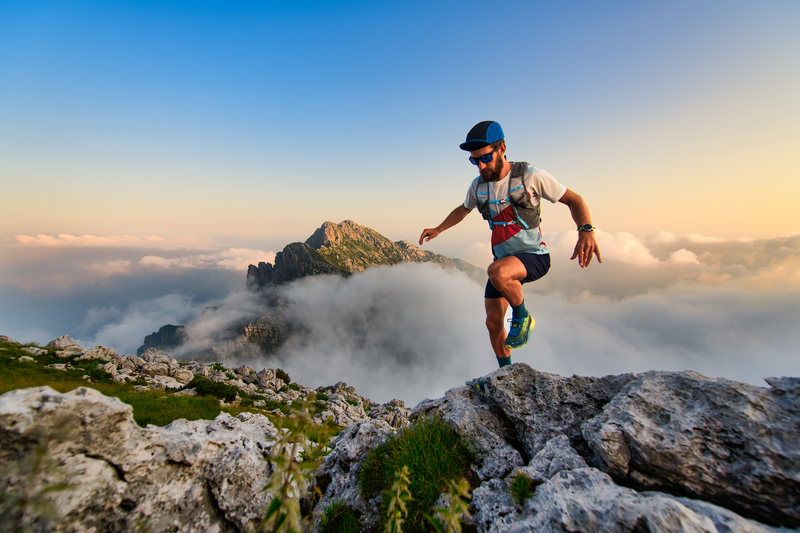
95% of researchers rate our articles as excellent or good
Learn more about the work of our research integrity team to safeguard the quality of each article we publish.
Find out more
ORIGINAL RESEARCH article
Front. Neurosci. , 18 May 2023
Sec. Sleep and Circadian Rhythms
Volume 17 - 2023 | https://doi.org/10.3389/fnins.2023.1170889
Introduction: Obstructive sleep apnea (OSA) has been associated with psychiatric disorders, especially depression and posttraumatic stress disorder (PTSD). FKBP5 genetic variants have been previously reported to confer the risk of depression and PTSD. This study aimed to investigate the association of single nucleotide polymorphisms (SNPs) in the FKBP5 gene with OSA and OSA-related quantitative traits.
Methods: Four SNPs within the FKBP5 gene (rs1360780, rs3800373, rs9296158, rs9470080) were genotyped in 5773 participants with anthropometric and polysomnography data. Linear regression and logistic regression analyses were performed to evaluate the relationship between FKBP5 SNPs and OSA-related traits. Binary logistic regression was used to assess the effect of SNPs on OSA susceptibility. Interacting genes of SNPs were assessed based on the 3DSNP database, and expression quantitative trait loci (eQTL) analysis for SNPs was adopted to examine the correlation of SNPs with gene expression. Gene expression analyses in human brains were performed with the aid of Brain Atlas.
Results: In moderate-to-severe OSA patients, all four SNPs were positively associated with AHIREM, and rs9296158 showed the strongest association (ß = 1.724, p = 0.001). Further stratified analyses showed that in men with moderate OSA, rs1360780, rs3800373 and rs9470080 were positively associated with wake time (p = 0.0267, p = 0.0254 and p = 0.0043, respectively). Rs1360780 and rs3800373 were 28 and 29.4%more likely to rate a higher ordered MAI category (OR (95% CI) = 1.280 (1.042 – 1.575), p = 0.019; OR (95% CI) = 1.294 (1.052 – 1.592), p = 0.015, respectively). Rs9296158 and rs9470080 increased the risk of low sleep efficiency by 25.7 and 28.1% (OR (95% CI) = 1.257 (1.003 – 1.575), p = 0.047; OR (95% CI) = 1.281 (1.026–1.6), p = 0.029, respectively). Integrated analysis of eQTL and gene expression patterns revealed that four SNPs may exert their effects by regulating FKBP5, TULP1, and ARMC12.
Conclusion: Single nucleotide polymorphisms in the FKBP5 gene were associated with sleep respiratory events in moderate-to-severe OSA patients during REM sleep and associated with sleep architecture variables in men with moderate OSA. FKBP5 variants may be a potential predisposing factor for sleep disorders, especially in REM sleep.
Obstructive sleep apnea (OSA) is one of the most prevalent sleep disorders around the globe, affecting 936 million adults worldwide aged 30–69 years and with a higher incidence in men and older people. China has the largest number of OSA individuals, with approximately 176 million estimated to have an apnea-hypopnea index (AHI) ≥ 5 per hour of sleep (Benjafield et al., 2019). OSA is characterized by recurrent episodes of obstruction in the upper airway during sleep, causing airflow reduction or cessation and resulting in intermittent hypoxia and fragmented sleep, and is usually accompanied by decreased sleep quality, daytime sleepiness and snoring symptoms (Lévy et al., 2015).
Obstructive sleep apnea is often associated with psychiatric disorders, a common comorbidity in patients with mental illness, especially depression and posttraumatic stress disorder (PTSD; Gupta and Simpson, 2015; Krakow et al., 2015; Stubbs et al., 2016; Zhang et al., 2017), both of which are stress-related psychiatric disorders. Depression exhibits negative alterations in mood, sleep, appetite, psychomotor activity and cognition (Dwyer et al., 2020). PTSD is characterized by intrusion, avoidance, cognitive and mood changes, and alterations in arousal after trauma events (Jaoude et al., 2015). Several studies have claimed a bidirectional and complicated connection linking OSA and psychiatric disorders (Gupta and Simpson, 2015; Jaoude et al., 2015). It has been reported that OSA prevalence is increased in individuals suffering from depression or PTSD (Hattori et al., 2009), and OSA patients are at higher risk of psychiatric comorbid conditions, including depression, PTSD, anxiety disorder or psychosis (Sharafkhaneh et al., 2005). Both OSA and depression/PTSD are reported to share overlapping symptoms, including sleep disturbances, negative changes in cognitions and mood, and a lower quality of life. Psychiatric symptoms such as nightmare, anxiety and depressive state in PTSD or depression patients were also significantly relieved after CPAP treatment for comorbid OSA, suggesting that a potential complex interplay may exist between the two disorders (Habukawa et al., 2010; Tamanna et al., 2014). Researchers have suggested that multiple factors may play a role in the association of depression and PTSD with OSA because they have similarities in disrupted biological pathways, including neurotransmitter imbalances, hypothalamus-pituitary–adrenal (HPA) axis disturbances, dysregulated inflammatory pathways, and increased oxidative stress (Gozal et al., 2008; Lopresti and Drummond, 2013; Tauman et al., 2014). However, potential clues regarding genetic links remain unclear, although genetic factors contribute not only to psychiatric disorder development but also to OSA etiology and phenotype (Colvonen et al., 2018; Xu et al., 2022). Potential genetic background overlap between stress-related psychiatric disorders and OSA has yet to be explored.
