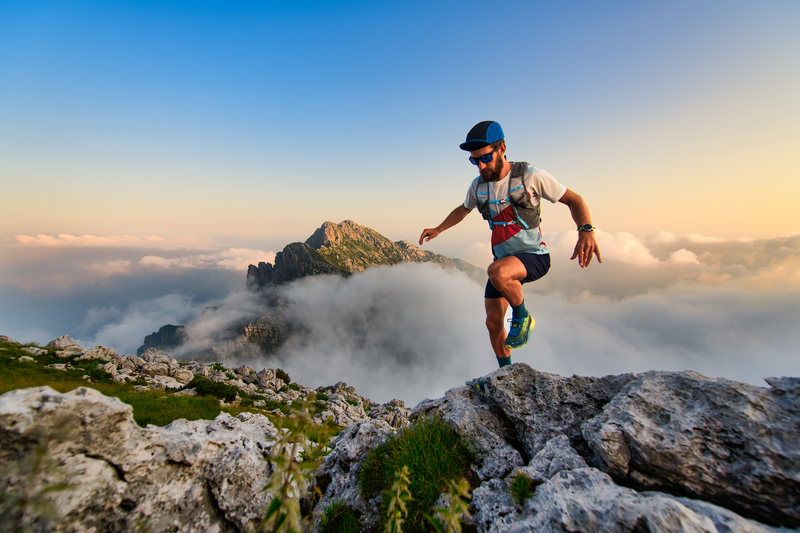
94% of researchers rate our articles as excellent or good
Learn more about the work of our research integrity team to safeguard the quality of each article we publish.
Find out more
EDITORIAL article
Front. Neurosci. , 30 May 2023
Sec. Brain Imaging Methods
Volume 17 - 2023 | https://doi.org/10.3389/fnins.2023.1222586
This article is part of the Research Topic Novel Perspectives and Improvements in fMRI Functional Connectivity Analysis Methods Used to Investigate Brain Networks and Cognitive Mechanisms in Humans View all 8 articles
Editorial on the Research Topic
Novel perspectives and improvements in fMRI functional connectivity analysis methods used to investigate brain networks and cognitive mechanisms in humans
Over the last decade, there has been growing interest and important developments for characterization of the brain connectome to obtain useful and meaningful information while exploring a wide range of pathological conditions and cognitive mechanisms (Bijsterbosch et al., 2021; Bernstein-Eliav and Tavor, 2022; Srivastava et al., 2022). The brain connectome can be investigated using various connectivity measures such as structural (anatomical) and functional (neuronal) connectivity within and between regions in the brain (Babaeeghazvini et al., 2021). Although functional connectivity (FC) can be shaped by structural connectivity (Honey et al., 2010), the interaction between two models may vary depending on the underlying cognitive mechanisms (Litwińczuk et al., 2022; Liu et al., 2023).
In this collection, we present novel perspectives and methods for analysis of functional connectivity modeled by functional MRI (fMRI) techniques which measure localized increase in blood oxygenation levels reflecting the increase in neuronal activity in the brain. The Research Topic “Novel Perspectives and Improvements in fMRI Functional Connectivity Analysis Methods Used to Investigate Brain Networks and Cognitive Mechanisms” consists of a collection of 7 contributions discussing new methods and systems for FC analysis of brain networks to explore the underlying cognitive mechanisms, and report the recent advances in brain research.
The first paper by Wang Q. et al. explores the evolution of intra- and inter-network FC in drug-naive Parkinson's disease (PD) patients with different motor subtypes. This study shows that FC varying among anterior and posterior salience networks can be an imaging marker to distinguish PD with tremor dominant from PD with postural instability and gait disorder. Their methods and results provide a basis that can lead to novel approaches for identification and precision treatment of PD motor subtypes.
In the second paper, Fang et al. first give a detailed review of the related work including applications and toolboxes used for analysis of multivariate connectivity of brain networks. Then, the authors discuss the challenges of modeling non-linear complex neural dynamics of cognitive processes in the brain. Finally, they characterize the steps that can be followed to model non-linear relationships between representations encoded in different brain regions. The proposed steps and open problems can guide researchers to develop novel multivariate connectivity models of non-linear dynamics of the brain.
The third paper by Li et al. investigates changes of brain networks in epilepsy patients without intracranial lesions under resting conditions using graph theoretic methods and methods from statistical signal processing such as independent component analysis (ICA). In the analyses, they observe that the topological characteristics and FC of brain networks are changed in non-lesional epilepsy patients. More precisely, the results suggest that reduced efficiency between brain networks in epilepsy patients and a compensatory response to brain function at earlier stages of the disease can be detected by abnormal FC. Their proposed methods and results can help researchers to identify and explore exclusive brain networks for different types of epilepsy.
In the fourth paper, de Rijk et al. examine homologous clusters in the periaqueductal gray (PAG), which is a brain stem area designated to play an essential role in lower urinary tract (LUT) control, between subjects. To explore the relationship between LUT symptoms, such as urgency, and activity patterns in the PAG in normal and pathological states, they propose employment of various clustering and cluster analysis methods. At the within-subject level, the results suggest that resting-state fMRI data of the PAG can be used to obtain clusters that show some of the anatomical characteristics known from animal and post-mortem studies. At the group level, they observe that PAG clusters additionally show high spatial organizational similarity. Their proposed analyses and findings can help to improve current therapies and patient selection strategies, and lead to the development of new therapies.
