- 1Department of Radiology, First Affiliated Hospital of Nanchang University, Nanchang, Jiangxi, China
- 2Clinical Research Center for Medical Imaging in Jiangxi Province, Nanchang, China
- 3Department of Radiology, Nanchang First Hospital, Nanchang, Jiangxi, China
- 4GE Healthcare, MR Research China, Beijing, China
Purpose: White matter hyperintensity lesions (WMHL) in the brain are a consequence of cerebral small vessel disease and microstructural damage. Patients with WMHL have diverse clinical features, and hypertension, advanced age, obesity, and cognitive decline are often observed. However, whether these clinical features are linked to interrupted structural connectivity in the brain requires further investigation. This study therefore explores the white matter pathways associated with WMHL, with the objective of identifying neural correlates for clinical features in patients with WMHL.
Methods: Diffusion magnetic resonance imaging (MRI) and several clinical features (MoCA scores, hypertension scores, body mass index (BMI), duration of hypertension, total white matter lesion loads, and education.) highly related to WMHL were obtained in 16 patients with WMHL and 20 health controls. We used diffusion MRI connectometry to explore the relationship between clinical features and specific white matter tracts using DSI software.
Results: The results showed that the anterior splenium of the corpus callosum, the inferior longitudinal fasciculus, the anterior corpus callosum and the middle cerebellar peduncle were significantly correlated with hypertension scores (false discovery rate (FDR) = 0.044). The anterior splenium of the corpus callosum, the left thalamoparietal tract, the inferior longitudinal fasciculus, and the left cerebellar were significantly correlated with MoCA scores (FDR = 0.016). The anterior splenium of corpus callosum, inferior fronto-occipital fasciculus, cingulum fasciculus, and fornix/fimbria were significantly correlated with body mass index (FDR = 0.001).
Conclusion: Our findings show that hypertension score, MoCA score, and BMI are important clinical features in patients with WMHL, hypertension degree and higher BMI are associated with whiter matter local disconnection in patients with WMHL, and may contribute to understanding the cognitive impairments observed in patients with WMHL.
Introduction
White matter hyperintensities lesions (WMHL) are characterized by multiple punctate, patchy, or confluent hyperintensities in the bilateral periventricular or subcortical white matter on T2-weighted images or T2-weighted fluid-attenuated inversion recovery images (T2-Flair) and are one of the common imaging markers of cerebral small vessel disease (CSVD) (Wardlaw et al., 2013). Various factors contribute to WMHL formation, including age, female sex, hypertension, hyperlipidemia, apolipoprotein Eε4 allele, diabetes, smoking, and alcohol consumption (Alber et al., 2019; Frey et al., 2019). Previous studies have demonstrated that WMHL are associated with an increased risk of stroke, cognitive decline, dementia, and death (Debette and Markus, 2010). Even in healthy aging, higher whole-brain lesion volume and regional WMHL load are consistently associated with poorer cognitive performance in processing speed, memory, and executive functioning (van Rooden et al., 2018).
Previous studies have shown anomalies not only within T2-WMHL but also in apparently “normal appearing white matter (NAWM)” (Maillard et al., 2011). Over time, abnormal changes in NAWM precede WMHL progression, known as WMHL penumbra (Wu et al., 2019). Cognitive impairment in patients with WMHL is correlated with microstructural destruction of various white matter fibers, which may include “disconnection” of cortical–subcortical pathways (Yuan et al., 2017; Lu et al., 2021), and the relationship between overall cognitive function and white matter integrity may be closer than that with blood supply (Zhong et al., 2017).
Serious vascular diseases can lead to cortical and subcortical infarction, affect brain functional areas, and result in vascular cognitive impairment (Frantellizzi et al., 2020; Meng et al., 2022). Compared to all other regions, the front permanent WMHL has more pronounced diffusion tensor imaging metrics changes with the same Fazekas grades, which may represent the effects of severe demyelination within the frontal periventricular WMHL (Min et al., 2021). The results also indicate an increased WMHL burden selectively in deep white matter in obese subjects with high visceral fat accumulation. Independent of common obesity comorbidities such as hypertension, it may be that the visceral obesity contributes to deep white matter lesions through increases in proinflammatory cytokines (Min et al., 2021). In fact, most patients with WMHL may not lead to typical clinical symptoms because of collateral circulation development in these ischemic regions.
However, consensus is lacking as to regarding which etiology or clinical characteristic and corresponding white matter tract provide the most accurate reflection of patients with WMHL and may be used as neural correlates (white matter fiber bundle segments correlated with study variables) to assist clinical diagnosis. We hypothesized that even asymptomatic patients with WMHL may have microstructural impairment. This study intends to use the diffusion MRI connectome analysis method to identify neural correlates that reflect the underlying deficits in WMHL and to identify the most representative neural correlates for WMHL.
Materials and methods
Subjects
All subjects provided signed, informed consent prior to inclusion in the study in accordance with the local institutional review board. From May 2020 to December 2021, patients with WHM (n = 16) from inpatient were recruited to the first affiliated hospital of Nanchang University. The inclusion criteria for WMHL subjects were as follows: (1) presence of grade 2 or 3 WMHL according to Fazekas scale on FLAIR; (2) no contraindications to MRI. Exclusion criteria were as follows: (1) history of ischemic stroke with infarct size greater than 1.5 cm in diameter or cardiogenic cerebral embolism, (2) cerebral hemorrhage, (3) internal carotid artery or vertebral artery stenosis (>50%) or coronary atherosclerosis heart disease, (4) WMHL due to immune-mediated inflammatory demyelinating disease (multiple sclerosis, neuromyelitis optica, acute disseminated encephalomyelitis), metabolic leukodystrophy and genetic leukoencephalopathy, and (5) other neurological disorders.
