- 1School of Biomedical Engineering, Shanghai Jiao Tong University, Shanghai, China
- 2Department of Nuclear Medicine, Ruijin Hospital, Shanghai Jiao Tong University School of Medicine, Shanghai, China
Objective: To investigate the relationship between glucose metabolism and functional activity in the epileptogenic network of patients with mesial temporal lobe epilepsy (MTLE) and to determine whether this relationship is associated with surgical outcomes.
Methods: 18F-FDG PET and resting-state functional MRI (rs-fMRI) scans were performed on a hybrid PET/MR scanner in 38 MTLE patients with hippocampal sclerosis (MR-HS), 35 MR-negative patients and 34 healthy controls (HC). Glucose metabolism was measured using 18F-FDG PET standardized uptake value ratio (SUVR) relative to cerebellum; Functional activity was obtained by fractional amplitude of low-frequency fluctuation (fALFF). The betweenness centrality (BC) of metabolic covariance network and functional network were calculated using graph theoretical analysis. Differences in SUVR, fALFF, BC and the spatial voxel-wise SUVR-fALFF couplings of the epileptogenic network, consisting of default mode network (DMN) and thalamus, were evaluated by Mann-Whitney U test (using the false discovery rate [FDR] for multiple comparison correction). The top ten SUVR-fALFF couplings were selected by Fisher score to predict surgical outcomes using logistic regression model.
Results: The results showed decreased SUVR-fALFF coupling in the bilateral middle frontal gyrus (PFDR = 0.0230, PFDR = 0.0296) in MR-HS patients compared to healthy controls. Coupling in the ipsilateral hippocampus was marginally increased (PFDR = 0.0802) in MR-HS patients along with decreased BC of metabolic covariance network and functional network (PFDR = 0.0152; PFDR = 0.0429). With Fisher score ranking, the top ten SUVR-fALFF couplings in regions from DMN and thalamic subnuclei could predict surgical outcomes with the best performance being a combination of ten SUVR-fALFF couplings with an AUC of 0.914.
Conclusion: These findings suggest that the altered neuroenergetic coupling in the epileptogenic network is associated with surgical outcomes of MTLE patients, which may provide insight into their pathogenesis and help with preoperative evaluation.
Highlights
- Both metabolic network and functional network are altered in mesial temporal lobe epilepsy (MTLE) patients.
- Metabolic-functional coupling is altered in the epileptogenic network of MTLE.
- SUVR-fALFF coupling in DMN and thalamic subnuclei are predictive of surgical outcomes.
1. Introduction
Mesial temporal lobe epilepsy (MTLE) is the most common type of drug-resistant epilepsy (Engel et al., 2012), characterized by abnormal neuronal discharge that results in repeated seizures arising from hippocampus and related mesial temporal lobe structures, which also play a key role in seizures propagation, and hippocampal sclerosis (HS) represents the most common pathology underlying MTLE (Bullmore and Sporns, 2009). Although anterior temporal lobectomy (ATL) is widely accepted as an effective surgical strategy for MTLE patients, ~40% of them have a poor prognosis 2 years after surgery (Liu et al., 2018). Emerging evidence indicates that MTLE is a network-based disease that exhibits widespread abnormalities affecting networks such as the default mode network (DMN) (Pittau et al., 2012; Chassoux et al., 2016; Englot et al., 2017; Tsuda et al., 2018; Zanao et al., 2021). Thalamus, an integrative hub for functional brain networks (Hwang et al., 2017), plays an important role in the process of seizure spread in MTLE (Zhang and Bertram, 2002), and particularly medial thalamic nuclei are involved in the limbic seizure circuitry (Guye et al., 2006; Bertram et al., 2008).
The hybrid PET/MR allows simultaneous measurement of 18F-FDG PET and functional MRI (fMRI), which gives us the opportunity of studying metabolic-functional coupling of the human brain. A previous study in healthy volunteers has found that changes in glucose metabolism were related to changes in rs-fMRI fluctuations (Tomasi et al., 2013). Another healthy volunteer research identified a significant spatial correlation between standardized uptake value ratio (SUVR) and fMRI metrics across the gray matter and DMN (Aiello et al., 2015). Further study demonstrated that brain-wide correlations between SUVR and amplitude of low-frequency fluctuation (ALFF) were significantly lower in TLE patients (Nugent et al., 2015). ALFF reflects the amplitude of spontaneous neural activities and fractional ALFF (fALFF) is demonstrated to be a more robust index than ALFF (Zang et al., 2007; Zou et al., 2008). Wang et al. (2020) reported that the coupling between SUVR and fALFF across gray matter was higher in patients with MR-HS compared to healthy controls and higher in patients who had bad outcomes after surgery than good ones. However, it is unclear whether the coupling within local epileptogenic network is disturbed in MTLE patients.
Since increasing evidence suggests that MTLE is a network disease and graph theory provides a promising method to describe topological and organizational properties of complex network (Bullmore and Sporns, 2009), more and more studies apply graph theoretical analysis with fMRI data and FDG PET data to investigate the abnormalities of whole brain network and epileptogenic networks in MTLE. A MR-negative MTLE study demonstrated that MR-negative was associated with impaired interictal functional connectivity (FC) of the ipsilateral temporal cortex, measured by betweenness centrality (BC) in graph theoretical analysis (Vaughan et al., 2016). Zhou et al. (2019) indicated that patients with TLE showed a functional disrupted topological reorganization within the DMN, characterized by decreased degree centrality of some brain regions in DMN. In addition, a previous investigation provided another view of evidence that MTLE was a system neurological disorder with disrupted networks, using hippocampus-based and whole-brain metabolic covariance networks (Wang et al., 2019). We hypothesized that the coupling between FDG SUVR and fALFF might be altered in epileptogenic network of MTLE patients.
In this study, we investigated the changes in glucose uptake, functional activity, metabolic and functional network properties and SUVR-fALFF couplings in the epileptogenic network of MTLE using simultaneous PET/MR, and further evaluated whether the couplings could predict surgical outcomes.
2. Materials and methods
2.1. Participants
In this IRB-approved study, we recruited 97 refractory unilateral mesial temporal lobe epilepsy patients between August 2018 and November 2021, and 32 healthy controls (HC). The inclusion criteria of patients are as follows: (1) diagnosis of temporal lobe epilepsy based on International League Against Epilepsy (ILAE) criteria (Blumcke et al., 2013); (2) MRI either normal or disclosed patterns suggestive of HS confirmed by histopathology. The exclusion criteria include (1) other neurological system lesion or other psychiatric disorders; (2) bilateral hippocampal sclerosis. For all patients, a comprehensive set of clinical evaluation including neurologic history, diagnostic MRI, FDG PET and scalp video-electroencephalography (EEG) recordings were collected.
Twenty-four patients were further excluded due to incomplete or low-quality data: missing resting-state functional MRI or PET data (N = 13), artifact in rs-fMRI data (N = 1), abnormally high PET signal in the whole brain (N = 1), head motion exceeding 3 mm in translation or 3 degrees in rotation (N = 9) (Figure 1). Finally, 73 MTLE patients were included in further analysis. Further, MTLE patients who had 2 years follow-up visit after anterior temporal lobectomy (ATL) surgery without any other resection beyond temporal lobe were selected for subsequent surgical outcome prediction analysis. Outcomes were evaluated by the Engel Epilepsy Surgery Outcome Scale (Engel, 1993) by an epileptologist, in which patients with Engel class I were considered seizure-free and subjects with Engel class II–IV were regarded as non-seizure-free. Inclusion criteria for healthy controls include (1) free of psychiatric or neurologic disorders; (2) no contraindications for MRI or PET scanning.
