- 1School of Medicine, Yale University, New Haven, CT, United States
- 2Department of Neuroscience, Tufts University School of Medicine, Boston, MA, United States
Psychosis spectrum disorder (PSD) affects 1% of the world population and results in a lifetime of chronic disability, causing devastating personal and economic consequences. Developing new treatments for PSD remains a challenge, particularly those that target its core cognitive deficits. A key barrier to progress is the tenuous link between the basic neurobiological understanding of PSD and its clinical phenomenology. In this perspective, we focus on a key opportunity that combines innovations in non-invasive human neuroimaging with basic insights into thalamic regulation of functional cortical connectivity. The thalamus is an evolutionary conserved region that forms forebrain-wide functional loops critical for the transmission of external inputs as well as the construction and update of internal models. We discuss our perspective across four lines of evidence: First, we articulate how PSD symptomatology may arise from a faulty network organization at the macroscopic circuit level with the thalamus playing a central coordinating role. Second, we discuss how recent animal work has mechanistically clarified the properties of thalamic circuits relevant to regulating cortical dynamics and cognitive function more generally. Third, we present human neuroimaging evidence in support of thalamic alterations in PSD, and propose that a similar “thalamocortical dysconnectivity” seen in pharmacological imaging (under ketamine, LSD and THC) in healthy individuals may link this circuit phenotype to the common set of symptoms in idiopathic and drug-induced psychosis. Lastly, we synthesize animal and human work, and lay out a translational path for biomarker and therapeutic development.
Introduction
Psychosis spectrum disorder (PSD) is a pervasive and debilitating syndrome that affects tens of millions of people worldwide, with a lifetime risk of about 1% regardless of geography (Rehm and Shield, 2019). Patients with PSD experience symptoms that greatly impact their daily functioning (Picchioni and Murray, 2007; Rehm and Shield, 2019), including hallucinations, delusions, disorganized speech, loss of motivation, flat affect, as well as pervasive deficits in cognitive control (Barch and Ceaser, 2012). Cognitive control deficits are a major focus for research and treatment development, as they are relatively unaffected by current medications and contribute to overall morbidity and loss of workforce by the illness (Auquier et al., 2006; Kooyman et al., 2007). Critically, PSD patients vary greatly in their symptom expression and consequently, appropriate stratification and connection to neurobiological mechanisms has been challenging (Jablensky, 2010; Foss-Feig et al., 2017; Ji et al., 2021). Nonetheless, advances in both human and non-human animal work over the last decade may provide mechanistic targets for PSD symptoms. For example, it is now widely recognized that PSD is associated with functional dysconnectivity across distributed neural systems measured using non-invasive human neuroimaging (Woodward et al., 2012; Anticevic et al., 2014a,b, 2015; Woodward and Heckers, 2016; Giraldo-Chica and Woodward, 2017; Murray and Anticevic, 2017; Baran et al., 2019; Sheffield et al., 2020; Avram et al., 2021; Fryer et al., 2021; Huang et al., 2021; Ji et al., 2021, 2019a; Kraguljac and Lahti, 2021; Kraguljac et al., 2021; Abram et al., 2022; Li et al., 2022; Sabaroedin et al., 2022). Identifying the circuit mechanisms underlying the functional systems-level alterations observed with neuroimaging is critical to rationally guide therapeutics for specific symptoms at the level of individual patients, especially those that do not respond adequately to antipsychotics. To make this goal possible, it is critical to focus on translationally tractable neural targets linked to specific symptoms, which can be effectively studied in animals and humans.
Like all mammalian counterparts, the human forebrain is organized into loops connecting the cortex, thalamus and basal ganglia (Shepherd and Yamawaki, 2021). Broadly, the cortex is largely composed of recurrent excitatory circuits that are capable of maintaining activity patterns across multiple time scales relevant to driving adaptive behavior (Macpherson et al., 2021). Local cortical interneurons gate and sculpt these activity patterns (Averbeck and O’Doherty, 2022). The basal ganglia are inhibitory structures that are largely driven by cortical inputs, and gated by reward-related signals (e.g., dopamine), allowing them to function at least in part as critical nodes in reinforcement learning (Averbeck and O’Doherty, 2022). The thalamus is a centrally located subcortical region that is divided up into multiple nuclei, some of which receive basal ganglia input, and almost all of which have extensive, segregated and well-organized connections to the cortex (Halassa, 2011; Nakajima and Halassa, 2017; Rikhye et al., 2018b). Because of the intimate relationship between the cortex and thalamus and the functional loops they form, they are often collectively referred to as the “thalamocortical system.” Importantly, the observed neuroimaging functional dysconnectivity in schizophrenia (and PSD more broadly) is likely a feature of a perturbed thalamocortical system (Anticevic et al., 2014a; Murray and Anticevic, 2017). This point is critical, because an increasing number of studies indicate that the thalamus may be a particularly attractive region to target in a number of brain disorders (Lee et al., 2019). The key for each disorder may be in the type of connectivity patterns the targeted thalamic subregion establishes with the cortex. The central thalamus, for example, has widespread connections to all cortical areas and can be targeted with deep brain stimulation to reverse anesthesia in animals (Bastos et al., 2021) and restore arousal in human disorders of consciousness (Schiff et al., 2007). The anterior thalamus, which has connections to medial temporal lobe structures, can be targeted to effectively control seizures in medication-resistant epilepsy (Ryvlin et al., 2021). Therefore, the thalamus can be perhaps viewed as a spatially restricted access point to large-scale cortical networks, but with each thalamic region providing a unique opportunity for functional correction (Figure 1).
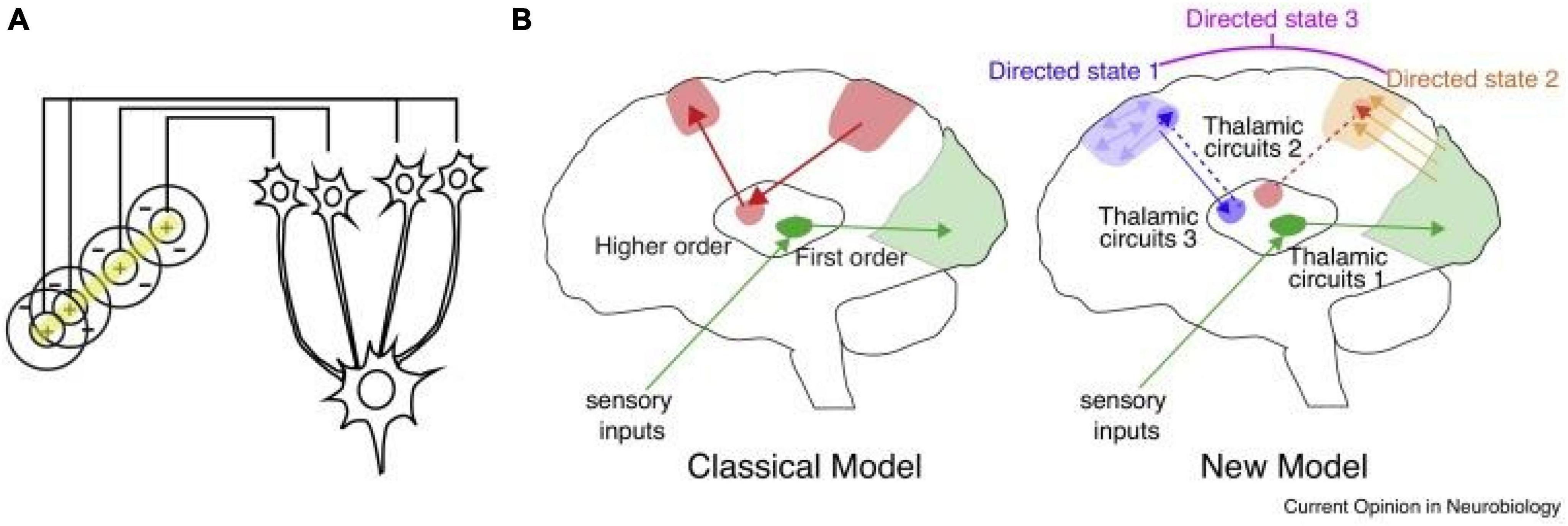
Figure 1. Thalamic control of functional cortical connectivity. (A) The classical transformation of visual representation from center/surround to orientation tuning involves drive from the lateral geniculate nucleus (LGN) to primary visual cortex, where the resulting cortical representation is dependent on thalamic input (information transmission). (B) The classical model reflects the idea of information relay from the LGN, which is shared by other sensory systems (green), and extended to account for thalamic nuclei that receive driving inputs from the cortex rather than subcortical or sensory inputs. The new model suggests that many of these circuits operate to enhance connectivity within a cortical region (e.g., blue), or across different cortical areas (e.g., orange). In these scenarios thalamic inputs do not necessarily drive spiking (do not dictate cortical spike times), but rather enhance how spike times are generated in response to specific inputs (either local, or from area A, B, C, etc.). A particular connectivity pattern reflects a directed arousal state, as defined in this review. Such states can encompass multiple cortical nodes and utilize multiple thalamic amplifiers (e.g., directed state 3), depending on the complexity of the associated cognitive process.
In addition to its unique anatomical features, converging lines of evidence suggest that the thalamus is prominently altered in patients with PSD and schizophrenia in particular, thus supporting the idea that it may not only be a target of intervention, but also a viable biomarker of specific symptoms associated with PSD (Behrendt, 2006; Cronenwett and Csernansky, 2010; Steullet, 2020). Interestingly, numerous studies have repeatedly shown altered thalamic structure and function, even before the onset of symptoms, suggesting a role in etiology (Andreasen, 1997; Ferrarelli and Tononi, 2011; Woodward et al., 2012; Anticevic et al., 2014a,2015; Pergola et al., 2015; Ji et al., 2019a; Ramsay, 2019; Steullet, 2020). Of note, some studies have shown that higher-order, associative thalamic nuclei may be particularly affected in schizophrenia relative to primary sensory nuclei (Andreasen, 1997; Anticevic et al., 2014a; Dorph-Petersen and Lewis, 2017; Sherman, 2017; Steullet, 2020) (Figure 2). This may reflect a fundamental difference in the vulnerability of associative thalamocortical systems to disease mechanisms that ultimately produce PSD symptoms. Specifically, in contrast to primary sensorimotor systems, prefrontal and associative cortical areas handle a variety of input types that require dynamic gating based on changing cognitive task demands and behavioral goals (Hazy et al., 2007). This may place associative thalamocortical systems in a particularly vulnerable state by disease processes that affect basic synaptic transmission and plasticity, both with genetic substrates known to be altered in schizophrenia (Anticevic and Lisman, 2017). Consistent with this notion is the clinical literature showing that localized vascular lesions in associative thalamic structures can actually lead to psychosis, particularly when it impacts projections to prefrontal cortical regions (Crail-Melendez et al., 2013).