Glucocorticoids (GCs) help control the circadian sleep–wake rhythm and maintain sleep homeostasis, and prolonged exposure to GCs can lead to sleep alterations (Nicolaides et al., 2000). FKBP5 is a glucocorticoid-responsive gene and is also essential for the negative feedback of the HPA axis (Hähle et al., 2019), indicating that FKBP5 may also exert an effect on sleep modulation. The FKBP5 gene has emerged as one of the most promising and comprehensively studied candidate genes for PTSD and depression thus far (Smoller, 2016). A large body of research has found that single nucleotide polymorphisms (SNPs) in FKBP5 are associated with a number of psychiatric disorders, including mood and anxiety disorders, PTSD, psychosis, and substance abuse disorders (Binder et al., 2008; Xie et al., 2010; Zimmermann et al., 2011; Klengel et al., 2013). Those findings mainly focused on depression and PTSD, reporting that FKBP5 variations are also associated with psychiatric-related phenotypes, including post-traumatic pain severity (Bortsov et al., 2013), and with recurrence of depressive episodes and response to antidepressant treatment (Binder et al., 2004), which has been widely observed and validated in patients across different races (Binder, 2009; Zannas and Binder, 2014; Matosin et al., 2018). In individuals with higher induction alleles, impaired negative feedback of the HPA axis altered by FKBP5 variants results in a prolongation of stress hormone system activation under stress exposure. Among these genotypes, the most widely studied four SNPs, namely, rs1360780, rs3800373, rs9296158, and rs9470080, contribute to the risk and disease severity of depression and PTSD (Binder et al., 2004, 2008; Xie et al., 2010; Zimmermann et al., 2011), so we chose the four variants as our research object.
Concerning the high susceptibility to OSA in mental disorders and the role of FKBP5 in glucocorticoid action, it is plausible to hypothesize that genetic variants of FKBP5, which is a PTSD and depression candidate gene, may have an impact on OSA. To date, no study has investigated the association between OSA and PTSD/depression at the genetic level. Given the lack of data implicating the influence of FKBP5 variants on sleep regulation, we conducted a large cross-sectional study to evaluate the association of FKBP5 polymorphisms with OSA and OSA-related quantitative clinical traits in a Chinese Han OSA population.
Subjects suspected of having OSA were enrolled from the Shanghai Sleep Health Study (SSHS) cohort, in which people were hospitalized and observed in the sleep center of Shanghai Sixth People’s Hospital Affiliated to Shanghai Jiao Tong University School of Medicine. In addition to the genomic study, demographic information and clinical measurement data were also collected for each individual, from whom written informed consent was obtained (Xu et al., 2022). Next, subjects from SSHS were screened according to the following exclusion criteria. (i) age less than 18 years old; (ii) history of continuous positive airway pressure (CPAP) treatment, oral appliance therapy or upper airway surgery; (iii) history of other sleep disorders (i.e., narcolepsy, restless leg syndrome, or upper airway resistance syndrome); (iv) systemic disease (i.e., chronic liver disease, chronic kidney disease), cancer, hyperparathyroidism, or hypoparathyroidism; (v) cardiopulmonary diseases. (vi) unavailable standard polysomnography data. A total of 5,773 participants were ultimately analyzed in this study. All cases and controls were of Han Chinese descent and mainly from eastern China.
Anthropometric parameters, including height (m) and weight (kg), were measured in barefoot subjects wearing lightweight clothing. Waist circumference (WC, cm) was measured in the middle of the lowest rib margin and iliac crest during gentle exhalation. Hip circumference (HC, cm) was defined as the maximum circumference over the buttocks at the baseline visit. The body mass index (BMI, kg/m2) was calculated as weight divided by height squared, and waist–hip ratio (WHR) was calculated as WC divided by HC.
The effect of sleep apnea on excessive daytime sleepiness was assessed using the verified Chinese version of the Epworth Sleepiness Scale (ESS; Johns, 1991). All participants were asked to complete a self-administered questionnaire evaluating their subjective daytime sleepiness level by eight items, with overall scores ranging from 0 to 24 and higher scores indicating more daytime sleepiness (Johns, 1991).
All participants underwent nocturnal polysomnography (PSG) monitoring (Alice 4 or 5; Respironics, Pittsburgh, PA, United States) in the sleep center. Data containing respiratory events, oxygen traits and objective sleep-related variables that were recorded by polysomnographic device were then manually scored and checked by experienced sleep technicians according to the 2012 criteria of the American Academy of Sleep Medicine (AASM; Berry et al., 2012).
Apnea was defined as a reduction in oronasal airflow from baseline by ≥ 90% for at least 10 s. Hypopnea was defined as a drop in signal excursions from baseline by ≥ 30% for ≥ 10 s, accompanied by a decrease in oxygen desaturation of ≥ 3% or an associated arousal from sleep. The apnea–hypopnea index (AHI) was calculated based on the mean number of apnea and hypopnea events per hour of sleep. OSA severity was quantified by AHI, and non-OSA, mild, moderate, and severe OSA were defined as AHI < 5.0, 5.0–14.9, 15.0–29.9 and ≥ 30.0, respectively. AHIREM and AHINREM were calculated as the numbers of AHI per hour of REM and non-REM (NREM) sleep.
The oxygen desaturation index (ODI) was defined as the total number of episodes of ≥ 3% arterial oxygen desaturation per hour of sleep. LSpO2 referred to the lowest oxyhemoglobin saturation.
Sleep period time (SPT) was defined as the time from the first epoch of sleep to final awakening, and total sleep time (TST) was defined as the total time in any stage of sleep. Sleep efficiency (SE) was calculated as TST/TIB (time in bed). Wake time (WK) during sleep was defined as the total time awake during SPT in our study when electroencephalogram (EEG) showed predominantly α rhythm activity on eye closure during quiet alertness, or eye blinking and eye movement with normal or high chin muscle tone detected by electrooculogram and electromyogram. Sleep time comprised non-rapid eye movement (NREM) sleep and rapid eye movement (REM) sleep, with the former categorized into stage N1, N2 and N3. Stage N1 represents sleep onset, in which α waves are attenuated and replaced by mostly θ waves. Stage N2 was characterized by “K-complexes” and “sleep spindles,” and stage N3 reflected slow wave sleep. Stage REM or R was defined when rapid eye movements, low-voltage mixed-frequency brain wave activity and low chin muscle tone were present. The ratios were also calculated as follows: Wake time during SPT (WK/SPT%); REM during SPT (REM/SPT%); REM during TST (REM/TST%); N1, N2, and N3 sleep times during SPT (N1, N2, and N3/SPT%); and N1, N2, and N3 sleep times during TST (N1, N2, and N3/TST%). Arousal was defined as an abrupt shift in the EEG frequency, including α, θ and/or other frequencies ≥16 Hz (but not spindles), lasting for ≥ 3 s. The microarousal index (MAI) referred to the mean number of arousals per hour of sleep.