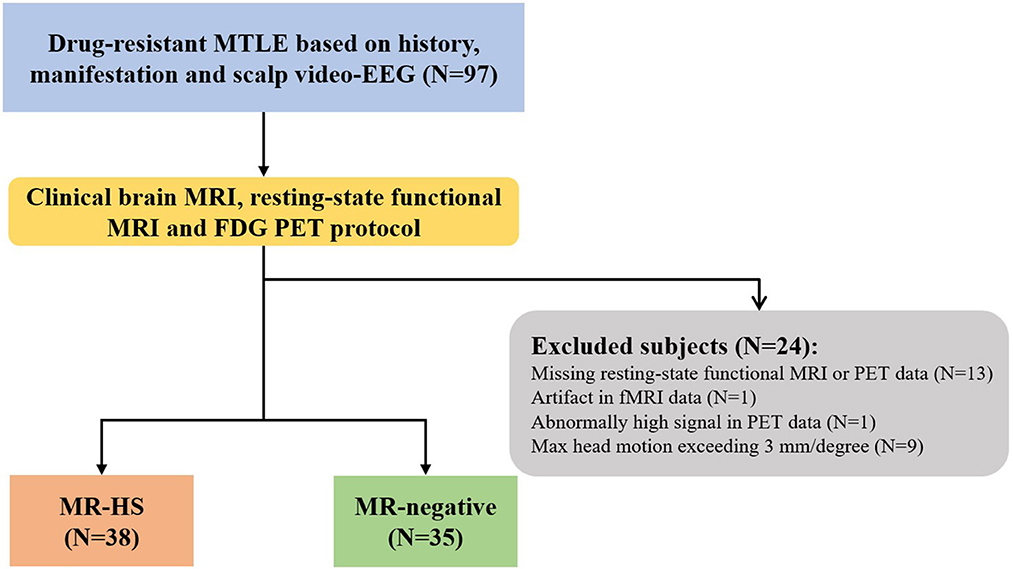
Figure 1. The flowchart of patient inclusion. MTLE, mesial temporal lobe epilepsy; HS, hippocampal sclerosis.
2.2. Data acquisition
All participants were scanned on a hybrid PET/MR scanner (Biograph mMR; Siemens Healthcare, Erlangen, Germany) using an 8-channel phase-array head coil. Note that, all patients discontinued anti-epileptic medicine for at least 24 h, and fast for 4–6 h, before the PET/MR scan. T1-weighted structural images were obtained employing a magnetization-prepared rapid gradient echo sequence (echo time = 2.44 ms, repetition time = 1,900 ms, flip angle = 9°, field of view = 250 mm × 250 mm, 192 slices per slab, slice thickness = 1.0 mm, gap = 0.5 mm, matrix = 256 × 256). T2-weighted FLAIR images were acquired with the following parameters: echo time = 92 ms, repetition time = 8,460 ms, flip angle = 150°, field of view = 220 mm × 220 mm, 45 slices per slab, slice thickness = 3.0 mm, gap = 0.4 mm, matrix = 256 × 256. Resting-state fMRI images were acquired using echo-planar imaging sequence (echo time = 30 ms, repetition time = 3,000 ms, flip angle = 90°, field of view = 192 mm × 192 mm, axial slices = 35, slice thickness = 3 mm, gap = 0.8 mm, matrix = 64 × 64, 200 time points). FDG PET images were obtained at 30–50 min post a bolus injection of 3.7 MBq/kg of 18F-FDG with the following parameters: 128 slices per slab, gap = 0.5 mm; matrix size = 344 × 344, reconstructed with 3D OSEM iterative reconstruction (subsets = 21, iterations = 4) and post-filtered with an isotropic full-width half-maximum (FWHM) Gaussian kernel of 2 mm.
2.3. Data preprocessing
The individual Desikan-Killiany parcellation and thalamic nuclei segmentations were obtained from T1-MPRAGE using FreeSurfer v7.0 package (Desikan et al., 2006; Iglesias et al., 2018), and individual T1-weighted images were resliced to an isotropic voxel size of 2 × 2 × 2 mm3. 18F-FDG PET images were preprocessed using the package of SPM12 (http://www.fil.ion.ucl.ac.uk/spm/software/spm12) in Matlab (R2019a). The images were co-registered to individual T1-weighted images. In order to reduce inter-subject variance before group analysis, registered FDG PET images were further normalized by cerebellar scaling to obtain SUVR images (Boscolo Galazzo et al., 2016; Lamarche et al., 2016; Wang et al., 2020), assuming little metabolic alterations in cerebellum in MTLE patients. Preprocessed PET images were further smoothed using a FWHM Gaussian kernel of 8 mm for DMN region analysis and 4 mm for subthalamic nuclei analysis.
The resting-state fMRI data were preprocessed using SPM12 and DPARSFA toolbox (Chao-Gan and Yu-Feng, 2010). After removing the first 10 volumes, the remaining fMRI images were realigned and slice-timing corrected. Subsequently, six head motion parameters, white matter and cerebrospinal fluid mean signals were regressed out as nuisance variables (Zuo et al., 2013). In addition, to take into account signal drifts that arise from scanner instability, linear trend was analogously removed from each voxel's time course. The images were then co-registered to individual T1-weighted images and smoothed using FWHM Gaussian kernels of 8 mm and 4 mm that matched the kernels for FDG PET. fALFF maps, which measures the relative contribution of specific low frequency range (0.01–0.08 HZ) oscillations to the whole frequency range of signal variations (Zou et al., 2008), were finally calculated.
The brain regions in Desikan-Killiany atlas were used to define DMN consisting of 28 brain regions according to previous studies (Desikan et al., 2006; Buckner et al., 2008; Andrews-Hanna et al., 2010; Kabbara et al., 2017; Buckner and DiNicola, 2019), and bilateral thalamus. We also segmented the DMN and thalamus in to 6 modules, as shown in Table 1. Based on the thalamic nuclei segmentations, we merged some small nuclei and then divided the thalamus into six large nuclei (see Table 2). Mean SUVR and fALFF of each region-of-interest (ROI) were extracted for subsequent analyses.
2.4. Network construction and graph theoretical analysis
The metabolic covariance network and functional network were constructed based on interregional metabolic correlation matrices and FC matrices, respectively. The nodes in two modality networks are both defined as 28 brain structures forming DMN plus bilateral thalamus. The interregional metabolic correlations across subjects between the SUVR of every pair of regions for each group, and FC between regions for each participant were calculated by the Pearson correlation coefficient as edges on networks. Negative metabolic and functional correlations were eliminated from further analysis (Tzourio-Mazoyer et al., 2002; Wang et al., 2015). We applied the network density range to the adjacent matrix to ensure the brain network of every subject contained the same number of sides (Xu et al., 2019), thus we constructed networks via a network density range of 5%-50% at an interval of 0.01 in concordance with another study (He et al., 2017).
Based on the constructed brain network, the topological network characteristics, BC was computed to describe the hubness of brain structures forming DMN and thalamus in MTLE patients. Nodes with high BC play the role of a gatekeeper to connect the nodes and sub-groups, so they can most frequently control information flows in the network (Burt, 2018). More precisely, BC of node k (i.e., pk) is formulated as follows:
where gij is the shortest paths linking pi and pj and gij(pk) is the shortest paths linking pi and pj that contains pk.
Then BC was calculated at each sparsity threshold, and we applied an area under the curve (AUC) approach to avoid the specific selection of a threshold and provided a summarized scalar for topological network characterization (Zhang et al., 2011; Kim et al., 2017).
2.5. Statistical analysis
Mann-Whitney U tests were used to perform all the group comparisons, and Chi-square was used to check gender difference between patient groups and HC group (using the false discovery rate [FDR] to correct for multiple comparisons). Effects of gender, age were removed using a multiple regression model. For SUVR and fALFF, group comparison was performed between MTLE patients and healthy controls in thalamus and brain regions of DMN. Spatial voxel-wise couplings between SUVR and fALFF were calculated using Spearman correlation coefficient in thalamus and DMN regions, and in thalamic nuclei. Group comparison of SUVR-fALFF couplings were performed between patient groups and HC group in thalamus and brain regions of DMN.
The AUC values of BC in functional network nodes and FC were compared between patient groups and HC group. Metabolic network metrics were validated by a bootstrap approach in the present study, by which 100 group-level metabolic covariance networks were generated for each group (Wang et al., 2019), which relied on random sampling with replacement in statistics (Roels et al., 2015), and between-group difference of BC and metabolic connectivity (MC) in metabolic covariance network were further obtained from 100 random bootstrap simulations. Before assessing the coupling between spontaneous neural activities strength and glucose metabolism, SUVR and fALFF maps were linearly standardized into Z-values by subtracting from the mean value of voxels from the individual's whole-brain mask (created by applying a 50% threshold onto the SPM's priori brain mask), and then dividing this difference by the standard deviation across brain mask voxels. Since both the FDG PET contrast and BOLD signal arise mainly from gray matter, to assess as much as possible the “pure” correlation between SUVR and fALFF maps, partial correlation analysis was performed, in which the gray matter fraction was regressed out voxel-wise from the Z-scores for each modality. Therefore, partial correlation coefficient was measured as Spearman ρ between the residuals from the linear regression of SUVR and fALFF map, and the GM fraction map.