Healthy controls (HC, n = 20) were randomly recruited, who had no history of hypertensive disease, traumatic brain injurie, neurologic diseases, or brain abnormalities based on conventional MRI (T1 weight image, T2 weight image, T2 fluid attenuated inversion recovery).
Image acquisition
A total of 36 diffusion MRI scans were included in the connectometry database. The diffusion images were obtained on a 3 T MR system (Premier, GE Healthcare, Madison, Wisconsin) using a 2D EPI diffusion sequence. Foam padding was used to position subjects on the coil so that the cervical spine was straight but comfortable to minimize head motion. The spatial resolution was 0.875 mm × 0.875 mm × 2.5 mm isotropic. TR = 5,500 ms, TE =71 ms. FOV 224 mm × 224 mm. The diffffusion data was acquired with 100 directions with b-values (3,000 s/mm2). Thickness of the slice was 2 mm. Axial conventional T1WI, T2WI, T2-FLAIR (fluid-attenuated inversion recovery) were acquired in the brain for each patient’s diagnosis. The following scan parameters were used: axial T1WI: TR = 250 msec, TE = 2.46 msec, slice number = 19, slice thickness = 5.0 mm, FOV = 24 × 24 cm, matrix size = 256 × 256; axial T2WI: repetition time (TR) = 4,000 msec, echo time (TE) = 113 msec, slice number = 19, slice thickness = 5.0 mm, field of view (FOV) = 24 × 24 cm, matrix size = 256 × 256; axial T2-FLAIR: TR =7,000 msec, TE = 162 msec, slice number = 19, slice thickness = 5.0 mm, FOV = 24 × 24 cm, and matrix size = 256 × 256.
Lesion load, hypertension scores assessments
A binary lesion mask was manually drawn from T2WI to identify all visible lesions by an experienced neuroradiologist (F.Z.) using MRIcron. The T2WI was coregistered with the T1-weighted structural image. After co-registration with the T1WI-based individual brain according to the Montreal Neurological Institute (MNI) standard brain dimensions, and this information was used to warp the lesion mask [as the normalized total white matter lesion load (TWMLL)] reflected the TWMLL relative to the standard MNI brain volume rather than the individual brain volume so that the effects of differences in brain volume were controlled (Pelletier et al., 2004).
According to the diagnosis of hypertension and the cardiovascular risk factors stratification standard (Williams et al., 2018), hypertension is divided into Grade I, Grade II, and Grade III, and we give corresponding scores of 1, 2 and 3; the degree of danger includes low risk, medium risk, high risk and very high risk, and the corresponding scores are 1, 2, 3, and 4. Total hypertension score is equal to hypertension level score plus hypertension risk score. Based on patient admission diagnosis, the score of the WMHL patient is 2–5, as shown in Table 1.
Demographic, BMI, clinical, and neuropsychological assessments
Demographic, clinical, and neuropsychological characteristics, including age, sex, disease duration (From the first diagnosis of the disease to enrollment), height (m) and weight (kg), Montreal Cognitive Assessment (MoCA). body mass index (BMI) is a person’s weight in kilograms divided by the square of height in meters (kg/m2).
Diffusion MRI connectometry analysis
The diffusion data were reconstructed in the MNI space using q-space diffeomorphic reconstruction (Yeh and Tseng, 2011) to obtain the spin distribution function (SDF) (Yeh et al., 2010) using DSI Studio.1 With the default settings, a diffusion sampling length ratio of 1.25 was used. The sdf values were used in the connectometry analysis.
Diffusion MRI connectometry analysis enabled us to further investigate the specific pathways associated with study variable (Yeh et al., 2016). In this study, our study variables included hypertension scores, MoCA scores, BMI, TWMLL, duration of hypertension, and education. Because most study variable were highly correlated with each other, we first conducted a principal component analysis (PCA) to isolate the principal components that explained overall score variability. This avoided overfitting in the regression model. A multiple regression model was used to adjust for sex and age, and the following default parameters were used. At the group level, we used concatenated HC and WMHL data to create a connectometry database. Considering of higher length thresholds provide more specific results for identifying affected tracks, while lower length thresholds are more sensitive to potentially affected tracks. In our study, different length thresholds (30 mm, 40 mm, and 50 mm) were used to study the correlation at different significance levels between the two groups. To further analyze the study variables associated with tracks, we only used WMHL group data to create a connectometry database, a 40 mm length threshold was used to select tracks. The seeding density was 20 seeds per mm3. Local connectomes were tracked using a deterministic fiber tracking algorithm. Track trimming was performed with 2 iterations. All tracks generated from bootstrap resampling were included. To estimate the false discovery rate (FDR), 2000 random permutations were applied to the group label to obtain the null distribution of the track length. An FDR lower than 0.05 can be considered as significant.
Results
Demographic and clinical data
Sixteen patients with WMHL and 20 HC were included in this study. Population and clinical characteristics are summarized in Table 1. The WMHL has significantly lower MOCA scores and higher BMI than HC (respective p = 0.000 and p = 0.003).
Principle component analysis
The PCA indicated that only three components were sufficient to explain 87.196% of the score variance. The first component was hypertension scores, with a coefficient of 0.712, which explained 32.936% of the behavioral data. The second component was MoCA, with a coefficient of 0.812, which explained 26.542% of the behavioral data. The third component was BMI, with a coefficient of 0.825, which explained the other 27.718%. The results are shown in Table 2.
Tracks correlated with group
We use different length thresholds (30 mm, 40 mm, and 50 mm) to study the correlation at different significance levels between the two groups. Figure 1 shows the WMHL group had decreased fiber connectivity in the brain network compared with the HC. The FDR was 0.023, 0.013, 0.020 when the length was 30 mm, 40 mm, 50 mm, respectively. The analysis showed that the differential tractography involving bilateral association bundles, corpus callosum, projection bundles and cerebellar peduncle.
