- Department of Psychology, School of Social Sciences, University of Mannheim, Mannheim, Germany
Introduction: Consumers’ emotional responses are the prime target for marketing commercials. Facial expressions provide information about a person’s emotional state and technological advances have enabled machines to automatically decode them.
Method: With automatic facial coding we investigated the relationships between facial movements (i.e., action unit activity) and self-report of commercials advertisement emotion, advertisement and brand effects. Therefore, we recorded and analyzed the facial responses of 219 participants while they watched a broad array of video commercials.
Results: Facial expressions significantly predicted self-report of emotion as well as advertisement and brand effects. Interestingly, facial expressions had incremental value beyond self-report of emotion in the prediction of advertisement and brand effects. Hence, automatic facial coding appears to be useful as a non-verbal quantification of advertisement effects beyond self-report.
Discussion: This is the first study to measure a broad spectrum of automatically scored facial responses to video commercials. Automatic facial coding is a promising non-invasive and non-verbal method to measure emotional responses in marketing.
Introduction
Consumer neuroscience promises a better understanding of consumers’ emotions and attitudes with objective measures. Emotions play a central role in attitude formation (Ito and Cacioppo, 2001), information processing (Lemerise and Arsenio, 2000; Fraser et al., 2012), and decision-making in general (Slovic et al., 2007; Pittig et al., 2014). Hence, a direct measurement of emotional responses with advanced technologies might be key to understanding consumers’ behavior and decisions (Ariely and Berns, 2010; Solnais et al., 2013). In particular, emotions play a central role in marketing communications, such as video commercials to elicit desired advertisement and brand effects (Achar et al., 2016). Correspondingly, advertisements influence customers’ perception of brands which potentially moderates their purchase decisions and behaviors (Plassmann et al., 2012). Furthermore, consumers’ expectancies about a particular brand have a strong psychological impact because they can modulate consumption experience (McClure et al., 2004; Shiv et al., 2005). Neural activation patterns elicited by video commercials were previously investigated to either predict advertisement effectiveness beyond self-report with fMRI (Berns and Moore, 2012; Falk et al., 2012) and electroencephalogram (EEG) (Dmochowski et al., 2014; Boksem and Smidts, 2015) or to display latent emotional processes on a continues basis (Ohme et al., 2010; Vecchiato et al., 2011). However, such measures either require obtrusive research settings (fMRI tubes) or sensors attached to the scalp (EEG) and are, thus, not entirely non-invasive.
Facial expression and emotion
Besides brain activity, emotional experiences also induce affective expressions (Sander et al., 2018; Scherer and Moors, 2019). Facial expression is the most investigated and predictive aspect of emotional expressions (Scherer and Ellgring, 2007; Plusquellec and Denault, 2018). In comparison to measures of brain activity, facial expressions responses are typically video-based, which requires no measurement preparation or application, can be obtained in ecologically valid environments, and is even applicable to online research. In order to capture emotionally relevant information from the whole face, researchers have heavily relied on observation techniques such as the Facial Action Coding System (FACS) to score intensity estimates of single facial movements called Action Units (AU) (Ekman et al., 2002; Mauss and Robinson, 2009). FACS is an extensive coding manual which allows for a very detailed description of facial responses through the combination of AU and shows good to excellent inter-rater reliabilities (Sayette et al., 2001). However, human FACS coding results in low scaling resolution of AU intensities and it is very time consuming (Schulte-Mecklenbeck et al., 2017).
Although there are several important theories that explain specific aspects of emotional facial expressions [for an overview, see Barrett et al. (2019)], there are currently only two relevant empirical approaches that map combinations of specific AUs to specific emotional states. On the one side, basic emotion theory predicts robust AU patterns that cohere with a limited amount of distinct emotion categories (i.e., joy, anger, disgust, sadness, fear, and surprise; e.g., Ekman et al., 1987). However, there is evidence for universal facial expressions beyond six basic emotions (Cordaro et al., 2018, 2020; Keltner et al., 2019; Cowen et al., 2021). Moreover, there are spontaneous emotional responses that are be much more variable and less universal for a distinct AU pattern (Durán and Fernández-Dols, 2021; Le Mau et al., 2021; Tcherkassof and Dupré, 2021). On the other side, componential process theory predicts several appraisal dimensions that elicit specific AU combinations like valence, novelty, and control (Sander et al., 2018; Scherer et al., 2018, 2021).
Although electromyography (EMG) research extensively measured corrugator and zygomaticus activity to approximate a valence dimension (e.g., Höfling et al., 2020), the investigation of other components in this theory is still preliminary. Hence, there is currently no consensus about the link between meaningful AU combinations regardless of the assumption of dimensional or categorial underlying emotional processes.
Validity of AFC
Recent advances in technology have enabled the measurement of facial expressions to obtain emotion-associated parameters through automatic facial coding based on machine-learning (AFC; Pantic and Rothkrantz, 2003; Cohn and Sayette, 2010). AFC parameters include separate facial movements, such as Action Units derived from the Facial Action Coding System on the one side and integrated “emotion-scores” such as joy or anger estimations, on the other side. There is evidence that AU parameters estimated by AFC correspond with estimates of human FACS raters between 71 and 93%, depending on the specific measurement system (Bartlett et al., 1999; Tian et al., 2001; Skiendziel et al., 2019; Seuss et al., 2021). Moreover, AFC classifies basic emotional facial expressions with impressive accuracy in prototypical facial expressions in pictures (Lewinski et al., 2014a; Lewinski, 2015; Beringer et al., 2019; Küntzler et al., 2021) as well as videos (Mavadati et al., 2013; Yitzhak et al., 2017; Calvo et al., 2018; Krumhuber et al., 2021).
While attempts to validate this innovative technology mainly focused on highly standardized and prototypical emotional facial expressions in the past, the number of validation studies that approximate more naturalistic or spontaneous facial expressions is still preliminary. AFC is less accurate in the detection of less intense and more naturalistic expressions (Büdenbender et al., 2023), which is also a commonly observed pattern in human emotion recognition (Krumhuber et al., 2021). Some studies find evidence that AFC can be transferred to the facial expressions of naïve participants that mimic emotional facial expressions in a typical laboratory setting (Stöckli et al., 2018; Sato et al., 2019; Höfling et al., 2022). To a limited extent, AFC detects highly unstandardized emotional facial expressions of professional actors depicted in movies (Küntzler et al., 2021). Furthermore, AFC can also track spontaneous emotional responses toward pleasant scenes, where AFC parameters correlate with emotional self-report and direct measures of muscle activity with EMG (Höfling et al., 2020). However, AFC is not sensitive to very subtle emotional responses, particularly if participants are motivated to suppress or control their facial responses (Höfling et al., 2021). Taken together, there is evidence that AFC validly captures spontaneous emotional states in a typical laboratory setting, especially for pleasant emotional responses.
AFC and advertisement
According to the affect-transfer hypothesis (Brown and Stayman, 1992), there is evidence for a relationship between consumers’ emotional responses to advertisement stimuli and the subsequently elicited advertisement and brand effects. In this model, an advertisement elicits emotional responses which influence the attitude toward the advertisement (i.e., advertisement likeability). In the following step, a favorable ad likeability leads to changes in the attitude toward the brand (brand likeability), which increases the purchase intention of products and services of a particular brand in the final step of this process model.
AFC has been used successfully to predict the effects of video commercials’ on the subsequent processes of this advertisement and brand effect framework, such as the self-reported emotional response, advertisement likeability, as well as brand likeability and purchase intention. In the domain of political influencing, AFC measures have been shown to correspond with emotional self-report of pleasant (Mahieu et al., 2019) as well as unpleasant advertisements (Fridkin et al., 2021). They are also predictive to measure intended emotional responses in an a priori defined target audience (Otamendi and Sutil Martín, 2020). Accordingly, AFC of smiling intensity correlates with advertisement likeability (Lewinski et al., 2014b; McDuff et al., 2014, 2015), brand likeability (Lewinski et al., 2014b), and the purchase intentions of advertised brands (Teixeira et al., 2014; McDuff et al., 2015). Furthermore, increased smiling was also found to reduce zapping behavior (Yang et al., 2014; Chen et al., 2016), decrease attention dispersion (Teixeira et al., 2012), and predict long-term attitude changes (Hamelin et al., 2017). Taken together, there is evidence that AFC of smiling predicts several steps of the advertisement and brand effects proposed by the affect-transfer hypothesis. In contrast to AFC, approaches to measure advertisement effects with human FACS were less successful (e.g., Derbaix, 1995).
Research gaps and overview
The scientific knowledge regarding a direct link between emotional facial expression on the one side and advertisement or brand effects on the other side is very limited due to the following reasons: First, it is unclear whether facial expressions significantly predict advertisement and brand effects beyond relevant precursor self-report dimensions. Such quantification is only possible if statistical models of facial expression parameters are compared with models that control for relevant self-report, which has not been investigated in previous research. Second, relevant research mainly used integrated parameters for joy or entertainment, relying almost exclusively on measurements of smiling (AU12). Reporting such integrated scores serves a lower level of scientific transparency in comparison to a description with AU terminology because it is largely unknown how AFC classifiers are trained and, correspondingly, how integrated parameters are estimated. As an additional consequence, it is unclear how different AUs that are relevant for emotional facial expressions ensembles to predict advertisement and brand effects of video commercials’ effectiveness beyond smiling. Third, there is no consensus on whether the degree of amusement or entertainment in video commercials shows a linear or non-linear relationship on branding effects: While Lewinski et al. (2014b) report a weak linear relationship between AFC of smiling and brand effects, Teixeira et al. (2014) report an inverted u-shaped relationship between AFC of smiling and brand effects. Finally, there is no study available that investigates the relationship between emotional facial expressions and all relevant steps of advertisement and brand effects according to the affect-transfer hypothesis in a within-subject study design.
In order to close these existing research gaps, we investigated the predictive value of facial expressions while viewing video commercials to forecast all subsequent components of the affect-transfer model of advertisement and brand effects: emotion ratings, ad likeability, changes between pre- and post-measurements of brand likeability, and purchase intention. To this end, we broaden the spectrum of analyzed movements in the face to 20 AU that can be measured with a state-of-the-art AFC algorithm to predict relevant outcome criteria (see Figure 1 for an overview of measured AUs and self-report ratings). In addition, we aim to determine non-linearities between facial expressions and self-report with semi-parametric additive mixed models that can account for non-linear effects (Wood, 2006; Wood et al., 2013; Wood and Scheipl, 2020). We expect strong relationships between self-reported emotion ratings and advertisement likeability and changes in brand likeability as well as purchase intention. In order to collect data from a variety of industries and emotional content, we established different stimulus groups which only differed in terms of advertisement videos and corresponding brand stimuli. This is the first study that investigates the relationship between emotional facial expressions measured by artificial intelligence and all relevant components of advertisement and brand effectiveness proposed by the affect-transfer framework.