We further investigated the predictive value of intermodality correlation for surgical outcomes. Firstly, we used Fisher score for feature selection to select the top ten couplings with higher scores as the optimal subset for further analysis (Gu et al., 2012). To evaluate the performance of altered SUVR-fALFF coupling in the surgical outcome prediction, logistic regression model was used to discriminate seizure-free and non-seizure-free groups, with leave-one-out cross-validation. The hyper-parameters of the model were optimized using a gradient search strategy in the training data with Python scikit-learn. The AUC of receiver operating characteristic curve (ROC) was calculated as a quantized measurement of the predictive performance. The data analysis framework of the study is shown in Figure 2.
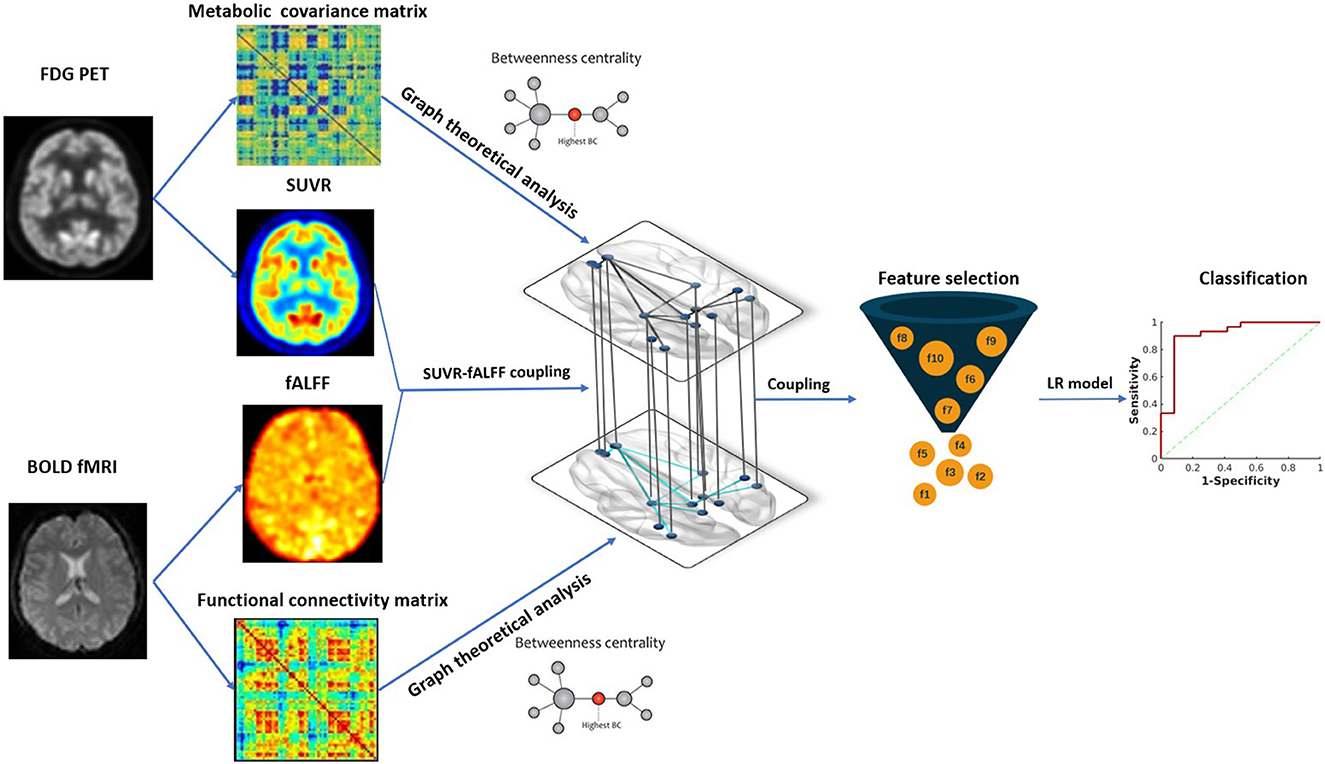
Figure 2. The framework of data analysis. Image features from hybrid FDG PET/fMRI imaging were extracted, including SUVR, fALFF and BC in metabolic and functional network. Metabolic-functional couplings were calculated by SUVR and fALFF map. All the image features and coulings were subsequently compared between MTLE patients and HC. SUVR-fALFF couplings were used for further surgical outcome prediction by Fisher score algorithm for feature selection and logistic regression model for classification. SUVR, standardized uptake value ratio; fALFF, fractional amplitude of low-frequency fluctuation; BC, betweenness centrality; LR, logistic regression.
3. Results
3.1. Patient demographics
Detailed demographics and clinical characteristics of all participants were listed in Table 3. Thirty-eight patients showed hippocampal sclerosis on routine MR images, and 35 were MR-negative. Of these 30 MTLE patients were seizure-free, 12 MTLE patients were non-seizure-free. Healthy controls were older than patients (P < 0.001). Thus, the group comparisons were performed after controlling for age by considering them as covariates.
3.2. Altered glucose uptake and metabolic covariance network properties in MTLE patients
Both MR-HS and MR-negative groups had significantly reduced SUVR in temporal structures, compared with HC. In MR-HS patients, decreased SUVR was mainly located in the ipsilateral hippocampus, thalamus, temporal cortex, lateral orbitofrontal and isthmus cingulate gyrus, whereas higher SUVR was found in the bilateral middle frontal gyrus, contralateral inferior parietal cortex. MR-negative subjects had lower SUVR in the bilateral hippocampus, thalamus, temporal cortex, orbitofrontal, isthmus cingulate gyrus, contralateral medial orbitofrontal and anterior cingulate gyrus than HC, while elevated SUVR was observed in the contralateral inferior parietal cortex (Figure 3A, Supplementary Table 1). MR-HS and MR-negative subjects had a majority of reduced intramodular and intermodular MCs in the module of temporal cortex, while increased intramodular and intermodular MCs were found in other areas of DMN, and MR-HS patients showed more increased MCs than MR-negative patients (Figure 3B). Compared to HC, MR-HS patients showed decreased BC in ipsilateral hippocampus (PFDR = 0.0152), thalamus (PFDR< 0.001), bilateral lateral orbitofrontal (PFDR< 0.001) and medial orbitofrontal (PFDR< 0.001), and increased BC in contralateral hippocampus (PFDR< 0.001) and thalamus (PFDR< 0.001); MR-negative patients had reduced BC in ipsilateral hippocampus (PFDR = 0.0125), thalamus (PFDR< 0.001) and bilateral medial orbitofrontal (PFDR = 0.00046, PFDR< 0.001), and increased BC in contralateral hippocampus (PFDR< 0.001), thalamus (PFDR< 0.001) and lateral orbitofrontal (PFDR< 0.001) (Figure 3C, Supplementary Table 2). Compared with HC group, MR-HS and MR-negative patients showed altered metabolic connectivity and altered Betweenness Centrality (Figure 3D).