Four SNPs in the FKBP5 gene that have previously been reported to be associated with both PTSD and depression (rs1360780, rs3800373, rs9296158, and rs9470080) were enrolled in this study (Binder et al., 2004, 2008; Xie et al., 2010; Zimmermann et al., 2011). Genomic DNA was extracted from blood samples of each participant by a DNA isolation kit (Qiagen). Affymetrix Axiom Genome-Wide CHB Array (CHB) was used to detect the specific base sequence, and information about these 4 SNPs for every participant was obtained from this genomic database. The call rates of rs1360780, rs3800373, rs9296158, and rs9470080 were 100, 97.7, 99.1 and 100%, respectively. All selected FKBP5 SNPs met Hardy–Weinberg equilibrium in cases and controls (p > 0.05), with minor allele frequencies >0.01.
Statistical analyses were performed using SPSS 26.0 software (IBM Corp., Armonk, NY, United States). The normally distributed data are presented as the means and standard deviation; skewed data are presented as the median (IQR), and categorical data are presented as the number (percentage). Differences in the baseline characteristics among the four groups were examined using one-way analysis of variance (ANOVA), non-parametric Kruskal–Wallis H test, or χ2 tests according to the type of data distribution. The Hardy–Weinberg equilibrium test was assessed using PLINK (v1.90) before association analysis (Purcell et al., 2007). Linkage disequilibrium analysis was performed by Haploview (version 4.2) by calculating |D′| and r2, and the haplotype block structure was estimated by the confidence interval (CI) algorithm (Gabriel et al., 2002; Barrett et al., 2005). OSA-related quantitative phenotypic variables were analyzed under the additive genetic model by linear regression. Logistic regression analyses were performed to evaluate the relationship between polymorphisms and categorical variables. For AHIREM or microarousal index analysis, all subjects were grouped into 4 ordered categories by AHIREM or MAI quartiles (Qs). Odds ratios (ORs) and 95% confidence intervals (CIs) were estimated by multinomial or ordinal logistic regression, using patients in the first quartile (Q1) of AHIREM or MAI as the reference category. The ordinal logistic model assumed that these odds ratios were equal between the adjacent ordered categorical dependent variables and was adopted under the proportional odds model, which was verified by parallel line testing (p > 0.05; Guan et al., 2016). If the proportional odds assumption was not met by the test of parallel lines (p < 0.05), we performed multinomial logistic regression with unordered categorical variables instead. Logistic regression analyses were also used to assess the effect of SNPs on the risk of OSA. A two-tailed p value < 0.05 was considered statistically significant.
Expression quantitative trait loci (eQTL) analysis of SNPs was obtained from Ensembl data.1 Data from the 3DSNP database were used to investigate the link between SNPs and their three-dimensional interacting genes through 3D chromatin loops.2 Human brain normalized microarray expression data were downloaded from the Allen Human Brain Atlas,3 and then a heatmap was drawn displaying relevant gene expression levels in different parts of the human brain by GraphPad Prism software (GraphPad Prism v9.0.0).
In the current study, we enrolled a total of 5,773 individuals of Han Chinese ancestry, comprising 5,029 OSA patients (314 mild OSA, 1199 moderate OSA, 3516 severe OSA) and 744 non-OSA controls. The baseline characteristics of the participants in the four groups are shown in Table 1. Age, the proportion of male patients, BMI, AHIREM and AHINREM, microarousal index, percentages of N1 time/TST, ODI and daytime sleepiness were positively correlated with increasing OSA severity. Meanwhile, the percentages of REM/TST, N3/TST and lowest oxyhemoglobin saturation decreased with increasing OSA severity.
Table 1. Basic characteristics of the overall population classified by the severity of obstructive sleep apnea (OSA).
The basic characteristics of SNPs within the FKBP5 gene are listed in Table 2. The genotype frequencies of all variants were in Hardy–Weinberg equilibrium (Supplementary Figure S1). The minor allele frequencies of rs3800373 and rs1360780 between the non-OSA and OSA groups were significantly different (p = 0.009 and p = 0.016, respectively).
We investigated the association between the four psychiatric disorder-associated SNPs in the FKBP5 gene and OSA-related quantitative sleep parameters, including respiratory events, oxygen traits and objective sleep-related variables. We observed no significant association between the SNPs and quantitative traits across all the participants and the non-OSA group, which are listed in the Supplementary Tables. However, in moderate-to-severe OSA patients (AHI ≥ 15, n = 4,715), linear regression analysis revealed that all SNPs were positively associated with AHIREM, and rs9296158 showed the strongest association with AHIREM (ß = 1.724, p = 0.001), even after adjusting for age, sex and BMI (Table 3). We then stratified AHIREM into quartiles corresponding to an AHIREM of Q1 (AHIREM < 20.3), Q2 (20.3 ≤ AHIREM < 44.9), Q3 (44.9 ≤ AHIREM < 60.9), and Q4 (AHIREM ≥ 60.9). Multinomial logistic regression analyses were employed to evaluate the impacts of SNPs on each quartile of AHIREM using Q1 as the reference category (Figure 1), which showed that all four SNPs increased the likelihood of belonging to higher quartiles of AHIREM. Rs9296158 or rs947000 carrying the psychiatric disorder-related risk A or T allele was also related to a graded increase in the risk of having the highest quartile (Q4) of AHIREM compared to the reference category (Q1) in the moderate-to-severe OSA population (OR (95% CI) = 1.26 (1.086–1.463), p = 0.002; OR (95% CI) = 1.261 (1.088–1.461), p = 0.002, respectively). There were no associations of SNPs with oxygen traits.
Table 3. Association of SNPs with clinical features related to respiratory events in moderate-to-severe OSA patients (n = 4,715).
Figure 1. Effects of single nucleotide polymorphisms (SNPs) and quartiles on each quartile of AHIREM in moderate to severe obstructive sleep apnea (OSA) patients (reference = Quartile1). OR (odds ratio) and 95% CI were obtained by multinomial logistic regression using the first quartile of AHIREM as the reference category, and regression models were adjusted for age, sex and BMI. Diamonds indicate ORs, and horizontal dark lines indicate 95% CIs.