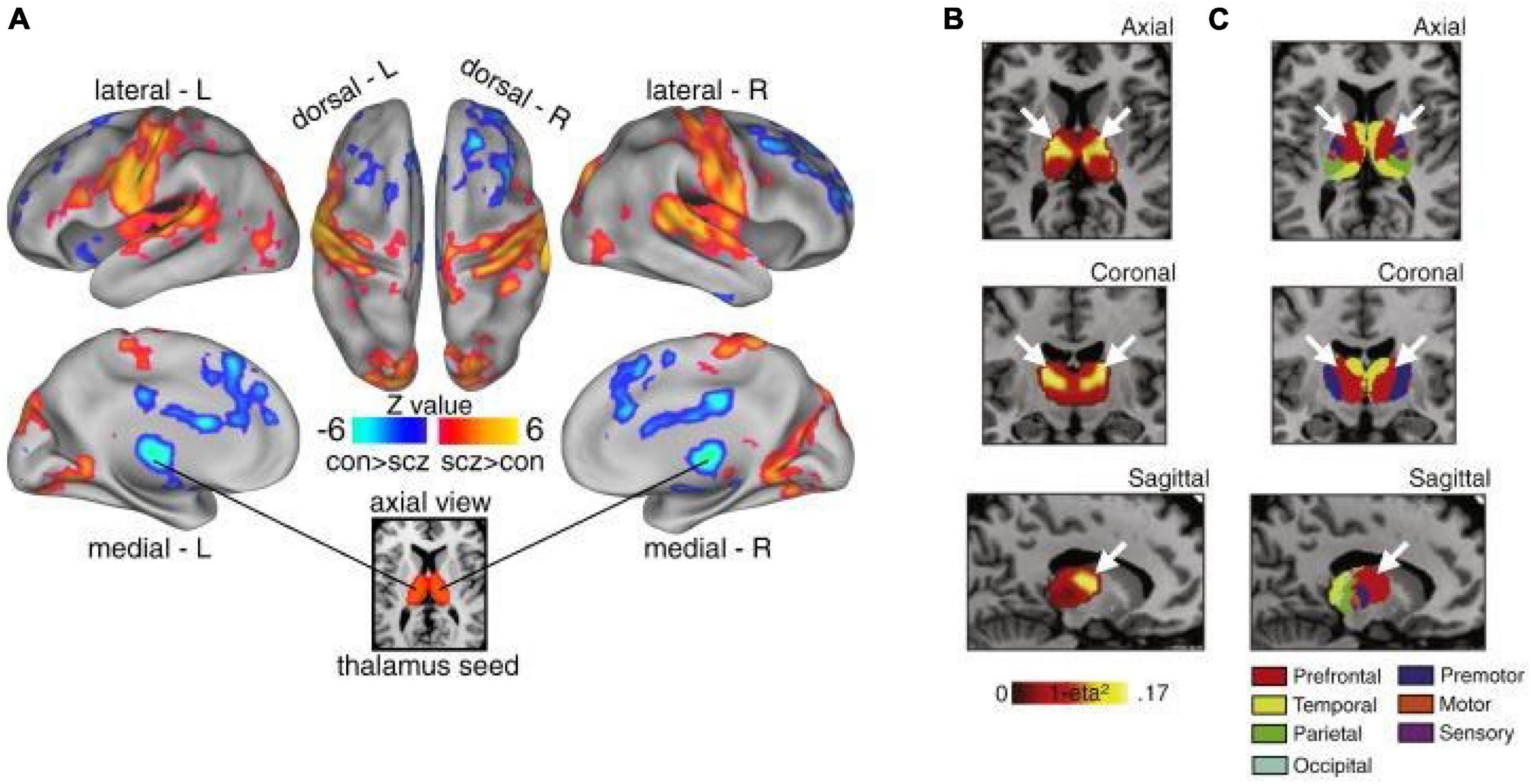
Figure 2. Thalamic dysconnectivity in SCZ. (A) Significant whole-brain between-group differences in thalamic connectivity between healthy controls (CON) and individuals with schizophrenia (SCZ). Red-orange (blue) foci mark areas where patients exhibited stronger (reduced) thalamic coupling. (B) Intrinsic thalamic dysconnectivity pattern based on group dissimilarity. The brightest voxels are associated with highest between-group differences. (C) Thalamus subdivisions based on the FSL thalamic atlas, defined by their connectivity to different regions of cortex. White arrows indicate the correspondence between thalamic regions with greatest dysconnectivity in SCZ and thalamic subdivisions strongly connected with prefrontal cortex (red). Adapted from Anticevic et al. (2014a).
Here we discuss a framework that considers the critical role of the thalamus in the neurobiology of PSD. We structure this piece into four sections. First, we outline our central hypothesis related to thalamocortical dysconnectivity and PSD symptomatology, with an overarching framing that PSD fundamentally affects computations supporting cognitive control and reasoning (Garety et al., 2005; Cardella and Gangemi, 2015; Burgher et al., 2021). Second, we discuss recent animal studies that have redefined the role of the thalamus in cognitive function, laying the groundwork for how its dysfunction can contribute to cognitive control and reasoning deficits in PSD. Third, we present robust human neuroimaging evidence that strongly implicates thalamic functional alterations in PSD, which opens a promising domain for treatment-relevant biomarker development in relation to specific PSD symptoms. Here we place an emphasis on studies that use so-called resting-state functional connectivity (rs-FC), which offers a powerful non-invasive tool for quantifying system-level thalamocortical coordinated activity at the single patient level. We link these findings to an emerging pharmacological imaging literature in healthy individuals, which shows how transient administration of specific drugs (e.g., ketamine, LSD, or THC) can mimic not only certain behavioral symptoms of PSD, but also key aspects of the rs-FC “thalamocortical dysconnectivity” observed in PSD patients. Fourth, we synthesize the animal and human work into a translational framework focused around thalamocortical systems in PSD, and speculate on a rational path for biomarker and therapeutic development.
Psychosis spectrum disorder as a dysconnectivity syndrome
A major idea in this piece is that PSD is a syndrome associated with perturbation in processing across distributed large-scale neural systems, which preferentially and severely impacts forebrain associative systems. This functional dysconnectivity underlies the debilitating, complex and highly heterogeneous symptoms seen in PSD patients. These symptoms include formal thought disorder, delusional beliefs, hallucinations, affective disturbances, and executive deficits (e.g., short-term memory, task switching, planning, logical reasoning to name a few). We suggest that all of these symptoms are to some extent cognitive in nature, and are linked to perturbations in coordinated activity patterns across higher-order associative circuits. Within this framework, associative thalamic circuits play an essential regulatory role, offering a window into PSD etiology and perhaps even rational correction.
It is important to recognize that the clinical reality of PSD is heterogeneity; the symptoms outlined above are variable in onset, severity, prevalence, and treatment response across individual patients. Therefore, it is likely impossible to define this syndrome by a single neurobiological “cause.” Instead, we suggest that the core deficits across the spectrum of psychotic illness are related to appropriately organizing information flow across levels of reasoning hierarchy (Hilgetag and Goulas, 2020). In other words, appropriately processing, maintaining, and inferring the causes of events in highly complex dynamic environments, particularly when many of them are unobservable, is perturbed in schizophrenia. There is now ample evidence to suggest that such operations are serial in nature, and require the iterative interactions between multiple frontal systems, each of which specialized for a particular class of operations within any given cognitive algorithm (e.g., orbitofrontal cortex in constructing value estimates) (Padoa-Schioppa and Conen, 2017), dorsolateral prefrontal cortex in coordinating and updating of task sets (Soltani and Koechlin, 2022), anterior cingulate in generating confidence weighted error signals (Sarafyazd and Jazayeri, 2019). The coordinated computations across these regions is effectively the neural substrate of forming internal representations upon which reasoning models are built (Langdon et al., 2022).
The key aspect of the framework that we offer here, is that the associative thalamus provides a functional bridge across these specialized frontal areas, allowing their interactions to be both dynamically efficient and highly flexible, depending on the behavioral context (Schmitt et al., 2017; Rikhye et al., 2018b; Hummos et al., 2022). Therefore, perturbations within any of these core associative cortical areas, the thalamus itself, or their interactive computations is likely to result in a variety of cognitive disruptions at the behavioral level, which present in patients along the psychosis spectrum. Perhaps more critically, is an essential neurobiological property whereby the thalamus represents a bottleneck for information exchange between distributed association cortical areas. Therefore, we posit that the thalamus may be a particularly sensitive access point for intervention in ways that may remain agnostic to where the primary perturbation actually is.
The functional role of thalamus in cognition
As noted, the thalamus is a centrally-located structure in the mammalian forebrain, which is traditionally divided into individual nuclei based on Nissl staining and gross connectivity (Jones, 2007). There are over fifty distinct nuclei, which may be grouped into seven major anatomical divisions: anterior, medial, lateral, ventral, intralaminar, midline and posterior (Rikhye et al., 2018b). At the microcircuit level, these nuclei are largely composed of excitatory projection neurons that are devoid of local lateral connections (Jones, 2007; Rikhye et al., 2018b; Halassa and Sherman, 2019). The thalamic reticular nucleus is a shell of GABAergic neurons that surrounds these excitatory nuclei (Pinault, 2004; Crabtree, 2018; Takata, 2020). In primates, excitatory thalamic nuclei contain local inhibitory interneurons (Jager et al., 2021), but little is known about their function beyond a few well-studied cases. For example, in the lateral geniculate nucleus, interneurons are thought to engage in a push-pull type of operation that ultimately improves signal-to-noise ratio of thalamocortical visual input transmission (Hirsch et al., 2015). Because much basic physiology is carried out in rodents, and the rodent thalamus is devoid of local interneurons outside of the lateral geniculate, we know little about the general properties of these circuits. Instead, considerable focus has been placed on the reticular nucleus, which provides a major source of inhibition to thalamic projection neurons across all nuclei (Halassa et al., 2014; Wimmer et al., 2015; Halassa and Acsády, 2016).
Given the lack of local excitatory recurrence in the thalamic nuclei, the functionality of thalamic projection neurons is anchored in their long-range input-output connectivity patterns (Halassa and Sherman, 2019). Arguably, the best understood thalamic structures are those involved in sensory processing, such as the lateral and medial geniculate (vision and audition), and the posteromedial nucleus (somatosensation). These structures are often referred to as thalamic “relays,” transmitting inputs from sensory organs to cortex, with minimal spatial transformation of these sensory signals en route to their primary cortical targets. The idea is consistent with the observation that lateral geniculate neurons, which are arguably the best studied in the entire mammalian thalamus, show spatial responses very similar to those exhibited by their retinal inputs (Hubel, 1960). In fact, individual geniculate neurons are driven by as few as three retinal ganglion cell inputs with similar on-off spatial responses (Usrey et al., 1999), suggesting that the thalamus is a computationally-redundant station from the retina to primary visual cortex [although this argument is challenged in the temporal domain (Briggs and Usrey, 2005)]. In this pathway, the transformation from center-surround seen in retina to oriented edges and local motion vectors happens at the thalamo-recipient cortical layer (Layer IV) (Reid and Alonso, 1995).
Recent studies outside of primary sensory thalamic areas have challenged the relay view, and ascribed a critical functional role for the thalamus in cognitive computations. For instance, studies in associative thalamic structures like the mediodorsal thalamus and pulvinar have shown that these nuclei may have convergent inputs from multiple cortical areas and that, in contrast to primary relay nuclei, they can integrate and transform these inputs into novel representations, including temporal context (Rikhye et al., 2018a), confidence (Komura et al., 2013) and sensory uncertainty (Mukherjee et al., 2021). Such representations, in turn, may be utilized as cognitive control signals that functionally regulate distributed cortical dynamics rather than relay signals in the classical sense (Halassa and Kastner, 2017).
Consistent with this revised model of the thalamus (Figure 1), work in the non-human primate pulvinar has shown that in attentional tasks, this thalamic structure plays critical roles in coordinating activity patterns across extrastriate cortical areas (Halassa and Kastner, 2017). Specifically, work by Saalmann et al. (2012) has shown that interactions between early and late stations of the dorsal visual pathway in the alpha-band range, are temporally coordinated by an intermediary connecting pulvinar. More recently, the same group showed that this relationship is causal; inactivation of the pulvinar diminishes this cortico-cortical alpha band coherence in a task-specific manner (Eradath et al., 2021). Work by Komura et al. (2013), also in the non-human primate, has shown that pulvinar neurons generate summary statistic type responses in a classical visual dot motion task. Specifically, when animals are required to make a perceptual judgment on these well-studied stimuli, pulvinar neurons show response patterns that are not reflective of the dominant motion direction, as is the case in cortical area LIP (lateral intraparietal) for example, but rather their responses reflect the coherence as a scalar quantity regardless of motion direction. Computational modeling of these responses suggests they may emerge due to convergence of these two motion-sensitive cortical ensembles onto single pulvinar neurons (Jaramillo et al., 2019). Therefore, responses of primate pulvinar neurons are not a simple reflection of upstream inputs, but under certain conditions can reflect a transformation of a vector input to a scalar signal. Adding Kastner’s work to these findings paints a picture in which the pulvinar may not “relay” such signals, but rather generate an output that coordinates activity patterns across distributed cortical ensembles (Halassa and Kastner, 2017).
Similar to the pulvinar, the mediodorsal thalamus establishes widespread connections across multiple cortical areas (Phillips et al., 2021). However, rather than being connected to higher-order visual areas, the mediodorsal thalamus is connected to multiple frontal cortical areas (Giguere and Goldman-Rakic, 1988). Recently, multiple studies have examined mediodorsal function in rodents, and showed that neurons within this region have non-relay properties that might be similar to the pulvinar studies described above. Specifically, in a cross-modal divided attention task developed in mice, mediodorsal neurons show unique response profiles that are distinct from their connected cortical areas. In the prefrontal cortex, neurons encode the inputs and outputs of the cross-modal task, whereas thalamic neurons encode changes in input patterns over both long and short timescales. Encoding of the long term input changes reflects the statistical regularity of inputs over multi-trial timescale, or the “cueing context” (Rikhye et al., 2018a). Encoding of short term input changes reflects their variance or uncertainty (Mukherjee et al., 2021). This may reflect an interesting point of divergence between the mediodorsal encoding of uncertainty versus the pulvinar encoding the opposite, confidence (Komura et al., 2013; Mukherjee et al., 2021). Critically, these uncertainty responses have not only been found in non-human animal studies, but also in task-based fMRI in humans (Grinband et al., 2006; Kosciessa et al., 2021). Therefore, although thalamic microcircuitry may be far simpler than that of the cortex, it is nonetheless likely capable of performing important computational transformations that are critical for coordination of distributed cortical signals and in turn cognitive operations. The rodent studies have also shown that the thalamic outputs do not necessarily drive cortical responses in the classical sense, but can drive feedforward inhibition to slow cortical dynamics in certain contexts, or alternatively disinhibit cortical ensembles to implement non-linear gain control of cortical activity patterns in others (Delevich et al., 2015; Logiaco et al., 2021).