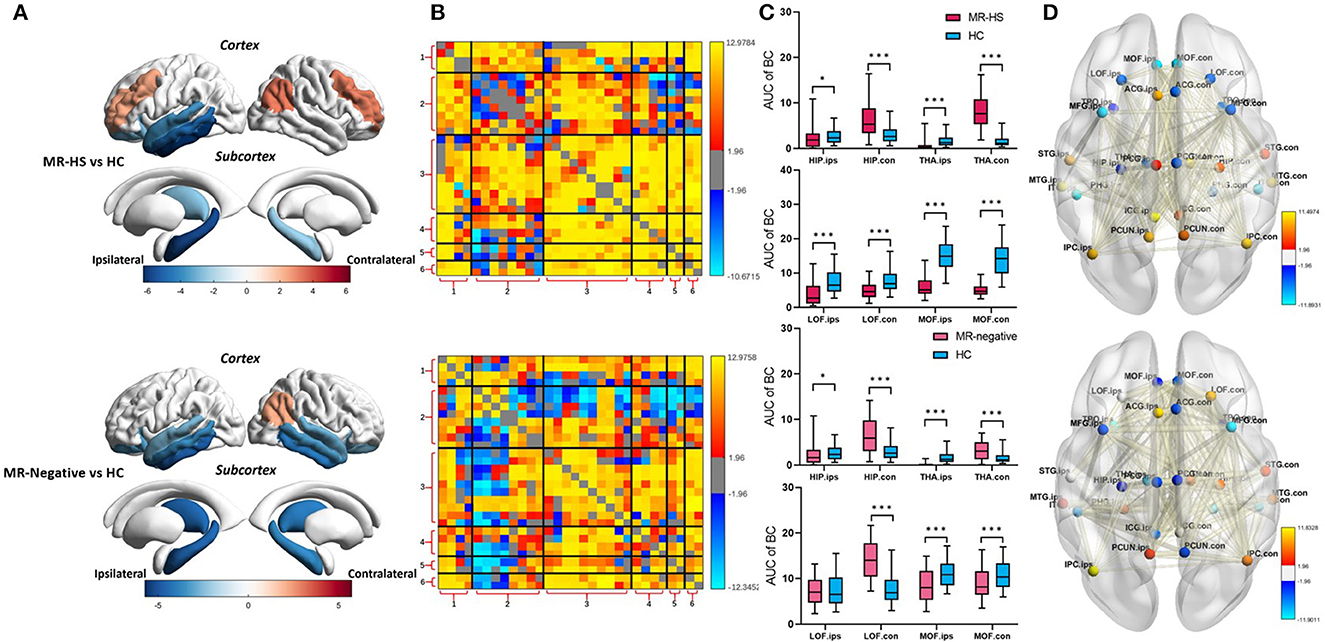
Figure 3. (A) SUVR, (B) MC, (C) BC, and (D) BC and MC of brain regions with significant differences in patients, compared with healthy controls. Statistical Z values of significant results of (A) SUVR, (B) MC, and (D) BC and MC were mapped into the corresponding regions or edges with thickness. Warm color represents a significant increase in patient groups, while cold color shows a significantly decreased trend. MR-HS, MTLE patients with hippocampal sclerosis; HC, healthy controls; SUVR, standardized uptake value ratio; BC, betweenness centrality; MC, metabolic connectivity; HIP, hippocampus; PHG, parahippocampal gyrus; ITG, inferior temporal gyrus; MTG, middle temporal gyrus; STG, superior temporal gyrus; TPO, temporal pole; LOF, lateral orbitofrontal; MOF, medial orbitofrontal; MFG, middle frontal gyrus; ICG, isthmus cingulate gyrus; ACG, anterior cingulate gyrus; PCG, posterior cingulate gyrus; PCUN, precuneus; IPC, inferior parietal cortex; THA, thalamus; ips, ipsilateral; ips, ipsilateral; con, contralateral; 1, medial temporal lobe; 2, temporal cortex; 3, dorsal and ventral medial prefrontal cortex; 4, precuneus/posterior cingulate cortex; 5, inferior parietal lobe; 6, thalamus; *PFDR < 0.05, **PFDR < 0.01, ***PFDR < 0.001.
3.3. Reduced functional activity and altered functional network properties in MTLE patients
Reduced fALFF in bilateral middle frontal gyrus were found in MR-negative subjects (PFDR< 0.001) (Supplementary Table 1). MR-HS and MR-negative patients both had increased FCs in most regions of DMN, whereas MR-HS showed reduced FCs between ipsilateral hippocampus and regions in dorsal and ventral medial prefrontal cortex (Figure 4A). Compared to HC, MR-HS patients showed decreased BC in ipsilateral hippocampus (PFDR = 0.0429), bilateral thalamus (PFDR = 0.0222, PFDR = 0.0429) and increased BC in contralateral medial orbitofrontal (PFDR = 0.0177); MR-negative subjects had reduced BC in ipsilateral hippocampus (PFDR = 0.0216), bilateral thalamus (PFDR < 0.001, PFDR = 0.0013) and marginally decreased BC in contralateral lateral orbitofrontal (PFDR = 0.0540) (Figure 4B, Supplementary Table 2). Compared to HC group, MR-HS and MR-negative patients showed altered functional connectivity and Betweenness Centrality (Figure 4C).
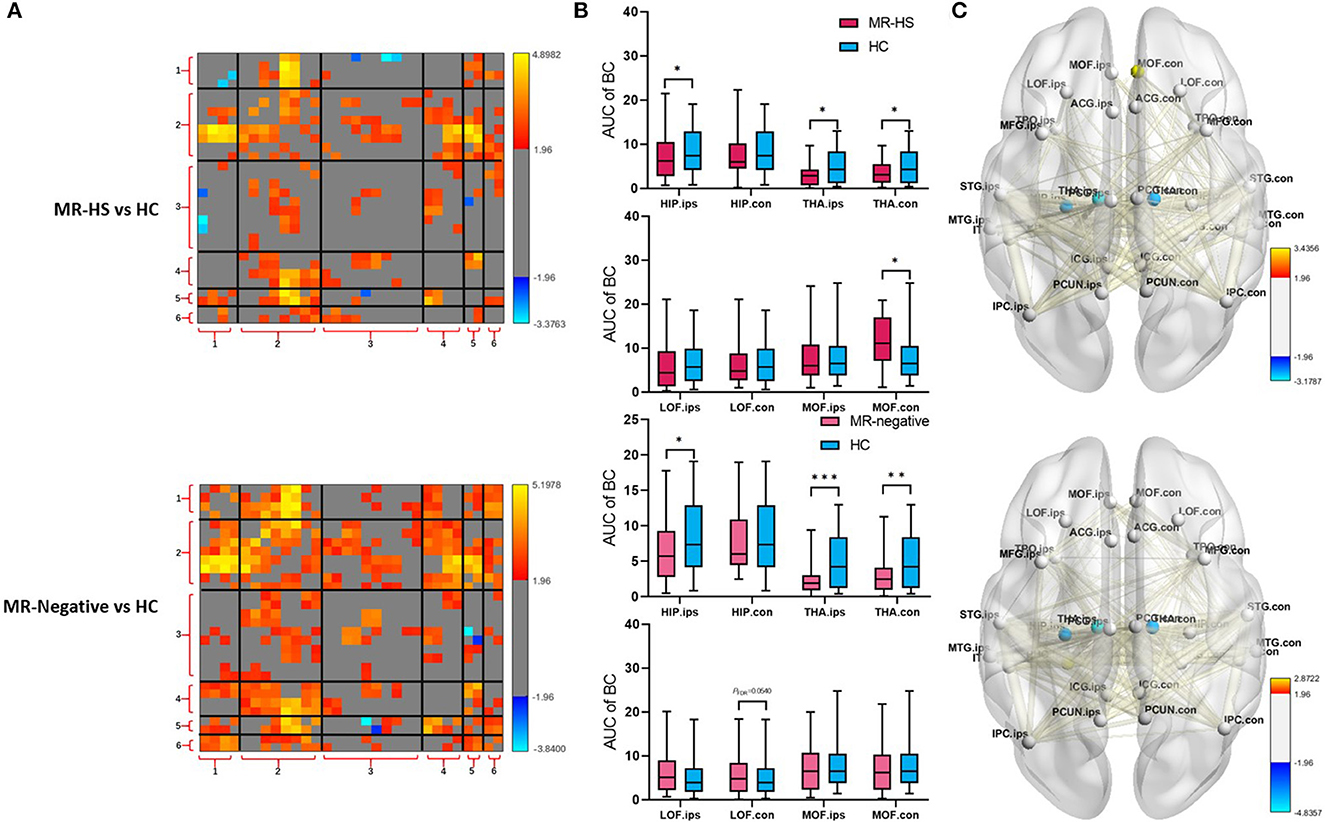
Figure 4. (A) FC, (B) BC, and (C) BC and FC of brain regions with significant differences in patients, compared with healthy controls. Statistical Z values of significant results of (A) FC and (C) BC and FC were mapped into the corresponding regions or edges with thickness. Warm color represents a significant increase in patient groups, while cold color shows a significantly decreased trend. MR-HS, MTLE patients with hippocampal sclerosis; HC, healthy controls; fALFF, fractional amplitude of low-frequency fluctuation; BC, betweenness centrality; FC, functional connectivity; HIP, hippocampus; PHG, parahippocampal gyrus; ITG, inferior temporal gyrus; MTG, middle temporal gyrus; STG, superior temporal gyrus; TPO, temporal pole; LOF, lateral orbitofrontal; MOF, medial orbitofrontal; MFG, middle frontal gyrus; ICG, isthmus cingulate gyrus; ACG, anterior cingulate gyrus; PCG, posterior cingulate gyrus; PCUN, precuneus; IPC, inferior parietal cortex; THA, thalamus; ips, ipsilateral; ips, ipsilateral; con, contralateral; 1, medial temporal lobe; 2, temporal cortex; 3, dorsal and ventral medial prefrontal cortex; 4, precuneus/posterior cingulate cortex; 5, inferior parietal lobe; 6, thalamus; *PFDR < 0.05, **PFDR < 0.01, ***PFDR < 0.001.