Further stratified analyses showed that in male moderate OSA subjects, FKBP5 SNPs rs1360780, rs3800373 and rs9470080 were positively associated with WK during SPT (p = 0.0267, p = 0.0254 and p = 0.0043, respectively; Figure 2). Rs1360780, rs3800373 and rs9470080 showed significant positive associations with percentage of wake time during sleep period time (WK/SPT%) after adjusting for age and BMI (all p < 0.05; Table 4). For microarousal, all subjects were grouped into 4 ordered categories by MAI quartile, and we adopted an ordinal logistic regression analysis (proportional odds assumption verified by parallel line test) to estimate the effects of SNPs on MAI (Table 5). The results showed that both rs1360780 and rs3800373 were 28 and 29.4%more likely to rate a higher ordered MAI category, which means that these two SNPs were positively associated with the severity of sleep fragmentation after adjustment for age and BMI (OR (95% CI) = 1.280 (1.042–1.575), p = 0.019; OR (95% CI) = 1.294 (1.052–1.592), p = 0.015, respectively). Moreover, rs9296158 and rs9470080 increased the risk of low sleep efficiency (TST/TIB ≤ 80%) by 25.7 and 28.1% (OR (95% CI) = 1.257(1.003–1.575), p = 0.047; OR (95% CI) = 1.281(1.026–1.6), p = 0.029, respectively; Table 6). No association was detected between FKBP5 genetic variants and the risk of moderate OSA (p > 0.05; Supplementary Table S4).
Figure 2. Association of SNPs with wake time during sleep period time in men with moderate OSA (n = 950). The bar charts showed the associations of rs1360780 (A), rs3800373 (B), rs9296158 (C) and rs9470080 (D) with wake time. Data are shown as the means ± SEMs. The p value was calculated using ANOVA.
We assessed the relationship between the four SNPs and their interacting genes. Based on data from the 3DSNP database, rs1360780 interacted with six protein-coding genes (ARMC12, CLPS, CLPSL1, CLPSL2, FKBP5, TULP1) in various tissues, and the other 3 SNPs were linked to five genes via chromatin loops (Supplementary Table S5). Among the SNP-interacting genes, TULP1 is located ~ 60 kb downstream from the FKBP5 gene, while ARMC12 is located ~ 3 kb upstream from the FKBP5 gene (Supplementary Figure S2). We examined the expression quantitative trait loci (eQTL) information from the Ensembl database to investigate whether these SNPs were correlated with the expression of nearby genes, by which these variants might exert their functions in different human tissues. These data suggested that the four SNPs were also all associated with several genes, including TULP1, ARMC12, ETV7, SRPK1, PXT1, BRPF3, RPL10A, STK38, and FKBP5, in multiple tissues. Among them, all four SNPs were correlated with the expression of TULP1 in multiple tissues, showing the strongest correlation, and rs1360780 was associated with a marked decrease in TULP1 expression in the heart atrial appendage (p = 8.93 × 10−13). Rs3800373 was shown to be associated with increased expression of ARMC12 in the muscular layer of the esophagus (p = 6.95 × 10−5), with the second strongest correlation (Supplementary Table S6). In addition, the four SNPs were also associated with FKBP5 gene expression changes in various tissues, including brain tissues (Supplementary Table S7). For instance, rs9470080 was associated with a significant increase in FKBP5 expression in induced pluripotent stem cells (p = 0.004).
Moreover, we also examined human brain normalized expression data of the aforementioned genes from the Allen Human Brain Atlas. Microarray data of six donors using four FKBP5 gene probes revealed that FKBP5 was predominantly expressed in the hippocampus and amygdala, which was consistent with previous literature showing that FKBP5 regulates the stress response mainly in the hippocampus and amygdala of the human and mouse brain. In addition, FKBP5 was expressed moderately in various neural pontine tegmentum nuclei and medullary nuclei (Figure 3), and neural circuits in the pons and medulla are known to control breathing during sleep, with the pontine tegmentum involved in the generation and maintenance of REM sleep (Cox et al., 2016). FKBP5 was also moderately expressed in the striatum, globus pallidus and ventral tegmental area located in the midbrain, all of which play a key role in wakefulness control (Eban-Rothschild et al., 2016; Luo et al., 2018; Yu et al., 2019; Dong et al., 2022). The expression of TULP1 was detected in several neurons in the hippocampus, subthalamus, midbrain and medulla, while ARMC12 expression was detected in some neurons in the striatum, midbrain and medulla (Supplementary Figure S3), indicating that TULP1 and ARMC12 may have potential roles in the regulation of sleep structure likewise. Taken together, these results suggested that the four SNPs may exert their function in sleep breathing regulation by affecting FKBP5, TULP1 and ARMC12. However, this speculation still requires further confirmation from experiments to determine whether these genes play a part in the regulation of breathing in REM sleep and wakefulness.
Figure 3. Expression of FKBP5 in human brains from the Allen Brain Atlas. Probe1 A_23_P111206, Probe2 A_24_P38081, Probe3 CUST_1369_PI416573500, Probe4 CUST_1412_PI416379584.
In the current study, we identified the association between FKBP5 SNPs and quantitative phenotypic variables in a large-scale Chinese OSA cohort study. Rs1360780, rs3800373, rs9296158, and rs9470080 are all positively associated with AHIREM, which suggests that carriers of psychiatric-related risk alleles are prone to suffering from sleep breathing events, especially in the REM sleep stage in OSA patients. FKBP5 variants potentially contribute to sleep breathing disorders.
Another finding in our study regarding sleep architecture showed that three of the four SNPs (rs1360780, rs3800373 and rs9470080) were positively associated with wake time. In addition, two SNPs (rs1360780 and rs3800373) were associated with the microarousal index, and the other two (rs9296158 and rs9470080) increased the risk of lower sleep efficiency in the male moderate OSA group. Considering that wake time during sleep period time and microarousal are microscopic indicators of sleep fragmentation, our results therefore suggested that FKBP5 SNPs may promote sleep fragmentation in moderate OSA. However, these findings were not observed in the severe OSA group, possibly because factors including age and BMI are more important risk factors than genetic variants for sleep architecture in severe OSA, and therefore, the correlation of FKBP5 variants with sleep parameters is not strong enough for the prediction of regression models. Additionally, the sample size of female participants diagnosed with moderate OSA might be too small to blame for the irrelevant findings in their female counterparts.
Posttraumatic stress disorder, depression and OSA share sleep disturbance as a common typical feature. Several studies have performed polysomnographic recording to examine the sleep patterns in PTSD and depression (Lavie, 2001; van Liempt et al., 2013; Baglioni et al., 2016; Zhang et al., 2019), which were characterized by a highly aroused state and fragmented sleep. Specific pathophysiological mechanisms underlying the relationship between psychiatric disorders and OSA remain unknown. Krakow et al. (2015) proposed a bidirectional pathway theory model assuming that nightmares and arousals occurring in PTSD result in sleep fragmentation concomitantly, leading to the development of OSA, which may in turn worsen PTSD symptoms. Sleep fragmentation significantly decreases pharyngeal critical closing pressure (Pcrit) corresponding to increased airway collapsibility (Sériès et al., 1994), leading to more sleep breathing events and higher susceptibility to OSA, predominantly in the REM stage. Obstructive respiratory events occur more frequently and with longer duration during REM sleep than NREM sleep in OSA due to increased airway collapsibility caused by reduced pharyngeal muscle response and respiratory effort throughout REM sleep (Findley et al., 1985; Shea et al., 1999). Coincidently, our finding that FKBP5 variants were associated with respiratory events only during REM sleep suggested a common genetic background underpinning the comorbidity of OSA and PTSD/depression. However, whether FKBP5 SNPs could function through Krakow’s model as common genetic factors still requires further functional verification. In addition, Tseng et al. (2022) recently demonstrated that stress can elicit arousal more rapidly from REM sleep compared to NREM sleep, and hypoxia is a stress stimulus caused by airway obstruction in OSA pathophysiology, which may explain why the association in our study was only in OSA patients who were under hypoxic stress but not in the non-OSA group.