In summary, the fact that associative thalamic structures provide a mechanism for regulating associative cortical computations in a precise manner, and in turn coordinate activity across large-scale cortical networks is consistent with our overall thesis that associative thalamic structures could be leveraged to identify and target cognitive control deficits in PSD.
Evidence for thalamocortical circuit alterations in psychosis spectrum disorders
BOLD neuroimaging
Advancing research in the field of PSD will require innovation in non-invasive neuroimaging which will provide the opportunity to link observed neural changes to the diversity of symptoms in this syndrome. More specifically, progress will be accelerated by neuroimaging signals that can be optimized for risk prediction, diagnostics, treatment development and prognosis. Here we argue that the thalamus presents a uniquely powerful opportunity for PSD neuroimaging biomarker development which may be linked to specific symptoms.
Human neuroimaging has witnessed nothing short of a revolution since the discovery of a phenomenon called “resting-state,” which relies on the coordinated spontaneous activity of large-scale neural systems that can be imaged via functional magnetic resonance imaging sensitive to blood-oxygen-level-dependent (BOLD) signal changes. It is now widely accepted that BOLD fMRI signal exhibits spatiotemporal coherence in humans. These relationships can be quantified using statistical association (i.e., Spearman’s correlation) (Biswal et al., 1995, 2010; Fox and Raichle, 2007; He et al., 2009; Deco et al., 2011), which in turn reveal large-scale spatio-temporal coordination between neural systems; i.e., resting-state functional connectivity (rs-FC). For instance, a canonical system that can be reliably quantified via rs-FC is the “default mode network”–a set of cortical areas that typically show strong suppression during active cognitive task performance (Shulman et al., 1997; Mantini et al., 2011; Anticevic et al., 2012a; Raichle, 2015). This approach can be extended, in principle, across any cortical area (Biswal et al., 1995; Yang et al., 2007; Zhou et al., 2007; Andoh et al., 2015; Taren et al., 2017) or subcortical structure (Cao et al., 2009; Roy et al., 2009; Tang et al., 2011; Szewczyk-Krolikowski et al., 2014; Schleifer et al., 2019), with increasing quantitative sophistication (Allen et al., 2011; Suk et al., 2016; Haak et al., 2018; Shafiei et al., 2020; Ji et al., 2021). Therefore, the “resting state” modality provides a very attractive approach to characterize disruptions in wide-spread neural systems that may occur in neuropsychiatric illness.
Indeed, studies in PSD and schizophrenia specifically have been some of the first clinical applications of rs-FC approaches to test the prevailing hypothesis that this illness causes disruptions in large-scale functional neural connectivity. Here the thalamus presented an ideal neural target for characterizing rs-FC alterations in schizophrenia (Anticevic et al., 2014a; Wang et al., 2015; Giraldo-Chica and Woodward, 2017). Indeed, the thalamus is a region which has emerged as an important locus of dysfunction across PSD neuroimaging studies, building on years of theory implicating its role in psychosis (Andreasen, 1997; Pergola et al., 2015; Steullet, 2020) (Figure 2). Examining functional and structural thalamic macro-connectivity capitalizes on key properties of this subcortical structure: (i) as noted, it is topographically connected to the entire cortex across its many nuclei, and thus may represent a node particularly sensitive to brain-wide neural disturbances that may cause symptoms observed in schizophrenia; (ii) the thalamus contains segregated nuclei, providing a lens for examining parallel yet distributed large-scale structural and functional dysconnectivity in schizophrenia patients, thus bypassing an otherwise daunting feature mapping problem; (iii) identifying thalamic nuclei can be reliably automated on an individual subject basis via whole-brain neuroimaging segmentation tools that leverage high-resolution structural scans (Fischl et al., 2004, 2002), which in turn allows for reliable and robust estimation of its functional coupling and/or task-evoked signals.
The first evidence of disrupted thalamo-cortical rs-FC in schizophrenia was reported by Welsh et al. (2008) in a small sample of n = 11 patients. They found that the mediodorsal thalamus exhibited rs-FC reductions with the prefrontal cortex in this initial study. This preliminary but promising effect was subsequently confirmed by Woodward et al. (2012) in a much larger sample of chronic schizophrenia patients. Specifically, they “seeded” large cortical areas that are known to form distinct patterns of cortico-thalamic connectivity and tested if these cortical territories form distinct patterns of rs-FC onto the thalamus in patients relative to healthy controls (Woodward et al., 2012). First, they replicated the Welsch effect showing reduced connectivity from the prefrontal cortex onto the mediodorsal thalamus. Second, they also discovered that sensorimotor cortices exhibit increased thalamic connectivity onto thalamic nuclei in patients with schizophrenia. This phenomenon of bi-directional disruption gives rise to the notion that there is indeed a lack of coordinated information flow through the thalamus in PSD, but in a way that uniquely affects association cortex relative to primary cortical areas (Woodward et al., 2012). A parallel study (Anticevic et al., 2014a) showed that when “seeding” the thalamus specifically (as opposed to large cortical regions), patients with schizophrenia exhibit a robust, bi-directional shift in thalamocortical connectivity across large-scale cortical areas. More specifically, this study found that associative thalamic nuclei show elevated rs-FC with sensorimotor cortical areas but at the same time reductions in rs-FC with prefrontal cortex, dorsal striatum and cerebellum – again supporting the notion of disrupted cortico-thalamic information flow. In addition, Anticevic et al. (2014a) showed that this effect extends across the PSD as it was also apparent (albeit to a lesser extent) in bipolar patients with psychosis history. This core observation of thalamic hypo/hyper connectivity at rest has been replicated a number of times to date in chronic patients with schizophrenia (Anticevic et al., 2015; Woodward and Heckers, 2016; Avram et al., 2018; Wu et al., 2022). It is not an overstatement at this point to say that this is one of the more robust effects in psychiatric neuroimaging literature; for instance, this finding was replicated in a large meta-analysis conducted by Ramsay (2019) that combined 20 experiments from 17 publications that explored resting-state alterations in schizophrenia.
This robust and highly characteristic clinical neuroimaging observation could be leveraged to characterize PSD symptom heterogeneity. There are two fundamental ways in which patients with schizophrenia vary: First, individual patients vary in terms of symptom type and severity; Second, patients vary in terms of specific illness stage (e.g., prodrome versus early course versus chronic syndrome) (Kay et al., 1986; Schultz et al., 2007; Häfner et al., 2013). These two axes of heterogeneity likely interact; it is well understood that schizophrenia is a complex polygenic neurodevelopmental disorder (Schizophrenia Psychiatric Genome-Wide Association Study (GWAS) Consortium., 2011; Ripke et al., 2013; Purcell et al., 2014; Schizophrenia Working Group of the Psychiatric Genomics Consortium., 2014; Rees et al., 2015; Fromer et al., 2016; Germine et al., 2016; Li et al., 2017; Lam et al., 2019; Smeland et al., 2020) and that individual patients present with highly variable and distinctly complex individualized symptom progression, across prodrome, early onset, and chronic stages (McGlashan and Fenton, 1993; Parnas, 1999; MacDonald and Schulz, 2009; Cole et al., 2011). Moreover, not all patients respond equally well even to available lines of care (i.e., 1St and 2nd generation antipsychotics). This implies that there is likely not one root cause for all symptoms across the PST spectrum, or alternatively that a ‘core’ disruption may cause downstream neural alterations that in turn give rise to the broad spectrum of PSD symptoms. However, it is likely possible that no matter the cause, all symptom expression in some way corrupts information flow through the thalamus, perhaps in a symptom-specific way. In support of this notion, the pattern of thalamic under-connectivity with prefrontal cortex and over-connectivity with sensorimotor cortex has been reported in patients during early and later stages of psychosis (Woodward and Heckers, 2016), as well as individuals at high risk for psychosis (Anticevic et al., 2015). Similar to findings in idiopathic PSD, Schleifer et al. (2019) reported thalamic rs-FC alterations in youth with 22q11 deletion syndrome. This observation is vital as 22q11 deletion syndrome is a rare genetic mutation that is associated with 30× higher risk for developing psychosis later in life. While not causal, this genetic risk supports the notion that thalamic circuits may be a neural marker for psychosis risk regardless of etiology. In fact, the hyper/hypo thalamic dysconnectivity pattern was even stronger for a subset of at-risk patients who later progressed to full-blown illness, supporting the idea that its disruption may track illness severity (Anticevic et al., 2015). Collectively, these findings strongly support the model whereby thalamic dysconnectivity may be a core feature of PSD or at least a neural marker that is associated with both genetic and clinical risk, which emerges prior to full disease expression (Anticevic et al., 2015; Woodward and Heckers, 2016; Schleifer et al., 2019). This places the thalamus in a key position to develop the next generation of targeted rs-FC measures that link thalamocortical dysconnectivity to PSD symptom variation.
As noted, the thalamus is composed of multiple nuclei that form different types of input and output connections with cortex and striatum, in turn forming functional loops. In the context of understanding neural disruptions in PSD it is particularly important to distinguish between sensory and associative thalamus, which form distinct large-scale connection patterns onto cortex in the service of two fundamentally different types of operations: primary sensory relays from the environment versus coordination and regulation of association cortex in the service of cognitive function. Therefore, one strong prediction would be that the association thalamic connectivity may be preferentially affected by PSD – a syndrome that corrupts computations relevant for cognitive control. Consistent with this notion, the mediodorsal thalamus appears to drive the main observation of neural dysconnectivity in schizophrenia; recent studies have found reduced connectivity between higher-order thalamus and dorsal striatum, suggesting altered prefrontal cortico-striato-thalamo-cortical functional connectivity (Anticevic et al., 2014a; Avram et al., 2018; Cao et al., 2018; Ji et al., 2019a). This observation may hold the key for dissecting the roles of distinct associative thalamo-cortical loops in the heterogenous symptoms associated with PSD. The key hypothesis here is that associative thalamocortical loops would be variably affected as a function of different PSD symptoms (Peters et al., 2016). For instance, patients with thought disorder may exhibit functional alterations in thalamic circuits that connect to the lateral frontal cortex and to the fronto-parietal control network (see Figure 3). In contrast, patients with severe hallucinations (but perhaps less thought disorder) may exhibit deficits in thalamic circuits regulating sensory cortex. Of note, associative thalamic circuits like the pulvinar project to primary sensory cortical areas and regulate the balance of responsiveness to feedforward versus feedback inputs (Cortes and van Vreeswijk, 2012; Jaramillo et al., 2019). This point is critical, as the arbitration between feedforward and feedback at different levels of sensory hierarchy is at the heart of many predictive processing theories of hallucinations (Wilkinson, 2014; Sterzer et al., 2018). In general, examining the thalamus with high spatiotemporal resolution is within the reach of state-of-the-art BOLD fMRI (Figure 3), and could provide much needed insight into PSD etiology, stratification and treatment monitoring. These lines of investigation can be augmented by parallel work in the pharmacological domain, which we discuss next.