The fifth paper by Wang Y. et al. investigates how phase-encoding (PE) direction in echo-planar imaging on gender differences can affect the outcome of a specific research on gender differences. In the analyses, they found that PE direction can partially influence gender differences in spontaneous brain activity of resting-state fMRI. The findings suggest appropriate selection of PE direction as an important criterion to be utilized in resting-state fMRI studies.
In the next paper, Cordes et al. study naturally occurring frequency bands of resting-state data obtained by group ICA using Empirical Mode Decomposition (EMD) which is an adaptive time-frequency method. They show that the intrinsic mode functions of blood-oxygenation level-dependent (BOLD) fMRI signals provide characteristic energy-period profiles that allow a data-driven arrangement of all resting-state networks which is not possible using the non-adaptive methods. The experimental results expand the current understanding of network dynamics in PD. Their proposed novel approach to understanding functional networks in PD, can lead to development of a clinically useful in vivo assay of PD network physiology.
In the last paper, Pillet et al. examine the similarities between neural networks derived from representational similarity analysis (RSA) with those from Univariate analysis (UNIVAR) and functional connectivity analysis (FCA) to explore how these methods relate to each other. This work provides first-time evidence that cortical networks derived from three commonly used neuroimaging approaches (RSA, UNIVAR, and FCA) are highly similar regardless of the structural variations of each network. Improving the understanding of the relationship between the functional networks derived from these methods will allow researchers to use these approaches more adequately.
These articles have made outstanding contributions to brain research and applications. We hope that our Research Topic can inspire new studies on the brain analysis leading to further advances in neuroscience using network theory, graph theory, machine learning and statistical signal processing.
MO has made a substantial, direct, and intellectual contribution to the work and approved it for publication.
MO is employed by Samsung Research.
All claims expressed in this article are solely those of the authors and do not necessarily represent those of their affiliated organizations, or those of the publisher, the editors and the reviewers. Any product that may be evaluated in this article, or claim that may be made by its manufacturer, is not guaranteed or endorsed by the publisher.
Babaeeghazvini, P., Rueda-Delgado, L. M., Gooijers, J., Swinnen, S. P., and Daffertshofer, A. (2021). Brain structural and functional connectivity: a review of combined works of diffusion magnetic resonance imaging and electro-encephalography. Front. Hum. Neurosci. 15, 721206. doi: 10.3389/fnhum.2021.721206
Bernstein-Eliav, M., and Tavor, I. (2022). The prediction of brain activity from connectivity: advances and applications. Neuroscientist. doi: 10.1177/10738584221130974
Bijsterbosch, J. D., Valk, S. L., Wang, D., and Glasser, M. F. (2021). Recent developments in representations of the connectome. Neuroimage 243, 118533. doi: 10.1016/j.neuroimage.2021.118533
Honey, C. J., Thivierge, J. P., and Sporns, O. (2010). Can structure predict function in the human brain? Neuroimage 52, 766–776. doi: 10.1016/j.neuroimage.2010.01.071
Litwińczuk, M. C., Muhlert, N., Cloutman, L., Trujillo-Barreto, N., and Woollams, A. (2022). Combination of structural and functional connectivity explains unique variation in specific domains of cognitive function. Neuroimage 262, 119531. doi: 10.1016/j.neuroimage.2022.119531
Liu, X., Qiu, S., Wang, X., Chen, H., Tang, Y., and Qin, Y. (2023). Aberrant dynamic functional-structural connectivity coupling of large-scale brain networks in poststroke motor dysfunction. Neuroimage Clin. 37, 103332. doi: 10.1016/j.nicl.2023.103332
Keywords: fMRI, brain networks, cognitive mechanisms, functional connectivity, brain connectivity
Citation: Ozay M (2023) Editorial: Novel perspectives and improvements in fMRI functional connectivity analysis methods used to investigate brain networks and cognitive mechanisms in humans. Front. Neurosci. 17:1222586. doi: 10.3389/fnins.2023.1222586
Received: 14 May 2023; Accepted: 17 May 2023;
Published: 30 May 2023.
Edited and reviewed by: Vince D. Calhoun, Georgia State University, United States
Copyright © 2023 Ozay. This is an open-access article distributed under the terms of the Creative Commons Attribution License (CC BY). The use, distribution or reproduction in other forums is permitted, provided the original author(s) and the copyright owner(s) are credited and that the original publication in this journal is cited, in accordance with accepted academic practice. No use, distribution or reproduction is permitted which does not comply with these terms.
*Correspondence: Mete Ozay, bS5vemF5QHNhbXN1bmcuY29t
Disclaimer: All claims expressed in this article are solely those of the authors and do not necessarily represent those of their affiliated organizations, or those of the publisher, the editors and the reviewers. Any product that may be evaluated in this article or claim that may be made by its manufacturer is not guaranteed or endorsed by the publisher.
Research integrity at Frontiers
Learn more about the work of our research integrity team to safeguard the quality of each article we publish.