
LB, XW, and FD collected data. WQ, MiY, and SY performed the analysis and interpretation of data. LB, HJ, and XW wrote the manuscript. MaY designed the study. All authors contributed to the article and approved the submitted version.
This work was supported by Changshu Science and Technology Bureau Project (CS202001) and Changshu Traditional Chinese Medicine Hospital Youth Project (cszyy201914).
The authors declare that the research was conducted in the absence of any commercial or financial relationships that could be construed as a potential conflict of interest.
All claims expressed in this article are solely those of the authors and do not necessarily represent those of their affiliated organizations, or those of the publisher, the editors and the reviewers. Any product that may be evaluated in this article, or claim that may be made by its manufacturer, is not guaranteed or endorsed by the publisher.
6MWT, 6-min walk test; 10MWT, 10-meter walk test; PT, physical therapy; RAGT, robot-assisted gait training; RCTs, randomized controlled trials; TUG, timed up and go.
Aguirre-Güemez, A. V., Pérez-Sanpablo, A. I., Quinzaños-Fresnedo, J., Pérez-Zavala, R., and Barrera-Ortiz, A. (2019). Walking speed is not the best outcome to evaluate the effect of robotic assisted gait training in people with motor incomplete spinal cord injury: a systematic review with meta-analysis. J. Spinal Cord Med. 42, 142–154. doi: 10.1080/10790268.2017.1390644
Alashram, A. R., Annino, G., and Padua, E. (2021). Robot-assisted gait training in individuals with spinal cord injury: a systematic review for the clinical effectiveness of Lokomat. J. Clin. Neurosci. 91, 260–269. doi: 10.1016/j.jocn.2021.07.019
Alcobendas-Maestro, M., Esclarín-Ruz, A., Casado-López, R. M., Muñoz-González, A., Pérez-Mateos, G., González-Valdizán, E., et al. (2012). Lokomat robotic-assisted versus overground training within 3 to 6 months of incomplete spinal cord lesion: randomized controlled trial. Neurorehabil. Neural Repair 26, 1058–1063. doi: 10.1177/1545968312448232
Alen, S. S., Clarke, C. J., AN, M. L., Allan, D. B., and Conway, B. A. (2014). Isometric hip and knee torque measurements as an outcome measure in robot assisted gait training. NeuroRehabilitation 34, 287–295. doi: 10.3233/NRE-131042
Bavelier, D., and Neville, H. J. (2002). Cross-modal plasticity: where and how? Nat. Rev. Neurosci. 3, 443–452. doi: 10.1038/nrn848
Boldt, I., Eriks-Hoogland, I., Brinkhof, M. W., de Bie, R., Joggi, D., and von Elm, E. (2014). Non-pharmacological interventions for chronic pain in people with spinal cord injury. Cochrane Database Syst. Rev. 11:CD009177. Published 2014 Nov 28. doi: 10.1002/14651858.CD009177.pub2
Bowman, T., Gervasoni, E., Amico, A. P., Antenucci, R., Benanti, P., Boldrini, P., et al. (2021). What is the impact of robotic rehabilitation on balance and gait outcomes in people with multiple sclerosis? A systematic review of randomized control trials. Eur. J. Phys. Rehabil. Med. 57, 246–253. doi: 10.23736/S1973-9087.21.06692-2
Calabrò, R. S., Sorrentino, G., Cassio, A., Mazzoli, D., Andrenelli, E., Bizzarini, E., et al. (2021). Robotic-assisted gait rehabilitation following stroke: a systematic review of current guidelines and practical clinical recommendations. Eur. J. Phys. Rehabil. Med. 57, 460–471. doi: 10.23736/S1973-9087.21.06887-8
Demofonti, A., Carpino, G., Zollo, L., and Johnson, M. J. (2021). Affordable robotics for upper limb stroke rehabilitation in developing countries: a systematic review. IEEE Trans. Med. Robot. Bionics. 3, 11–20. doi: 10.1109/TMRB.2021.3054462
Dietz, V., and Fouad, K. (2014). Restoration of sensorimotor functions after spinal cord injury. Brain 137, 654–667. doi: 10.1093/brain/awt262
Duffell, L. D., Brown, G. L., and Mirbagheri, M. M. (2015). Interventions to reduce spasticity and improve function in people with chronic incomplete spinal cord injury: distinctions revealed by different analytical methods. Neurorehabil. Neural Repair 29, 566–576. doi: 10.1177/1545968314558601
Esclarín-Ruz, A., Alcobendas-Maestro, M., Casado-Lopez, R., Perez-Mateos, G., Florido-Sanchez, M. A., Gonzalez-Valdizan, E., et al. (2014). A comparison of robotic walking therapy and conventional walking therapy in individuals with upper versus lower motor neuron lesions: a randomized controlled trial. Arch. Phys. Med. Rehabil. 95, 1023–1031. doi: 10.1016/j.apmr.2013.12.017