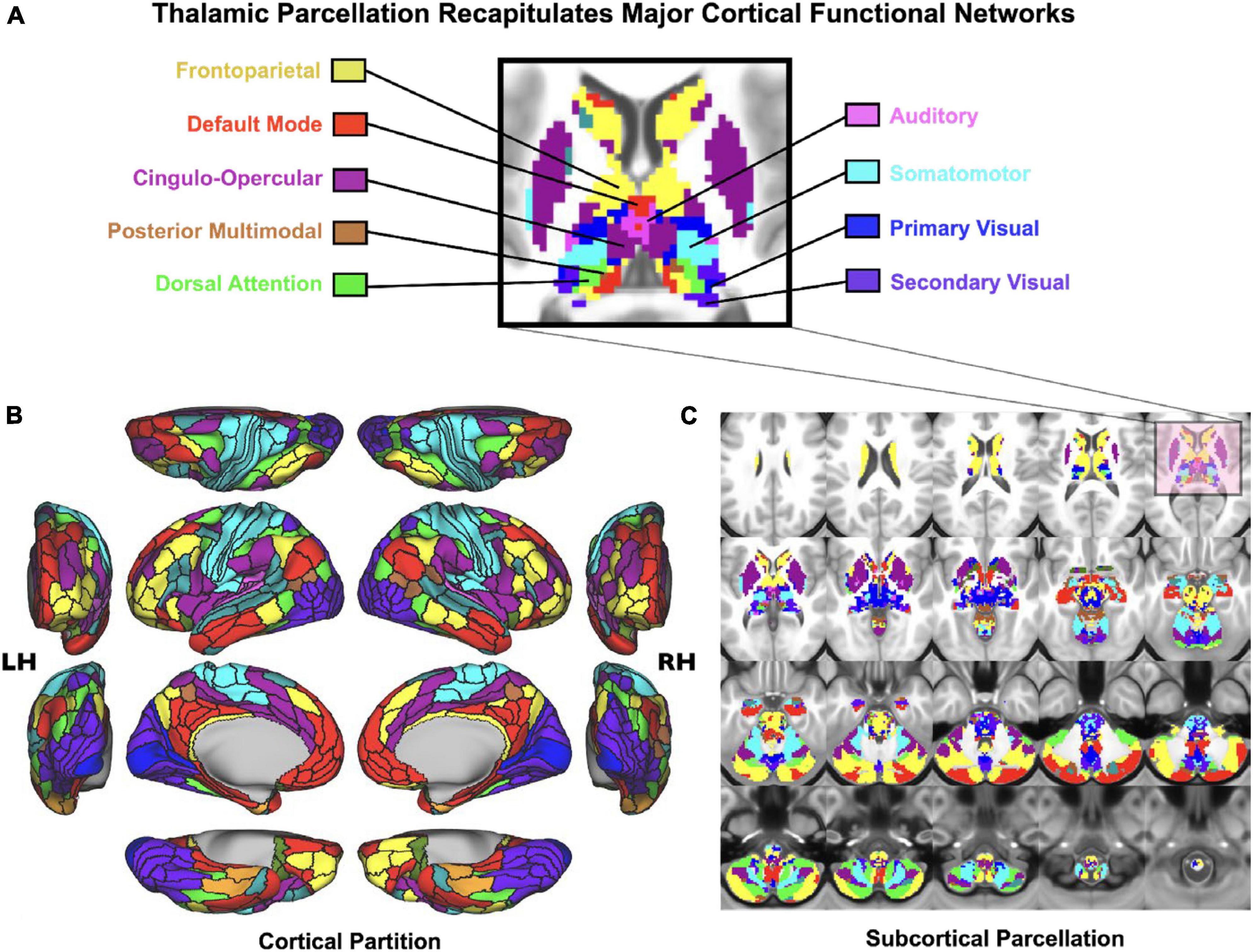
Figure 3. Cortico-subcortical whole-brain functional network parcellation derived from the Human Connectome Project dataset. (A) Zoomed in axial view onto the thalamus and striatum. Each color represents voxels mapping to a given functional network. (B) Cortical functional network view. (C) Subcortical functional network view. This effect highlights that it is possible to reliably and robustly map functional subdivisions of major sub-thalamic circuits that functionally couple with brain-wide networks Adapted from Ji et al. (2019b). This supports the notion that the thalamus indeed provides a functional “lens” onto distributed yet segregated functional pathways.
Pharmacological neuroimaging
Studies have concluded that, although PSD has a strong heritable component, there is considerable heterogeneity in the types of genetic changes underlying this syndrome (Chumakov et al., 2002; Straub et al., 2002). This has made it difficult to look for mechanistic insights based on analysis at the genetic level due to small effects across many genes (Craddock et al., 2007). Luckily, a number of pharmacological manipulations are known to replicate behavioral features of PSD, and therefore offer a tool to uncover mechanisms. Cardinal examples are ketamine, lysergic acid diethylamide (LSD), psilocybin, and delta-9-tetrahydrocannabinol (THC) (Paparelli et al., 2011; Steeds et al., 2015). The fact that these compounds induce key symptoms of PSD, yet have fundamental differences in their molecular site of action, indicates that overemphasizing the molecular level of analysis in both explaining and treating PSD symptoms may be misplaced. Critically, some of the large-scale neural system perturbations that impinge upon associative thalamocortical processing appear to be impacted by these diverse compounds (Höflich et al., 2015; Avram et al., 2022). It is also worth repeating that PSD symptomatology is quite heterogeneous and similarly these various compounds may individually induce stereotypical behaviors, but collectively reproduce this heterogeneity.
Ketamine is a non-competitive NMDA receptor antagonist that rapidly and transiently induces symptoms seen in PSD when administered at subanesthetic doses, predominantly impacting executive function (Krystal et al., 2003). A working model is that ketamine at these doses (i.e., 0.6 mg/kg) preferentially blocks NMDA receptors on interneurons compared to excitatory neurons resulting in output disinhibition (i.e., causing an increase in excitatory/inhibitory E/I ratio). At the mesoscopic scale, this results in reduced precision of neural representation underlying short term memory and integration (Morgan and Curran, 2006). At the macroscale, these changes translate to large-scale network perturbations that underlie the various symptoms of PSD (Olney et al., 1999). In support of these ideas, one study (Höflich et al., 2015) showed that administration of ketamine leads to alterations in thalamic functional connectivity at rest similar to the patterns reported in PSD. Anticevic et al. (2012b) showed that ketamine administration in a spatial working memory task altered task-evoked activity patterns that were also accompanied by altered resting state functional connectivity in the same networks. This experimental observation was captured by a neural model that simulates ketamine’s effect by elevating E/I ratio (Anticevic et al., 2012b). Given that thalamic inputs have recently been shown to independently modulate E and I components of cortical networks (Mukherjee et al., 2021), future models that incorporate thalamic microcircuits will provide important insights into how changes in E/I balance across distributed cortical systems may contribute to the dysconnectivity patterns seen under ketamine, and ultimately in PSD itself (Mukherjee et al., 2020; Lam et al., 2022).
Lysergic acid diethylamide (LSD) is a psychedelic substance, which activates serotonin 5HT2A receptors and induces symptoms of PSD with a dominant effect on perception and thought organization (De Gregorio et al., 2016; Preller et al., 2019). As with ketamine, LSD administration alters connectivity across thalamocortical circuits (Preller et al., 2019). Specifically, LSD appears to enhance thalamic connectivity to a number of cortical areas at rest, which may be related to altered sensory experiences under LSD (Müller et al., 2017). It will be interesting to investigate the specific impact of LSD on associative thalamic structures and their engagement in reasoning operations. In fact, this particular avenue of research may be quite critical in testing cognitive explanations for perceptual disruption in PSD (Avram et al., 2022).
Tetrahydrocannabinol is a compound that activates endogenous endocannabinoid receptors, which are known to modulate E/I balance across cortical circuitry (den Boon et al., 2015). Administering THC in otherwise healthy humans elicits key symptoms of PSD, including delusional thought content (Kleinloog et al., 2014). Similar to both ketamine and LSD, THC also perturbs large-scale neural system organization, and reproduces thalamocortical dysconnectivity to some extent (Camchong et al., 2017; Cupo et al., 2021).
Collectively, these human neuroimaging studies highlight the value of using pharmacology as a tool to perturb the brain in a manner that reproduces core symptoms of PSD. A major conclusion of these studies is that thalamocortical dysconnectivity may be an obligatory intermediate phenotype when mimicking PSD behavior. If true, this supports an etiological role of these circuits in psychosis. Future studies can exploit the individual differences within and across these manipulations in order to make even more precise mechanistic discoveries at the neural systems level. It is worth noting that the speed of these interventions provides therapeutic hope. For example, the fact that, under some conditions, certain individuals can experience well-formed delusions indicates that this feature of PSD may not require years of aberrant neural processing in order to form (Favrat et al., 2005), but rather may be the result of a rapid perturbation in executive control of mnemonic processing (Krystal et al., 2000; Honey et al., 2004). This provisional conclusion indicates that perhaps reversing these symptoms may also be achievable with mechanistically-informed targeted approaches.
Computational modeling
As described above, pharmacological neuroimaging can reveal how a molecular perturbation produces systems-level effects in brain-wide neural dynamics, which in turn correlate with evoked symptoms. Such experimental studies highlight the explanatory gaps across levels of analysis–from receptors to circuit, and behavior–that need to be bridged to have a mechanistic understanding of how disease processes and therapeutics impact system-level brain function and in turn relate to symptoms. One approach to integrate findings across levels is through computational modeling of neural systems. Computational models of thalamic and cortical circuits offer opportunities to study the impact of hypothesized molecular alterations on neural dynamics and cognitive function relevant to PSD and other neuropsychiatric disorders.
Recent studies using biophysically grounded circuit models have characterized mechanisms for how alterations to excitation-inhibition balance can impact neural function and cognitive behavior. For instance, in a thalamic circuit model inhibition via the reticular nucleus enables effective regulation of thalamic processing by top-down cortical control signals (Min, 2010). Biophysically grounded models of association cortical circuits have been developed for core cognitive functions such as decision making. In these models, perturbations of excitation-inhibition balance impacts the integration of evidence for decision making leading to behavioral deficits (Lam et al., 2022). An important future direction is to complement these models with performance optimized artificial neural networks that can also be fit to patient data. Such models can simultaneously do so while also making predictions about neural signals (Hummos et al., 2022). Another exciting direction is to combine modeling with theoretical analysis, which would answer “why” questions regarding thalamocortical organization (Wang and Halassa, 2022).
Computational models of large-scale brain network dynamics have also been productively applied to clinical and pharmacological neuroimaging. These models typically simulate a brain region in terms of its overall neural activity level (Murray et al., 2018; Shine et al., 2021). Correlated fluctuations across regions arise from inter-regional interactions via long-range projections which can be estimated in humans via diffusion tractography. Such models have been applied to simulate resting-state fMRI in patients with schizophrenia, and pharmacological effects of LSD. A recent focus in this literature underscores the importance of heterogeneity of local circuit properties across brain regions, such as hierarchical specialization from sensory to associative cortical regions (Demirtas et al.2019). Prior modeling studies have focused on cortico-cortical interactions but have yet to incorporate thalamic nuclei. Future modeling incorporating thalamo-cortical interactions can help explore the role of associative thalamus in shaping large-scale brain network dynamics and their alteration in PSD or by pharmacology.
A translational framework for thalamocortical system targeting in PSD
Targeting the thalamus with deep brain stimulation (DBS), or more recently non-invasive ultrasound, has been useful in a number of clinical applications. Could a similar approach be useful in targeting treatment resistant PSD? This question is quite pertinent as ∼30% of patients continue to experience debilitating symptoms despite treatment with clozapine as their last resort antipsychotic agent (Lally et al., 2016).
As highlighted throughout this perspective, the thalamus occupies a central location not only in forebrain structural organization but its functional one as well. This conclusion is supported by both invasive recordings in non-human animals and neuroimaging studies in humans. The animal studies provide mechanistic insight that is hard to obtain from human studies, whereas the human studies provide direct relevance to cognitive operations that are difficult to implement in animals. Therefore, the combination of these approaches is necessary and jointly support the idea that associative thalamic structures are engaged in regulating the interactions within and across associative cortical areas, and that perturbations within these areas lead to a host of abnormalities characteristic of PSD.
This mesoscopic-to-macroscopic level of description is critical for bridging the findings at the molecular and cellular level with PSD symptoms, and ultimately develop the next generation diagnostics and therapeutics. Consistent with this notion, a recent circuit study on mice identified two distinct cell types in the mediodorsal thalamus that either enhance or suppress task-relevant prefrontal activity patterns (Mukherjee et al., 2021). The key bridging link to schizophrenia is that these cell types straddle two major hypotheses of its etiology at the molecular level–dopamine and parvalbumin interneurons. Specifically, the mediodorsal cell type that suppresses prefrontal activity patterns does so through the preferential innervation of prefrontal parvalbumin interneurons, and the experimental suppression of this thalamic subpopulation leads to a “jumping to conclusions” phenotype reminiscent of human PSD (Evans et al., 2015). This is because these thalamic cells preferentially suppress unreliable cortical inputs based on their computation for sensory uncertainty, allowing for appropriate deliberation when faced with noisy evidence. The second thalamic cell type is one that expresses the dopamine D2 receptor, and its activation of prefrontal disinhibitory interneurons (VIP+) enhances prefrontal inputs. This allows the prefrontal cortex to more effectively process sparse inputs by boosting these otherwise faint signals. Interestingly, a number of studies examining D2 binding in the human thalamus show that it is diminished in schizophrenia (Talvik et al., 2003; Yasuno et al., 2004). This is particularly salient in associative thalamic regions that form loops with the prefrontal cortex. Understanding how these changes relate to thalamic regulation of prefrontal dynamics may be a critical next step, and may clarify whether existing therapeutic strategies (e.g., D2 antagonists) are helpful or, in fact, add to the existing deficits. Given the noted PSD heterogeneity, one testable hypothesis is that certain PSD patients with thalamic D2 reduction are particularly impaired in appropriate prefrontal functions (e.g., working memory and task switching). This hypothesis can be tested through a combination of task-based fMRI and D2 PET imaging. In this context, resting state fMRI may serve as an additional tool of stratifying patients, and ultimately predicting their response to different types of treatments (including existing classes of antipsychotics). Such studies have the capacity to inform rational and patient-specific selection strategies for clinical trial design.