Perhaps the association of FKBP5 variants with sleep disorder was related to the biological role of FKBP5 in HPA-axis functioning within the neuroendocrine system. FKBP5 regulates the sensitivity of glucocorticoid receptor (GR) by reducing its cortisol-binding affinity and nuclear translocation, modulating the negative feedback of glucocorticoids to stabilize HPA-axis glucocorticoid output (Dwyer et al., 2020). The HPA axis regulates many vital pathways, including the stress response and sleep regulation, and has been postulated as a possible mechanism for the link between OSA and PTSD/depression (Buckley and Schatzberg, 2005; Gupta and Simpson, 2015; Jaoude et al., 2015). The HPA axis is necessary for maintaining alertness and sleep modulation, and OSA is also correlated with HPA axis dysfunction interactively (Buckley and Schatzberg, 2005). Hyperactivation of the HPA axis can cause more arousals, awakenings and lighter sleep (Nicolaides et al., 2000), which promotes sleep events in OSA, while secondary arousals following intermittent hypoxia episodes can induce HPA activation in turn (Späth-Schwalbe et al., 1991; Buckley and Schatzberg, 2005; Kritikou et al., 2016). Notably, it was also observed that nondepressed offspring with a parental history of depression showed higher cortisol awakening curves, indicating that HPA-axis hyperactivity may partly reflect a genetic susceptibility marker for depression (Vreeburg et al., 2010). In our study, the heritability of HPA activity may be presented by FKBP5 variations in OSA patients. Previous genetic studies have identified that FKBP5 polymorphisms rs1360780, rs9470080, and rs9394309 were related to dysfunctional HPA activity (Velders et al., 2011; White et al., 2012; Fani et al., 2013; Pagliaccio et al., 2014). However, it remains unknown whether FKBP5 variants could regulate sleep and act as a shared genetic factor for the comorbidity by influencing HPA activity or by other biological mechanisms, which merits further biological and clinical investigation. In addition, whether the variants were correlated with other HPA-axis physiological functions, including nutrition metabolism regulation in OSA, was not clear either. Additionally, integrated analysis of eQTL, 3D interacting gene analysis and gene expression patterns revealed that these four SNPs may exert their effects by regulating FKBP5, TULP1, and ARMC12. We found that several genes other than FKBP5 were also affected by these four SNPs, especially TULP1 and ARMC12, both of which were also detected in neurons involved in sleep regulation. However, there has been no relevant report available on the relationship between the two genes and sleep disorders thus far. Tubby-like protein 1 (TULP1) is located approximately 60 kb downstream from FKBP5 and has been reported to be important for vesicular trafficking of photoreceptor proteins and associated with early-onset retinal degeneration (Remez et al., 2020; Jia et al., 2022). Armadillo repeat containing 12 (ARMC12) is located 3 kb upstream of FKBP5 and has been reported to promote neuroblastoma progression and play crucial roles in spermiogenesis (Li et al., 2018; Shimada et al., 2021). However, the mechanisms underlying the roles of FKBP5, TULP1 and ARMC12 in regulating sleep need further exploration.
Based on the previous studies presented above, we speculate that FKBP5 variations increase genetic vulnerability to depression and PTSD, and its potential role in prolonged HPA axis activation may result in a higher hyperarousal state than non-risk allele carriers. Resultant greater sleep fragmentation tends to increase upper airway collapsibility, leading to more sleep breathing events, especially in REM sleep. It is postulated that the two types of diseases may be reciprocal catalysts for each other through possible genetic background overlap, such as FKBP5 variants. In summary, FKBP5 variants may connect psychiatric disturbances with OSA and influence OSA phenotypic traits via HPA dysregulation. Additionally, FKBP5 SNPs might affect sleep breathing via the FKBP5, TULP1, and ARMC12 genes.
There were several limitations in this study that should be noted. First, males are predominant in sex distribution among OSA patients, whereas adult women are more likely to develop depression and PTSD than adult men; hence, the sample size of female participants impeded us from analyzing the association comprehensively and in a gender-specific way, and the findings were more generalizable to men than to women. Second, we discussed only the role of the candidate FKBP5 gene without considering the effect of stress exposure and were unable to evaluate the classic gene by environment interactions in the FKBP5 gene. Third, we did not consider other complex genetic variations, including indels, DNA methylation and structural variation. The correlation between HPA axis activity and FKBP5 genotype in OSA patients has not been clarified to acquire a deeper understanding of the connection. Additionally, this study could not provide causative evidence due to its cross-sectional cohort design. Moreover, the study was limited to the Han Chinese population, and further studies are needed to validate whether these genetic variants contribute to OSA risk and related phenotypes in other ethnic groups. Clinical trials and functional experiments are needed to further reveal the mechanism of the FKBP5 gene and its variation in sleep regulation in the future. The pleiotropic effect of FKBP5 variants on sleep disorders is worth exploring because of its important biological functions.
The original contributions presented in the study are included in the article/Supplementary material, further inquiries can be directed to the corresponding authors.
The studies involving human participants were reviewed and approved by the Ethics Committee of Shanghai Sixth People’s Hospital Affiliated to Shanghai Jiao Tong University School of Medicine. The patients/participants provided their written informed consent to participate in this study.
XL, FL, and SY contributed to the conception and design of the study. XL organized the database. AW and ZW wrote the first draft of the manuscript. AW, ZW, and HY performed the statistical analysis. All authors commented on previous versions of the manuscript and approved the submitted version.
The work was supported by STI2030-Major Projects (2021ZD0201900); National Natural Science Foundation of China (82000967, 81971240, 82271153, 82100105); China Postdoctoral Science Foundation (2021M702175); and Shanghai Sixth People’s Hospital (ynts202103).
The authors would like to thank all the participants and acknowledge the work of the entire medical staff at the Department of Otolaryngology Head and Neck Surgery and Center of Sleep Medicine, Shanghai Sixth People’s Hospital Affiliated to Shanghai Jiao Tong University School of Medicine. The human brain gene expression data used for the analyses described in this manuscript were obtained from the Allen Brain Atlas.