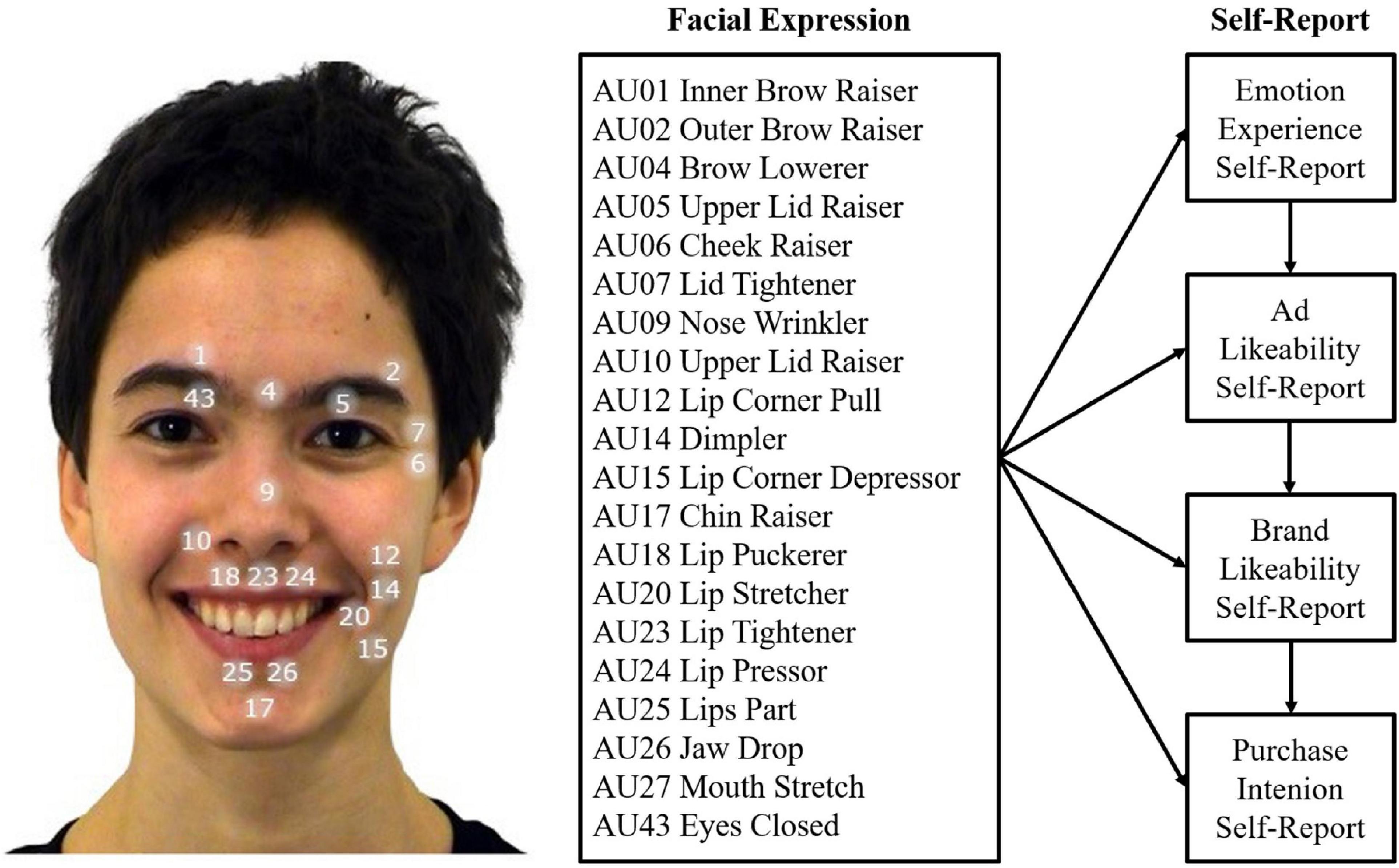
Figure 1. Overview of the measured Action Units and hypothesized relations to self-report ratings. Depicted is a happy facial expression from the ADFES inventory (model F04; van der Schalk et al., 2011).
Materials and methods
Participants
A total of 257 volunteers were randomly assigned to one of eight stimulus groups. General exclusion criteria were age under 18, acute psychoactive medication use, acute mental disorder episode, severe somatic disease, and wearing glasses. Participants with corrected-to-normal vision were asked to wear contact lenses during the experiment. After visual inspection of the analyzed videos, data from 38 participants were excluded because of face detection problems of the AFC software (e.g., partially covered face with the hand, scarfs, and other accessories or large body movements that lead to false face detection). Hence, we only used data from participants with good recording and analysis quality, resulting in an overall sample of 219 (115 females) participants of mainly Caucasian descent. Age ranged from 19 to 58 years (M = 23.79, SD = 5.22). All participants received compensation of either 8€ or student course credit, and they signed informed consent before the data collection. University Research Ethics Committee approved the experiment.
Questionnaires
Participants filled in various questionnaires to compare relevant states and traits related to emotional responsiveness and expressivity to ensure comparability between experimental groups. After a socio-demographic questionnaire (e.g., gender, age, and educational level), the Social Interaction Anxiety Scale (SIAS; Stangier et al., 1999), the State-Trait Anxiety Inventory (STAI State and STAI Trait; Laux et al., 1981), the Positive-and-Negative-Affect-Schedule (PANAS PA and PANAS NA; Krohne et al., 1996), the Self-Rating Depression Scale (SDS; Zung, 1965), the Berkley Expressivity Questionnaire (BEQ; Mohiyeddini et al., 2008), and the Behavioral Inhibition and Activation Scale (BIS and BAS; Strobel et al., 2001) were administered before starting the main experiment.
Study design and procedure
Following informed consent and completion of the questionnaires, participants were seated in front of a computer screen. In order to collect data from a variety of industries and emotional content, we established eight different stimulus groups to which participants were randomly assigned. These groups only differed in terms of advertisement videos and corresponding brand stimuli; all other aspects remained constant between groups. Before the main experiment started, participants were instructed to maintain a neutral facial expression for 10 s, which was later utilized to calibrate the AFC analysis individually. The main experiment comprised three experimental blocks: pre-evaluation of brands, viewing video advertisements and evaluation, and post-evaluation of brands.
The pre- and post-evaluations of brands were set up identically, and hence, effects on brands elicited by the video advertisement can be traced by pre-post rating changes. The logo of the eight advertised brands was also presented in both brand evaluation blocks, each started with a 1 s fixation cross. The presentation duration of the brand logos lasted until participants decided when to proceed with several ratings of a specific brand by pressing the space bar. All scales were presented as a nine-point semantic differential. Participants rated their familiarity with the brand (1 = familiar and 9 = unfamiliar), brand likeability (1 = like and 9 = dislike; 1 = good and 9 = bad), and brand purchase intention (1 = probable purchase and 9 = unprobeable purchase; 1 = purchasing definitely and 9 = purchasing definitely not) after the brand presentation. All advertisements were indicated by a 1 s fixation cross in the advertisement block. After watching a particular video, each video was rated on nine-point semantic differentials containing advertisement familiarity (1 = familiar and 9 = unfamiliar) and advertisement likeability (1 = like and 9 = dislike; 1 = good and 9 = bad). In addition, participants rated how they felt during each video presentation on several one-item emotion scales (i.e., joy, sadness, anger, disgust, fear, surprise; 1 = strong emotion and 9 = no emotion) immediately after the video presentation. All scales were inverted to improve the readability of the results. If two items were used to measure a construct (i.e., advertisement likeability, brand likeability, brand purchase intention), the average of both items would have been calculated. Internal consistencies were excellent for these three scales (Cronbach’s α > 0.90).
Stimulus material
Each group watched eight different commercials and corresponding brand logos in randomized order. The video material was selected from the list of award-winning commercials at the Cannes Lions International Festival of Creativity between 2016 and 2017. The videos were counterbalanced between groups in terms of video duration, familiarity with the brand, familiarity with the video, and emotional content (see also Section “Results”). Appendix 1 contains all commercial video advertisements sorted by groups. Two other non-commercial video advertisements per group were presented but not included in the analysis because the brand ratings did not apply to the non-commercial section.
Apparatus and measurements
High-precision software (E-Prime; Version 2.0.10; Psychology Software Tools, Pittsburgh, PA, USA) was used to run the experiment. Stimuli were shown centrally on a 19-inch monitor with a resolution of 1,024 × 768, approximately 70 cm away from the participant. Optimal illumination with diffused frontal light was maintained throughout. Videos of participants’ faces were recorded with a Logitech HD C615 web camera placed above the computer screen. Videos were processed off-line with FaceReader Software (FR; Version 7.0, Noldus Information Technology) and further analyzed with Observer XT (Version 12.5, Noldus Information Technology). Furthermore, Observer XT synchronized stimulus onset trigger from E-Prime and the video recordings. FR is a visual pattern classifier based on deep learning approaches and extracts visual features from videos frame by frame. In accordance with neuro-computational models of human face processing (Dailey et al., 2002; Calder and Young, 2005), FR detects facial configurations in the following steps (Van Kuilenburg et al., 2005, 2008): (1) The Cascade classifier algorithm finds the position of the face (Viola and Jones, 2004). (2) Face textures are normalized and the active appearance model synthesizes a digital face model representing facial structure with over 500 location points. (3) Compressed distance information is then transmitted to an artificial neural network. (4) Finally, the artificial neural network connects these scores with relevant emotional labels through supervised training with over 10,000 samples (pictures) of emotional faces to classify the relative intensity of a given facial configuration. As a result, FR estimates activity of 20 AU, which includes AU01 Inner Brow Raiser, AU02 Outer Brow Raiser, AU04 Brow Lowerer, AU05 Upper Lid Raiser, AU06 Cheek Raiser, AU07 Lid Tightener, AU09 Nose Wrinkler, AU10 Upper Lid Raiser, AU12 Lip Corner Pull, AU14 Dimpler, AU15 Lip Corner Depressor, AU17 Chin Raiser, AU18 Lip Puckerer, AU20 Lip Stretcher, AU23 Lip Tightener, AU24 Lip Pressor, AU25 Lips Part, AU26 Jaw Drop, AU27 Mouth Stretch, and AU43 Eyes Closed. The estimated parameter of each AU ranges from 0 to 1. FR measures were calibrated per participant based on the baseline measurement at the beginning of the experiment. “East-Asian” or “elderly” face models were presented instead of the general face model as recommended by the user manual. For the duration of each video, the mean and peak activity for all AUs were exported and analyzed.
Data reduction and analysis
Across all participants (N = 219) and commercial video stimuli (n = 64; eight per participant), 1,752 data points were collected on advertisements and corresponding brand ratings. Our data analysis required several steps: analysis of participant characteristics, aggregation of video- and brand-wise means for analysis of stimulus characteristics, and also to report correlations between facial expression parameters and relevant self-report ratings, as well as semi-parametric mixed additive regression models to predict emotion, advertisement and brand effects based on facial expression parameters.
First, we calculated ANOVA with the factor stimulus group (eight groups) to determine differences in participant characteristics separately for STAI State, STAI Trait, SIAS, BIS, BAS, PANAS PA, PANAS NA, BEQ, and SDS. In addition, we report differences in gender distribution in stimulus groups with the Chi-Squared test.
Second, we calculated pre-post difference scores for brand likeability and brand purchase intention and averaged relevant self-report ratings and AU parameters separately for each video and the corresponding brand. On the one side, we determined differences in stimulus characteristics based on stimulus-wise averages for the stimulus groups with ANOVA for video duration, brand familiarity (pre-rating), video familiarity, and emotion ratings (i.e., joy, sadness, anger, fear, disgust, and surprise). We applied a Greenhouse–Geisser correction for ANOVA when appropriate. Eta-squared (η2) is reported as an effect size for F-tests (η2 ≥ 0.01 small; η2 ≥ 0.06 medium; η2 ≥ 0.14 large; Pierce et al., 2004).
Third, we identified AU that showed zero or near-zero variance to exclude AU that will not contribute to the prediction of relevant outcomes. This exclusion criterion applied for eight AU, in particular, AU01 Inner Brow Raiser, AU02 Outer Brow Raiser, AU09 Nose Wrinkler, AU10 Upper Lid Raiser, AU18 Lip Puckerer, AU20 Lip Stretcher, AU26 Jaw Drop, and AU27 Mouth Stretch, which were excluded from further analysis. Further, we report spearman’s rho correlations between relevant AU and self-report based on averages for each video and the corresponding brand. Effect sizes were interpreted following Cohen (1988): r ≥ 0.1 small, d ≥ 0.3 medium, and d ≥ 0.5 large.