3.4. Altered coupling between SUVR and fALFF in MTLE patients
Regarding metabolic-functional coupling, all significant and marginally significant group differences were mapped into the solid lines and dotted lines between two brain regions nodes, respectively (Figure 5A, Supplementary Table 3). Of note, compared with positive SUVR-fALFF correlations in corresponding brain regions of HC group, MR-HS patients showed marginally elevated coupling in the ipsilateral hippocampus (PFDR = 0.0802), lateral orbitofrontal (PFDR = 0.0827) and contralateral posterior cingulate gyrus (PFDR = 0.0630), whereas decreased coupling in bilateral middle frontal gyrus (PFDR = 0.0230, PFDR = 0.0296) were found. Although there was no difference in SUVR-fALFF coupling within thalamus between MR-HS, MR-negative and HC groups, increased coupling in contralateral anterior thalamic nuclei and ipsilateral lateral thalamic nuclei (PFDR = 0.0230, PFDR = 0.0470) and marginally increased coupling in ipsilateral anterior thalamic nuclei were obtained in MR-HS patients (PFDR = 0.0552), compared with HC (Figure 5B, Supplementary Table 3).
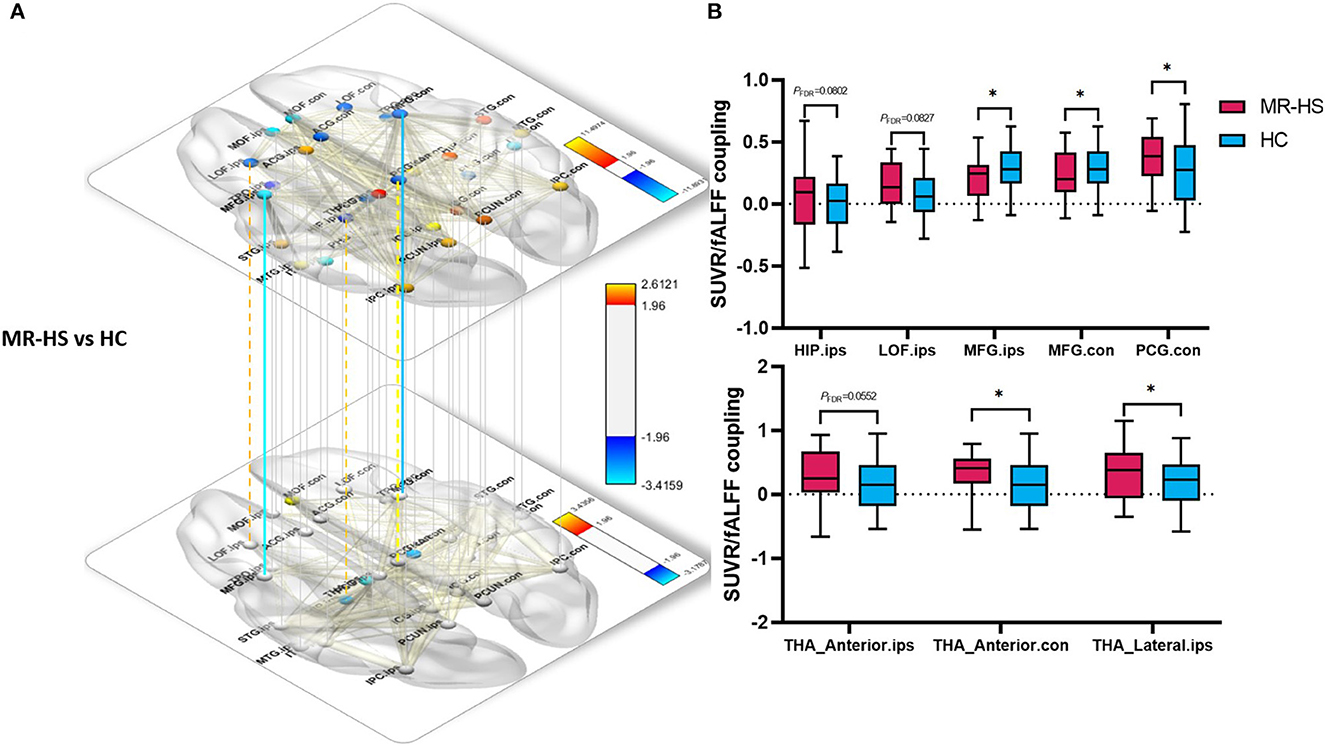
Figure 5. Spatial voxel-wise correlations in regions of DMN thalamic nuclei between SUVR and fALFF with significant group differences between MR-HS patients and HC. (A, B) Solid lines represent significantly different SUVR-fALFF couplings, while dotted lines represent marginally different SUVR-fALFF couplings. Warm color represents a significant increase in patient groups, while cold color shows a significantly decreased trend. SUVR, standardized uptake value ratio; fALFF, fractional amplitude of low-frequency fluctuation; MR-HS, MTLE patients with hippocampal sclerosis; HC, healthy controls; ORBmed, medial orbitofrontal cortex; PCUN, precuneus; HIP, hippocampus; LOF, lateral orbitofrontal; MFG, middle frontal gyrus; PCG, posterior cingulate gyrus; THA_Anterior, anterior thalamic nuclei; THA_Lateral, lateral thalamic nuclei; ips, ipsilateral; con, contralateral; *PFDR < 0.05, **PFDR < 0.01, ***PFDR < 0.001.
3.5. Intermodality coupling could help predict surgical outcomes
Further, we evaluated the potential of the intermodality coupling in surgical outcome prediction in 42 MTLE patients with 2 years follow-up visits after ATL surgery. With Fisher score ranking, SUVR-fALFF couplings in regions from thalamic subnuclei such as bilateral lateral thalamic nuclei and ipsilateral anterior thalamic nuclei, regions from DMN, as well as ipsilateral posterior cingulate gyrus, contralateral hippocampus and ipsilateral middle frontal gyrus were selected for the subsequent classification analysis (Figure 6A).
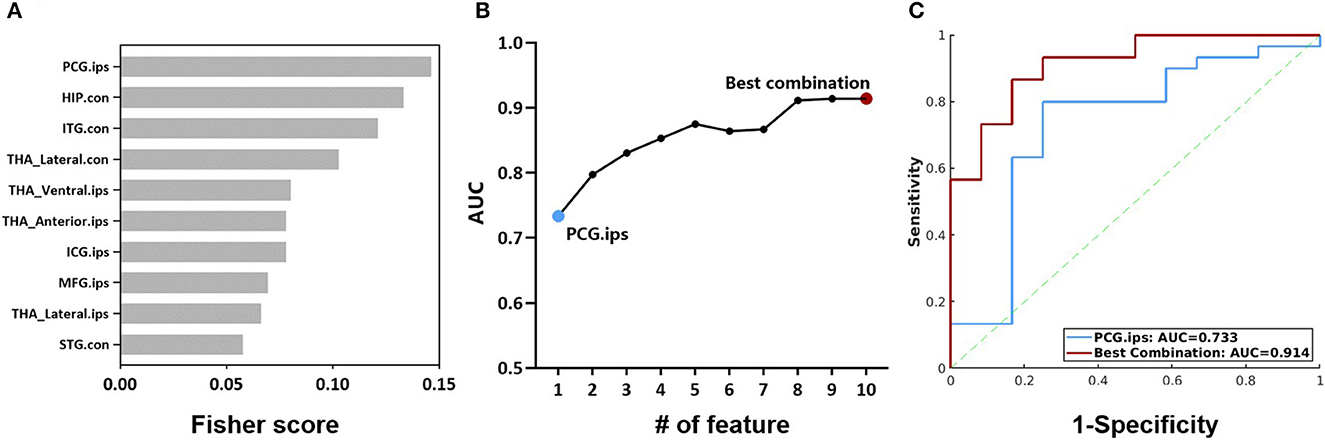
Figure 6. (A) Fisher scores of the top 10 SUVR-fALFF couplings. (B) AUC of couplings with the top 10 Fisher scores, accumulated from the coupling with the highest Fisher score. (C) ROC curves of the classification models with SUVR-fALFF coupling in ipsilateral posterior cingulate gyrus and the best combination. HIP, hippocampus; ITG, inferior temporal gyrus; STG, superior temporal gyrus; MFG, middle frontal gyrus; ICG, isthmus cingulate gyrus; PCG, posterior cingulate gyrus; THA_Anterior, anterior thalamic nuclei; THA_Lateral, lateral thalamic nuclei; THA_Ventral, Ventral thalamic nuclei; ips, ipsilateral; con, contralateral; AUC, the area under ROC curve; SUVR, standardized uptake value ratio; fALFF, fractional amplitude of low-frequency fluctuation.
The performances of the combination of SUVR-fALFF couplings from different brain regions were evaluated by logistic regression models, characterized by the ROC curves shown in Figures 6B, C. The top one feature of ipsilateral posterior cingulate gyrus gave a surgical outcome prediction at AUC of 0.733, while the best performance was the combination of ten SUVR-fALFF couplings with the AUC of 0.914 (Figure 6C).