The research was conducted in the absence of any commercial or financial relationships that could be construed as a potential conflict of interest.
All claims expressed in this article are solely those of the authors and do not necessarily represent those of their affiliated organizations, or those of the publisher, the editors and the reviewers. Any product that may be evaluated in this article, or claim that may be made by its manufacturer, is not guaranteed or endorsed by the publisher.
The Supplementary material for this article can be found online at: https://www.frontiersin.org/articles/10.3389/fnins.2023.1170889/full#supplementary-material
Baglioni, C., Nanovska, S., Regen, W., Spiegelhalder, K., Feige, B., Nissen, C., et al. (2016). Sleep and mental disorders: a META-analysis of polysomnographic research. Psychol. Bull. 142, 969–990. doi: 10.1037/bul0000053
Barrett, J. C., Fry, B., Maller, J., and Daly, M. J. (2005). Haploview: analysis and visualization of LD and haplotype maps. Bioinformatics 21, 263–265. doi: 10.1093/bioinformatics/bth457
Benjafield, A. V., Ayas, N. T., Eastwood, P. R., Heinzer, R., Ip, M. S. M., Morrell, M. J., et al. (2019). Estimation of the global prevalence and burden of obstructive sleep apnoea: a literature-based analysis. Lancet Respir. Med. 7, 687–698. doi: 10.1016/S2213-2600(19)30198-5
Berry, R. B., Budhiraja, R., Gottlieb, D. J., Gozal, D., Iber, C., Kapur, V. K., et al. (2012). Rules for scoring respiratory events in sleep: update of the 2007 AASM manual for the scoring of sleep and associated events. J. Clin. Sleep Med. 8, 597–619. doi: 10.5664/jcsm.2172
Binder, E. B. (2009). The role of FKBP5, a co-chaperone of the glucocorticoid receptor in the pathogenesis and therapy of affective and anxiety disorders. Psychoneuroendocrinology 34, S186–S195. doi: 10.1016/j.psyneuen.2009.05.021
Binder, E. B., Bradley, R. G., Liu, W., Epstein, M. P., Deveau, T. C., Mercer, K. B., et al. (2008). Association of FKBP5 polymorphisms and childhood abuse with risk of posttraumatic stress disorder symptoms in adults. JAMA 299, 1291–1305. doi: 10.1001/jama.299.11.1291
Binder, E. B., Salyakina, D., Lichtner, P., Wochnik, G. M., Ising, M., Pütz, B., et al. (2004). Polymorphisms in FKBP5 are associated with increased recurrence of depressive episodes and rapid response to antidepressant treatment. Nat. Genet. 36, 1319–1325. doi: 10.1038/ng1479
Bortsov, A. V., Smith, J. E., Diatchenko, L., Soward, A. C., Ulirsch, J. C., Rossi, C., et al. (2013). Polymorphisms in the glucocorticoid receptor co-chaperone FKBP5 predict persistent musculoskeletal pain after traumatic stress exposure. Pain 154, 1419–1426. doi: 10.1016/j.pain.2013.04.037
Buckley, T. M., and Schatzberg, A. F. (2005). On the interactions of the hypothalamic-pituitary-adrenal (HPA) axis and sleep: normal HPA axis activity and circadian rhythm, exemplary sleep disorders. J. Clin. Endocrinol. Metab. 90, 3106–3114. doi: 10.1210/jc.2004-1056
Colvonen, P. J., Straus, L. D., Stepnowsky, C., McCarthy, M. J., Goldstein, L. A., and Norman, S. B. (2018). Recent advancements in treating sleep disorders in co-occurring PTSD. Curr. Psychiatry Rep. 20:48. doi: 10.1007/s11920-018-0916-9
Cox, J., Pinto, L., and Dan, Y. (2016). Calcium imaging of sleep–wake related neuronal activity in the dorsal pons. Nat. Commun. 7:10763. doi: 10.1038/ncomms10763
Dong, H., Chen, Z.-K., Guo, H., Yuan, X.-S., Liu, C.-W., Qu, W.-M., et al. (2022). Striatal neurons expressing dopamine D1 receptor promote wakefulness in mice. Curr. Biol. 32, 600–613.e4. doi: 10.1016/j.cub.2021.12.026
Dwyer, J. B., Aftab, A., Radhakrishnan, R., Widge, A., Rodriguez, C. I., Carpenter, L. L., et al. (2020). Hormonal treatments for major depressive disorder: state of the art. Am. J. Psychiatry 177, 686–705. doi: 10.1176/appi.ajp.2020.19080848
Eban-Rothschild, A., Rothschild, G., Giardino, W. J., Jones, J. R., and de Lecea, L. (2016). VTA dopaminergic neurons regulate ethologically relevant sleep-wake behaviors. Nat. Neurosci. 19, 1356–1366. doi: 10.1038/nn.4377
Fani, N., Gutman, D., Tone, E. B., Almli, L., Mercer, K. B., Davis, J., et al. (2013). FKBP5 and attention bias for threat: associations with hippocampal function and shape. JAMA Psychiat. 70, 392–400. doi: 10.1001/2013.jamapsychiatry.210
Findley, L. J., Wilhoit, S. C., and Suratt, P. M. (1985). Apnea duration and hypoxemia during REM sleep in patients with obstructive sleep apnea. Chest 87, 432–436. doi: 10.1378/chest.87.4.432
Gabriel, S. B., Schaffner, S. F., Nguyen, H., Moore, J. M., Roy, J., Blumenstiel, B., et al. (2002). The structure of haplotype blocks in the human genome. Science 296, 2225–2229. doi: 10.1126/science.1069424
Gozal, D., Serpero, L. D., Sans Capdevila, O., and Kheirandish-Gozal, L. (2008). Systemic inflammation in non-obese children with obstructive sleep apnea. Sleep Med. 9, 254–259. doi: 10.1016/j.sleep.2007.04.013
Guan, J., Yi, H., Zou, J., Meng, L., Tang, X., Zhu, H., et al. (2016). Distinct severity stages of obstructive sleep apnoea are correlated with unique dyslipidaemia: large-scale observational study. Thorax 71, 347–355. doi: 10.1136/thoraxjnl-2015-207403
Gupta, M. A., and Simpson, F. C. (2015). Obstructive sleep apnea and psychiatric disorders: a systematic review. J. Clin. Sleep Med. 11, 165–175. doi: 10.5664/jcsm.4466
Habukawa, M., Uchimura, N., Kakuma, T., Yamamoto, K., Ogi, K., Hiejima, H., et al. (2010). Effect of CPAP treatment on residual depressive symptoms in patients with major depression and coexisting sleep apnea: contribution of daytime sleepiness to residual depressive symptoms. Sleep Med. 11, 552–557. doi: 10.1016/j.sleep.2010.02.007