Fourth, all self-report ratings were z-transformed on the group level for better interpretability and comparability of the effects. To resolve the limitation of the one-item scale of emotion ratings for joy, z-scores are calculated based on individual participant ratings. In contrast to self-report ratings, AU variables were not transformed in any way because of their scale properties (e.g., exact zero-point) and correspondence to the intensity measurement of the Facial Action Coding System: Values from >0.00–0.16 are classified as trace (E), 0.16–0.26 as slight (D), 0.26–0.58 as pronounced (C), 0.58–0.90 as severe (B), and 0.90–1 as max intensity (A).
Fifth, as predictive models, we carried out semi-parametric additive mixed modeling with the R-package “gamm4” (Wood and Scheipl, 2020). In the first step, we developed a basic model controlling gender and stimuli as fixed factors and the participants and stimulus group as random factors. Next, we calculated main effect models for all AU separately for peak and mean activity to predict joy ratings, advertisement likeability, brand likeability change, and brand purchase intention change. Furthermore, we estimated the combined effect of AU and relevant rating scales to determine the relative predictiveness of AFC versus self-report resulting in 17 independent models. Visualization of the most substantial effect patterns is presented as smoothed effect plots with 95% confidence intervals. We report R2adj, Akaike-Information-Criterion (AIC), and Bayesian-Information-Criterion (BIC) as a goodness of fit indices (Burnham and Anderson, 2004). According to Cohen (1988), we interpreted the adjusted R2 ≥ 0.01 as small, R2 ≥ 0.09 as moderate, and R2 ≥ 0.25 as a large proportion of explained variance of each predictive model. A large change in model fit was interpreted by an absolute change of 10 for AIC and BIC. The significance level was always set to α = 0.05.
Results
Manipulation checks
Questionnaire group differences
Participants were randomly assigned to one of eight groups with different stimulus materials. Appendix 2 shows descriptive statistics of the questionnaires separately for the groups. There were no significant differences between groups regarding STAI State, STAI Trait, SIAS, BIS, BAS, PANAS PA, PANAS NA, BEQ, SDS, and gender distribution. Hence, no meaningful differences in participant characteristics between groups can be reported.
Stimulus group differences and stimulus emotion characteristics
Appendix 1 shows descriptive statistics for all advertisement videos and averaged scores of relevant brand and advertisement evaluations. Comparison of the different stimulus groups revealed no significant differences for video duration, F(7, 56) = 0.32, p = 0.944, η2 = 0.04, brand familiarity (pre-rating), F(7, 56) = 0.20, p = 0.985, η2 = 0.02, video familiarity, F(7, 56) = 1.62, p = 0.148, η2 = 0.17, joy, F(7, 56) = 1.58, p = 0.162, η2 = 0.17, sadness, F(7, 56) = 0.09, p = 0.999, η2 = 0.01, anger, F(7, 56) = 0.93, p = 0.489, η2 = 0.10, fear, F(7, 56) = 0.95, p = 0.476, η2 = 0.11, disgust, F(7, 56) = 0.91, p = 0.503, η2 = 0.10, and surprise ratings, F(7, 56) = 1.22, p = 0.299, η2 = 0.13. Hence, no meaningful differences in stimulus characteristics between groups were found.
Importantly, different videos elicited different emotions, F(5, 315) = 166.59, p < 0.001, η2 = 0.73. While participants reported generally higher amounts of joy (M = 5.42, SD = 1.43) and surprise (M = 4.33, SD = 1.01), other emotions were reported substantially less (sadness: M = 2.15, SD = 1.33; anger: M = 1.79, SD = 0.60; fear: M = 1.58, SD = 0.61; disgust: M = 1.65, SD = 0.89) and, hence, were not included in the main analysis (i.e., semiparametric models of AU activity).
Correlations between self-reports and facial expressions
Spearman correlations based on unstandardized average values per stimulus between emotion, advertisement, brand ratings, and AU intensity for mean and peak activity over the video duration are reported in Table 1. There were strong and positive correlations between facial expression measures of AU6, AU12, and AU25 for ratings of joy as well as surprise for both mean and peak AU activity. Although feelings of surprise can be elicited by pleasant to unpleasant emotional events, the advertisements presented in this study elicited the same correlational patterns of facial activity for higher surprise and higher joy ratings. Therefore, these two emotion ratings might be confounded in the present study design, probably because the videos mainly triggered pleasant emotions. Hence, we focused on joy ratings as a self-report measure of emotion elicited by video commercials in the main analysis (i.e., semiparametric models of AU activity).
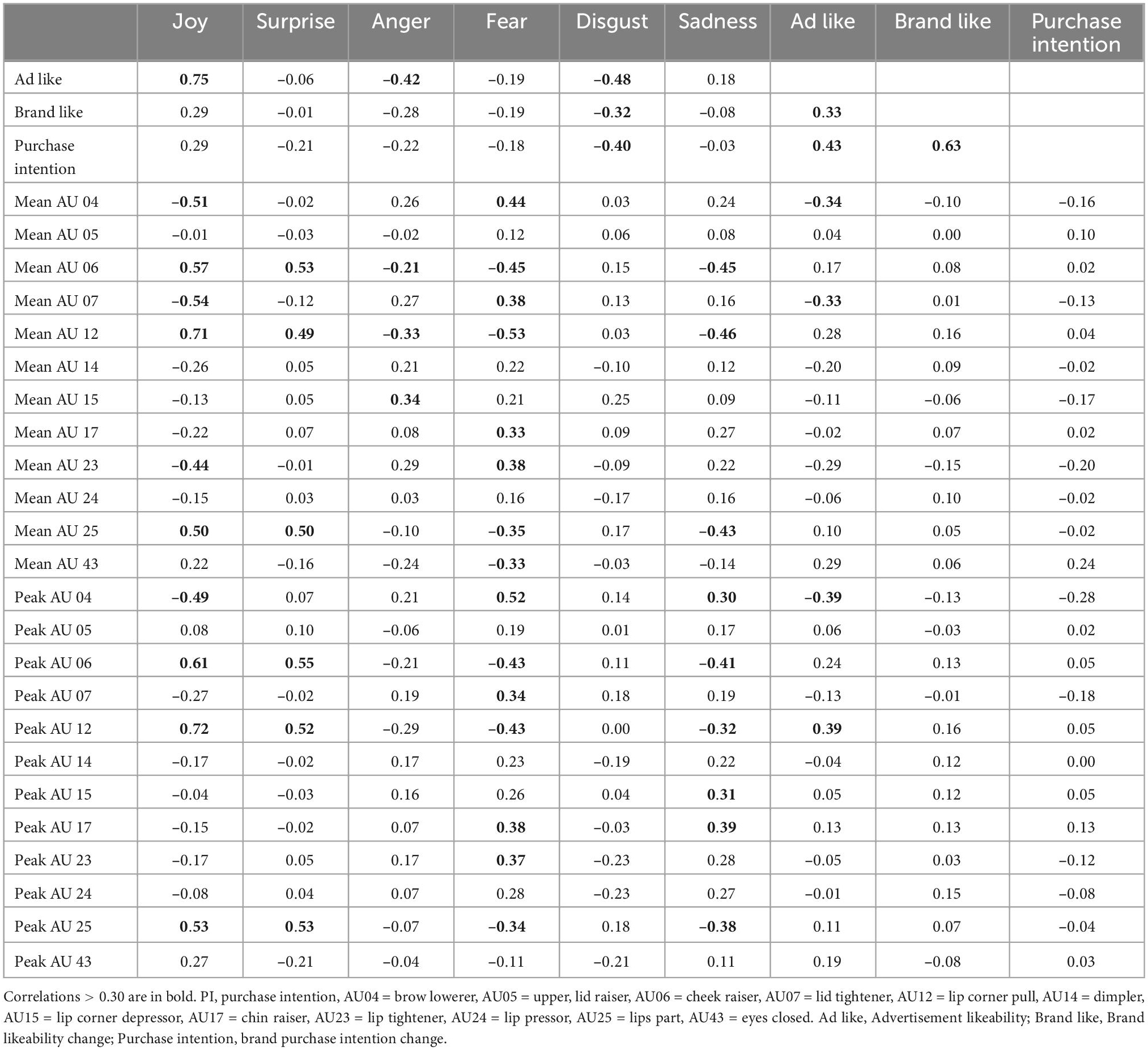
Table 1. Spearman correlations based on unstandardized average values per stimulus between self-report ratings and Action Unit (AU) activity (mean and peak).
Semiparametric models of AU activity
We fitted separate semiparametric additive mixed models to account for non-linear relationships while controlling for gender and stimuli as fixed factors and the participants and stimulus group as random factors. In the first step, we calculated regression models exclusively based on self-report ratings to test for specific relationships proposed by the affect-transfer hypothesis (see Table 2). It is evident that advertisement likeability is strongly predicted by joy ratings, brand likeability change is only significantly predicted by advertisement likeability, and purchase intention change is strongly predicted by brand likeability. This pattern strongly supports a hierarchical influence of advertisement and brand effects as postulated by the affect-transfer hypothesis.
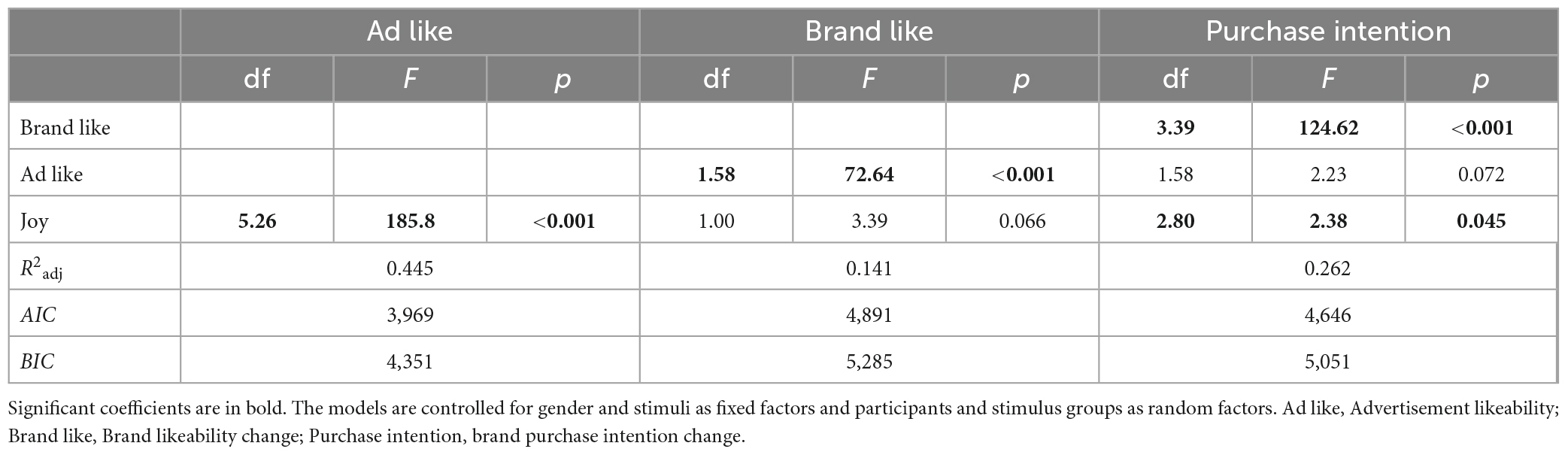
Table 2. Prediction of ad likeability, brand likeability change, and brand purchase intention change based on relevant self-report ratings.