4. Discussion
In this study, we investigated whether coupling exist between glucose metabolism and functional activity within the epileptogenic network in MTLE patients using simultaneous 18F-FDG PET/rs-fMRI, and examined the predictive value of metabolic-functional couplings in surgical outcome. Specifically, among regions with altered SUVR-fALFF couplings, ipsilateral hippocampus was also decreased in BC of metabolic covariance network and functional network in MR-HS patients. Further, patients with different surgical outcomes were found to have different SUVR-fALFF couplings within the epileptogenic network. Our findings indicated that neuroenergetic coupling measured by PET/MR could improve the comprehending of the pathogenesis in MTLE patients and provide a new neuroimaging metric related to surgical outcome prediction.
Regional hypometabolism could result from repetitive seizures, which is typically characterized by neuronal damage (Patel et al., 2019; Reddy et al., 2019). Chassoux et al. (2016) demonstrated that hypermetabolism was observed in areas partly coincided with DMN, which is in general agreement with the observation of elevated glucose uptake within extratemporal regions of DMN in this study. Hippocampus appears as an altered metabolic hub node in the epileptogenic network as BC decreased in the ipsilateral hippocampus while increased in the contralateral hippocampus. A previous study on metabolic network of MTLE patients found reduced connectivity in ipsilateral hippocampus and increased connectivity in contralateral hippocampus using hippocampus-based metabolic covariant network (Wang et al., 2019). It has been shown that thalamic structural disconnections occur with bilateral mesial temporal lobe and extratemporal regions in MTLE (Barron et al., 2014; Besson et al., 2014), which reveals the structural foundation for metabolic network changes in the thalamus. In addition, decreased connectivities were observed in more brain regions of MR-negative patients compared to MR-HS group, which might suggest a wider epileptogenic network in MR-negative group (Muhlhofer et al., 2017).
In line with previous study, decreased fALFF was also found in medial frontal gyrus in MR-negative patients, which might be explained that the function of DMN was impaired in MTLE subjects and the impairment was attributed to the seizures generation (Zhang et al., 2010). A previous study suggested that impairment of FCs of the affected hippocampus could relate to the anatomic injury of this region with the findings that functional and structural connectivity between mesial temporal lobe structures and other DMN regions are disturbed in patients with MTLE (Liao et al., 2011).
The reason for the elevated coupling between glucose uptake and neural activity remains incompletely understood. Correlations between fALFF and SUVR is generally interpreted as synergy of synaptic currents and action potentials and metabolic demands (Leithner and Royl, 2014; Aiello et al., 2015). In epileptic lesion and epileptogenic network, it is conceivable that modulated by neurovascular coupling both neuronal activity and energy consumption go through great increase, during ictal discharges. During interictal period, altered coupling between metabolism and blood supply has also been reported within the epileptic brain (Song et al., 2016). Ipsilateral hippocampus has been reported to show pathological evidence as a result of excessive oxidative stress even neuronal damage (Engel, 2001), whose marginally increased SUVR-fALFF coupling could be pathology driven. In addition, higher SUVR-fALFF coupling in the ipsilateral posterior cingulate gyrus, one of the functional cores of DMN (Liao et al., 2011), may also be driven by pathological origin, confirming MTLE as a network-based disease. On the other hand, bilateral middle frontal gyrus showed reduced SUVR-fALFF coupling which might be explained by neurovascular decoupling. In this case, the mechanism would prevent blood flow from being effectively stimulated by the abnormal neuronal activity at epileptic discharges (Nugent et al., 2015).
The thalamus is a complex brain structure consisting of multiple nuclei having different connectivity with multiple brain regions (Kumar et al., 2017). In this study, alterations in metabolic-functional coupling were not observed in thalamus as a whole. MR-HS group tends to have elevated coupling in bilateral anterior thalamic nuclei and ipsilateral lateral thalamic nuclei. Anterior nucleus of the thalamus is a crucial component of Papez circuit, an important neural circuit for the generation and propagation of limbic seizures in MTLE (Ferreira et al., 2020), and has become a popular target of deep brain stimulation to treat drug-refractory epilepsy (Jankowski et al., 2013). A memory fMRI and probabilistic diffusion tractography study demonstrated that MR-HS patients with pronounced enhancement of connections between ipsilateral lateral thalamic nuclei and epileptic hippocampus, which might ultimately contribute to the preferred seizures propagation pathway (Dinkelacker et al., 2015).
Using the Fisher score ranking, we pinpointed brain regions within the DMN and thalamus where the coupling between SUVR and fALFF contributes to the prediction of surgical outcomes. Especially, the combination of the top ten gave the best performance for prediction. Notably, these regions do not include often resected regions such as ipsilateral hippocampus, amygdala, but are most likely involved in seizure propagation (Chabardes et al., 2005; Bertram et al., 2008; Li et al., 2016; Abel et al., 2018). The mechanism behind how changed metabolic-functional couplings contribute to the seizure recurrence post-surgery merits further investigation.
This study has some limitations. Firstly, healthy controls are not age-matched with MTLE patients, mainly due to the difficulty of recruiting young healthy volunteers in a full protocol with radioactive tracers. Previous studies have demonstrated that older adults had lower glucose uptake in several DMN regions, like frontal lobe, temporal lobe and cingulate, and they showed higher glucose uptake in thalamus (Nugent et al., 2015; Bonte et al., 2017). In addition, average functional connectivity within resting state networks has been reported to decrease with age (Betzel et al., 2014). In this study, we used multiple regression models for age correction to mitigate the effect of age differences between patients and healthy controls on the results. Secondly, in this preliminary study the sample size for surgical outcome prediction is relatively small, though we used leave-one-out cross-validation to test the classification models, further independent datasets are needed to cross validate the findings. Finally, the complex interactions between functional activity and metabolic consumption is incompletely represented by Tomasi et al. (2013). Potentially non-linear models could be constructed to capture the associations in future studies.
5. Conclusions
In summary, our study identified altered coupling between glucose metabolism and functional activity within epileptogenic network in patients with MTLE by simultaneous 18F-FDG PET/rs-fMRI and its predictive value in surgical outcomes. These findings may provide potentially useful markers for MTLE patients in their preoperative evaluation.
Data availability statement
The raw data supporting the conclusions of this article will be made available by the authors, without undue reservation.
Ethics statement
The studies involving human participants were reviewed and approved by Internal Review Board of Ruijin Hospital. Written informed consent to participate in this study was provided by the participants' legal guardian/next of kin.
Author contributions
SY: conceive design, experimental design, data analysis, figures organization, and manuscript writing. HH: experimental design and manuscript writing. BC: methodology and manuscript review and editing. JLi: investigation, manuscript review, and editing. MZ: data collection and curation. JLu: conceive design, manuscript review and editing, and manuscript writing. All authors contributed to the article and approved the submitted version.
Funding
This study was partially supported by the National Natural Science Foundation of China (No. 62101321) and Shanghai Municipal Health Commission (No. 202240031).
Conflict of interest
The authors declare that the research was conducted in the absence of any commercial or financial relationships that could be construed as a potential conflict of interest.
Publisher's note
All claims expressed in this article are solely those of the authors and do not necessarily represent those of their affiliated organizations, or those of the publisher, the editors and the reviewers. Any product that may be evaluated in this article, or claim that may be made by its manufacturer, is not guaranteed or endorsed by the publisher.