The studies above highlight an important idea; translational progress may be best achieved when it focuses on evolutionarily conserved areas between the human brain and its non-human counterparts. It is no secret that the human cortex is one of the most fascinating pieces of machinery nature has generated over the last 4 billion years of evolution on this planet. However, its unique expansion poses a challenge, insofar that many of its divisions are difficult to map on species normally studied in the laboratory (including macaque monkeys). The thalamus may provide unique translational opportunities in that domain; there are far fewer disputes about homology across divisions of the thalamus between species (even with mice) compared to, say, areas of frontal cortex (Neubert et al., 2014; Carlén, 2017).
That said, we should mention that the mechanistic accessibility that rodents provide needs to be augmented by the cognitive complexity that non-human primate models afford. These organisms, which can perform much higher level cognitive functions including well-parametrized versions of cognitive tasks used in PSD clinical practice (Castner et al., 2004; Ebitz et al., 2020), are indispensable in the larger context of determining the precise circuit perturbations in PSD.
Similar to non-human primates, our view is that computational models will play essential bridging roles across levels of analysis in the quest for next generation PSD diagnostics and therapeutics. In addition to biophysically constrained models, which provide a link from molecules to circuit function (Wang, 2010), recent advances in task-optimized neural network models promise to bridge circuit function to behavior (Wang et al., 2018; Richards et al., 2019; Schrimpf et al., 2020). The integration of these two modeling traditions, with the additional constraints offered by normative behavioral models (Collins and Shenhav, 2022), is likely going to be key in the community’s broader goal of understanding psychosis. In fact, there will be certain questions that may be impossible to test in animals (including humans), in which different classes of neural models end up being a better testbed (e.g., specific computational theories of cognition that require dynamical perturbations that cannot be experimentally implemented at this point). Importantly, effort in the direction of imposing brain-inspired architectural constraints on these task-optimized networks, suggest that we may even be able to use them to understand the specific role(s) of associative thalamic circuits in cognitive computations and how they can be leveraged to rescue cognitive abnormalities in PSD.
Conclusion
The thalamus has emerged as a critical node in large-scale functional forebrain organization. Its continued exploration may offer mechanistic insights into how the brain implements cognitive operations whose perturbation, we have argued, form the basis of most PSD symptoms. Given the recent advances in targeting the thalamus through DBS and focused ultrasound, this new understanding may tell us where in the thalamus to target and when in the illness to do so.
Data availability statement
The original contributions presented in this study are included in the article/supplementary material, further inquiries can be directed to the corresponding authors.
Author contributions
AA and MH conceptualized and wrote the manuscript. Both authors contributed to the article and approved the submitted version.
Conflict of interest
AA was a member of the technology advisory board for Neumora Therapeutics, Inc. AA was a co-founder, board member, and a scientific advisor for Manifest Technologies, Inc.
The remaining author declares that the research was conducted in the absence of any commercial or financial relationships that could be construed as a potential conflict of interest.
Publisher’s note
All claims expressed in this article are solely those of the authors and do not necessarily represent those of their affiliated organizations, or those of the publisher, the editors and the reviewers. Any product that may be evaluated in this article, or claim that may be made by its manufacturer, is not guaranteed or endorsed by the publisher.
References
Abram, S. V., Roach, B. J., Fryer, S. L., Calhoun, V. D., Preda, A., van Erp, T. G. M., et al. (2022). Validation of ketamine as a pharmacological model of thalamic dysconnectivity across the illness course of schizophrenia. Mol. Psychiatry 2022:1502. doi: 10.1038/s41380-022-01502-0
Allen, E. A., Erhardt, E. B., Damaraju, E., Gruner, W., Segall, J. M., Silva, R. F., et al. (2011). A baseline for the multivariate comparison of resting-state networks. Front. Syst. Neurosci. 5:2. doi: 10.3389/fnsys.2011.00002
Andoh, J., Matsushita, R., and Zatorre, R. J. (2015). Asymmetric interhemispheric transfer in the auditory network: evidence from TMS, resting-state fMRI, and diffusion imaging. J. Neurosci. 35, 14602–14611. doi: 10.1523/JNEUROSCI.2333-15.2015
Andreasen, N. C. (1997). The role of the thalamus in schizophrenia. Can. J. Psychiatry 42, 27–33. doi: 10.1177/070674379704200104
Anticevic, A., and Lisman, J. (2017). How can global alteration of excitation/inhibition balance lead to the local dysfunctions that underlie schizophrenia? Biol. Psychiatry 81, 818–820. doi: 10.1016/j.biopsych.2016.12.006
Anticevic, A., Cole, M. W., Murray, J. D., Corlett, P. R., Wang, X.-J., and Krystal, J. H. (2012a). The role of default network deactivation in cognition and disease. Trends Cogn. Sci. 16, 584–592. doi: 10.1016/j.tics.2012.10.008
Anticevic, A., Gancsos, M., Murray, J. D., Repovs, G., Driesen, N. R., Ennis, D. J., et al. (2012b). NMDA receptor function in large-scale anticorrelated neural systems with implications for cognition and schizophrenia. Proc. Natl. Acad. Sci. U.S.A. 109, 16720–16725. doi: 10.1073/pnas.1208494109
Anticevic, A., Cole, M. W., Repovs, G., Murray, J. D., Brumbaugh, M. S., Winkler, A. M., et al. (2014a). Characterizing thalamo-cortical disturbances in schizophrenia and bipolar illness. Cereb. Cortex 24, 3116–3130. doi: 10.1093/cercor/bht165
Anticevic, A., Yang, G., Savic, A., Murray, J. D., Cole, M. W., Repovs, G., et al. (2014b). Mediodorsal and visual thalamic connectivity differ in schizophrenia and bipolar disorder with and without psychosis history. Schizophr. Bull. 40, 1227–1243. doi: 10.1093/schbul/sbu100
Anticevic, A., Haut, K., Murray, J. D., Repovs, G., Yang, G. J., Diehl, C., et al. (2015). Association of thalamic dysconnectivity and conversion to psychosis in youth and young adults at elevated clinical risk. JAMA Psychiatry 72, 882–891. doi: 10.1001/jamapsychiatry.2015.0566
Auquier, P., Lançon, C., Rouillon, F., Lader, M., and Holmes, C. (2006). Mortality in schizophrenia. Pharmacoepidemiol. Drug Saf. 15, 873–879. doi: 10.1002/pds.1325
Averbeck, B., and O’Doherty, J. P. (2022). Reinforcement-learning in fronto-striatal circuits. Neuropsychopharmacology 47, 147–162. doi: 10.1038/s41386-021-01108-0
Avram, M., Brandl, F., Bäuml, J., and Sorg, C. (2018). Cortico-thalamic hypo- and hyperconnectivity extend consistently to basal ganglia in schizophrenia. Neuropsychopharmacology 43, 2239–2248. doi: 10.1038/s41386-018-0059-z
Avram, M., Müller, F., Rogg, H., Korda, A., Andreou, C., Holze, F., et al. (2022). Characterizing thalamocortical (dys)connectivity following d-amphetamine, LSD, and MDMA administration. Biol. Psychiatry Cogn. Neurosci. Neuro. 2022:3. doi: 10.1016/j.bpsc.2022.04.003
Avram, M., Rogg, H., Korda, A., Andreou, C., Müller, F., and Borgwardt, S. (2021). Bridging the gap? Altered thalamocortical connectivity in psychotic and psychedelic states. Front. Psychiatry 12:706017. doi: 10.3389/fpsyt.2021.706017
Baran, B., Karahanoðlu, F. I., Mylonas, D., Demanuele, C., Vangel, M., Stickgold, R., et al. (2019). Increased thalamocortical connectivity in schizophrenia correlates with sleep spindle deficits: evidence for a common pathophysiology. Biol. Psychiatry Cogn. Neurosci. Neuro. 4, 706–714. doi: 10.1016/j.bpsc.2019.04.012
Barch, D. M., and Ceaser, A. (2012). Cognition in schizophrenia: core psychological and neural mechanisms. Trends Cogn. Sci. 16, 27–34. doi: 10.1016/j.tics.2011.11.015
Bastos, A. M., Donoghue, J. A., Brincat, S. L., Mahnke, M., Yanar, J., Correa, J., et al. (2021). Neural effects of propofol-induced unconsciousness and its reversal using thalamic stimulation. Elife 10:60824. doi: 10.7554/eLife.60824
Behrendt, R.-P. (2006). Dysregulation of thalamic sensory “transmission” in schizophrenia: neurochemical vulnerability to hallucinations. J. Psychopharmacol. 20, 356–372. doi: 10.1177/0269881105057696
Biswal, B. B., Mennes, M., Zuo, X.-N., Gohel, S., Kelly, C., Smith, S. M., et al. (2010). Toward discovery science of human brain function. Proc. Natl. Acad. Sci. U.S.A. 107, 4734–4739. doi: 10.1073/pnas.0911855107
Biswal, B., Yetkin, F. Z., Haughton, V. M., and Hyde, J. S. (1995). Functional connectivity in the motor cortex of resting human brain using echo-planar MRI. Magn. Reson. Med. 34, 537–541. doi: 10.1002/mrm.1910340409
Briggs, F., and Usrey, W. M. (2005). Temporal properties of feedforward and feedback pathways between the thalamus and visual cortex in the ferret. Thalamus Relat. Syst. 3, 133–139. doi: 10.1017/S1472928807000131
Burgher, B., Whybird, G., Koussis, N., Scott, J. G., Cocchi, L., and Breakspear, M. (2021). Sub-optimal modulation of gain by the cognitive control system in young adults with early psychosis. Trans. Psychiatry 11:549. doi: 10.1038/s41398-021-01673-4
Camchong, J., Lim, K. O., and Kumra, S. (2017). Adverse effects of cannabis on adolescent brain development: a longitudinal study. Cereb. Cortex 27, 1922–1930. doi: 10.1093/cercor/bhw015
Cao, X., Cao, Q., Long, X., Sun, L., Sui, M., Zhu, C., et al. (2009). Abnormal resting-state functional connectivity patterns of the putamen in medication-naïve children with attention deficit hyperactivity disorder. Brain Res. 1303, 195–206. doi: 10.1016/j.brainres.2009.08.029
Cao, H., Chén, O. Y., Chung, Y., Forsyth, J. K., McEwen, S. C., Gee, D. G., et al. (2018). Cerebello-thalamo-cortical hyperconnectivity as a state-independent functional neural signature for psychosis prediction and characterization. Nat Commun. 9:3836. doi: 10.1038/s41467-018-06350-7
Cardella, V., and Gangemi, A. (2015). Reasoning in schizophrenia. review and analysis from the cognitive perspective. Clin. Neuropsychiatry. 12, 3–8.