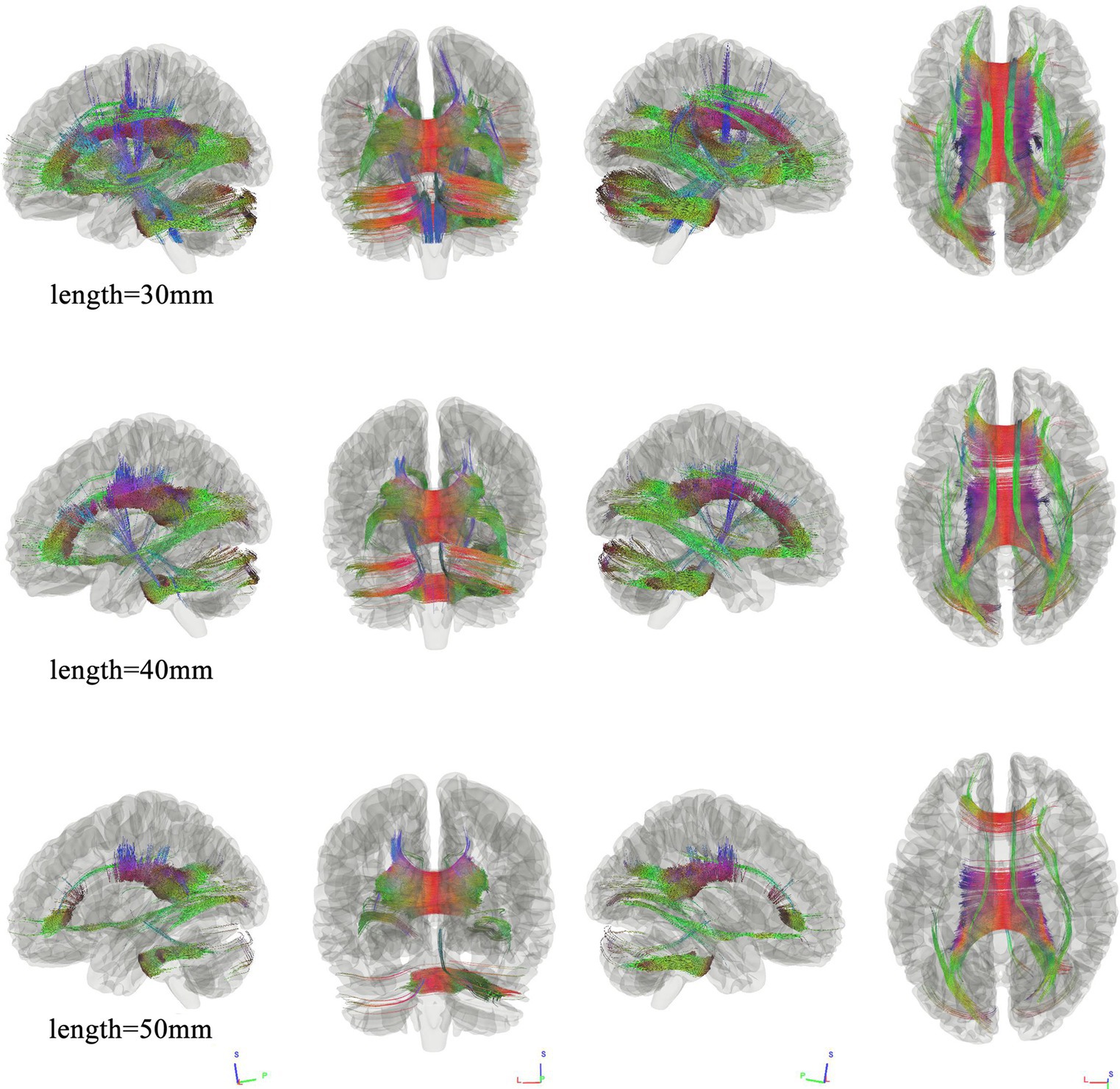
Figure 1. Connectometry results under different length thresholds between the two groups. Connectometry analysis identified connectivity differences that appeared and wide-spread the whole brain, involving bilateral association bundles, corpus callosum, projection bundles, and cerebellar peduncle. (FDR was 0.023, 0.013, 0.020 when the length was 30 mm, 40 mm, 50 mm, respectively).
Tracks correlated with hypertension scores
We first used connectometry analysis to map the fiber pathways correlated with the first component of PCA, which was predominantly weighted by the hypertension score. Figure 2 shows the tracks negatively correlated with hypertension scores. The FDR was 0.044. The analysis indicated that the pathways correlated with hypertension cores were anterior splenium of corpus callosum (ASCC), bilateral inferior longitudinal fasciculus(ILF), anterior corpus callosum (ACC), middle cerebellar peduncle.