Next, we fitted separate models to predict joy ratings (Table 3), advertisement likeability (Table 4), brand likeability change (Table 5), and purchase intention change (Table 6) based on mean and peak AU activity. In addition, we estimated the combined effect of AU and rating scales to determine the relative predictiveness of AFC versus self-report for the models that contain advertisement and brand effect self-report ratings.
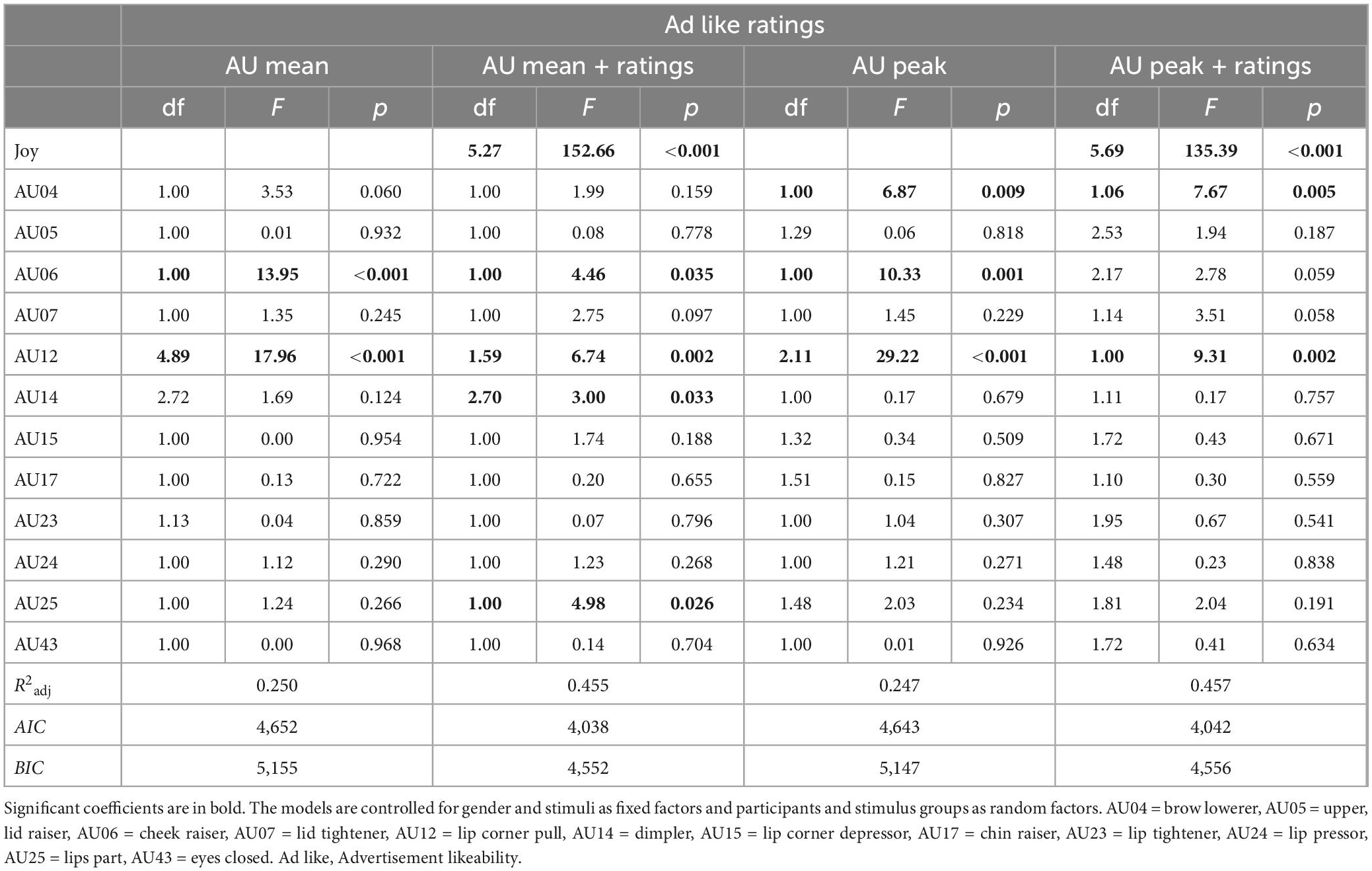
Table 4. Prediction of advertisement likeability ratings based on mean and peak Action Unit (AU) activity with and without self-report ratings.
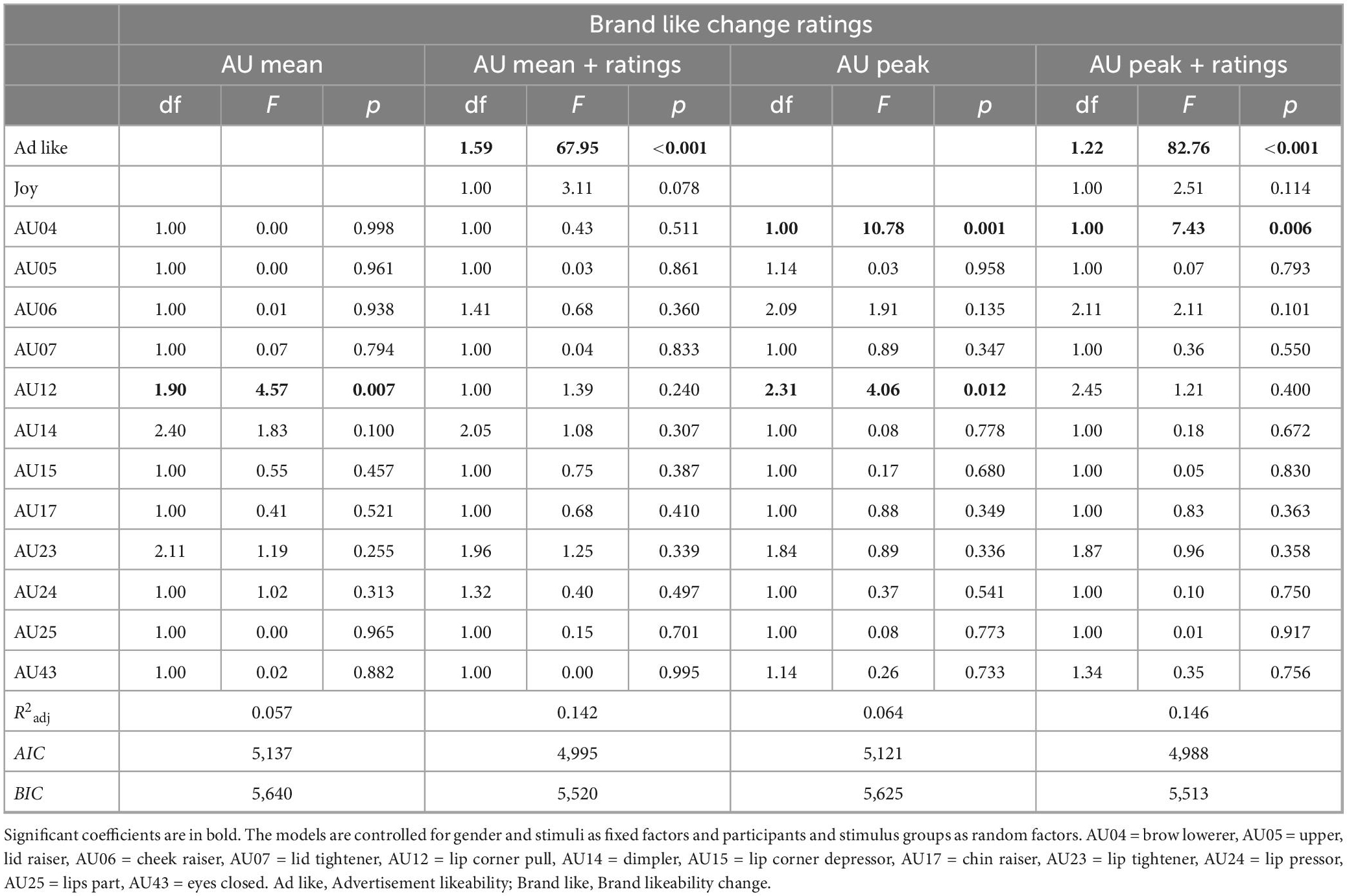
Table 5. Prediction of brand likeability change ratings based on mean and peak Action Unit (AU) activity with and without self-report ratings.
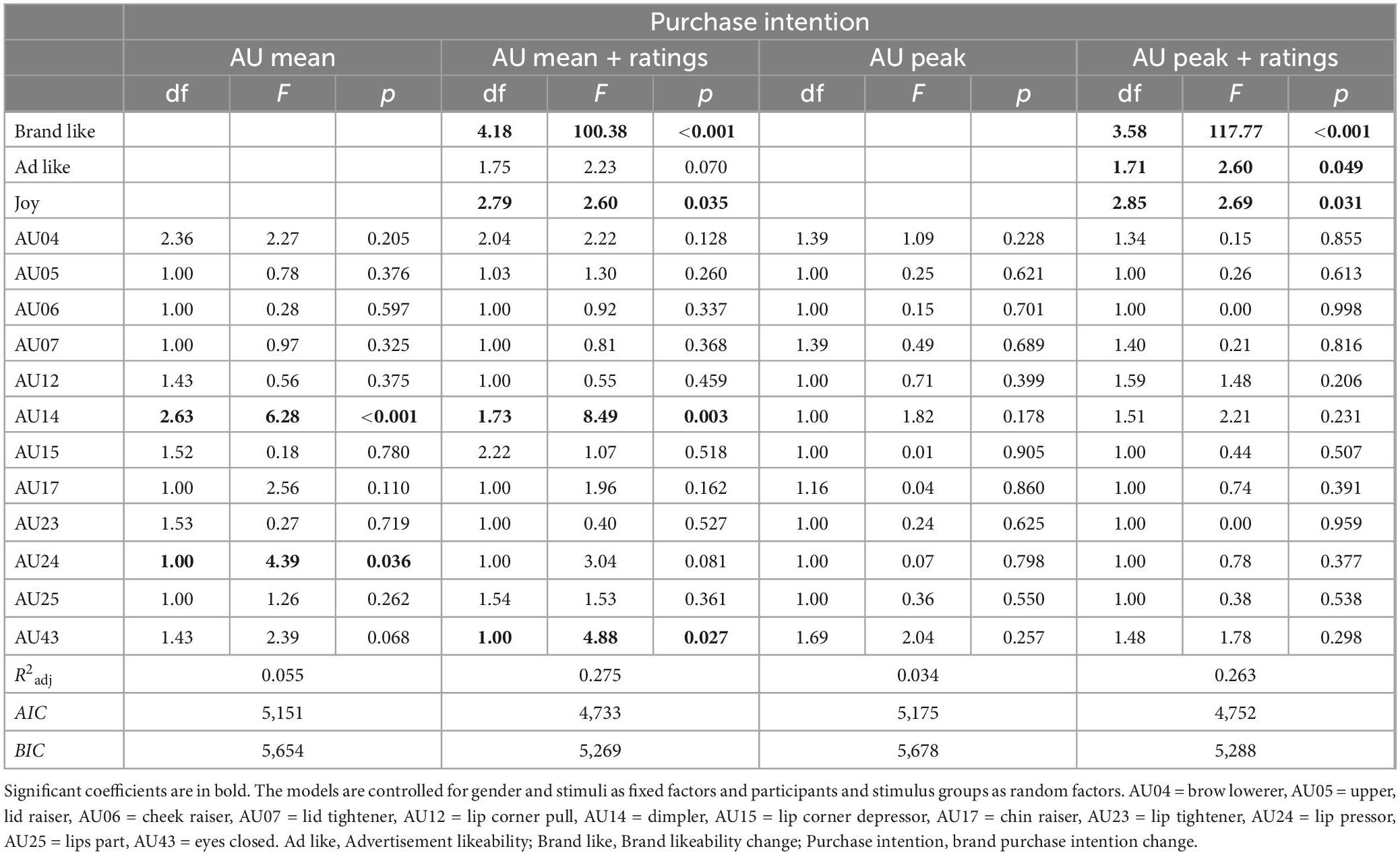
Table 6. Prediction of brand purchase intention change rating based on mean and peak Action Unit (AU) activity with and without self-report ratings.
