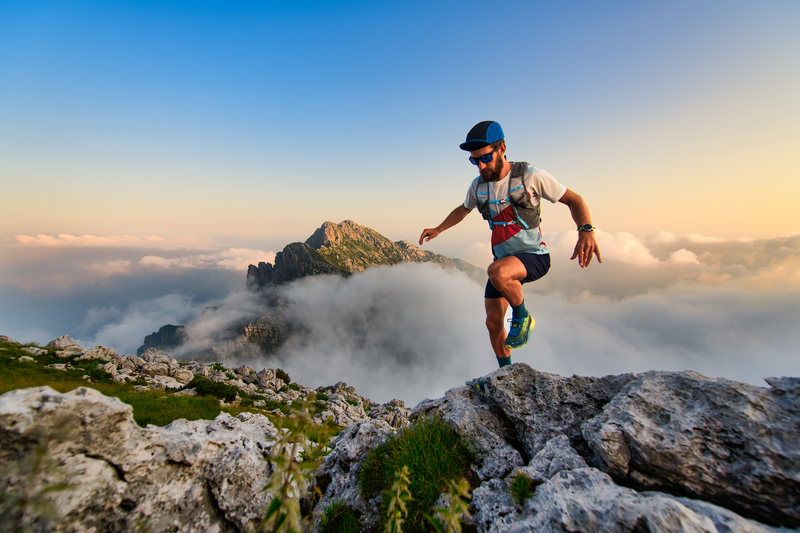
95% of researchers rate our articles as excellent or good
Learn more about the work of our research integrity team to safeguard the quality of each article we publish.
Find out more
ORIGINAL RESEARCH article
Front. Neurosci. , 08 November 2022
Sec. Neurogenomics
Volume 16 - 2022 | https://doi.org/10.3389/fnins.2022.974838
This article is part of the Research Topic Regulatory Networks Involved in Neurodegeneration View all articles
Parkinson's disease (PD) is a common neurodegenerative disease, and there is still a lack of effective diagnostic and treatment methods. This study aimed to search for hub genes that might serve as diagnostic or therapeutic targets for PD. All the analysis was performed in R software. The expression profile data of PD (number: GSE7621) was acquired from the Gene Expression Omnibus (GEO) database. Differentially expressed genes (DEGs) associated with PD were screened by the “Limma” package of the R software. Key genes associated with PD were screened by the “WGCNA” package of the R software. Target genes were screened by merging the results of “Limma” and “WGCNA.” Enrichment analysis of target genes was performed by Gene Ontology (GO), Disease Ontology (DO), and Kyoto Enrichment of Genes and Genomes (KEGG). Machine learning algorithms were employed to screen for hub genes. Nomogram was constructed using the “rms” package. And the receiver operating characteristic curve (ROC) was plotted to detect and validate our prediction model sensitivity and specificity. Additional expression profile data of PD (number: GSE20141) was acquired from the GEO database to validate the nomogram. GSEA was used to determine the biological functions of the hub genes. Finally, RPL3L, PLEK2, PYCRL, CD99P1, LOC100133130, MELK, LINC01101, and DLG3-AS1 were identified as hub genes of PD. These findings can provide a new direction for the diagnosis and treatment of PD.
Parkinson's disease (PD) is one of the common neurodegenerative diseases, second only to Alzheimer's disease (Di Stefano and Marinelli, 2021; Pan et al., 2021). The global prevalence of PD is rising, affecting nearly 2% of people over the age of 65 and 5% of people over the age of 85 (Bloem et al., 2021; Dorszewska et al., 2021). The pathological features of PD are mainly the loss of dopaminergic neurons in the substantia nigra and the formation of Lewy bodies, which is determined by genetic and environmental factors, and related to age, immune-inflammatory mechanisms, mitochondrial dysfunction, oxidative stress, apoptosis, lysosomal dysfunction, etc (Pan-Montojo and Reichmann, 2014; Su and Federoff, 2014; Kalia and Lang, 2015; Mullin and Schapira, 2015; Vivekanantham et al., 2015; Hu and Wang, 2016; Collier et al., 2017; Vascellari and Manzin, 2021). The role of genetic factors in PD is receiving more attention, and dozens of genes have been found to be related to the incidence of PD, including SNCA, LRRK2, and so on (Manto and Marmolino, 2009; Deng et al., 2018; Poujois and Woimant, 2018). Neurologists' assessments of clinical manifestations, movement disorders, and some routine laboratory tests are the most important diagnostic methods for PD. However, these methods have disadvantages and limitations, such as low sensitivity, selectivity, and high cost (Bindas et al., 2021; Mobed et al., 2021).