Carlén, M. (2017). What constitutes the prefrontal cortex? Science 358, 478–482. doi: 10.1126/science.aan8868
Castner, S. A., Goldman-Rakic, P. S., and Williams, G. V. (2004). Animal models of working memory: insights for targeting cognitive dysfunction in schizophrenia. Psychopharmacology 174, 111–125. doi: 10.1007/s00213-003-1710-9
Chumakov, I., Blumenfeld, M., Guerassimenko, O., Cavarec, L., Palicio, M., Abderrahim, H., et al. (2002). Genetic and physiological data implicating the new human gene G72 and the gene for D-amino acid oxidase in schizophrenia. Proc. Natl. Acad. Sci. U.S.A. 99, 13675–13680. doi: 10.1073/pnas.182412499
Cole, M. W., Anticevic, A., Repovs, G., and Barch, D. (2011). Variable global dysconnectivity and individual differences in schizophrenia. Biol. Psychiatry 70, 43–50. doi: 10.1016/j.biopsych.2011.02.010
Collins, A. G. E., and Shenhav, A. (2022). Advances in modeling learning and decision-making in neuroscience. Neuropsychopharmacology 47, 104–118. doi: 10.1038/s41386-021-01126-y
Cortes, N., and van Vreeswijk, C. (2012). The role of pulvinar in the transmission of information in the visual hierarchy. Front. Comput. Neurosci. 6:29. doi: 10.3389/fncom.2012.00029
Crabtree, J. W. (2018). Functional diversity of thalamic reticular subnetworks. Front. Syst. Neurosci. 12:41. doi: 10.3389/fnsys.2018.00041
Craddock, N., O’Donovan, M. C., and Owen, M. J. (2007). Phenotypic and genetic complexity of psychosis: invited commentary on schizophrenia: a common disease caused by multiple rare alleles. Br. J. Psychiatry 190, 200–203. doi: 10.1192/bjp.bp.106.033761
Crail-Melendez, D., Atriano-Mendieta, C., Carrillo-Meza, R., and Ramirez-Bermudez, J. (2013). Schizophrenia-like psychosis associated with right lacunar thalamic infarct. Neurocase 19, 22–26. doi: 10.1080/13554794.2011.654211
Cronenwett, W. J., and Csernansky, J. (2010). “Thalamic pathology in schizophrenia,” in Behavioral neurobiology of schizophrenia and its treatment, ed. N. R. Swerdlow (Berlin), 509–528. doi: 10.1007/7854_2010_55
Cupo, L., Plitman, E., Guma, E., and Chakravarty, M. M. (2021). A systematic review of neuroimaging and acute cannabis exposure in age-of-risk for psychosis. Trans. Psychiatry 11, 217. doi: 10.1038/s41398-021-01295-w
De Gregorio, D., Comai, S., Posa, L., and Gobbi, G. (2016). d-lysergic acid diethylamide (LSD) as a model of psychosis: mechanism of action and pharmacology. Int. J. Mol. Sci. 17:953. doi: 10.3390/ijms17111953
Deco, G., Jirsa, V. K., and McIntosh, A. R. (2011). Emerging concepts for the dynamical organization of resting-state activity in the brain. Nat. Rev. Neurosci. 12, 43–56. doi: 10.1038/nrn2961
Delevich, K., Tucciarone, J., Huang, Z. J., and Li, B. (2015). The mediodorsal thalamus drives feedforward inhibition in the anterior cingulate cortex via parvalbumin interneurons. J. Neurosci. 35, 5743–5753. doi: 10.1523/JNEUROSCI.4565-14.2015
Demirtas, M., Burt, J. B., Helmer, M., Ji, J. L., Adkinson, B. D., Glasser, M. F., et al. (2019). Hierarchical heterogeneity across human cortex shapes large-scale neural dynamics. Neuron 101, 1181–1194.e13. doi: 10.1016/j.neuron.2019.01.017
den Boon, F. S., Werkman, T. R., Schaafsma-Zhao, Q., Houthuijs, K., Vitalis, T., Kruse, C. G., et al. (2015). Activation of type-1 cannabinoid receptor shifts the balance between excitation and inhibition towards excitation in layer II/III pyramidal neurons of the rat prelimbic cortex. Pflugers Arch. 467, 1551–1564. doi: 10.1007/s00424-014-1586-z
Dorph-Petersen, K.-A., and Lewis, D. A. (2017). Postmortem structural studies of the thalamus in schizophrenia. Schizophr. Res. 180, 28–35. doi: 10.1016/j.schres.2016.08.007
Ebitz, R. B., Tu, J. C., and Hayden, B. Y. (2020). Rules warp feature encoding in decision-making circuits. PLoS Biol. 18:e3000951. doi: 10.1371/journal.pbio.3000951
Eradath, M. K., Pinsk, M. A., and Kastner, S. (2021). A causal role for the pulvinar in coordinating task-independent cortico-cortical interactions. J. Comp. Neurol. 529, 3772–3784. doi: 10.1002/cne.25193
Evans, S. L., Averbeck, B. B., and Furl, N. (2015). Jumping to conclusions in schizophrenia. Neuropsychiatr. Dis. Treat. 11, 1615–1624. doi: 10.2147/NDT.S56870
Favrat, B., Ménétrey, A., Augsburger, M., Rothuizen, L. E., Appenzeller, M., Buclin, T., et al. (2005). Two cases of “cannabis acute psychosis” following the administration of oral cannabis. BMC Psychiatry 5:17. doi: 10.1186/1471-244X-5-17
Ferrarelli, F., and Tononi, G. (2011). The thalamic reticular nucleus and schizophrenia. Schizophr. Bull. 37, 306–315. doi: 10.1093/schbul/sbq142
Fischl, B., Salat, D. H., Busa, E., Albert, M., Dieterich, M., Haselgrove, C., et al. (2002). Whole brain segmentation: automated labeling of neuroanatomical structures in the human brain. Neuron 33, 341–355. doi: 10.1016/S0896-6273(02)00569-X
Fischl, B., Salat, D. H., van der Kouwe, A. J. W., Makris, N., Ségonne, F., Quinn, B. T., et al. (2004). Sequence-independent segmentation of magnetic resonance images. Neuroimage 23, S69–S84. doi: 10.1016/j.neuroimage.2004.07.016
Foss-Feig, J. H., Adkinson, B. D., Ji, J. L., Yang, G., Srihari, V. H., McPartland, J. C., et al. (2017). Searching for cross-diagnostic convergence: neural mechanisms governing excitation and inhibition balance in schizophrenia and autism spectrum disorders. Biol. Psychiatry 81, 848–861. doi: 10.1016/j.biopsych.2017.03.005
Fox, M. D., and Raichle, M. E. (2007). Spontaneous fluctuations in brain activity observed with functional magnetic resonance imaging. Nat. Rev. Neurosci. 8, 700–711. doi: 10.1038/nrn2201
Fromer, M., Roussos, P., Sieberts, S. K., Johnson, J. S., Kavanagh, D. H., Perumal, T. M., et al. (2016). Gene expression elucidates functional impact of polygenic risk for schizophrenia. Nat. Neurosci. 19, 1442–1453. doi: 10.1038/nn.4399
Fryer, S. L., Ferri, J. M., Roach, B. J., Loewy, R. L., Stuart, B. K., Anticevic, A., et al. (2021). Thalamic dysconnectivity in the psychosis risk syndrome and early illness schizophrenia. Psychol. Med. 2021, 1–9.
Garety, P. A., Freeman, D., Jolley, S., Dunn, G., Bebbington, P. E., Fowler, D. G., et al. (2005). Reasoning, emotions, and delusional conviction in psychosis. J. Abnorm. Psychol. 114, 373–384. doi: 10.1037/0021-843X.114.3.373
Germine, L., Robinson, E. B., Smoller, J. W., Calkins, M. E., Moore, T. M., Hakonarson, H., et al. (2016). Association between polygenic risk for schizophrenia, neurocognition and social cognition across development. Trans. Psychiatry 6:e924. doi: 10.1038/tp.2016.147
Giguere, M., and Goldman-Rakic, P. S. (1988). Mediodorsal nucleus: areal, laminar, and tangential distribution of afferents and efferents in the frontal lobe of rhesus monkeys. J. Comp. Neurol. 277, 195–213. doi: 10.1002/cne.902770204
Giraldo-Chica, M., and Woodward, N. D. (2017). Review of thalamocortical resting-state fMRI studies in schizophrenia. Schizophr. Res. 180, 58–63. doi: 10.1016/j.schres.2016.08.005
Grinband, J., Hirsch, J., and Ferrera, V. P. (2006). A neural representation of categorization uncertainty in the human brain. Neuron 49, 757–763. doi: 10.1016/j.neuron.2006.01.032
Haak, K. V., Marquand, A. F., and Beckmann, C. F. (2018). Connectopic mapping with resting-state fMRI. Neuroimage 170, 83–94. doi: 10.1016/j.neuroimage.2017.06.075
Häfner, H., Maurer, K., and der Heiden, W. (2013). ABC Schizophrenia study: an overview of results since 1996. Soc. Psychiatry Psychiatr. Epidemiol. 48, 1021–1031. doi: 10.1007/s00127-013-0700-4
Halassa, M. M. (2011). Thalamocortical dynamics of sleep: roles of purinergic neuromodulation. Sem. Cell Dev. Biol. 22, 245–251. doi: 10.1016/j.semcdb.2011.02.008
Halassa, M. M., and Acsády, L. (2016). Thalamic inhibition: diverse sources, diverse scales. Trends Neurosci. 39, 680–693. doi: 10.1016/j.tins.2016.08.001
Halassa, M. M., and Kastner, S. (2017). Thalamic functions in distributed cognitive control. Nat. Neurosci. 20, 1669–1679. doi: 10.1038/s41593-017-0020-1
Halassa, M. M., and Sherman, S. M. (2019). Thalamocortical circuit motifs: a general framework. Neuron 103, 762–770. doi: 10.1016/j.neuron.2019.06.005
Halassa, M. M., Chen, Z., Wimmer, R. D., Brunetti, P. M., Zhao, S., Zikopoulos, B., et al. (2014). State-dependent architecture of thalamic reticular subnetworks. Cell 158, 808–821. doi: 10.1016/j.cell.2014.06.025
Hazy, T. E., Frank, M. J., and O’reilly, R. C. (2007). Towards an executive without a homunculus: computational models of the prefrontal cortex/basal ganglia system. Philos. Trans. R. Soc. Lond. B Biol. Sci. 362, 1601–1613. doi: 10.1098/rstb.2007.2055
He, Y., Wang, J., Wang, L., Chen, Z. J., Yan, C., Yang, H., et al. (2009). Uncovering intrinsic modular organization of spontaneous brain activity in humans. PLoS One 4:e5226. doi: 10.1371/journal.pone.0005226
Hilgetag, C. C., and Goulas, A. (2020). “Hierarchy” in the organization of brain networks. Philos. Trans. R. Soc. Lond. B Biol. Sci. 375:20190319. doi: 10.1098/rstb.2019.0319
Hirsch, J. A., Wang, X., Sommer, F. T., and Martinez, L. M. (2015). How inhibitory circuits in the thalamus serve vision. Annu. Rev. Neurosci. 38, 309–329. doi: 10.1146/annurev-neuro-071013-014229
Höflich, A., Hahn, A., Küblböck, M., Kranz, G. S., Vanicek, T., Windischberger, C., et al. (2015). Ketamine-induced modulation of the thalamo-cortical network in healthy volunteers as a model for schizophrenia. Int. J. Neuropsychopharmacol. 18:40. doi: 10.1093/ijnp/pyv040
Honey, R. A. E., Honey, G. D., O’Loughlin, C., Sharar, S. R., Kumaran, D., Bullmore, E. T., et al. (2004). Acute ketamine administration alters the brain responses to executive demands in a verbal working memory task: an FMRI study. Neuropsychopharmacology 29, 1203–1214. doi: 10.1038/sj.npp.1300438
Huang, A. S., Rogers, B. P., Sheffield, J. M., Vandekar, S., Anticevic, A., and Woodward, N. D. (2021). Characterizing effects of age, sex and psychosis symptoms on thalamocortical functional connectivity in youth. Neuroimage 243:118562. doi: 10.1016/j.neuroimage.2021.118562
Hubel, D. H. (1960). Single unit activity in lateral geniculate body and optic tract of unrestrained cats. J. Physiol. 150, 91–104. doi: 10.1113/jphysiol.1960.sp006375
Hummos, A., Wang, B. A., Drammis, S., Halassa, M. M., and Pleger, B. (2022). Thalamic regulation of frontal interactions in human cognitive flexibility. PLoS Comput. Biol. 18:e1010500. doi: 10.1371/journal.pcbi.1010500
Jablensky, A. (2010). The diagnostic concept of schizophrenia: its history, evolution, and future prospects. Dialogues Clin. Neurosci. 12, 271–287. doi: 10.31887/DCNS.2010.12.3/ajablensky
Jager, P., Moore, G., Calpin, P., Durmishi, X., Salgarella, I., Menage, L., et al. (2021). Dual midbrain and forebrain origins of thalamic inhibitory interneurons. Elife 10:59272. doi: 10.7554/eLife.59272
Jaramillo, J., Mejias, J. F., and Wang, X.-J. (2019). Engagement of pulvino-cortical feedforward and feedback pathways in cognitive computations. Neuron 101, 321–336.e9. doi: 10.1016/j.neuron.2018.11.023
Ji, J. L., Diehl, C., Schleifer, C., Tamminga, C. A., Keshavan, M. S., Sweeney, J. A., et al. (2019a). Schizophrenia exhibits bi-directional brain-wide alterations in cortico-striato-cerebellar circuits. Cereb. Cortex 29, 4463–4487. doi: 10.1093/cercor/bhy306
Ji, J. L., Spronk, M., Kulkarni, K., Repovš, G., Anticevic, A., and Cole, M. W. (2019b). Mapping the human brain’s cortical-subcortical functional network organization. Neuroimage 185, 35–57. doi: 10.1016/j.neuroimage.2018.10.006
Ji, J. L., Helmer, M., Fonteneau, C., Burt, J. B., Tamayo, Z., Demšar, J., et al. (2021). Mapping brain-behavior space relationships along the psychosis spectrum. Elife 10:66968. doi: 10.7554/eLife.66968
Kay, S. R., Fiszbein, A., Lindenmayer, J. P., and Opler, L. A. (1986). Positive and negative syndromes in schizophrenia as a function of chronicity. Acta Psychiatr. Scand. 74, 507–518. doi: 10.1111/j.1600-0447.1986.tb06276.x
Kleinloog, D., Roozen, F., De Winter, W., Freijer, J., and Van Gerven, J. (2014). Profiling the subjective effects of Δ9-tetrahydrocannabinol using visual analogue scales. Int. J. Methods Psychiatr. Res. 23, 245–256. doi: 10.1002/mpr.1424
Komura, Y., Nikkuni, A., Hirashima, N., Uetake, T., and Miyamoto, A. (2013). Responses of pulvinar neurons reflect a subject’s confidence in visual categorization. Nat. Neurosci. 16, 749–755. doi: 10.1038/nn.3393