Supplementary material
The Supplementary Material for this article can be found online at: https://www.frontiersin.org/articles/10.3389/fnins.2023.1165982/full#supplementary-material
References
Abel, T. J., Woodroffe, R. W., Nourski, K. V., Moritani, T., Capizzano, A. A., Kirby, P., et al. (2018). Role of the temporal pole in temporal lobe epilepsy seizure networks: an intracranial electrode investigation. J. Neurosurg. 129, 165–173. doi: 10.3171/2017.3.JNS162821
Aiello, M., Salvatore, E., Cachia, A., Pappata, S., Cavaliere, C., Prinster, A., et al. (2015). (2015). Relationship between simultaneously acquired resting-state regional cerebral glucose metabolism and functional MRI: a PET/MR hybrid scanner study. Neuroimage 113, 111–121. doi: 10.1016/j.neuroimage.0317
Andrews-Hanna, J. R., Reidler, J. S., Sepulcre, J., Poulin, R., and Buckner, R. L. (2010). Functional-anatomic fractionation of the brain's default network. Neuron 65, 550–562. doi: 10.1016/j.neuron.02005
Barron, D. S., Tandon, N., Lancaster, J. L., and Fox, P. T. (2014). Thalamic structural connectivity in medial temporal lobe epilepsy. Epilepsia 55, e50–55. doi: 10.1111/epi.12637
Bertram, E. H., Zhang, D., and Williamson, J. M. (2008). Multiple roles of midline dorsal thalamic nuclei in induction and spread of limbic seizures. Epilepsia 49, 256–268. doi: 10.1111/j.1528-2007.01408.x
Besson, P., Dinkelacker, V., Valabregue, R., Thivard, L., Leclerc, X., Baulac, M., et al. (2014). (2014). Structural connectivity differences in left and right temporal lobe epilepsy. Neuroimage 100, 135–144. doi: 10.1016/j.neuroimage.04071
Betzel, R. F., Byrge, L., He, Y., Goni, J., Zuo, X. N., Sporns, O., et al. (2014). (2014). Changes in structural and functional connectivity among resting-state networks across the human lifespan. Neuroimage 102(Pt 2), 345–357. doi: 10.1016/j.neuroimage.07067
Blumcke, I., Thom, M., Aronica, E., Armstrong, D. D., Bartolomei, F., Bernasconi, A., et al. (2013). International consensus classification of hippocampal sclerosis in temporal lobe epilepsy: a task force report from the ILAE commission on diagnostic methods. Epilepsia 54, 1315–1329. doi: 10.1111/epi.12220
Bonte, S., Vandemaele, P., Verleden, S., Audenaert, K., Deblaere, K., Goethals, I., et al. (2017). Healthy brain ageing assessed with 18F-FDG PET and age-dependent recovery factors after partial volume effect correction. Eur. J. Nucl. Med. Mol. Imaging 44, 838–849. doi: 10.1007/s00259-016-3569-0
Boscolo Galazzo, I., Mattoli, M. V., Pizzini, F. B., Vita, D. e., Barnes, E., Duncan, A.J.S., et al. (2016). Cerebral metabolism and perfusion in MR-negative individuals with refractory focal epilepsy assessed by simultaneous acquisition of (18)F-FDG PET and arterial spin labeling. Neuroimage Clin. 11, 648–657. doi: 10.1016/j.nicl.04005
Buckner, R. L., Andrews-Hanna, J. R., and Schacter, D. L. (2008). The brain's default network: anatomy, function, and relevance to disease. Ann. N. Y. Acad. Sci. 1124, 1–38. doi: 10.1196/annals.1440.011
Buckner, R. L., and DiNicola, L. M. (2019). The brain's default network: updated anatomy, physiology and evolving insights. Nat. Rev. Neurosci. 20, 593–608. doi: 10.1038/s41583-019-0212-7
Bullmore, E., and Sporns, O. (2009). Complex brain networks: graph theoretical analysis of structural and functional systems. Nat. Rev. Neurosci. 10, 186–198. doi: 10.1038/nrn2575
Chabardes, S., Kahane, P., Minotti, L., Tassi, L., Grand, S., Hoffmann, D., et al. (2005). The temporopolar cortex plays a pivotal role in temporal lobe seizures. Brain 128(Pt 8), 1818–1831. doi: 10.1093./brain/awh512
Chao-Gan, Y., and Yu-Feng, Z. (2010). DPARSF: a MATLAB toolbox for “Pipeline” data analysis of resting-state fMRI. Front. Syst. Neurosci. 4, 13. doi: 10.3389/fnsys.2010.00013
Chassoux, F., Artiges, E., Semah, F., Desarnaud, S., Laurent, A., Landre, E., et al. (2016). Determinants of brain metabolism changes in mesial temporal lobe epilepsy. Epilepsia 57, 907–919. doi: 10.1111/epi.13377
Desikan, R. S., Segonne, F., Fischl, B., Quinn, B. T., Dickerson, B. C., Blacker, D., et al. (2006). (2006). An automated labeling system for subdividing the human cerebral cortex on MRI scans into gyral based regions of interest. Neuroimage 31, 968–980. doi: 10.1016/j.neuroimage.01021
Dinkelacker, V., Valabregue, R., Thivard, L., Lehericy, S., Baulac, M., Samson, S., et al. (2015). Hippocampal-thalamic wiring in medial temporal lobe epilepsy: enhanced connectivity per hippocampal voxel. Epilepsia 56, 1217–1226. doi: 10.1111/epi.13051
Engel, J. Jr. (1993). Update on surgical treatment of the epilepsies. Summary of the Second international palm desert conference on the surgical treatment of the epilepsies (1992). Neurology 43, 1612–1617. doi: 10.1212/wnl.43.8.1612
Engel, J. Jr. (2001). Mesial temporal lobe epilepsy: what have we learned? Neuroscientist 7, 340–352. doi: 10.1177/107385840100700410
Engel, J. Jr., McDermott, M.P., Wiebe, S., Langfitt, J.T., Stern, J.M., Dewar, S., et al. (2012). Early surgical therapy for drug-resistant temporal lobe epilepsy: a randomized trial. JAMA 307, 922–930. doi: 10.1001/jama.2012.220
Englot, D. J., D'Haese, P. F., Konrad, P. E., Jacobs, M. L., Gore, J. C., Abou-Khalil, B. W., et al. (2017). Functional connectivity disturbances of the ascending reticular activating system in temporal lobe epilepsy. J. Neurol. Neurosurg. Psychiatr. 88, 925–932. doi: 10.1136/jnnp-2017-315732
Ferreira, T. A. Jr., Middlebrooks, E.H., Tzu, W. H., Neto, M. R., and Holanda, V. M. (2020). Postmortem dissections of the papez circuit and non-motor targets for functional neurosurgery. World Neurosurg. 144, e866–e875. doi: 10.1016/j.wneu.09088
Gu, Q., Li, Z., and Han, J. (2012). Generalized fisher score for feature selection. arXiv [Preprint]. arXiv: 1202.3725. doi: 10.48550/arXiv.1202.3725
Guye, M., Regis, J., Tamura, M., Wendling, F., McGonigal, A., Chauvel, P., et al. (2006). The role of corticothalamic coupling in human temporal lobe epilepsy. Brain 129(Pt 7), 1917–1928. doi: 10.1093./brain/awl151
He, X., Doucet, G. E., Pustina, D., Sperling, M. R., Sharan, A. D., Tracy, J. I., et al. (2017). Presurgical thalamic “hubness” predicts surgical outcome in temporal lobe epilepsy. Neurology 88, 2285–2293. doi: 10.1212/WNL.0000000000004035
Hwang, K., Bertolero, M. A., Liu, W. B., and D'Esposito, M. (2017). The human thalamus is an integrative hub for functional brain networks. J. Neurosci. 37, 5594–5607. doi: 10.1523/JNEUROSCI.0067-17.2017
Iglesias, J. E., Insausti, R., Lerma-Usabiaga, G., Bocchetta, M., Van Leemput, K., Greve, D. N., et al. (2018). A probabilistic atlas of the human thalamic nuclei combining ex vivo MRI and histology. Neuroimage 183, 314–326. doi: 10.1016/j.neuroimage.2018.08.012
Jankowski, M. M., Ronnqvist, K. C., Tsanov, M., Vann, S. D., Wright, N. F., Erichsen, J. T., et al. (2013). The anterior thalamus provides a subcortical circuit supporting memory and spatial navigation. Front. Syst. Neurosci. 7, 45. doi: 10.3389/fnsys.2013.00045
Kabbara, A., El Falou, W., Khalil, M., Wendling, F., and Hassan, M. (2017). The dynamic functional core network of the human brain at rest. Sci. Rep. 7, 2936. doi: 10.1038/s41598-017-03420-6
Kim, J., Criaud, M., Cho, S. S., Diez-Cirarda, M., Mihaescu, A., Coakeley, S., et al. (2017). Abnormal intrinsic brain functional network dynamics in Parkinson's disease. Brain 140, 2955–2967. doi: 10.1093/brain/awx233
Kumar, V. J., van Oort, E., Scheffler, K., Beckmann, C. F., and Grodd, W. (2017). Functional anatomy of the human thalamus at rest. Neuroimage 147, 678–691. doi: 10.1016/j.neuroimage.12.071.