Prediction of joy ratings
Joy ratings were significantly predicted by mean and peak activities of AU6 and AU12 (see Table 3). Mean AU12 (Figure 2, Panel 1A) showed a non-linear association with joy ratings, with the highest values for moderate AU intensities. In contrast, AU12 peak (Figure 3, Panel 1A), AU6 mean (Figure 2, Panel 2A), and AU6 peak (Figure 3, Panel 2A) activity showed a linear and strictly monotonically increasing function with regard to joy ratings.
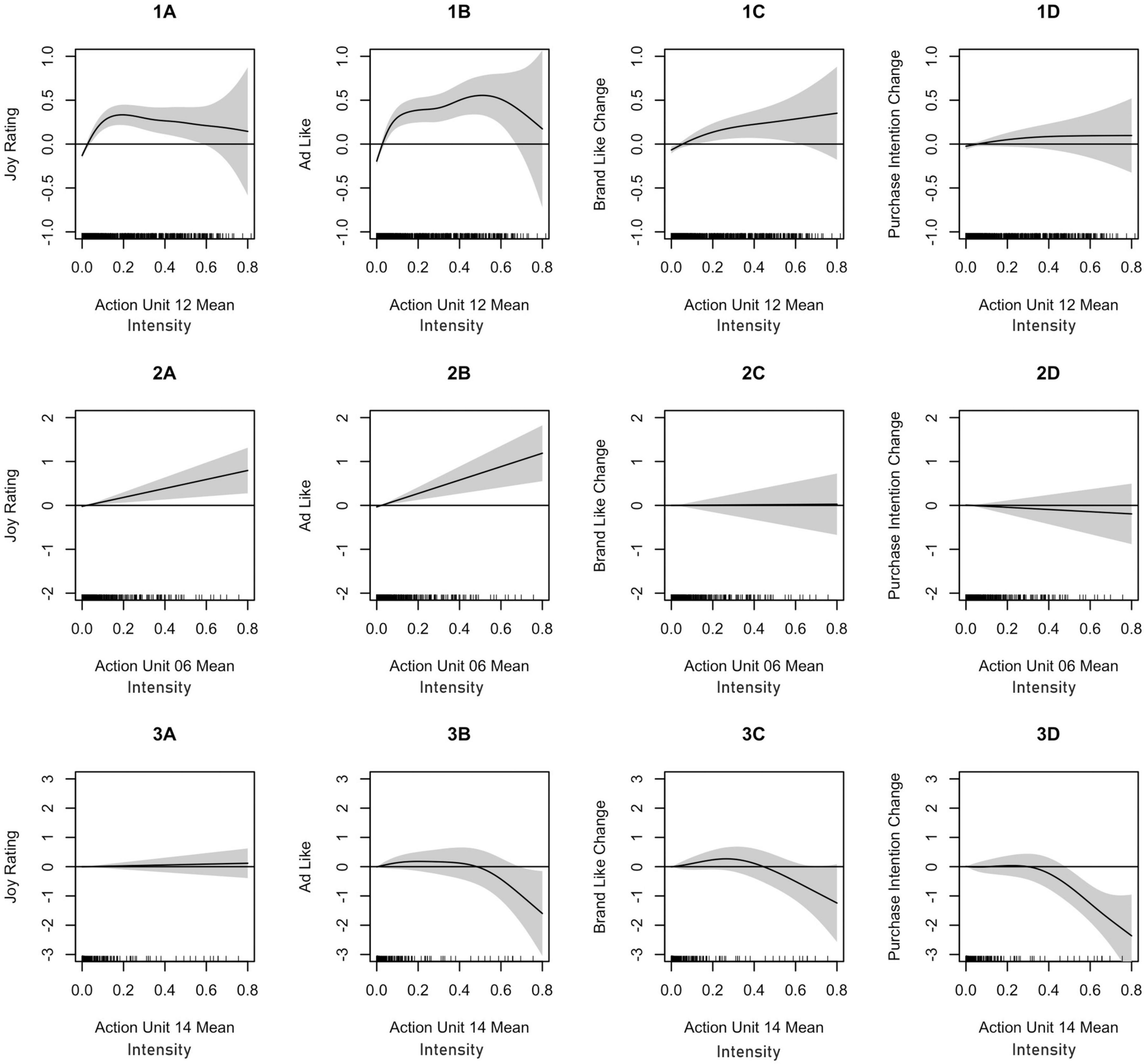
Figure 2. Fitted smooth main effects model for Action Unit means of AU12 [lip corner pull, (1A–1D)], AU06 [cheek raiser, (2A–2D)], and AU14 [dimpler, (3A–3D)]. The graphs show the estimated marginal effects on joy ratings (1A–3A), advertisement likeability (1B–3B), brand likeability change (1C–3C), and purchase intention change (1D–3D). The effects are centered around zero. The shaded areas show 95% pointwise confidence intervals.
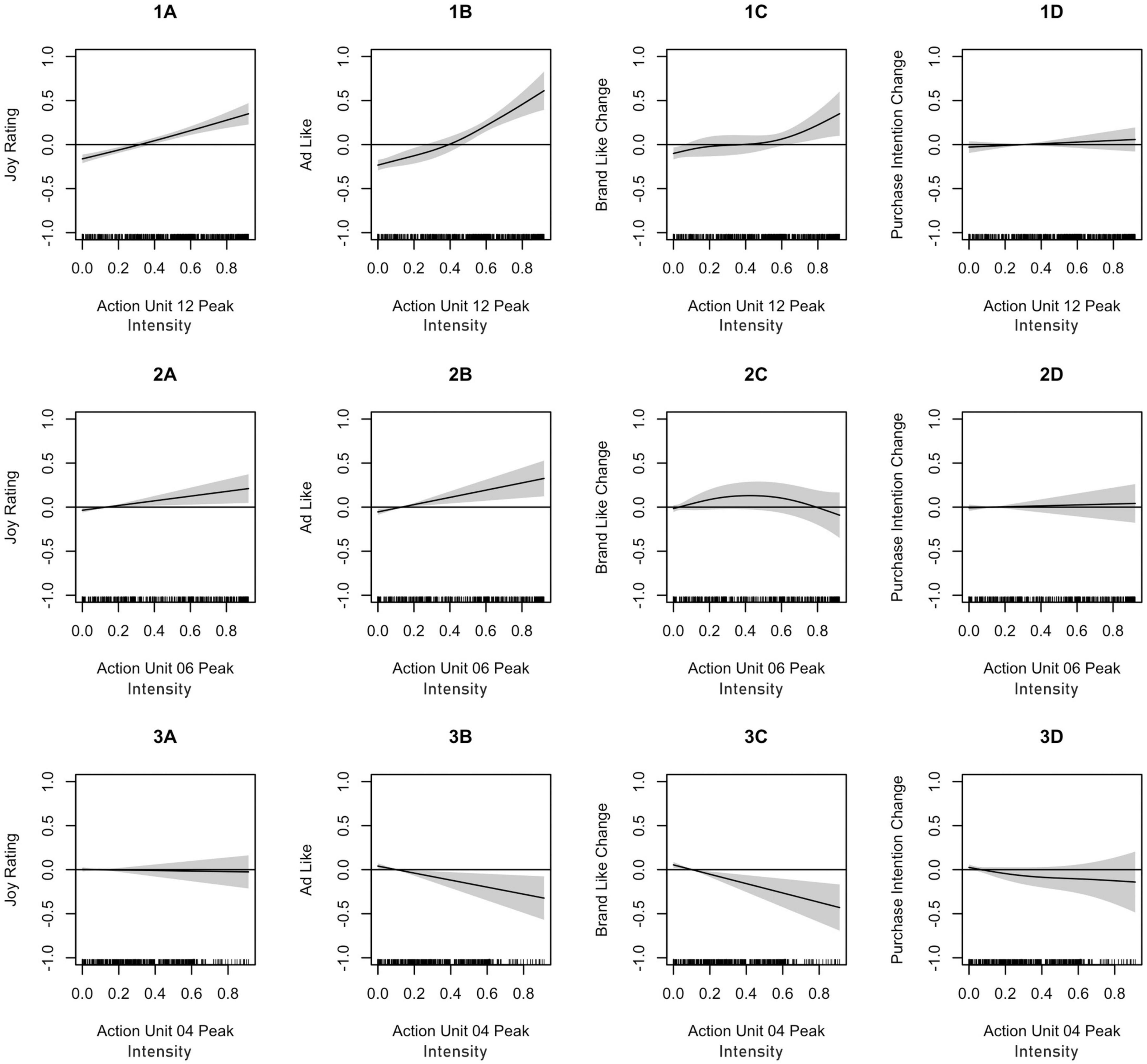
Figure 3. Fitted smooth main effects model for Action Unit peaks of AU12 [lip corner pull, (1A–1D)], AU06 [cheek raiser, (2A–2D)], and AU04 [brow raiser, (3A–3D)]. The graphs show the estimated marginal effects on joy ratings (A), advertisement likeability (B), brand likeability change (C), and purchase intention change (D). The effects are centered around zero. The shaded areas show 95% pointwise confidence intervals.
Prediction of advertisement likeability
Advertisement likeability ratings were significantly predicted by mean activity of AU6 and AU12 as well as peak activity of AU4, AU6, and AU12 (see Table 4). Mean AU12 (Figure 2, Panel 1B) showed a non-linear association with advertisement likeability ratings, with the highest values for moderate AU intensities. In contrast, AU12 peak (Figure 3, Panel 1B), AU6 mean (Figure 2, Panel 2B), AU6 peak (Figure 3, Panel 2B) showed a linear and strictly monotonically increasing function, whereas AU4 peak activity (Figure 3, Panel 3B) showed a linear and strictly monotonically decreasing function with regard to advertisement ratings. These effects remained still significant for most AUs if joy ratings were included in the models.
Prediction of brand likeability change
Brand likeability change ratings were significantly predicted by mean and peak activity of AU12 as well as peak activity of AU4 (see Table 5). AU12 mean (Figure 2, Panel 1C) and AU12 peak (Figure 3, Panel 1C) showed strictly monotonically increasing functions, whereas AU4 peak activity (Figure 3, Panel 3C) showed a strictly monotonically decreasing function with regard to brand likeability change ratings. These effects for brand likeability change ratings remained only significant for peak AU4 activity if joy and advertisement likeability ratings are included in the models.
Prediction of purchase intention change
Purchase intention change ratings were significantly predicted by mean activity of AU14 and AU 24 (see Table 6). AU14 mean (Figure 2, Panel 3D) and AU24 mean activity showed strictly monotonically decreasing functions with regard to purchase intention change ratings. These effects for purchase intention change ratings remained only significant for mean AU14 activity if joy, advertisement likeability, and brand likeability change ratings are included in the model.