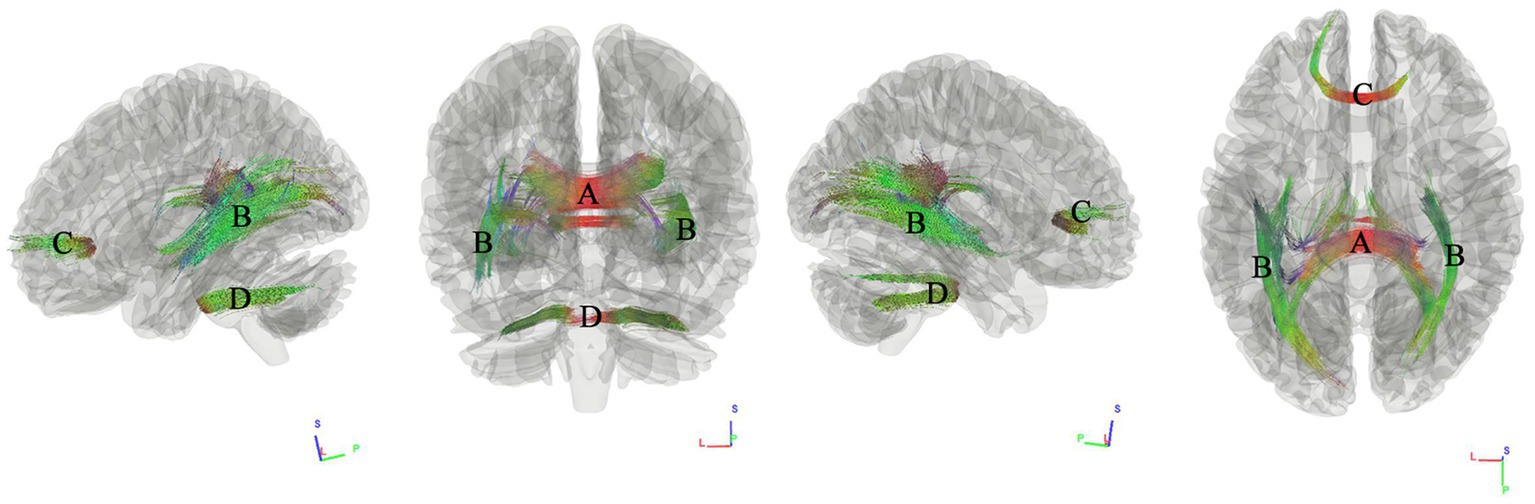
Figure 2. Tracks negatively correlated with hypertension scores in patients with WMHL. Connectometry analysis identified (A) anterior splenium of corpus callosum, (B) inferior longitudinal fasciculus, (C) anterior corpus callosum, (D) middle cerebellar peduncle with decreased connectivity to hypertension cores (FDR = 0.044).
Tracks correlated with MoCA
We used connectometry analysis to map fiber pathways correlated with the second PCA component, which was predominantly weighted by the MoCA scores. Figure 3 shows the tracks negatively correlated with MoCA scores. The FDR was 0.016. The analysis indicated that the pathways correlated with MoCA scores were ASCC, left thalamoparietal pathways, right inferior longitudinal fasciculus ILF, and left cerebellar.
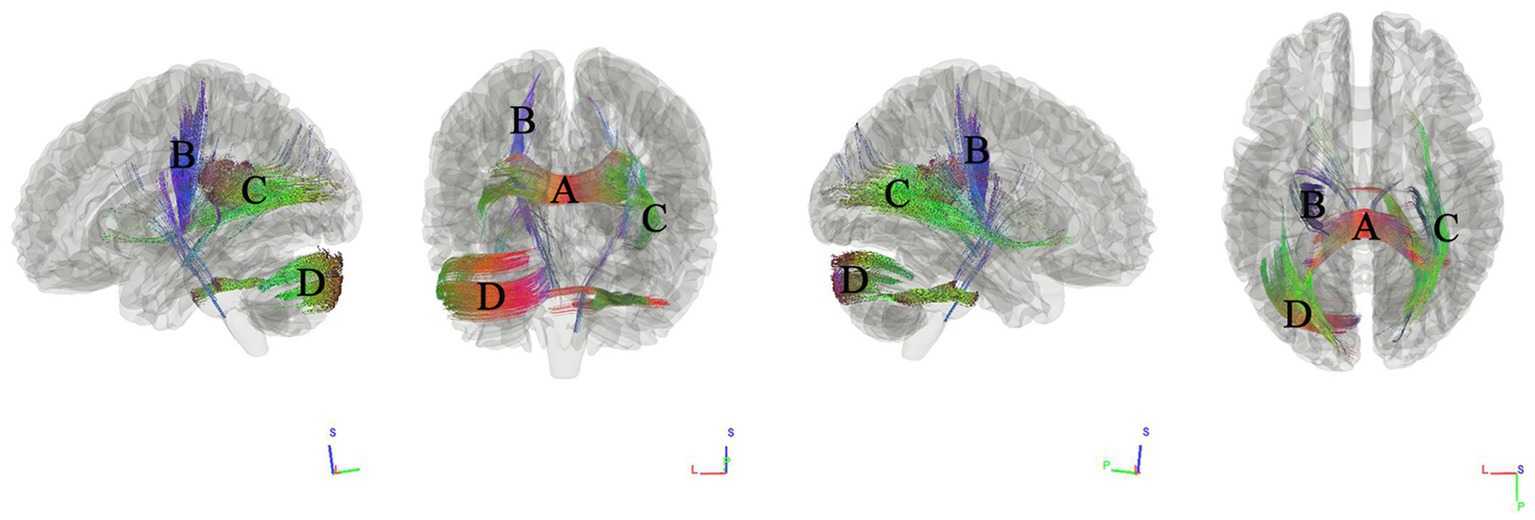
Figure 3. Tracks negatively correlated with MoCA scores in patients with WMHL. Connectometry analysis identified (A) anterior splenium of the corpus callosum, (B) left thalamoparietal pathways, (C) right inferior longitudinal fasciculus, and (D) left cerebellar with decreased connectivity to MoCA scores (FDR = 0.016).
Tracks correlated with BMI
We used connectometry analysis to map the fiber pathways correlated with the third PCA component, which was predominantly weighted by the BMI. Figure 4 shows the tracks negatively correlated with BMI. The FDR was 0.001. The analysis indicated that the pathways correlated with BMI were ASCC, bilateral inferior fronto-occipital fasciculus (IFOF), bilateral cingulum fasciculus, fornix/fimbria.