Field-Fote, E. C., and Roach, K. E. (2011). Influence of a locomotor training approach on walking speed and distance in people with chronic spinal cord injury: a randomized clinical trial. Phys. Ther. 91, 48–60. doi: 10.2522/ptj.20090359
Haji Hassani, R., Bannwart, M., Bolliger, M., Seel, T., Brunner, R., and Rauter, G. (2022). Real-time motion onset recognition for robot-assisted gait rehabilitation. J. Neuroeng. Rehabil. 19:11. Published 2022 Jan 28. doi: 10.1186/s12984-022-00984-x
Hayes, S. C., James Wilcox, C. R., Forbes White, H. S., and Vanicek, N. (2018). The effects of robot assisted gait training on temporal-spatial characteristics of people with spinal cord injuries: a systematic review. J. Spinal Cord Med. 41, 529–543. doi: 10.1080/10790268.2018.1426236
Hornby, T. G. C. D., Zemon, D. H., and Kahn, J. H. (2005). Clinical and quantitative evaluation of robotic-assisted treadmill walking to retrain ambulation after spinal cord injury. Top. Spinal Cord Inj. Rehabil. 11, 1–17. doi: 10.1310/14Q9-AD7M-FXX9-1G2J
Hötting, K., and Röder, B. (2013). Beneficial effects of physical exercise on neuroplasticity and cognition. Neurosci. Biobehav. Rev. 37, 2243–2257. doi: 10.1016/j.neubiorev.2013.04.005
Hu, X. L., Tong, R. K. Y., Ho, N. S. K., Xue, J. J., Rong, W., and Li, L. S. W. (2015). Wrist rehabilitation assisted by an electromyography-driven neuromuscular electrical stimulation robot after stroke. Neurorehabil. Neural Repair 29, 767–776. doi: 10.1177/1545968314565510
Kuwahara, W., Sasaki, S., Yamamoto, R., Kawakami, M., and Kaneko, F. (2022). The effects of robot-assisted gait training combined with non-invasive brain stimulation on lower limb function in patients with stroke and spinal cord injury: a systematic review and meta-analysis. Front. Hum. Neurosci. 16:969036. Published 2022 Aug 16. doi: 10.3389/fnhum.2022.969036
Labruyere, R., and van Hedel, H. J. (2014). Strength training versus robot-assisted gait training after incomplete spinal cord injury: a randomized pilot study in patients depending on walking assistance. J. Neuroeng. Rehabil. 11:4. doi: 10.1186/1743-0003-11-4
Lam, T., Pauhl, K., Ferguson, A., Malik, R. N., BKin, Krassioukov, A., et al. (2015). Training with robot-applied resistance in people with motor-incomplete spinal cord injury: pilot study. J. Rehabil. Res. Dev. 52, 113–130. doi: 10.1682/JRRD.2014.03.0090
McDonald, J. W., and Sadowsky, C. (2002). Spinal-cord injury. Lancet 359, 417–425. doi: 10.1016/S0140-6736(02)07603-1
Mekki, M., Delgado, A. D., Fry, A., Putrino, D., and Huang, V. (2018). Robotic rehabilitation and spinal cord injury: a narrative review. Neurotherapeutics 15, 604–617. doi: 10.1007/s13311-018-0642-3
Mıdık, M., Paker, N., Buğdaycı, D., and Mıdık, A. C. (2020). Effects of robot-assisted gait training on lower extremity strength, functional independence, and walking function in men with incomplete traumatic spinal cord injury. Turk. J. Phys. Med. Rehabil. 66, 54–59. Published 2020 Mar 3. doi: 10.5606/tftrd.2020.3316
Niu, X., Varoqui, D., Kindig, M., and Mirbagheri, M. M. (2014). Prediction of gait recovery in spinal cord injured individuals trained with robotic gait orthosis. J. Neuroeng. Rehabil. 11:42. doi: 10.1186/1743-0003-11-42
Picelli, A., Capecci, M., Filippetti, M., Varalta, V., Fonte, C., di Censo, R., et al. (2021). Effects of robot-assisted gait training on postural instability in Parkinson's disease: a systematic review. Eur. J. Phys. Rehabil. Med. 57, 472–477. doi: 10.23736/S1973-9087.21.06939-2
Qian, Q., Hu, X., Lai, Q., Ng, S. C., Zheng, Y., and Poon, W. (2017). Early stroke rehabilitation of the upper limb assisted with an electromyography-driven neuromuscular electrical stimulation-robotic arm. Front. Neurol. 8, 1–13. doi: 10.3389/fneur.2017.00447
Rong, W., Li, W., Pang, M., Hu, J., Wei, X., Yang, B., et al. (2017). A neuromuscular electrical stimulation (NMES) and robot hybrid system for multi-joint coordinated upper limb rehabilitation after stroke. J. Neuroeng. Rehabil. 14, 1–13. doi: 10.1186/s12984-017-0245-y
Sally Green, J. P. H. (2011). Cochrane handbook for systematic reviews of interventions version 5.1.0. The Cochrane Collaboration.