Hähle, A., Merz, S., Meyners, C., and Hausch, F. (2019). The many faces of FKBP51. Biomol. Ther. 9:E35. doi: 10.3390/biom9010035
Hattori, M., Kitajima, T., Mekata, T., Kanamori, A., Imamura, M., Sakakibara, H., et al. (2009). Risk factors for obstructive sleep apnea syndrome screening in mood disorder patients. Psychiatry Clin. Neurosci. 63, 385–391. doi: 10.1111/j.1440-1819.2009.01956.x
Jaoude, P., Vermont, L. N., Porhomayon, J., and El-Solh, A. A. (2015). Sleep-disordered breathing in patients with post-traumatic stress disorder. Annals ATS 12, 259–268. doi: 10.1513/AnnalsATS.201407-299FR
Jia, D., Gao, P., Lv, Y., Huang, Y., Reilly, J., Sun, K., et al. (2022). Tulp1 deficiency causes early-onset retinal degeneration through affecting ciliogenesis and activating ferroptosis in zebrafish. Cell Death Dis. 13:962. doi: 10.1038/s41419-022-05372-w
Johns, M. W. (1991). A new method for measuring daytime sleepiness: the Epworth sleepiness scale. Sleep 14, 540–545. doi: 10.1093/sleep/14.6.540
Klengel, T., Mehta, D., Anacker, C., Rex-Haffner, M., Pruessner, J. C., Pariante, C. M., et al. (2013). Allele-specific FKBP5 DNA demethylation mediates gene-childhood trauma interactions. Nat. Neurosci. 16, 33–41. doi: 10.1038/nn.3275
Krakow, B. J., Ulibarri, V. A., Moore, B. A., and McIver, N. D. (2015). Posttraumatic stress disorder and sleep-disordered breathing: a review of comorbidity research. Sleep Med. Rev. 24, 37–45. doi: 10.1016/j.smrv.2014.11.001
Kritikou, I., Basta, M., Vgontzas, A. N., Pejovic, S., Fernandez-Mendoza, J., Liao, D., et al. (2016). Sleep apnoea and the hypothalamic-pituitary-adrenal axis in men and women: effects of continuous positive airway pressure. Eur. Respir. J. 47, 531–540. doi: 10.1183/13993003.00319-2015
Lavie, P. (2001). Sleep disturbances in the wake of traumatic events. N. Engl. J. Med. 345, 1825–1832. doi: 10.1056/NEJMra012893
Lévy, P., Kohler, M., McNicholas, W. T., Barbé, F., McEvoy, R. D., Somers, V. K., et al. (2015). Obstructive sleep apnoea syndrome. Nat. Rev. Dis. Primers. 1:15015. doi: 10.1038/nrdp.2015.15
Li, D., Song, H., Mei, H., Fang, E., Wang, X., Yang, F., et al. (2018). Armadillo repeat containing 12 promotes neuroblastoma progression through interaction with retinoblastoma binding protein 4. Nat. Commun. 9:2829. doi: 10.1038/s41467-018-05286-2
Lopresti, A. L., and Drummond, P. D. (2013). Obesity and psychiatric disorders: commonalities in dysregulated biological pathways and their implications for treatment. Prog. Neuro-Psychopharmacol. Biol. Psychiatry 45, 92–99. doi: 10.1016/j.pnpbp.2013.05.005
Luo, Y.-J., Li, Y.-D., Wang, L., Yang, S.-R., Yuan, X.-S., Wang, J., et al. (2018). Nucleus accumbens controls wakefulness by a subpopulation of neurons expressing dopamine D1 receptors. Nat. Commun. 9:1576. doi: 10.1038/s41467-018-03889-3
Matosin, N., Halldorsdottir, T., and Binder, E. B. (2018). Understanding the molecular mechanisms underpinning gene by environment interactions in psychiatric disorders: the FKBP5 model. Biol. Psychiatry 83, 821–830. doi: 10.1016/j.biopsych.2018.01.021
Nicolaides, N. C., Vgontzas, A. N., Kritikou, I., Chrousos, G., Feingold, K. R., Anawalt, B., et al. (2000). “HPA Axis and sleep,” in Endotext, (eds.) K. R. Feingold, B. Anawalt, A. Boyce, G. Chrousos, W. W. Herderde, and K. Dhatariya, et al. South Dartmouth, MA: MDText.com, Inc.
Pagliaccio, D., Luby, J. L., Bogdan, R., Agrawal, A., Gaffrey, M. S., Belden, A. C., et al. (2014). Stress-system genes and life stress predict cortisol levels and amygdala and hippocampal volumes in children. Neuropsychopharmacology 39, 1245–1253. doi: 10.1038/npp.2013.327
Purcell, S., Neale, B., Todd-Brown, K., Thomas, L., Ferreira, M. A. R., Bender, D., et al. (2007). PLINK: a tool set for whole-genome association and population-based linkage analyses. Am. J. Hum. Genet. 81, 559–575. doi: 10.1086/519795
Remez, L., Cohen, B., Nevet, M. J., Rizel, L., and Ben-Yosef, T. (2020). TULP1 and TUB are required for specific localization of PRCD to photoreceptor outer segments. Int. J. Mol. Sci. 21:8677. doi: 10.3390/ijms21228677
Sériès, F., Roy, N., and Marc, I. (1994). Effects of sleep deprivation and sleep fragmentation on upper airway collapsibility in normal subjects. Am. J. Respir. Crit. Care Med. 150, 481–485. doi: 10.1164/ajrccm.150.2.8049833
Sharafkhaneh, A., Giray, N., Richardson, P., Young, T., and Hirshkowitz, M. (2005). Association of psychiatric disorders and sleep apnea in a large cohort. Sleep 28, 1405–1411. doi: 10.1093/sleep/28.11.1405
Shea, S. A., Edwards, J. K., and White, D. P. (1999). Effect of wake–sleep transitions and rapid eye movement sleep on pharyngeal muscle response to negative pressure in humans. J. Physiol. 520, 897–908. doi: 10.1111/j.1469-7793.1999.00897.x
Shimada, K., Park, S., Miyata, H., Yu, Z., Morohoshi, A., Oura, S., et al. (2021). ARMC12 regulates spatiotemporal mitochondrial dynamics during spermiogenesis and is required for male fertility. Proc. Natl. Acad. Sci. U. S. A. 118:e2018355118. doi: 10.1073/pnas.2018355118
Smoller, J. W. (2016). The genetics of stress-related disorders: PTSD, depression, and anxiety disorders. Neuropsychopharmacology 41, 297–319. doi: 10.1038/npp.2015.266
Späth-Schwalbe, E., Gofferje, M., Kern, W., Born, J., and Fehm, H. L. (1991). Sleep disruption alters nocturnal ACTH and cortisol secretory patterns. Biol. Psychiatry 29, 575–584. doi: 10.1016/0006-3223(91)90093-2
Stubbs, B., Vancampfort, D., Veronese, N., Solmi, M., Gaughran, F., Manu, P., et al. (2016). The prevalence and predictors of obstructive sleep apnea in major depressive disorder, bipolar disorder and schizophrenia: a systematic review and meta-analysis. J. Affect. Disord. 197, 259–267. doi: 10.1016/j.jad.2016.02.060