Lamarche, F., Job, A. S., Deman, P., Bhattacharjee, M., Hoffmann, D., Gallazzini-Crepin, C., et al. (2016). Correlation of FDG-PET hypometabolism and SEEG epileptogenicity mapping in patients with drug-resistant focal epilepsy. Epilepsia 57, 2045–2055. doi: 10.1111/epi.13592
Leithner, C., and Royl, G. (2014). The oxygen paradox of neurovascular coupling. J. Cereb. Blood Flow Metab. 34, 19–29. doi: 10.1038/jcbfm.2013.181
Li, J., Chen, X., Ye, W., Jiang, W., Liu, H., Zheng, J., et al. (2016). (2016). Alteration of the alertness-related network in patients with right temporal lobe epilepsy: a resting state fMRI study. Epilepsy Res. 127, 252–259. doi: 10.1016/j.eplepsyres.09013
Liao, W., Zhang, Z., Pan, Z., Mantini, D., Ding, J., Duan, X., et al. (2011). Default mode network abnormalities in mesial temporal lobe epilepsy: a study combining fMRI and DTI. Hum. Brain Mapp. 32, 883–895. doi: 10.1002/hbm.21076
Liu, J. T., Liu, B., and Zhang, H. (2018). Surgical versus medical treatment of drug-resistant epilepsy: a systematic review and meta-analysis. Epilepsy Behav. 82, 179–188. doi: 10.1016/j.yebeh.11012
Muhlhofer, W., Tan, Y. L., Mueller, S. G., and Knowlton, R. (2017). MRI-negative temporal lobe epilepsy-What do we know? Epilepsia 58, 727–742. doi: 10.1111/epi.13699
Nugent, A. C., Martinez, A., D'Alfonso, A., Zarate, C. A., and Theodore, W. H. (2015). The relationship between glucose metabolism, resting-state fMRI BOLD signal, and GABAA-binding potential: a preliminary study in healthy subjects and those with temporal lobe epilepsy. J. Cereb. Blood Flow Metab. 35, 583–591. doi: 10.1038/jcbfm.2014.228
Patel, D. C., Tewari, B. P., Chaunsali, L., and Sontheimer, H. (2019). Neuron-glia interactions in the pathophysiology of epilepsy. Nat. Rev. Neurosci. 20, 282–297. doi: 10.1038/s41583-019-0126-4
Pittau, F., Grova, C., Moeller, F., Dubeau, F., and Gotman, J. (2012). Patterns of altered functional connectivity in mesial temporal lobe epilepsy. Epilepsia 53, 1013–1023. doi: 10.1111/j.1528-1167.2012.03464.x
Reddy, S. D., Younus, I., Sridhar, V., and Reddy, D. S. (2019). Neuroimaging biomarkers of experimental epileptogenesis and refractory epilepsy. Int. J. Mol. Sci. 20, 220. doi: 10.3390./ijms20010220
Roels, S. P., Moerkerke, B., and Loeys, T. (2015). Bootstrapping fMRI data: dealing with misspecification. Neuroinformatics 13, 337–352. doi: 10.1007/s12021-015-9261-x
Song, Y., Torres, R. A., Garcia, S., Frometa, Y., Bae, J., Deshmukh, A., et al. (2016). Dysfunction of neurovascular/metabolic coupling in chronic focal epilepsy. IEEE Trans. Biomed. Eng. 63, 97–110. doi: 10.1109/TBME.2015.2461496
Tomasi, D., Wang, G. J., and Volkow, N. D. (2013). Energetic cost of brain functional connectivity. Proc. Natl. Acad. Sci. USA. 110, 13642–13647. doi: 10.1073/pnas.1303346110
Tsuda, K., Tsuji, T., Ishida, T., Takahashi, S., Yamada, S., Ohoshi, Y., et al. (2018). Widespread abnormalities in white matter integrity and their relationship with duration of illness in temporal lobe epilepsy. Epilepsia Open 3, 247–254. doi: 10.1002/epi4.12222
Tzourio-Mazoyer, N., Schonen, De., Crivello, S., Reutter, F., Aujard, B. Y., and Mazoyer, B. (2002). Neural correlates of woman face processing by 2-month-old infants. Neuroimage 15, 454–461. doi: 10.1006/nimg.2001.0979
Vaughan, D. N., Rayner, G., Tailby, C., and Jackson, G. D. (2016). MRI-negative temporal lobe epilepsy: a network disorder of neocortical connectivity. Neurology 87, 1934–1942. doi: 10.1212/WNL.0000000000003289
Wang, J., Shan, Y., Dai, J., Cui, B., Shang, K., Yang, H., et al. (2020). Altered coupling between resting-state glucose metabolism and functional activity in epilepsy. Ann. Clin. Transl. Neurol. 7, 1831–1842. doi: 10.1002/acn3.51168
Wang, J., Wang, X., He, Y., Yu, X., Wang, H., He, Y., et al. (2015). Apolipoprotein E epsilon4 modulates functional brain connectome in Alzheimer's disease. Hum. Brain Mapp. 36, 1828–1846. doi: 10.1002/hbm.22740
Wang, K. L., Hu, W., Liu, T. H., Zhao, X. B., Han, C. L., Xia, X. T., et al. (2019). Metabolic covariance networks combining graph theory measuring aberrant topological patterns in mesial temporal lobe epilepsy. CNS Neurosci. Ther. 25, 396–408. doi: 10.1111/cns.13073
Xu, J., Chen, F., Liu, T., Wang, T., Zhang, J., Yuan, H., et al. (2019). Brain functional networks in type 2 diabetes mellitus patients: a resting-state functional MRI study. Front. Neurosci. 13, 239. doi: 10.3389/fnins.2019.00239
Zanao, T. A., Lopes, T. M., Campos, d. e., Yasuda, B. M. C.L., and Cendes, F. (2021). Patterns of default mode network in temporal lobe epilepsy with and without hippocampal sclerosis. Epilepsy Behav. 121(Pt B), 106523. doi: 10.1016./j.yebeh.2019.106523
Zang, Y. F., He, Y., Zhu, C. Z., Cao, Q. J., Sui, M. Q., Liang, M., et al. (2007). Altered baseline brain activity in children with ADHD revealed by resting-state functional MRI. Brain Dev. 29, 83–91. doi: 10.1016/j.braindev.07002
Zhang, D. X., and Bertram, E. H. (2002). Midline thalamic region: widespread excitatory input to the entorhinal cortex and amygdala. J. Neurosci. 22, 3277–3284. doi: 10.1523/JNEUROSCI.22-08-03277.2002
Zhang, F., Chen, Q., Ye, T., Lipska, B. K., Straub, R. E., Vakkalanka, R., et al. (2011). (2011). Evidence of sex-modulated association of ZNF804A with schizophrenia. Biol. Psychiatry 69, 914–917. doi: 10.1016/j.biopsych.01003
Zhang, Z., Lu, G., Zhong, Y., Tan, Q., Chen, H., Liao, W., et al. (2010). fMRI study of mesial temporal lobe epilepsy using amplitude of low-frequency fluctuation analysis. Hum. Brain Mapp. 31, 1851–1861. doi: 10.1002/hbm.20982
Zhou, X., Zhang, Z., Liu, J., Qin, L., and Zheng, J. (2019). Aberrant topological organization of the default mode network in temporal lobe epilepsy revealed by graph-theoretical analysis. Neurosci. Lett. 708, 134351. doi: 10.1016/j.neulet.2019.134351
Zou, Q. H., Zhu, C. Z., Yang, Y., Zuo, X. N., Long, X. Y., Cao, Q. J., et al. (2008). (2008). An improved approach to detection of amplitude of low-frequency fluctuation (ALFF) for resting-state fMRI: fractional ALFF. J. Neurosci. Methods 172, 137–141. doi: 10.1016/j.jneumeth.04012
Keywords: MTLE, PET/MR, standardized uptake value ratio (SUVR), fractional amplitude of low-frequency fluctuation (fALFF), default mode network (DMN), epileptogenic network
Citation: Yuan S, Huang H, Cai B, Li J, Zhang M and Luo J (2023) Altered metabolic-functional coupling in the epileptogenic network could predict surgical outcomes of mesial temporal lobe epilepsy. Front. Neurosci. 17:1165982. doi: 10.3389/fnins.2023.1165982
Received: 14 February 2023; Accepted: 09 May 2023;
Published: 08 June 2023.
Edited by:
Xin Di, New Jersey Institute of Technology, United StatesReviewed by:
Tommaso Volpi, Yale University, United StatesDaniele Bertoglio, University of Antwerp, Belgium
Copyright © 2023 Yuan, Huang, Cai, Li, Zhang and Luo. This is an open-access article distributed under the terms of the Creative Commons Attribution License (CC BY). The use, distribution or reproduction in other forums is permitted, provided the original author(s) and the copyright owner(s) are credited and that the original publication in this journal is cited, in accordance with accepted academic practice. No use, distribution or reproduction is permitted which does not comply with these terms.
*Correspondence: Jie Luo, jieluo@sjtu.edu.cn
†These authors share first authorship