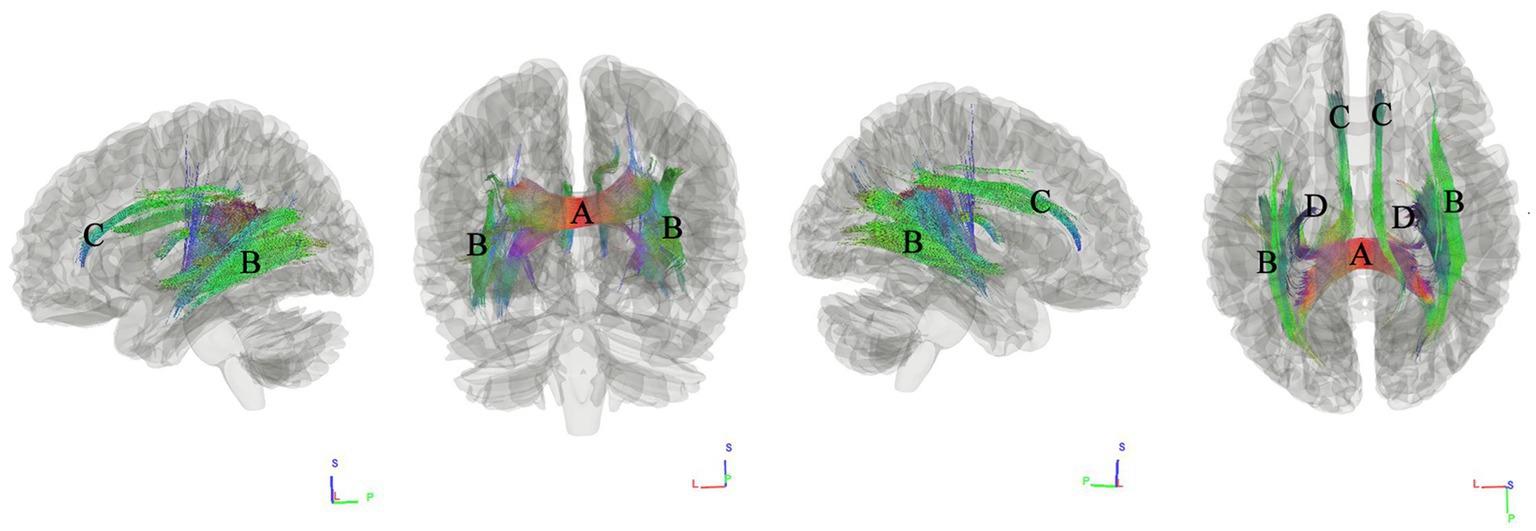
Figure 4. Tracks negatively correlated with BMI in patients with WMHL. Connectometry analysis identified (A) the anterior splenium of the corpus callosum, (B) inferior fronto-occipital fasciculus, (C) cingulum fasciculus, and (D) fornix/fimbria with decreased connectivity to BMI (FDR = 0.001).
Discussion
This study provides evidence for the association between white matter fibers and hypertension scores, MoCA scores, and BMI in patients with WMHL. Anatomically, ASCC, bilateral ILF, ACC, and middle cerebellar peduncle were significantly correlated with hypertension scores. ASCC, left thalamoparietal pathways, right ILF, and left cerebellar were significantly correlated with MoCA scores. ASCC, IFOF, cingulum fasciculus, fornix/fimbria were significantly correlated with BMI.
Hypertension is a well-known significant risk factor for WMHL, which is closely related to WMHL progression. Among hypertensive individuals, the prevalence of WMHL may rise to 40–44% (Liao et al., 1996; Verhaaren et al., 2013; Gebeily et al., 2014; Sargurupremraj et al., 2020). In addition, hypertension is highly relevant to the annual progression of WMHL. People with uncontrolled untreated hypertension had significantly more WMHL progression than regular treatment (Verhaaren et al., 2013; Shen et al., 2022). In our study, we found that decreased connectivity in ASCC, bilateral ILF, ACC, and middle cerebellar peduncle were significantly negatively correlated with hypertension scores. Previous studies have reported the relationship between hypertension and lower microstructural fiber density, macrostructural fiber bundle cross-section, and a combination of both, such as corpus callosum, ILF, and middle cerebellar peduncle (Gons et al., 2012; Li et al., 2015; Wong et al., 2017; Andica et al., 2022). Studies have also found impaired microstructural integrity of the corpus callosum in hypertensive patients compared to normotensive patients in individuals with cerebral small vessel disease (Gons et al., 2012). This may be related to the vulnerability of posterior brain regions to vascular risk factors (Artero et al., 2004). The fibers in splenium are projections from the occipital-parietal and temporal cortex. It has been shown to be involved in language, reading, and calculation skills, IQ, conduct, and consciousness (Blaauw and Meiners, 2020). The ILF is a long-range white matter pathway that primarily connects the occipital lobe of the brain with the anterior temporal lobe. It may involve a wide range of brain functions concerning the visual modality, including object, face and place processing, reading, lexical and semantic processing, emotion processing, and visual memory (Herbet et al., 2018). Previous studies have mostly found that hypertension shows significantly reduced white matter integrity in the bilateral superior longitudinal fasciculus (Li et al., 2015). In patients with WMHL with higher FA values, the integrity of the ILF moderates the association between higher WMHL and slower gait speed (Rosario et al., 2016). The middle cerebellar peduncles are the main afferent pathway to the cerebellum and are composed of white matter fibers originating from the contralateral pontine nuclei. The pontine nuclei are intermediate gray matter scattered in the basis pons and part of the corticopontocerebellar pathway that controls not only the action of motor tasks but also the planning and initiation of movements. Thus, difficulty walking (cerebellar ataxia), difficulty speaking (scanning speech), and in some cases vertigo and facial weakness are common clinical manifestations of middle cerebellar peduncles lesion (Morales and Tomsick, 2015).