Tamanna, S., Parker, J. D., Lyons, J., and Ullah, M. I. (2014). The effect of continuous positive air pressure (CPAP) on nightmares in patients with posttraumatic stress disorder (PTSD) and obstructive sleep apnea (OSA). J. Clin. Sleep Med. 10, 631–636. doi: 10.5664/jcsm.3786
Tauman, R., Lavie, L., Greenfeld, M., and Sivan, Y. (2014). Oxidative stress in children with obstructive sleep apnea syndrome. J. Clin. Sleep Med. 10, 677–681. doi: 10.5664/jcsm.3800
Tseng, Y.-T., Zhao, B., Chen, S., Ye, J., Liu, J., Liang, L., et al. (2022). The subthalamic corticotropin-releasing hormone neurons mediate adaptive REM-sleep responses to threat. Neuron 110, 1223–1239.e8. doi: 10.1016/j.neuron.2021.12.033
van Liempt, S., Arends, J., Cluitmans, P. J. M., Westenberg, H. G. M., Kahn, R. S., and Vermetten, E. (2013). Sympathetic activity and hypothalamo-pituitary-adrenal axis activity during sleep in post-traumatic stress disorder: a study assessing polysomnography with simultaneous blood sampling. Psychoneuroendocrinology 38, 155–165. doi: 10.1016/j.psyneuen.2012.05.015
Velders, F. P., Kuningas, M., Kumari, M., Dekker, M. J., Uitterlinden, A. G., Kirschbaum, C., et al. (2011). Genetics of cortisol secretion and depressive symptoms: a candidate gene and genome wide association approach. Psychoneuroendocrinology 36, 1053–1061. doi: 10.1016/j.psyneuen.2011.01.003
Vreeburg, S. A., Hartman, C. A., Hoogendijk, W. J. G., van Dyck, R., Zitman, F. G., Ormel, J., et al. (2010). Parental history of depression or anxiety and the cortisol awakening response. Br. J. Psychiatry 197, 180–185. doi: 10.1192/bjp.bp.109.076869
White, M. G., Bogdan, R., Fisher, P. M., Muñoz, K. E., Williamson, D. E., and Hariri, A. R. (2012). FKBP5 and emotional neglect interact to predict individual differences in amygdala reactivity. Genes Brain Behav. 11, 869–878. doi: 10.1111/j.1601-183X.2012.00837.x
Xie, P., Kranzler, H. R., Poling, J., Stein, M. B., Anton, R. F., Farrer, L. A., et al. (2010). Interaction of FKBP5 with childhood adversity on risk for post-traumatic stress disorder. Neuropsychopharmacology 35, 1684–1692. doi: 10.1038/npp.2010.37
Xu, H., Liu, F., Li, Z., Li, X., Liu, Y., Li, N., et al. (2022). Genome-wide association study of obstructive sleep apnea and objective sleep-related traits identifies novel risk loci in Han Chinese individuals. Am. J. Respir. Crit. Care Med. 206, 1534–1545. doi: 10.1164/rccm.202109-2044OC
Yu, X., Li, W., Ma, Y., Tossell, K., Harris, J. J., Harding, E. C., et al. (2019). GABA and glutamate neurons in the VTA regulate sleep and wakefulness. Nat. Neurosci. 22, 106–119. doi: 10.1038/s41593-018-0288-9
Zannas, A. S., and Binder, E. B. (2014). Gene-environment interactions at the FKBP5 locus: sensitive periods, mechanisms and pleiotropism. Genes Brain Behav. 13, 25–37. doi: 10.1111/gbb.12104
Zhang, Y., Ren, R., Sanford, L. D., Yang, L., Zhou, J., Zhang, J., et al. (2019). Sleep in posttraumatic stress disorder: a systematic review and meta-analysis of polysomnographic findings. Sleep Med. Rev. 48:101210. doi: 10.1016/j.smrv.2019.08.004
Zhang, Y., Weed, J. G., Ren, R., Tang, X., and Zhang, W. (2017). Prevalence of obstructive sleep apnea in patients with posttraumatic stress disorder and its impact on adherence to continuous positive airway pressure therapy: a meta-analysis. Sleep Med. 36, 125–132. doi: 10.1016/j.sleep.2017.04.020
Zimmermann, P., Brückl, T., Nocon, A., Pfister, H., Binder, E. B., Uhr, M., et al. (2011). Interaction of FKBP5 gene variants and adverse life events in predicting depression onset: results from a 10-year prospective community study. Am. J. Psychiatry 168, 1107–1116. doi: 10.1176/appi.ajp.2011.10111577
Keywords: obstructive sleep apnea, depression, posttraumatic stress disorder, FKBP5 gene, single nucleotide polymorphism
Citation: Wang A, Wei Z, Yuan H, Zhu Y, Peng Y, Gao Z, Liu Y, Shen J, Xu H, Guan J, Yin S, Liu F and Li X (2023) FKBP5 genetic variants are associated with respiratory- and sleep-related parameters in Chinese patients with obstructive sleep apnea. Front. Neurosci. 17:1170889. doi: 10.3389/fnins.2023.1170889
Received: 21 February 2023; Accepted: 27 April 2023;
Published: 18 May 2023.
Edited by:
Zhi-Li Huang, Fudan University, ChinaCopyright © 2023 Wang, Wei, Yuan, Zhu, Peng, Gao, Liu, Shen, Xu, Guan, Yin, Liu and Li. This is an open-access article distributed under the terms of the Creative Commons Attribution License (CC BY). The use, distribution or reproduction in other forums is permitted, provided the original author(s) and the copyright owner(s) are credited and that the original publication in this journal is cited, in accordance with accepted academic practice. No use, distribution or reproduction is permitted which does not comply with these terms.
*Correspondence: Shankai Yin, c2t5aW5Ac2p0dS5lZHUuY24=; Feng Liu, bGl1ZmVuZ0BzaWJzLmFjLmNu; Xinyi Li, bGl4aW55aWxpeGlueWkxMjNAMTYzLmNvbQ==
†These authors have contributed equally to this work and share first authorship
Disclaimer: All claims expressed in this article are solely those of the authors and do not necessarily represent those of their affiliated organizations, or those of the publisher, the editors and the reviewers. Any product that may be evaluated in this article or claim that may be made by its manufacturer is not guaranteed or endorsed by the publisher.
Research integrity at Frontiers
Learn more about the work of our research integrity team to safeguard the quality of each article we publish.