The treatment of PD is constantly developing, including drug therapy, surgical treatment, gene therapy, rehabilitation, etc. However, drug therapy is still the preferred treatment of Parkinson's disease in the clinic and is the main treatment method. However, drug therapy can only improve symptoms and cannot control the progression of the disease. With the prolongation of the medication duration and the dose increase, there will be a decrease in the efficacy of the drug and complications. Some researchers are developing new gene promoters to control gene expression in different subsets of neurons, stimulating the growth and development of specific neurons (such as dopaminergic neurons), thereby promoting the recovery of Parkinson's disease. Another emerging form of gene therapy is using novel drugs that directly deliver key proteins involved in dopamine metabolism to the basal ganglia region. Using gene therapies to clear disease-causing proteins is another beneficial exploration (Brundin et al., 1987; Lindvall et al., 1988; Ropper and Samuels, 1997). Although many genes are associated with PD, the specific pathogenesis is not clear, and there is still a lack of understanding of the genes that can be used for the diagnosis and treatment of PD. It is important to find new genes related to PD, which can be used for new targets for treatment.
Recently, bioinformatics analysis has been widely used as a new technique to screen for underlying biomarkers for both tumor and non-tumor diseases (Zhang et al., 2022). In this study, we downloaded a microarray dataset and analyzed gene expression to obtain differentially expressed genes from persons with PD and healthy individuals. We combined the data with WGCNA and machine learning algorithms, screened out a total of eight core genes, verified the prediction accuracy by area size (AUC) under the ROC curve, and performed GSEA analysis on each hub gene. We aimed to identify candidate genes that may be used as PD biomarkers.
The raw data of the substantia nigra tissue from 16 PD and nine American normal samples in the GSE7621 (Lesnick et al., 2007) dataset, which was sequenced using the GPL570 platform, was obtained from the GEO database.
The “Limma” R package was used to screen DEGs between PD and normal samples, and genes with P < 0.05 and |log2FC| >1 were regarded as DEGs (Liu et al., 2021).
To screen potential genes associated with PD, the gene expression matrix of the substantia nigra tissue from 16 PD and nine normal American samples was used to create a weighted gene co-expression network using the “WGCNA” R package (Langfelder and Horvath, 2008; Yu et al., 2012). First, we clustered all samples to guarantee a reliable network. Second, we calculated the Pearson correlation coefficient between each pair of genes to evaluate the expression similarity of genes and acquire a correlation matrix. We also used the soft threshold function to convert the correlation matrix into a weighted neighborhood matrix and used a soft connectivity algorithm to select the optimal soft threshold to ensure that gene correlations were maximally consistent with scale-free distribution. Subsequently, the neighborhood matrix was transformed into a topological overlap matrix (TOM). Furthermore, co-expression modules were obtained based on the criteria of dynamic tree cutting by setting the minimum number of genes in a module as 50. Finally, key modules were selected by correlation analysis, and the key modules' genes were considered key genes. Target genes were obtained by intersecting DEGs with key genes based on WGCNA screening.
Biological function enrichment of Gene Ontology (GO), Disease Ontology (DO), and Kyoto Enrichment of Genes and Genomes (KEGG) analyses were performed using the “clusterProfiler” R (Yu et al., 2012) package. GO enrichment analysis was performed to investigate the gene-related biological process (BP), molecular functions (MF), and cellular components (CC). DO enrichment analysis was used to explore genes-related diseases. KEGG enrichment analysis was conducted to explore gene-related signaling pathways. Statistical significance was set at an adjusted P-value < 0.05.
To begin with, the LASSO logistic regression algorithm was performed to screen potential genes by using the “glmnet” R package (Tibshirani, 1996; Friedman et al., 2010), and receiver operating characteristic (ROC) analysis was selected to test the model reliability by calculating the area under the curve (AUC) value through the “pROC” R package (Robin et al., 2011). Next, the SVM-RFE algorithm was used to screen potential genes using the “e1071” R package (Suykens and Vandewalle, 2004; Huang et al., 2014). In addition, the random forest (RF) algorithm was also conducted to screen potential genes using the “randomForest” R package (Liaw and Wiener, 2007; Cutler et al., 2011). Similarly, the ROC curve was used to test the model reliability by using the “pROC” R package, and the top 10 genes based on %IncMSE ranking were regarded as potential genes (Robin et al., 2011). Finally, overlapping genes among potential genes generated via LASSO, SVM-RFE, and RF algorithms were considered hub genes of PD.