Compared to HC, patients with WMHL has been associated with an increased risk of cognitive impairment and a significantly greater burden (Hu et al., 2021; Dadar et al., 2022). Our study found that hypertension scores and MoCA scores show a significant correlation with decreased connectivity in corpus callosum and ILF. Previous studies have reported that disruption of the corpus callosum microstructure especially the WMHL penumbra of the corpus callosum body may contribute to cognitive deficits associated with subcortical ischemic vascular disease (Qiu et al., 2021). Microstructural disruption of the right IFOF and ILF contributes to WMHL-related cognitive impairment (Chen et al., 2020) which is basically consistent with our research results, the effect of WMHL on the microstructural integrity of WM tracts may propagate along tracts to distal regions beyond the penumbra (Liu et al., 2021), In addition, in our study, decreased connectivity in left thalamoparietal pathways and left cerebellar were negatively correlated with MoCA scores. The thalamus is a crucial node in networks supporting cognitive functions known to deteriorate with normal aging, including component processes of memory and executive functions of attention and information processing (Fama and Sullivan, 2015). The cerebellum has both sensorimotor and cognitive effects. The lesions of the anterior lobe lead to dyskinesia, and the lesions of the posterior lobe result in cerebellar cognitive affective syndrome, which may explain WMHL susceptibility to motor and emotional disorders or other symptoms (Schmahmann, 2019).
In our study, patients with WMHL had higher BMI. Interestingly, previous studies have reported that higher BMI contributed to an increased deep-periventricular WMHL ratio (Lampe et al., 2019), which may be due to obesity leading to higher white matter burden through inflammatory processes, as indexed by elevated IL-6. Negative BMI associations are widely distributed across white matter pathways in a largely bilateral pattern primarily in corpus callosum, which is consistent with our findings (Karlsson et al., 2013; Verstynen et al., 2013). In particular, we found that BMI was negatively correlated with cingulum fasciculus, fornix/fimbria, which are the most prominent tracts within the limbic system. The fornix is a C-shaped fiber bundle that provides strong connections from the hippocampus to other brain regions. The cingulum is another C-shaped structure of WM fiber wrapped around the frontal and temporal lobe above the corpus callosum. The anterior part is important for emotion processing, while the posterior region is involved in cognition. This result may show BMI’s heterogeneous association with white matter pathways across the brain (Verstynen et al., 2013).
Our research still has several limitations. First, the age range of patients and sample sizes were small, a larger sample should be used in future studies. Secondly, the lack of longitudinal traces cannot provide evidence for progressive changes between white matter hyperintensities and the main clinical features, in furture, research based on longitudinal data may make the correlations we observe more specific. In addition to the clinical features discussed in this paper, other factors such as smoking, alcohol consumption, diabetes, and hyperlipemia are risk factors for WMHL. Further research is needed to include hypertensive patients with different accompanying symptoms (eg. cerebrovascular disease, cardiovascular disease, kidney disease, etc.) in the future. Especially longitudinal studies may better describe this characteristic in patients with WMHL.
In conclusion, this study employed a novel approach to diffusion connectometry to identify hypertension related clinical features and corresponding white matter structures. This preliminary study results support hypertension degree and higher BMI are associated with white matter local disconnection in patients with WMHL, and may contribute to understanding the cognitive impairments observed in patients with WMHL.
Data availability statement
The raw data supporting the conclusions of this article will be made available by the authors, without undue reservation.
Ethics statement
The studies involving human participants were reviewed and approved by Medical Research Ethics Committee of the First Affiliated Hospital of Nanchang University. The patients/participants provided their written informed consent to participate in this study.
Author contributions
FZ, QK, MH, YL, and LW: conceptualization. YL, QK, and MH: data collection. FZ, MH, QK, and LW: data analysis. FZ: funding analysis. MH, QK, FZ, LW, JD, and YL: investigation, writing—original draft, and writing—review and editing. FZ, LW, and YL: supervision. All authors contributed to the article and approved the submitted version.
Funding
This study was supported by the National Science Foundation of China (Grant no 82160331).
Acknowledgments
The authors thank their patients and volunteers for participating in this study. The funders had no role in the study design, data collection and analysis, decision to publish, or preparation of the manuscript.
Conflict of interest
The authors declare that the research was conducted in the absence of any commercial or financial relationships that could be construed as a potential conflict of interest.
Publisher’s note
All claims expressed in this article are solely those of the authors and do not necessarily represent those of their affiliated organizations, or those of the publisher, the editors and the reviewers. Any product that may be evaluated in this article, or claim that may be made by its manufacturer, is not guaranteed or endorsed by the publisher.