Schulz, K. F., Altman, D. G., and Moher, D., CONSORT Group (2010). CONSORT 2010 statement: updated guidelines for reporting parallel group randomised trials. BMC Med. 8:18. doi: 10.1186/1741-7015-8-18
Selph, S. S., Skelly, A. C., Wasson, N., Dettori, J. R., Brodt, E. D., Ensrud, E., et al. (2021). Physical activity and the health of wheelchair users: a systematic review in multiple sclerosis, cerebral palsy, and spinal cord injury. Arch. Phys. Med. Rehabil. 102, 2464–2481.e33. doi: 10.1016/j.apmr.2021.10.002
Shin, J. C., Kim, J. Y., Park, H. K., and Kim, N. Y. (2014). Effect of robotic-assisted gait training in patients with incomplete spinal cord injury. Ann. Rehabil. Med. 38, 719–725. doi: 10.5535/arm.2014.38.6.719
Stampacchia, G., Gazzotti, V., Olivieri, M., Andrenelli, E., Bonaiuti, D., Calabro, R. S., et al. (2022). Gait robot-assisted rehabilitation in persons with spinal cord injury: a scoping review. NeuroRehabilitation 51, 609–647. doi: 10.3233/NRE-220061
Swinnen, E., Duerinck, S., Baeyens, J. P., Meeusen, R., and Kerckhofs, E. (2010). Effectiveness of robot-assisted gait training in persons with spinal cord injury: a systematic review. J. Rehabil. Med. 42, 520–526. doi: 10.2340/16501977-0538
Tang, Q., Huang, Q., and Hu, C. (2014). Research on design theory and compliant control for Underactuated lower-extremity rehabilitation robotic systems code: (51175368); 2012.01-2015.12. J. Phys. Ther. Sci. 26, 1597–1599. doi: 10.1589/jpts.26.1597
Varoqui, D., Niu, X., and Mirbagheri, M. M. (2014). Ankle voluntary movement enhancement following robotic-assisted locomotor training in spinal cord injury. J. Neuroeng. Rehabil. 11:46. doi: 10.1186/1743-0003-11-46
Yang, F. A., Chen, S. C., Chiu, J. F., Shih, Y. C., Liou, T. H., Escorpizo, R., et al. (2022). Body weight-supported gait training for patients with spinal cord injury: a network meta-analysis of randomised controlled trials. Sci. Rep. 12:19262. Published 2022 Nov 10. doi: 10.1038/s41598-022-23873-8
Keywords: robot-assisted, gait training, spinal cord injury, gait distance, gait speed
Citation: Bin L, Wang X, Jiatong H, Donghua F, Qiang W, Yingchao S, Yiming M and Yong M (2023) The effect of robot-assisted gait training for patients with spinal cord injury: a systematic review and meta-analysis. Front. Neurosci. 17:1252651. doi: 10.3389/fnins.2023.1252651
Received: 04 July 2023; Accepted: 28 July 2023;
Published: 22 August 2023.
Edited by:
Bo Li, Sun Yat-sen University, ChinaReviewed by:
Nicoleta Negrut, University of Oradea, RomaniaCopyright © 2023 Bin, Wang, Jiatong, Donghua, Qiang, Yingchao, Yiming and Yong. This is an open-access article distributed under the terms of the Creative Commons Attribution License (CC BY). The use, distribution or reproduction in other forums is permitted, provided the original author(s) and the copyright owner(s) are credited and that the original publication in this journal is cited, in accordance with accepted academic practice. No use, distribution or reproduction is permitted which does not comply with these terms.
*Correspondence: Ma Yong, ZnN5eTAxMDMxQG5qdWNtLmVkdS5jbg==
†These authors have contributed equally to this work
Disclaimer: All claims expressed in this article are solely those of the authors and do not necessarily represent those of their affiliated organizations, or those of the publisher, the editors and the reviewers. Any product that may be evaluated in this article or claim that may be made by its manufacturer is not guaranteed or endorsed by the publisher.
Research integrity at Frontiers
Learn more about the work of our research integrity team to safeguard the quality of each article we publish.