Kooyman, I., Dean, K., Harvey, S., and Walsh, E. (2007). Outcomes of public concern in schizophrenia. Br. J. Psychiatry Suppl. 50, s29–s36. doi: 10.1192/bjp.191.50.s29
Kosciessa, J. Q., Lindenberger, U., and Garrett, D. D. (2021). Thalamocortical excitability modulation guides human perception under uncertainty. Nat. Commun. 12:2430. doi: 10.1038/s41467-021-22511-7
Kraguljac, N. V., and Lahti, A. C. (2021). Neuroimaging as a window into the pathophysiological mechanisms of schizophrenia. Front. Psychiatry 12:613764. doi: 10.3389/fpsyt.2021.613764
Kraguljac, N. V., McDonald, W. M., Widge, A. S., Rodriguez, C. I., Tohen, M., and Nemeroff, C. B. (2021). Neuroimaging biomarkers in schizophrenia. Am. J. Psychiatry 178, 509–521. doi: 10.1176/appi.ajp.2020.20030340
Krystal, J. H., Bennett, A., Abi-Saab, D., Belger, A., Karper, L. P., D’Souza, D. C., et al. (2000). Dissociation of ketamine effects on rule acquisition and rule implementation: possible relevance to NMDA receptor contributions to executive cognitive functions. Biol. Psychiatry 47, 137–143. doi: 10.1016/S0006-3223(99)00097-9
Krystal, J. H., D’Souza, D. C., Mathalon, D., Perry, E., Belger, A., and Hoffman, R. (2003). NMDA receptor antagonist effects, cortical glutamatergic function, and schizophrenia: toward a paradigm shift in medication development. Psychopharmacology (Berl). 169:215–33. doi: 10.1007/s00213-003-1582-z
Lally, J., Gaughran, F., Timms, P., and Curran, S. R. (2016). Treatment-resistant schizophrenia: current insights on the pharmacogenomics of antipsychotics. Pharmgenomics. Pers. Med. 9, 117–129. doi: 10.2147/PGPM.S115741
Lam, M., Chen, C.-Y., Li, Z., Martin, A. R., Bryois, J., Ma, X., et al. (2019). Comparative genetic architectures of schizophrenia in East Asian and European populations. Nat. Genet. 51, 1670–1678. doi: 10.1038/s41588-019-0512-x
Lam, N. H., Borduqui, T., Hallak, J., Roque, A., Anticevic, A., Krystal, J. H., et al. (2022). Effects of altered excitation-inhibition balance on decision making in a cortical circuit model. J. Neurosci. 42, 1035–1053. doi: 10.1523/JNEUROSCI.1371-20.2021
Langdon, A., Botvinick, M., Nakahara, H., Tanaka, K., Matsumoto, M., and Kanai, R. (2022). Meta-learning, social cognition and consciousness in brains and machines. Neural Netw. 145, 80–89. doi: 10.1016/j.neunet.2021.10.004
Lee, D. J., Lozano, C. S., Dallapiazza, R. F., and Lozano, A. M. (2019). Current and future directions of deep brain stimulation for neurological and psychiatric disorders. J. Neurosurg. 131, 333–342. doi: 10.3171/2019.4.JNS181761
Li, Y., Zeng, W., Deng, J., Shi, Y., Nie, W., Luo, S., et al. (2022). Exploring dysconnectivity of the large-scale neurocognitive network across psychiatric disorders using spatiotemporal constrained nonnegative matrix factorization method. Cereb. Cortex 2022:503. doi: 10.1093/cercor/bhab503
Li, Z., Chen, J., Yu, H., He, L., Xu, Y., Zhang, D., et al. (2017). Genome-wide association analysis identifies 30 new susceptibility loci for schizophrenia. Nat. Genet. 49, 1576–1583. doi: 10.1038/ng.3973
Logiaco, L., Abbott, L. F., and Escola, S. (2021). Thalamic control of cortical dynamics in a model of flexible motor sequencing. Cell Rep. 35:109090. doi: 10.1016/j.celrep.2021.109090
MacDonald, A. W., and Schulz, S. C. (2009). What we know: findings that every theory of schizophrenia should explain. Schizophr. Bull. 35, 493–508. doi: 10.1093/schbul/sbp017
Macpherson, T., Churchland, A., Sejnowski, T., DiCarlo, J., Kamitani, Y., Takahashi, H., et al. (2021). Natural and artificial intelligence: a brief introduction to the interplay between AI and neuroscience research. Neural Netw. 144, 603–613. doi: 10.1016/j.neunet.2021.09.018
Mantini, D., Gerits, A., Nelissen, K., Durand, J.-B., Joly, O., Simone, L., et al. (2011). Default mode of brain function in monkeys. J. Neurosci. 31, 12954–12962. doi: 10.1523/JNEUROSCI.2318-11.2011
McGlashan, T. H., and Fenton, W. S. (1993). Subtype progression and pathophysiologic deterioration in early schizophrenia. Schizophr. Bull. 19, 71–84. doi: 10.1093/schbul/19.1.71
Min, B.-K. (2010). A thalamic reticular networking model of consciousness. Theor. Biol. Med. Model. 7:10. doi: 10.1186/1742-4682-7-10
Morgan, C. J. A., and Curran, H. V. (2006). Acute and chronic effects of ketamine upon human memory: a review. Psychopharmacology 188, 408–424. doi: 10.1007/s00213-006-0572-3
Mukherjee, A., Bajwa, N., Lam, N. H., Porrero, C., Clasca, F., and Halassa, M. M. (2020). Variation of connectivity across exemplar sensory and associative thalamocortical loops in the mouse. Elife 9:62554. doi: 10.7554/eLife.62554
Mukherjee, A., Lam, N. H., Wimmer, R. D., and Halassa, M. M. (2021). Thalamic circuits for independent control of prefrontal signal and noise. Nature 600, 100–104. doi: 10.1038/s41586-021-04056-3
Müller, F., Lenz, C., Dolder, P., Lang, U., Schmidt, A., Liechti, M., et al. (2017). Increased thalamic resting-state connectivity as a core driver of LSD-induced hallucinations. Acta Psychiatr. Scand. 136, 648–657. doi: 10.1111/acps.12818
Murray, J. D., and Anticevic, A. (2017). Toward understanding thalamocortical dysfunction in schizophrenia through computational models of neural circuit dynamics. Schizophr. Res. 180, 70–77. doi: 10.1016/j.schres.2016.10.021
Murray, J. D., DemirtaŞ, M., and Anticevic, A. (2018). Biophysical modeling of large-scale brain dynamics and applications for computational psychiatry. Biol. Psychiatry Cogn. Neurosci. Neuro. 3, 777–787. doi: 10.1016/j.bpsc.2018.07.004
Nakajima, M., and Halassa, M. M. (2017). Thalamic control of functional cortical connectivity. Curr. Opin. Neurobiol. 44, 127–131. doi: 10.1016/j.conb.2017.04.001
Neubert, F.-X., Mars, R. B., Thomas, A. G., Sallet, J., and Rushworth, M. F. S. (2014). Comparison of human ventral frontal cortex areas for cognitive control and language with areas in monkey frontal cortex. Neuron 81, 700–713. doi: 10.1016/j.neuron.2013.11.012
Olney, J. W., Newcomer, J. W., and Farber, N. B. (1999). NMDA receptor hypofunction model of schizophrenia. J. Psychiatr. Res. 33, 523–533. doi: 10.1016/S0022-3956(99)00029-1
Padoa-Schioppa, C., and Conen, K. E. (2017). Orbitofrontal cortex: a neural circuit for economic decisions. Neuron 96, 736–754. doi: 10.1016/j.neuron.2017.09.031
Paparelli, A., Di Forti, M., Morrison, P. D., and Murray, R. M. (2011). Drug-induced psychosis: how to avoid star gazing in schizophrenia research by looking at more obvious sources of light. Front. Behav. Neurosci. 5:1. doi: 10.3389/fnbeh.2011.00001
Parnas, J. (1999). From predisposition to psychosis: progression of symptoms in schizophrenia. Acta Psychiatr. Scand. Suppl. 395, 20–29. doi: 10.1111/j.1600-0447.1999.tb05979.x
Pergola, G., Selvaggi, P., Trizio, S., Bertolino, A., and Blasi, G. (2015). The role of the thalamus in schizophrenia from a neuroimaging perspective. Neurosci. Biobehav. Rev. 54, 57–75. doi: 10.1016/j.neubiorev.2015.01.013
Peters, S. K., Dunlop, K., and Downar, J. (2016). Cortico-striatal-thalamic loop circuits of the salience network: a central pathway in psychiatric disease and treatment. Front. Syst. Neurosci. 10:104. doi: 10.3389/fnsys.2016.00104
Phillips, J. M., Kambi, N. A., Redinbaugh, M. J., Mohanta, S., and Saalmann, Y. B. (2021). Disentangling the influences of multiple thalamic nuclei on prefrontal cortex and cognitive control. Neurosci. Biobehav. Rev. 128, 487–510. doi: 10.1016/j.neubiorev.2021.06.042
Picchioni, M. M., and Murray, R. M. (2007). Schizophrenia. BMJ 335, 91–95. doi: 10.1136/bmj.39227.616447.BE
Pinault, D. (2004). The thalamic reticular nucleus: structure, function and concept. Brain Res. Brain Res. Rev. 46, 1–31. doi: 10.1016/j.brainresrev.2004.04.008
Preller, K. H., Razi, A., Zeidman, P., Stämpfli, P., Friston, K. J., and Vollenweider, F. X. (2019). Effective connectivity changes in LSD-induced altered states of consciousness in humans. Proc. Natl. Acad. Sci. U.S.A. 116, 2743–2748. doi: 10.1073/pnas.1815129116
Purcell, S. M., Moran, J. L., Fromer, M., Ruderfer, D., Solovieff, N., Roussos, P., et al. (2014). A polygenic burden of rare disruptive mutations in schizophrenia. Nature 506, 185–190. doi: 10.1038/nature12975
Raichle, M. E. (2015). The brain’s default mode network. Annu. Rev. Neurosci. 38, 433–447. doi: 10.1146/annurev-neuro-071013-014030
Ramsay, I. S. (2019). An activation likelihood estimate meta-analysis of thalamocortical dysconnectivity in psychosis. Biol. Psychiatry Cogn. Neurosci. Neuro. 4, 859–869. doi: 10.1016/j.bpsc.2019.04.007
Rees, E., O’Donovan, M. C., and Owen, M. J. (2015). Genetics of schizophrenia. Curr. Opin. Behav. Sci. 2, 8–14. doi: 10.1016/j.cobeha.2014.07.001
Rehm, J., and Shield, K. D. (2019). Global burden of disease and the impact of mental and addictive disorders. Curr. Psychiatry Rep. 21:10. doi: 10.1007/s11920-019-0997-0
Reid, R. C., and Alonso, J. M. (1995). Specificity of monosynaptic connections from thalamus to visual cortex. Nature 378, 281–284. doi: 10.1038/378281a0
Richards, B. A., Lillicrap, T. P., Beaudoin, P., Bengio, Y., Bogacz, R., and Christensen, A. (2019). A deep learning framework for neuroscience. Nat. Neurosci. 22, 1761–1770. doi: 10.1038/s41593-019-0520-2
Rikhye, R. V., Gilra, A., and Halassa, M. M. (2018a). Thalamic regulation of switching between cortical representations enables cognitive flexibility. Nat. Neurosci. 21, 1753–1763. doi: 10.1038/s41593-018-0269-z
Rikhye, R. V., Wimmer, R. D., and Halassa, M. M. (2018b). Toward an integrative theory of thalamic function. Annu. Rev. Neurosci. 41, 163–183. doi: 10.1146/annurev-neuro-080317-062144
Ripke, S., O’Dushlaine, C., Chambert, K., Moran, J. L., Kähler, A. K., Akterin, S., et al. (2013). Genome-wide association analysis identifies 13 new risk loci for schizophrenia. Nat. Genet. 45, 1150–1159. doi: 10.1038/ng.2742
Roy, A. K., Shehzad, Z., Margulies, D. S., Kelly, A. M. C., Uddin, L. Q., Gotimer, K., et al. (2009). Functional connectivity of the human amygdala using resting state fMRI. Neuroimage 45, 614–626. doi: 10.1016/j.neuroimage.2008.11.030
Ryvlin, P., Rheims, S., Hirsch, L. J., Sokolov, A., and Jehi, L. (2021). Neuromodulation in epilepsy: state-of-the-art approved therapies. Lancet Neurol. 20, 1038–1047. doi: 10.1016/S1474-4422(21)00300-8
Saalmann, Y. B., Pinsk, M. A., Wang, L., Li, X., and Kastner, S. (2012). The pulvinar regulates information transmission between cortical areas based on attention demands. Science 337, 753–756. doi: 10.1126/science.1223082
Sabaroedin, K., Razi, A., Chopra, S., Tran, N., Pozaruk, A., Chen, Z., et al. (2022). Frontostriatothalamic effective connectivity and dopaminergic function in the psychosis continuum. Brain 2022:18. doi: 10.1093/brain/awac018
Sarafyazd, M., and Jazayeri, M. (2019). Hierarchical reasoning by neural circuits in the frontal cortex. Science 364:8911. doi: 10.1126/science.aav8911
Schiff, N. D., Giacino, J. T., Kalmar, K., Victor, J. D., Baker, K., Gerber, M., et al. (2007). Behavioural improvements with thalamic stimulation after severe traumatic brain injury. Nature 448, 600–603. doi: 10.1038/nature06041
Schizophrenia Psychiatric Genome-Wide Association Study (GWAS) Consortium. (2011). Genome-wide association study identifies five new schizophrenia loci. Nat. Genet. 43, 969–976. doi: 10.1038/ng.940
Schizophrenia Working Group of the Psychiatric Genomics Consortium. (2014). Biological insights from 108 schizophrenia-associated genetic loci. Nature 511, 421–427. doi: 10.1038/nature13595
Schleifer, C., Lin, A., Kushan, L., Ji, J. L., Yang, G., Bearden, C. E., et al. (2019). Dissociable disruptions in thalamic and hippocampal resting-state functional connectivity in youth with 22q11.2 deletions. J. Neurosci. 39, 1301–1319. doi: 10.1523/JNEUROSCI.3470-17.2018
Schmitt, L. I., Wimmer, R. D., Nakajima, M., Happ, M., Mofakham, S., and Halassa, M. M. (2017). Thalamic amplification of cortical connectivity sustains attentional control. Nature 545, 219–223. doi: 10.1038/nature22073
Schrimpf, M., Kubilius, J., Lee, M. J., Ratan Murty, N. A., Ajemian, R., and DiCarlo, J. J. (2020). Integrative benchmarking to advance neurally mechanistic models of human intelligence. Neuron 108, 413–423. doi: 10.1016/j.neuron.2020.07.040
Schultz, S. H., North, S. W., and Shields, C. G. (2007). Schizophrenia: a review. Am. Fam. Physician 75, 1821–1829.