Variation in model fit
Notably, there was a large variation in model fit (i.e., adjusted explained variance), which depends on the specific criterium and whether self-report rating scales are included in the model in addition to the facial expression parameters. For models including only AU parameters, we observed that the explanation of variance was strong for emotion ratings of joy, moderate to strong for advertisement likeability, and small for brand effects such as likeability and purchase intention change. If AU parameters and relevant rating scales are jointly used, we were able to improve model fit significantly and found a strong variance explanation for advertisement likeability mainly driven by joy ratings, a moderate variance explanation for brand likeability change mainly driven by advertisement likeability ratings, and a strong variance explanation for purchase intention change mainly driven by brand likeability change. Taken together, we demonstrated that AU parameters predicted relevant advertisement and brand criteria beyond self-report (see also Table 7).
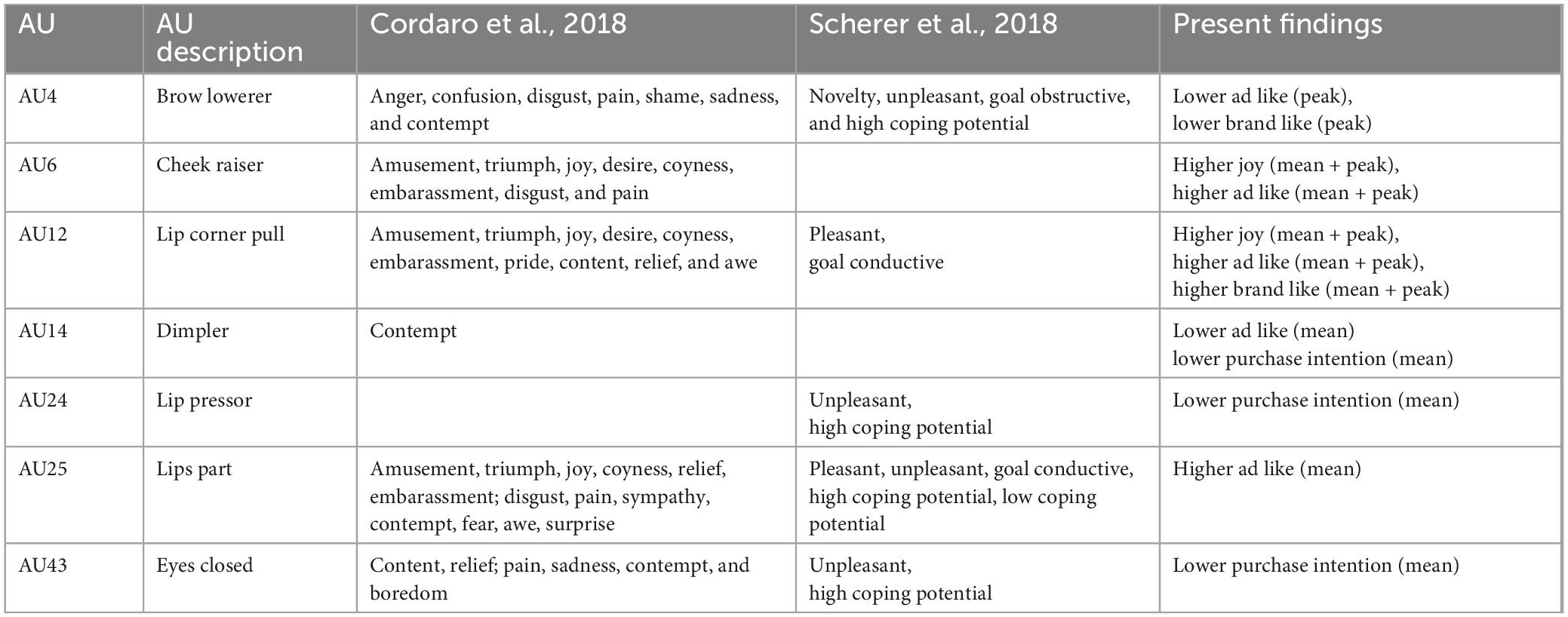
Table 7. Predictions of emotional processes according to a universalist (Cordaro et al., 2018), an appraisal-driven approach (Scherer et al., 2018), and a summary of observations in the present study for the relevant subset of Action Units (AU).
Discussion
Commercials are thought to elicit emotions, but it is difficult to quantify viewers’ emotional responses objectively and whether this has the intended impact on consumers. With novel technology, we automatically analyzed facial expressions in response to a broad array of video commercials and predicted self-reports of advertisement and brand effects. Taken together, parameters extracted by an automated facial coding technology significantly predicted all dimensions of self-report measures. Hence, automatic facial coding can contribute to a better understanding of advertisement effects in addition to self-report.
However, there was also a tremendous difference in model fit and, particularly, the strength of effects: facial expressions predicted emotion ratings with strong effects, advertisement likeability with moderate effects, changes in brand likeability, and purchase intention only with small effects. Furthermore, relations between self-report ratings strongly support a hierarchical influence of advertisement and brand effects as postulated by the affect-transfer-hypothesis: We found strong associations between joy and advertisement likeability, moderate effects between advertisement likeability and changes in brand likeability and again strong relations between brand likeability and change in purchase intention elicited by video commercials intention. Accordingly, AFC might be a valid indicator for measuring joy experience, advertisement, and brand effects, but the relevant self-report dimension still predicted the investigated criteria with more substantial effects.
Table 7 summarizes the specific effects of mean and peak AU activity on the investigated criteria. AU12 (lip corner pull) compared to other AU was a significant predictor of joy experience, ad likeability, and brand likeability change, which is in line with previous research (Lewinski et al., 2014b; McDuff et al., 2014, 2015). In contrast to the previous report (Teixeira et al., 2014; McDuff et al., 2015), we observed no significant relationship between AU12 and purchase intention. However, we found facial activity related to unpleasant emotional states to predict purchase intention, such as AU14 (Dimpler), AU24 (Lip Pressor), and AU43 (Eyes Closed). Such contradicting results may be explained by different operationalization of the purchase intention across studies: While previous literature measured purchase intention only per post-advertisement measures (Lewinski et al., 2014b; McDuff et al., 2014, 2015; Teixeira et al., 2014), in the present study this construct was measured by pre to post changes. This is preferable because post-advertisement brand ratings are highly confounded with the selected brand stimuli and cannot validly measure the effects of commercials. Hence, we found several Action Unit patterns that were not investigated before, expanding our knowledge of the relation between facial expressions and advertisement and brand effects.
The presented findings also contribute to a better understanding of the relationships of different statistical aggregation strategies of AFC parameters. In particular, the experience and memorization of emotional events is influenced or even biased by different aggregates of such a dynamic time-series (Fredrickson, 2000). Specifically, we analyzed mean and peak AU activities which are both widely used aggregates in emotion research. Importantly, we found coherence and exclusive contributions of peak and mean statistics. Mean and peak statistics of AU06 (cheek raiser) and AU12 (lip corner pull) show no meaningful differences in the prediction of joy ratings. However, predictions of advertisement likeability and brand likeability change also demonstrate a differential impact of specific AU patterns. For example, peak activity of AU04 (Brow Lowerer) had a significant effect on these criteria, which was not the case for mean values of AU04. Hence, our findings contribute to a better understanding of the differential impact of facial expression aggregates in advertisement and brand research. Future studies should investigate the role of other associated phenomena, such as the peak-end-bias (e.g., Do et al., 2008) and the stability of effects over time in advertisement research.
Limitations and future directions
One aspect of the present study is the exclusive use of self-report ratings as the criteria in a cross-sectional design. The usage of ad hoc self-report scales has two significant limitations: First, it is unclear how stable reported advertisement effects on relevant brand dimensions are over time. Long-term effects of advertisement might be explored through longitudinal study design in future research, for example, by inviting participants again weeks or months after the main experiment to probe the stability of brand likeability and purchase intention changes. Second, it is unclear whether psychologically assessed intentions to purchase products or services of a particular brand elicit an actual purchase behavior. Future research should focus on predictions of actual behavior or even population-wide effects like it is approached with other methods in the consumer neuroscience literature (Berkman and Falk, 2013). Hence, out-of-sample criteria in the consumer research area that facial expression parameters might predict advertisement effects on a market-level response, such as monetary advertising elasticity estimates (Venkatraman et al., 2015) or video view frequencies on media platforms (Tong et al., 2020). Furthermore, facial responses toward music and movie trailers could predict actual sales figures in the music and movie industry, as already demonstrated with measures of neural response (Berns and Moore, 2012; Boksem and Smidts, 2015). Hence, future research needs to explore the predictive capability of facial expression recognition technology beyond within-subject measured self-report.
Automatic facial coding has also some advantages in comparison to emotional self-report because it enables a passive and non-contact assessment of emotional responses on a continuous basis. In contrast, emotional self-report is typically rated after stimulus presentation, and hence, reflect a more global and possibly biased evaluation of the recipients (e.g., Müller et al., 2019). Furthermore, AFC provides a rich data stream of emotion-relevant facial movements, whereas self-report is typically assessed on a limited number of emotion scales. AFC technology enables a moment-to-moment analysis of elicited emotional responses, which allows for the assessment of emotional responses toward dynamic emotional content as in video commercials. For example, stories can have very different emotional dynamics such as an unpleasant beginning and a pleasant end and vice versa (Reagan et al., 2016). Hence, future research should investigate the differential impact of emotional dynamics of advertisement commercials and whether differences in the emotional dynamics affect relevant advertisement and brand effects.
Conclusion
The present study identified facial expressions that were validated by self-reported emotional experience and predicted changes in brand likeability and purchase intention. Hence, this novel technology may be an excellent tool for tracking advertisement effects in real-time. Automatic facial coding enables a moment-to-moment analysis of emotional responses, non-invasive and non-contact. Accordingly, automatic emotional facial expression recognition is suitable for advertisement optimization based on emotional responses and for online research. Future research needs to evaluate the capability of such technology to predict actual consumer behavior beyond self-report and with out-of-sample criteria. Facial expressions can reveal very private emotional states and there will probably be a remarkable increase in the use of face recognition technology and its integration in everyday situations. Consequentially, many ethical issues will arise, specifically if applied in commercial and political contexts, and in particular if facial information is collected or analyzed without consent.
Data availability statement
The datasets presented in this study can be found in online repositories. The names of the repository/repositories and accession number(s) can be found below: https://madata.bib.uni-mannheim.de/id/eprint/410.
Ethics statement
The studies involving human participants were reviewed and approved by the Research Ethics Committee of the University of Mannheim. The patients/participants provided their written informed consent to participate in this study.
Author contributions
TH contributed to the conception and design of the study, collected the data, performed the statistical analysis, and wrote the first draft of the manuscript. TH and GA reviewed and approved the final manuscript.
Funding
The publication of this manuscript was funded by the Ministry of Science, Research and the Arts Baden-Württemberg and the University of Mannheim.
Acknowledgments
We thank Ulrich Föhl and both reviewers for the valuable feedback on the manuscript.
Conflict of interest
The authors declare that the research was conducted in the absence of any commercial or financial relationships that could be construed as a potential conflict of interest.
Publisher’s note
All claims expressed in this article are solely those of the authors and do not necessarily represent those of their affiliated organizations, or those of the publisher, the editors and the reviewers. Any product that may be evaluated in this article, or claim that may be made by its manufacturer, is not guaranteed or endorsed by the publisher.