Footnotes
References
Alber, J., Alladi, S., Bae, H. J., Barton, D. A., Beckett, L. A., Bell, J. M., et al. (2019). White matter hyperintensities in vascular contributions to cognitive impairment and dementia (VCID): knowledge gaps and opportunities. Alzheimers Dement (N Y) 5, 107–117. doi: 10.1016/j.trci.2019.02.001
Andica, C., Kamagata, K., Uchida, W., Takabayashi, K., Shimoji, K., Kaga, H., et al. (2022). White matter fiber-specific degeneration in older adults with metabolic syndrome. Mol Metab 62:101527. doi: 10.1016/j.molmet.2022.101527
Artero, S., Tiemeier, H., Prins, N. D., Sabatier, R., Breteler, M. M., and Ritchie, K. (2004). Neuroanatomical localisation and clinical correlates of white matter lesions in the elderly. J. Neurol. Neurosurg. Psychiatry 75, 1304–1308. doi: 10.1136/jnnp.2003.023713
Blaauw, J., and Meiners, L. C. (2020). The splenium of the corpus callosum: embryology, anatomy, function and imaging with pathophysiological hypothesis. Neuroradiology 62, 563–585. doi: 10.1007/s00234-019-02357-z
Chen, H. F., Huang, L. L., Li, H. Y., Qian, Y., Yang, D., Qing, Z., et al. (2020). Microstructural disruption of the right inferior fronto-occipital and inferior longitudinal fasciculus contributes to WMHL-related cognitive impairment. CNS Neurosci. Ther. 26, 576–588. doi: 10.1111/cns.13283
Dadar, M., Mahmoud, S., Zhernovaia, M., Camicioli, R., Maranzano, J., and Duchesne, S. (2022). White matter hyperintensity distribution differences in aging and neurodegenerative disease cohorts. Neuroimage Clin. 36:103204. doi: 10.1016/j.nicl.2022.103204
Debette, S., and Markus, H. S. (2010). The clinical importance of white matter hyperintensities on brain magnetic resonance imaging: systematic review and meta-analysis. BMJ 341:c3666. doi: 10.1136/bmj.c3666
Fama, R., and Sullivan, E. V. (2015). Thalamic structures and associated cognitive functions: relations with age and aging. Neurosci. Biobehav. Rev. 54, 29–37. doi: 10.1016/j.neubiorev.2015.03.008
Frantellizzi, V., Pani, A., Ricci, M., Locuratolo, N., Fattapposta, F., and De Vincentis, G. (2020). Neuroimaging in vascular cognitive impairment and dementia: a systematic review. J. Alzheimers Dis. 73, 1279–1294. doi: 10.3233/jad-191046
Frey, B. M., Petersen, M., Mayer, C., Schulz, M., Cheng, B., and Thomalla, G. (2019). Characterization of white matter Hyperintensities in large-scale MRI-studies. Front. Neurol. 10:238. doi: 10.3389/fneur.2019.00238
Gebeily, S., Fares, Y., Kordahi, M., Khodeir, P., Labaki, G., and Fazekas, F. (2014). Cerebral white matter hyperintensities (WMHL): an analysis of cerebrovascular risk factors in Lebanon. Int. J. Neurosci. 124, 799–805. doi: 10.3109/00207454.2014.884087
Gons, R. A., van Oudheusden, L. J., de Laat, K. F., van Norden, A. G., van Uden, I. W., Norris, D. G., et al. (2012). Hypertension is related to the microstructure of the corpus callosum: the RUN DMC study. J. Alzheimers Dis. 32, 623–631. doi: 10.3233/jad-2012-121006
Herbet, G., Zemmoura, I., and Duffau, H. (2018). Functional anatomy of the inferior longitudinal fasciculus: from historical reports to current hypotheses. Front. Neuroanat. 12:77. doi: 10.3389/fnana.2018.00077
Hu, H. Y., Ou, Y. N., Shen, X. N., Qu, Y., Ma, Y. H., Wang, Z. T., et al. (2021). White matter hyperintensities and risks of cognitive impairment and dementia: a systematic review and meta-analysis of 36 prospective studies. Neurosci. Biobehav. Rev. 120, 16–27. doi: 10.1016/j.neubiorev.2020.11.007
Karlsson, H. K., Tuulari, J. J., Hirvonen, J., Lepomäki, V., Parkkola, R., Hiltunen, J., et al. (2013). Obesity is associated with white matter atrophy: a combined diffusion tensor imaging and voxel-based morphometric study. Obesity (Silver Spring) 21, 2530–2537. doi: 10.1002/oby.20386
Lampe, L., Zhang, R., Beyer, F., Huhn, S., Kharabian Masouleh, S., Preusser, S., et al. (2019). Visceral obesity relates to deep white matter hyperintensities via inflammation. Ann. Neurol. 85, 194–203. doi: 10.1002/ana.25396
Li, X., Liang, Y., Chen, Y., Zhang, J., Wei, D., Chen, K., et al. (2015). Disrupted Frontoparietal network mediates white matter structure dysfunction associated with cognitive decline in hypertension patients. J. Neurosci. 35, 10015–10024. doi: 10.1523/jneurosci.5113-14.2015
Liao, D., Cooper, L., Cai, J., Toole, J. F., Bryan, N. R., Hutchinson, R. G., et al. (1996). Presence and severity of cerebral white matter lesions and hypertension, its treatment, and its control. The ARIC study. Atherosclerosis risk in communities study. Stroke 27, 2262–2270. doi: 10.1161/01.str.27.12.2262
Liu, Y., Xia, Y., Wang, X., Wang, Y., Zhang, D., Nguchu, B. A., et al. (2021). White matter hyperintensities induce distal deficits in the connected fibers. Hum. Brain Mapp. 42, 1910–1919. doi: 10.1002/hbm.25338
Lu, T., Wang, Z., Cui, Y., Zhou, J., Wang, Y., and Ju, S. (2021). Disrupted structural brain connectome is related to cognitive impairment in patients with ischemic Leukoaraiosis. Front. Hum. Neurosci. 15:654750. doi: 10.3389/fnhum.2021.654750
Maillard, P., Fletcher, E., Harvey, D., Carmichael, O., Reed, B., Mungas, D., et al. (2011). White matter hyperintensity penumbra. Stroke 42, 1917–1922. doi: 10.1161/strokeaha.110.609768
Meng, F., Yang, Y., and Jin, G. (2022). Research Progress on MRI for white matter Hyperintensity of presumed vascular origin and cognitive impairment. Front. Neurol. 13:865920. doi: 10.3389/fneur.2022.865920
Min, Z. G., Shan, H. R., Xu, L., Yuan, D. H., Sheng, X. X., Xie, W. C., et al. (2021). Diffusion tensor imaging revealed different pathological processes of white matter hyperintensities. BMC Neurol. 21:128. doi: 10.1186/s12883-021-02140-9