Shafiei, G., Markello, R. D., de Wael, R., Bernhardt, B. C., Fulcher, B. D., and Misic, B. (2020). Topographic gradients of intrinsic dynamics across neocortex. Elife 9:62116. doi: 10.7554/eLife.62116
Sheffield, J. M., Huang, A. S., Rogers, B. P., Giraldo-Chica, M., Landman, B. A., Blackford, J. U., et al. (2020). Thalamocortical anatomical connectivity in schizophrenia and psychotic bipolar disorder. Schizophr. Bull. 2020:22. doi: 10.1093/schbul/sbaa022
Shepherd, G. M. G., and Yamawaki, N. (2021). Untangling the cortico-thalamo-cortical loop: cellular pieces of a knotty circuit puzzle. Nat. Rev. Neurosci. 22, 389–406. doi: 10.1038/s41583-021-00459-3
Sherman, S. M. (2017). Functioning of circuits connecting thalamus and cortex. Compr. Physiol. 7, 713–739. doi: 10.1002/cphy.c160032
Shine, J. M., Müller, E. J., Munn, B., Cabral, J., Moran, R. J., and Breakspear, M. (2021). Computational models link cellular mechanisms of neuromodulation to large-scale neural dynamics. Nat. Neurosci. 24, 765–776. doi: 10.1038/s41593-021-00824-6
Shulman, G. L., Fiez, J. A., Corbetta, M., Buckner, R. L., Miezin, F. M., Raichle, M. E., et al. (1997). Common blood flow changes across visual tasks: II. Decreases in cerebral cortex. J. Cogn. Neurosci. 9, 648–663. doi: 10.1162/jocn.1997.9.5.648
Smeland, O. B., Frei, O., Dale, A. M., and Andreassen, O. A. (2020). The polygenic architecture of schizophrenia - rethinking pathogenesis and nosology. Nat. Rev. Neurol. 16, 366–379. doi: 10.1038/s41582-020-0364-0
Soltani, A., and Koechlin, E. (2022). Computational models of adaptive behavior and prefrontal cortex. Neuropsychopharmacology 47, 58–71. doi: 10.1038/s41386-021-01123-1
Steeds, H., Carhart-Harris, R. L., and Stone, J. M. (2015). Drug models of schizophrenia. Ther Adv Psychopharmacol 5, 43–58. doi: 10.1177/2045125314557797
Sterzer, P., Adams, R. A., Fletcher, P., Frith, C., Lawrie, S. M., Muckli, L., et al. (2018). The predictive coding account of psychosis. Biol. Psychiatry 84, 634–643. doi: 10.1016/j.biopsych.2018.05.015
Steullet, P. (2020). Thalamus-related anomalies as candidate mechanism-based biomarkers for psychosis. Schizophr. Res. 226, 147–157. doi: 10.1016/j.schres.2019.05.027
Straub, R. E., Jiang, Y., MacLean, C. J., Ma, Y., Webb, B. T., Myakishev, M. V., et al. (2002). Genetic variation in the 6p22.3 gene DTNBP1, the human ortholog of the mouse dysbindin gene, is associated with schizophrenia. Am. J. Hum. Genet. 71, 337–348. doi: 10.1086/341750
Suk, H.-I., Wee, C.-Y., Lee, S.-W., and Shen, D. (2016). State-space model with deep learning for functional dynamics estimation in resting-state fMRI. Neuroimage 129, 292–307. doi: 10.1016/j.neuroimage.2016.01.005
Szewczyk-Krolikowski, K., Menke, R. A. L., Rolinski, M., Duff, E., Salimi-Khorshidi, G., Filippini, N., et al. (2014). Functional connectivity in the basal ganglia network differentiates PD patients from controls. Neurology 83, 208–214. doi: 10.1212/WNL.0000000000000592
Takata, N. (2020). Thalamic reticular nucleus in the thalamocortical loop. Neurosci. Res. 156, 32–40. doi: 10.1016/j.neures.2019.12.004
Talvik, M., Nordström, A.-L., Olsson, H., Halldin, C., and Farde, L. (2003). Decreased thalamic D2/D3 receptor binding in drug-naive patients with schizophrenia: a PET study with [11C]FLB 457. Int. J. Neuropsychopharmacol. 6, 361–370. doi: 10.1017/S1461145703003699
Tang, L., Ge, Y., Sodickson, D. K., Miles, L., Zhou, Y., Reaume, J., et al. (2011). Thalamic resting-state functional networks: disruption in patients with mild traumatic brain injury. Radiology 260, 831–840. doi: 10.1148/radiol.11110014
Taren, A. A., Gianaros, P. J., Greco, C. M., Lindsay, E. K., Fairgrieve, A., Brown, K. W., et al. (2017). Mindfulness meditation training and executive control network resting state functional connectivity: a randomized controlled trial. Psychosom. Med. 79, 674–683. doi: 10.1097/PSY.0000000000000466
Usrey, W. M., Reppas, J. B., and Reid, R. C. (1999). Specificity and strength of retinogeniculate connections. J. Neurophysiol. 82, 3527–3540. doi: 10.1152/jn.1999.82.6.3527
Wang, H.-L. S., Rau, C.-L., Li, Y.-M., Chen, Y.-P., and Yu, R. (2015). Disrupted thalamic resting-state functional networks in schizophrenia. Front. Behav. Neurosci. 9:45. doi: 10.3389/fnbeh.2015.00045
Wang, J. X., Kurth-Nelson, Z., Kumaran, D., Tirumala, D., Soyer, H., Leibo, J. Z., et al. (2018). Prefrontal cortex as a meta-reinforcement learning system. Nat. Neurosci. 21, 860–868. doi: 10.1038/s41593-018-0147-8
Wang, M. B., and Halassa, M. M. (2022). Thalamocortical contribution to flexible learning in neural systems. Network neuroscience 6, 980–997. doi: 10.1162/netn_a_00235
Wang, X.-J. (2010). Neurophysiological and computational principles of cortical rhythms in cognition. Physiol. Rev. 90, 1195–1268. doi: 10.1152/physrev.00035.2008
Welsh, R. C., Chen, A. C., and Taylor, S. F. (2008). Low-frequency BOLD fluctuations demonstrate altered thalamocortical connectivity in schizophrenia. Schizophr. Bull. 36, 713–722. doi: 10.1093/schbul/sbn145
Wilkinson, S. (2014). Accounting for the phenomenology and varieties of auditory verbal hallucination within a predictive processing framework. Conscious. Cogn. 30, 142–155. doi: 10.1016/j.concog.2014.09.002
Wimmer, R. D., Schmitt, L. I., Davidson, T. J., Nakajima, M., Deisseroth, K., and Halassa, M. M. (2015). Thalamic control of sensory selection in divided attention. Nature 526, 705–709. doi: 10.1038/nature15398
Woodward, N. D., and Heckers, S. (2016). Mapping thalamocortical functional connectivity in chronic and early stages of psychotic disorders. Biol. Psychiatry 79, 1016–1025. doi: 10.1016/j.biopsych.2015.06.026
Woodward, N. D., Karbasforoushan, H., and Heckers, S. (2012). Thalamocortical dysconnectivity in schizophrenia. Am. J. Psychiatry 169, 1092–1099. doi: 10.1176/appi.ajp.2012.12010056
Wu, G., Palaniyappan, L., Zhang, M., Yang, J., Xi, C., Liu, Z., et al. (2022). Imbalance between prefronto-thalamic and sensorimotor-thalamic circuitries associated with working memory deficit in schizophrenia. Schizophr. Bull. 48, 251–261. doi: 10.1093/schbul/sbab086
Yang, H., Long, X.-Y., Yang, Y., Yan, H., Zhu, C.-Z., Zhou, X.-P., et al. (2007). Amplitude of low frequency fluctuation within visual areas revealed by resting-state functional MRI. Neuroimage 36, 144–152. doi: 10.1016/j.neuroimage.2007.01.054
Yasuno, F., Suhara, T., Okubo, Y., Sudo, Y., Inoue, M., Ichimiya, T., et al. (2004). Low dopamine D2 receptor binding in subregions of the thalamus in schizophrenia. AJP 161, 1016–1022. doi: 10.1176/appi.ajp.161.6.1016
Keywords: thalamus, psychosis, cognition, functional connectivity, pharmacology, mediodorsal nucleus, computation
Citation: Anticevic A and Halassa MM (2023) The thalamus in psychosis spectrum disorder. Front. Neurosci. 17:1163600. doi: 10.3389/fnins.2023.1163600
Received: 11 February 2023; Accepted: 21 March 2023;
Published: 13 April 2023.
Edited by:
Lucy Maree Palmer, University of Melbourne, AustraliaReviewed by:
Miguel Ángel Sánchez-González, Autonomous University of Madrid, SpainKelvin O. Lim, University of Minnesota, United States
Copyright © 2023 Anticevic and Halassa. This is an open-access article distributed under the terms of the Creative Commons Attribution License (CC BY). The use, distribution or reproduction in other forums is permitted, provided the original author(s) and the copyright owner(s) are credited and that the original publication in this journal is cited, in accordance with accepted academic practice. No use, distribution or reproduction is permitted which does not comply with these terms.
*Correspondence: Alan Anticevic, YWxhbi5hbnRpY2V2aWNAeWFsZS5lZHU=; Michael M. Halassa, bWljaGFlbC5oYWxhc3NhQHR1ZnRzLmVkdQ==