Supplementary material
The Supplementary Material for this article can be found online at: https://www.frontiersin.org/articles/10.3389/fnins.2023.1125983/full#supplementary-material
References
Achar, C., So, J., Agrawal, N., and Duhachek, A. (2016). What we feel and why we buy: the influence of emotions on consumer decision-making. Curr. Opin. Psychol. 10, 166–170. doi: 10.1016/j.copsyc.2016.01.009
Ariely, D., and Berns, G. S. (2010). Neuromarketing: the hope and hype of neuroimaging in business. Nat. Rev. Neurosci. 11, 284–292. doi: 10.1038/nrn2795
Barrett, L. F., Adolphs, R., Marsella, S., Martinez, A. M., and Pollak, S. D. (2019). Emotional expressions reconsidered: challenges to inferring emotion from human facial movements. Psychol. Sci. Public Interest 20, 1–68. doi: 10.1177/1529100619832930
Bartlett, M. S., Hager, J. C., Ekman, P., and Sejnowski, T. J. (1999). Measuring facial expressions by computer image analysis. Psychophysiology 36, 253–263. doi: 10.1017/S0048577299971664
Beringer, M., Spohn, F., Hildebrandt, A., Wacker, J., and Recio, G. (2019). Reliability and validity of machine vision for the assessment of facial expressions. Cogn. Syst. Res. 56, 119–132. doi: 10.1016/j.cogsys.2019.03.009
Berkman, E. T., and Falk, E. B. (2013). Beyond brain mapping: using neural measures to predict real-world outcomes. Curr. Direct. Psychol. Sci. 22, 45–50. doi: 10.1177/0963721412469394
Berns, G. S., and Moore, S. E. (2012). A neural predictor of cultural popularity. J. Consum. Psychol. 22, 154–160. doi: 10.1016/j.jcps.2011.05.001
Boksem, M. A. S., and Smidts, A. (2015). Brain responses to movie trailers predict individual preferences for movies and their population-wide commercial success. J. Market. Res. 52, 482–492. doi: 10.1509/jmr.13.0572
Brown, S. P., and Stayman, D. M. (1992). Antecedents and consequences of attitude toward the ad: a meta-analysis. J. Consum. Res. 19, 34–51. doi: 10.1086/209284
Büdenbender, B., Höfling, T. T., Gerdes, A. B., and Alpers, G. W. (2023). Training machine learning algorithms for automatic facial coding: The role of emotional facial expressions’ prototypicality. PLoS One 18:e0281309. doi: 10.1371/journal.pone.0281309
Burnham, K. P., and Anderson, D. R. (2004). Multimodel inference: understanding AIC and BIC in model selection. Sociol. Methods Res. 33, 261–304. doi: 10.1177/0049124104268644
Calder, A. J., and Young, A. W. (2005). Understanding the recognition of facial identity and facial expression. Nat. Rev. Neurosci. 6, 641–651. doi: 10.1038/nrn1724
Calvo, M. G., Fernández-Martín, A., Recio, G., and Lundqvist, D. (2018). Human observers and automated assessment of dynamic emotional facial expressions: KDEF-dyn database validation. Front. Psychol. 9:2052. doi: 10.3389/fpsyg.2018.02052
Chen, X., An, L., and Yang, S. (2016). Zapping prediction for online advertisement based on cumulative smile sparse representation. Neurocomputing 175, 667–673. doi: 10.1016/j.neucom.2015.10.107
Cohen, J. (1988). Statistical Power Analysis for the Behavioral Sciences, 2nd Edn. Mahwah, NJ: Lawrence Erlbaum Associates, Inc.
Cohn, J. F., and Sayette, M. A. (2010). Spontaneous facial expression in a small group can be automatically measured: an initial demonstration. Behav. Res. Methods 42, 1079–1086. doi: 10.3758/BRM.42.4.1079
Cordaro, D. T., Sun, R., Kamble, S., Hodder, N., Monroy, M., Cowen, A., et al. (2020). The recognition of 18 facial-bodily expressions across nine cultures. Emotion 20, 1292–1300. doi: 10.1037/emo0000576
Cordaro, D. T., Sun, R., Keltner, D., Kamble, S., Huddar, N., and McNeil, G. (2018). Universals and cultural variations in 22 emotional expressions across five cultures. Emotion 18, 75–93. doi: 10.1037/emo0000302
Cowen, A. S., Keltner, D., Schroff, F., Jou, B., Adam, H., and Prasad, G. (2021). Sixteen facial expressions occur in similar contexts worldwide. Nature 589, 251–257. doi: 10.1038/s41586-020-3037-7
Dailey, M. N., Cottrell, G. W., Padgett, C., and Adolphs, R. (2002). EMPATH: a neural network that categorizes facial expressions. J. Cogn. Neurosci. 14, 1158–1173. doi: 10.1162/089892902760807177
Derbaix, C. M. (1995). The impact of affective reactions on attitudes toward the advertisement and the brand: a step toward ecological validity. J. Market. Res. 32, 470–479.
Dmochowski, J. P., Bezdek, M. A., Abelson, B. P., Johnson, J. S., Schumacher, E. H., and Parra, L. C. (2014). Audience preferences are predicted by temporal reliability of neural processing. Nat. Commun. 5:4567. doi: 10.1038/ncomms5567
Do, A. M., Rupert, A. V., and Wolford, G. (2008). Evaluations of pleasurable experiences: the peak-end rule. Psychon. Bull. Rev. 15, 96–98. doi: 10.3758/PBR.15.1.96
Durán, J. I., and Fernández-Dols, J.-M. (2021). Do emotions result in their predicted facial expressions? A meta-analysis of studies on the co-occurrence of expression and emotion. Emotion 21, 1550–1569. doi: 10.1037/emo0001015
Ekman, P., Friesen, W. V., and Hager, J. C. (2002). Facial Action Coding System. Manual and Investigator’s Guide. New York, NY: Research Nexus.
Ekman, P., Friesen, W. V., O’Sullivan, M., Chan, A., and Diacoyanni-Tarlatzis, I. (1987). Universals and cultural differences in the judgments of facial expressions of emotion. J. Pers. Soc. Psychol. 53, 712–717.
Falk, E. B., Berkman, E. T., and Lieberman, M. D. (2012). From neural responses to population behavior: neural focus group predicts population-level media effects. Psychol. Sci. 23, 439–445. doi: 10.1177/0956797611434964
Fraser, K., Ma, I., Teteris, E., Baxter, H., Wright, B., and McLaughlin, K. (2012). Emotion, cognitive load and learning outcomes during simulation training: emotion and cognitive load during simulation. Med. Educ. 46, 1055–1062. doi: 10.1111/j.1365-2923.2012.04355.x
Fredrickson, B. L. (2000). Extracting meaning from past affective experiences: the importance of peaks, ends, and specific emotions. Cogn. Emot. 14, 577–606. doi: 10.1080/026999300402808
Fridkin, K., Kenney, P. J., Cooper, B., Deutsch, R., Gutierrez, M., and Williams, A. (2021). Measuring emotional responses to negative commercials: a comparison of two methods. Polit. Res. Q. 74, 526–539. doi: 10.1177/1065912920912840
Hamelin, N., Moujahid, O. E., and Thaichon, P. (2017). Emotion and advertising effectiveness: a novel facial expression analysis approach. J. Retail. Consum. Serv. 36, 103–111. doi: 10.1016/j.jretconser.2017.01.001
Höfling, T. T. A., Alpers, G. W., Büdenbender, B., Föhl, U., and Gerdes, A. B. M. (2022). What’s in a face: automatic facial coding of untrained study participants compared to standardized inventories. PLoS One 17:e0263863. doi: 10.1371/journal.pone.0263863
Höfling, T. T. A., Alpers, G. W., Gerdes, A. B. M., and Föhl, U. (2021). Automatic facial coding versus electromyography of mimicked, passive, and inhibited facial response to emotional faces. Cogn. Emot. 35, 874–889. doi: 10.1080/02699931.2021.1902786
Höfling, T. T. A., Gerdes, A. B. M., Föhl, U., and Alpers, G. W. (2020). Read my face: automatic facial coding versus psychophysiological indicators of emotional valence and arousal. Front. Psychol. 11:1388. doi: 10.3389/fpsyg.2020.01388
Ito, T. A., and Cacioppo, J. T. (2001). “Affect and attitudes: a social neuroscience approach,” in Handbook of Affect and Social Cognition, ed. J. P. Forgas (Mahwah, NJ: Lawrence Erlbaum Associates Publishers), 50–74.
Keltner, D., Sauter, D., Tracy, J., and Cowen, A. (2019). Emotional expression: advances in basic emotion theory. J. Nonverb. Behav. 43, 133–160. doi: 10.1007/s10919-019-00293-3
Krohne, H., Egloff, B., Kohlmann, C., and Tausch, A. (1996). Untersuchungen mit einer deutschen Version der “positive and negative affect schedule”(PANAS) [Studies with a German version of the “positive and negative affect schedule”(PANAS)]. Diagnostica 42, 139–156.
Krumhuber, E. G., Küster, D., Namba, S., and Skora, L. (2021). Human and machine validation of 14 databases of dynamic facial expressions. Behav. Res. Methods 53, 686–701. doi: 10.3758/s13428-020-01443-y
Küntzler, T., Höfling, T. T. A., and Alpers, G. W. (2021). Automatic facial expression recognition in standardized and non-standardized emotional expressions. Front. Psychol. 12:627561. doi: 10.3389/fpsyg.2021.627561
Laux, L., Glanzmann, P., Schaffner, P., and Spielberger, C. D. (1981). Das State-Trait-Angstinventar [The State-Trait Anxiety Inventory]. Weinheim: Beltz.