Morales, H., and Tomsick, T. (2015). Middle cerebellar peduncles: magnetic resonance imaging and pathophysiologic correlate. World J. Radiol. 7, 438–447. doi: 10.4329/wjr.v7.i12.438
Pelletier, D., Garrison, K., and Henry, R. (2004). Measurement of whole-brain atrophy in multiple sclerosis. J. Neuroimaging 14, 11S–19S. doi: 10.1111/j.1552-6569.2004.tb00274.x
Qiu, Y., Yu, L., Ge, X., Sun, Y., Wang, Y., Wu, X., et al. (2021). Loss of integrity of Corpus callosum white matter Hyperintensity penumbra predicts cognitive decline in patients with subcortical vascular mild cognitive impairment. Front. Aging Neurosci. 13:605900. doi: 10.3389/fnagi.2021.605900
Rosario, B. L., Rosso, A. L., Aizenstein, H. J., Harris, T., Newman, A. B., Satterfield, S., et al. (2016). Cerebral white matter and slow gait: contribution of Hyperintensities and Normal-appearing parenchyma. J. Gerontol. A Biol. Sci. Med. Sci. 71, 968–973. doi: 10.1093/gerona/glv224
Sargurupremraj, M., Suzuki, H., Jian, X., Sarnowski, C., Evans, T. E., Bis, J. C., et al. (2020). Cerebral small vessel disease genomics and its implications across the lifespan. Nat. Commun. 11:6285. doi: 10.1038/s41467-020-19111-2
Schmahmann, J. D. (2019). The cerebellum and cognition. Neurosci. Lett. 688, 62–75. doi: 10.1016/j.neulet.2018.07.005
Shen, X., Raghavan, S., Przybelski, S. A., Lesnick, T. G., Ma, S., Reid, R. I., et al. (2022). Causal structure discovery identifies risk factors and early brain markers related to evolution of white matter hyperintensities. Neuroimage Clin. 35:103077. doi: 10.1016/j.nicl.2022.103077
van Rooden, S., van den Berg-Huysmans, A. A., Croll, P. H., Labadie, G., Hayes, J. M., Viviano, R., et al. (2018). Subjective cognitive decline is associated with greater white matter Hyperintensity volume. J. Alzheimers Dis. 66, 1283–1294. doi: 10.3233/jad-180285
Verhaaren, B. F., Vernooij, M. W., de Boer, R., Hofman, A., Niessen, W. J., van der Lugt, A., et al. (2013). High blood pressure and cerebral white matter lesion progression in the general population. Hypertension 61, 1354–1359. doi: 10.1161/hypertensionaha.111.00430
Verstynen, T. D., Weinstein, A., Erickson, K. I., Sheu, L. K., Marsland, A. L., and Gianaros, P. J. (2013). Competing physiological pathways link individual differences in weight and abdominal adiposity to white matter microstructure. NeuroImage 79, 129–137. doi: 10.1016/j.neuroimage.2013.04.075
Wardlaw, J. M., Smith, E. E., Biessels, G. J., Cordonnier, C., Fazekas, F., Frayne, R., et al. (2013). Neuroimaging standards for research into small vessel disease and its contribution to ageing and neurodegeneration. Lancet Neurol. 12, 822–838. doi: 10.1016/s1474-4422(13)70124-8
Williams, B., Mancia, G., Spiering, W., Agabiti Rosei, E., Azizi, M., Burnier, M., et al. (2018). 2018 ESC/ESH guidelines for the management of arterial hypertension: the task force for the management of arterial hypertension of the European Society of Cardiology and the European Society of Hypertension: the task force for the management of arterial hypertension of the European Society of Cardiology and the European Society of Hypertension. J. Hypertens. 36, 1953–2041. doi: 10.1097/hjh.0000000000001940
Wong, N. M. L., Ma, E. P., and Lee, T. M. C. (2017). The integrity of the Corpus callosum mitigates the impact of blood pressure on the ventral attention network and information processing speed in healthy adults. Front. Aging Neurosci. 9:108. doi: 10.3389/fnagi.2017.00108
Wu, X., Ge, X., Du, J., Wang, Y., Sun, Y., Han, X., et al. (2019). Characterizing the penumbras of white matter Hyperintensities and their associations with cognitive function in patients with subcortical vascular mild cognitive impairment. Front. Neurol. 10:348. doi: 10.3389/fneur.2019.00348
Yeh, F. C., Badre, D., and Verstynen, T. (2016). Connectometry: a statistical approach harnessing the analytical potential of the local connectome. NeuroImage 125, 162–171. doi: 10.1016/j.neuroimage.2015.10.053
Yeh, F. C., and Tseng, W. Y. (2011). NTU-90: a high angular resolution brain atlas constructed by q-space diffeomorphic reconstruction. NeuroImage 58, 91–99. doi: 10.1016/j.neuroimage.2011.06.021
Yeh, F. C., Wedeen, V. J., and Tseng, W. Y. (2010). Generalized q-sampling imaging. IEEE Trans. Med. Imaging 29, 1626–1635. doi: 10.1109/tmi.2010.2045126
Yuan, J. L., Wang, S. K., Guo, X. J., Teng, L. L., Jiang, H., Gu, H., et al. (2017). Disconnections of Cortico-subcortical pathways related to cognitive impairment in patients with Leukoaraiosis: a preliminary diffusion tensor imaging study. Eur. Neurol. 78, 41–47. doi: 10.1159/000477899
Keywords: white matter hyperintensity, diffusion magnetic resonance imaging, hypertension, cognition, body mass index, tractography
Citation: Kuang Q, Huang M, Lei Y, Wu L, Jin C, Dai J and Zhou F (2023) Clinical and cognitive correlates tractography analysis in patients with white matter hyperintensity of vascular origin. Front. Neurosci. 17:1187979. doi: 10.3389/fnins.2023.1187979
Edited by:
Eduard Rodriguez-Farre, Spanish National Research Council (CSIC), SpainReviewed by:
Diana L. Giraldo, University of Antwerp, BelgiumMaryam Haghshomar, Northwestern Medicine, United States
Copyright © 2023 Kuang, Huang, Lei, Wu, Jin, Dai and Zhou. This is an open-access article distributed under the terms of the Creative Commons Attribution License (CC BY). The use, distribution or reproduction in other forums is permitted, provided the original author(s) and the copyright owner(s) are credited and that the original publication in this journal is cited, in accordance with accepted academic practice. No use, distribution or reproduction is permitted which does not comply with these terms.
*Correspondence: Fuqing Zhou, bmR5ZnkwMjMwMUBuY3UuZWR1LmNu
†These authors have contributed equally to this work