Le Mau, T., Hoemann, K., Lyons, S. H., Fugate, J. M. B., Brown, E. N., Gendron, M., et al. (2021). Professional actors demonstrate variability, not stereotypical expressions, when portraying emotional states in photographs. Nat. Commun. 12:5037. doi: 10.1038/s41467-021-25352-6
Lemerise, E. A., and Arsenio, W. F. (2000). An integrated model of emotion processes and cognition in social information processing. Child Dev. 71, 107–118. doi: 10.1111/1467-8624.00124
Lewinski, P. (2015). Automated facial coding software outperforms people in recognizing neutral faces as neutral from standardized datasets. Front. Psychol. 6:1386. doi: 10.3389/fpsyg.2015.01386
Lewinski, P., den Uyl, T. M., and Butler, C. (2014a). Automated facial coding: validation of basic emotions and FACS AUs in facereader. J. Neurosci. Psychol. Econ. 7, 227–236. doi: 10.1037/npe0000028
Lewinski, P., Fransen, M. L., and Tan, E. S. H. (2014b). Predicting advertising effectiveness by facial expressions in response to amusing persuasive stimuli. J. Neurosci. Psychol. Econ. 7, 1–14. doi: 10.1037/npe0000012
Mahieu, B., Visalli, M., Schlich, P., and Thomas, A. (2019). Eating chocolate, smelling perfume or watching video advertisement: does it make any difference on emotional states measured at home using facial expressions? Food Qual. Pref. 77, 102–108. doi: 10.1016/j.foodqual.2019.05.011
Mauss, I. B., and Robinson, M. D. (2009). Measures of emotion: a review. Cogn. Emot. 23, 209–237. doi: 10.1080/02699930802204677
Mavadati, S. M., Mahoor, M. H., Bartlett, K., Trinh, P., and Cohn, J. F. (2013). DISFA: a spontaneous facial action intensity database. IEEE Trans. Affect. Comput. 4, 151–160. doi: 10.1109/T-AFFC.2013.4
McClure, S. M., Li, J., Tomlin, D., Cypert, K. S., Montague, L. M., and Montague, P. R. (2004). Neural correlates of behavioral preference for culturally familiar drinks. Neuron 44, 379–387. doi: 10.1016/j.neuron.2004.09.019
McDuff, D., El Kaliouby, R., Senechal, T., Demirdjian, D., and Picard, R. (2014). Automatic measurement of ad preferences from facial responses gathered over the internet. Image Vis. Comput. 32, 630–640. doi: 10.1016/j.imavis.2014.01.004
McDuff, D., Kaliouby, R. E., Cohn, J. F., and Picard, R. W. (2015). Predicting ad liking and purchase intent: large-scale analysis of facial responses to ads. IEEE Trans. Affect. Comput. 6, 223–235. doi: 10.1109/TAFFC.2014.2384198
Mohiyeddini, C., John, O., and Gross, J. J. (2008). Der “berkeley expressivity questionnaire”: deutsche adaption und erste validierungsbefunde. Diagnostica 54, 117–128. doi: 10.1026/0012-1924.54.3.117
Müller, U. W. D., Witteman, C. L. M., Spijker, J., and Alpers, G. W. (2019). All’s bad that ends bad: there is a peak-end memory bias in anxiety. Front. Psychol. 10:1272. doi: 10.3389/fpsyg.2019.01272
Ohme, R., Reykowska, D., Wiener, D., and Choromanska, A. (2010). Application of frontal EEG asymmetry to advertising research. J. Econ. Psychol. 31, 785–793. doi: 10.1016/j.joep.2010.03.008
Otamendi, F. J., and Sutil Martín, D. L. (2020). The emotional effectiveness of advertisement. Front. Psychol. 11:2088. doi: 10.3389/fpsyg.2020.02088
Pantic, M., and Rothkrantz, L. J. M. (2003). Toward an affect-sensitive multimodal human-computer interaction. Proc. IEEE 91, 1370–1390. doi: 10.1109/JPROC.2003.817122
Pierce, C. A., Block, R. A., and Aguinis, H. (2004). Cautionary note on reporting eta-squared values from multifactor ANOVA designs. Educ. Psychol. Meas. 64, 916–924. doi: 10.1177/0013164404264848
Pittig, A., Schulz, A. R., Craske, M. G., and Alpers, G. W. (2014). Acquisition of behavioral avoidance: task-irrelevant conditioned stimuli trigger costly decisions. J. Abnorm. Psychol. 123, 314–329. doi: 10.1037/a0036136
Plassmann, H., Ramsøy, T. Z., and Milosavljevic, M. (2012). Branding the brain: a critical review and outlook. J. Consum. Psychol. 22, 18–36. doi: 10.1016/j.jcps.2011.11.010
Plusquellec, P., and Denault, V. (2018). The 1000 most cited papers on visible nonverbal behavior: a bibliometric analysis. J. Nonverb. Behav. 42, 347–377. doi: 10.1007/s10919-018-0280-9
Reagan, A. J., Mitchell, L., Kiley, D., Danforth, C. M., and Dodds, P. S. (2016). The emotional arcs of stories are dominated by six basic shapes. EPJ Data Sci. 5:31. doi: 10.1140/epjds/s13688-016-0093-1
Sander, D., Grandjean, D., and Scherer, K. R. (2018). An appraisal-driven componential approach to the emotional brain. Emot. Rev. 10, 219–231. doi: 10.1177/1754073918765653
Sato, W., Hyniewska, S., Minemoto, K., and Yoshikawa, S. (2019). Facial expressions of basic emotions in Japanese laypeople. Front. Psychol. 10:259. doi: 10.3389/fpsyg.2019.00259
Sayette, M. A., Cohn, J. F., Wertz, J. M., Perrott, M. A., and Parrott, D. J. (2001). A psychometric evaluation of the facial action coding system for assessing spontaneous expression. J. Nonverb. Behav. 25, 167–185.
Scherer, K. R., Dieckmann, A., Unfried, M., Ellgring, H., and Mortillaro, M. (2021). Investigating appraisal-driven facial expression and inference in emotion communication. Emotion 21, 73–95. doi: 10.1037/emo0000693
Scherer, K. R., and Ellgring, H. (2007). Multimodal expression of emotion: affect programs or componential appraisal patterns? Emotion 7, 158–171. doi: 10.1037/1528-3542.7.1.158
Scherer, K. R., and Moors, A. (2019). The emotion process: event appraisal and component differentiation. Annu. Rev. Psychol. 70, 719–745. doi: 10.1146/annurev-psych-122216-011854
Scherer, K. R., Mortillaro, M., Rotondi, I., Sergi, I., and Trznadel, S. (2018). Appraisal-driven facial actions as building blocks for emotion inference. J. Pers. Soc. Psychol. 114, 358–379. doi: 10.1037/pspa0000107
Schulte-Mecklenbeck, M., Johnson, J. G., Böckenholt, U., Goldstein, D. G., Russo, J. E., Sullivan, N. J., et al. (2017). Process-tracing methods in decision making: on growing up in the 70s. Curr. Direct. Psychol. Sci. 26, 442–450. doi: 10.1177/0963721417708229
Seuss, D., Hassan, T., Dieckmann, A., Unfried, M., Scherer, K. R. R., Mortillaro, M., et al. (2021). “Automatic estimation of action unit intensities and inference of emotional appraisals,” in Proceedings of the IEEE Transactions on Affective Computing (Piscataway, NJ: IEEE).
Shiv, B., Carmon, Z., and Ariely, D. (2005). Placebo effects of marketing actions: consumers may get what they pay for. J. Market. Res. 42, 383–393. doi: 10.1509/jmkr.2005.42.4.383
Skiendziel, T., Rösch, A. G., and Schultheiss, O. C. (2019). Assessing the convergent validity between the automated emotion recognition software Noldus FaceReader 7 and facial action coding system scoring. PLoS One 14:e0223905. doi: 10.1371/journal.pone.0223905
Slovic, P., Finucane, M. L., Peters, E., and MacGregor, D. G. (2007). The affect heuristic. Eur. J. Operat. Res. 177, 1333–1352. doi: 10.1016/j.ejor.2005.04.006
Solnais, C., Andreu-Perez, J., Sánchez-Fernández, J., and Andréu-Abela, J. (2013). The contribution of neuroscience to consumer research: a conceptual framework and empirical review. J. Econ. Psychol. 36, 68–81. doi: 10.1016/j.joep.2013.02.011
Stangier, U., Heidenreich, T., Berardi, A., Golbs, U., and Hoyer, J. (1999). Die Erfassung sozialer Phobie durch die Social Interaction Anxiety Scale (SIAS) und die Social Phobia Scale (SPS) [Measurement of social phobia with the Social Interaction Anxiety Scale (SIAS) and the Social Phobia Scale (SPS)]. Zeitschrift Für Klinische Psychol. Psychother. 28, 28–36. doi: 10.1026//0084-5345.28.1.28
Stöckli, S., Schulte-Mecklenbeck, M., Borer, S., and Samson, A. C. (2018). Facial expression analysis with AFFDEX and FACET: a validation study. Behav. Res. Methods 50:4. doi: 10.3758/s13428-017-0996-1
Strobel, A., Beauducel, A., Debener, S., and Brocke, B. (2001). Eine deutschsprachige Version des BIS/BAS-Fragebogens von Carver und White [A German version of Carver and White’s BIS/BAS scales]. Zeitschrift Für Diff. Diagn. Psychol. 22, 216–227. doi: 10.1024/0170-1789.22.3.216
Tcherkassof, A., and Dupré, D. (2021). The emotion–facial expression link: evidence from human and automatic expression recognition. Psychol. Res. 85, 2954–2969. doi: 10.1007/s00426-020-01448-4
Teixeira, T., Picard, R., and El Kaliouby, R. (2014). Why, when, and how much to entertain consumers in advertisements? A web-based facial tracking field study. Market. Sci. 33, 809–827. doi: 10.1287/mksc.2014.0854
Teixeira, T., Wedel, M., and Pieters, R. (2012). Emotion-induced engagement in internet video advertisements. J. Market. Res. 49, 144–159. doi: 10.1509/jmr.10.0207
Tian, Y.-I., Kanade, T., and Cohn, J. F. (2001). Recognizing action units for facial expression analysis. IEEE Trans. Pattern Anal. Mach. Intellig. 23, 97–115. doi: 10.1109/34.908962
Tong, L. C., Acikalin, M. Y., Genevsky, A., Shiv, B., and Knutson, B. (2020). Brain activity forecasts video engagement in an internet attention market. Proc. Natl. Acad. Sci. U.S.A. 117, 6936–6941. doi: 10.1073/pnas.1905178117
van der Schalk, J., Hawk, S. T., Fischer, A. H., and Doosje, B. (2011). Moving faces, looking places: validation of the amsterdam dynamic facial expression set (ADFES). Emotion 11, 907–920. doi: 10.1037/a0023853
Van Kuilenburg, H., Den Uyl, M. J., Israel, M. L., and Ivan, P. (2008). “Advances in face and gesture analysis,” in Proceedings of 6th International Conference on Methods and Techniques in Behavioral Research, Maastricht, 371–372.
Van Kuilenburg, H., Wiering, M. A., and Den Uyl, M. J. (2005). “A model based method for automatic facial expression recognition,” in Proceedings of the 16th European Conference on Machine Learning, Porto, 194–205.
Vecchiato, G., Astolfi, L., De Vico Fallani, F., Toppi, J., Aloise, F., Bez, F., et al. (2011). On the use of EEG or MEG brain imaging tools in neuromarketing research. Comput. Intellig. Neurosci. 2011, 1–12. doi: 10.1155/2011/643489
Venkatraman, V., Dimoka, A., Pavlou, P. A., Vo, K., Hampton, W., Bollinger, B., et al. (2015). Predicting advertising success beyond traditional measures: new insights from neurophysiological methods and market response modeling. J. Market. Res. 52, 436–452. doi: 10.1509/jmr.13.0593
Viola, P., and Jones, M. J. (2004). Robust real-time face detection. Int. J. Comput. Vis. 57, 137–154. doi: 10.1023/B:VISI.0000013087.49260.fb
Wood, S. N. (2006). Low-rank scale-invariant tensor product smooths for generalized additive mixed models. Biometrics 62, 1025–1036. doi: 10.1111/j.1541-0420.2006.00574.x
Wood, S. N., Scheipl, F., and Faraway, J. J. (2013). Straightforward intermediate rank tensor product smoothing in mixed models. Stat. Comput. 23, 341–360. doi: 10.1007/s11222-012-9314-z
Yang, S., Kafai, M., An, L., and Bhanu, B. (2014). Zapping index:using smile to measure advertisement zapping likelihood. IEEE Trans. Affect. Comput. 5, 432–444. doi: 10.1109/TAFFC.2014.2364581
Yitzhak, N., Giladi, N., Gurevich, T., Messinger, D. S., Prince, E. B., Martin, K., et al. (2017). Gently does it: humans outperform a software classifier in recognizing subtle, nonstereotypical facial expressions. Emotion 17, 1187–1198. doi: 10.1037/emo0000287
Keywords: automatic facial coding, action units (AU), FACS, facial expression, emotion, advertisement, brand, semiparametric additive mixed models
Citation: Höfling TTA and Alpers GW (2023) Automatic facial coding predicts self-report of emotion, advertisement and brand effects elicited by video commercials. Front. Neurosci. 17:1125983. doi: 10.3389/fnins.2023.1125983
Received: 16 December 2022; Accepted: 10 February 2023;
Published: 02 May 2023.
Edited by:
Giulia Cartocci, Sapienza University of Rome, ItalyReviewed by:
Marc Baker, University of Portsmouth, United KingdomPeter Lewinski, University of Oxford, United Kingdom
Copyright © 2023 Höfling and Alpers. This is an open-access article distributed under the terms of the Creative Commons Attribution License (CC BY). The use, distribution or reproduction in other forums is permitted, provided the original author(s) and the copyright owner(s) are credited and that the original publication in this journal is cited, in accordance with accepted academic practice. No use, distribution or reproduction is permitted which does not comply with these terms.
*Correspondence: Georg W. Alpers, YWxwZXJzQHVuaS1tYW5uaGVpbS5kZQ==