A diagnostic nomogram was established based on the hub genes by using the “rms” package in R software. The receiver operating characteristic curve (ROC) was used to investigate the efficiency of this diagnostic model. The area under curve >0.7 was considered significant. Additional expression profile data of PD [number: GSE20141 (Zheng et al., 2010)] was acquired from the GEO database to validate the nomogram.
Wilcoxon's rank-sum test was used to analyze the expression levels of hub genes. ROC analysis was performed to evaluate whether hub genes could differentiate PD samples from normal samples using the “pROC” R package (Robin et al., 2011).
Gene Set Enrichment Analysis (GSEA) was performed using the “clusterProfiler” R package to investigate the biological functions of hub genes by the ordered gene expression matrix based on the Pearson correlation between each hub gene and other genes (Yu et al., 2012).
By setting the cut-off value as P < 0.05 and |log2FC| >1, a total of 117 DEGs, including 42 upregulated and 75 downregulated genes were identified in the substantia nigra of PD patients compared with normal samples (Table 1). A volcano diagram was constructed for the DEGs (Figure 1A). The top 60 DEGs are presented using a cluster heatmap (Figure 1B).
Figure 1. DEGs between PD patients and normal samples. (A) Volcano plot showing the expression levels of DEGs. Red dots indicate upregulated genes in PD patients compared with normal samples. In contrast, blue dots indicate downregulated genes in PD patients compared with normal samples, and gray dots indicate nonsignificant differences in genes between PD patients and normal samples. (B) Heat map showing the expression levels of the top 60 DEGs. Red indicates high expression, while blue indicates low expression.
We extracted the expression data of differentially expressed genes in samples of persons with PD for co-expression analysis. First, the soft threshold was selected for subsequent co-expression network construction (Figure 2A). The principle was to make the constructed network more in line with the characteristics of the scale-free network. The R-square was set as 0.85 (Figure 2A). WGCNA was used to construct the co-expression network module and visually display the modules' gene correlation. Fourteen co-expression modules were obtained, and the number of genes in each module was at least 50. The results were displayed in a hierarchical clustering diagram (Figure 2B). Then, a heat map was mapped on module-trait relationships according to the Spearman correlation coefficient to evaluate the association between each module and the disease (Figure 2C). Two modules “MEdarkgrey” and “MEdarkorange” had high association with PD and were selected as PD-related modules (MEdarkgrey module: r = 0.91, P = 4e−10; MEdarkorange module: r = 0.89, P = 4e−08). The MEdarkgrey and MEdarkorange modules were positively correlated with PD, 5,801, and 7,763 genes, respectively. Target genes were obtained by intersecting DEGs with key genes based on WGCNA screening (Figure 2D).
Figure 2. Weighted gene co-expression network analysis (WGCNA). (A) Soft threshold analysis suggested gene associations were maximally consistent with the scale-free distribution when β = 26. (B) The cluster dendrogram of co-expression genes in PD. (C) Module-trait relationships in PD. Each cell contains the corresponding correlation and P-value. (D) A Venn diagram was made to obtain the intersection of the target genes screened by the two methods.
The GO analyses associated the most enriched biological process (BP) terms with dopamine biosynthetic process, synapse organization regulation, and synapse structure or activity. The most enriched terms for cellular components (CC) were mainly associated with terminal bouton. The most enriched molecular function (MF) terms were associated with G protein-coupled peptide receptor activity and peptide receptor activity (Figures 3A,B). In the DO analysis, the target genes were enriched in neurodegenerative diseases, such as PD, and tumors of the nervous system (Figures 3C,D). In the KEGG analysis, the target genes were enriched in cocaine addiction and the calcium signaling pathway (Figures 3E,F).
Figure 3. GO, DO, and KEGG analyses of target genes. (A,B) GO analyses of target genes. (C,D) DO analysis of target genes. (E,F) KEGG analysis of target genes. BP, biological process; CC, cellular component; MF, molecular function.
Machine learning algorithms were selected and executed to further identify the hub genes of PD from 91 target genes. First, while constructing the LASSO model based on PD and normal samples, λ analysis suggested that the model could accurately predict PD with λ = 11 (Figure 4A). Thus, RPL3L, PLEK2, PYCRL, ABCA11P, DACH2, CD99P1, SNORD114-3, LOC100133130, MELK, LINC01101, and DLG3-AS1 were identified to build the LASSO module. We acquired the LASSO coefficient spectrum of the potential genes according to λ = 11(Figure 4A). However, SVM-RFE analysis revealed that the SVM model based on one characteristic gene showed an optimum error rate (0.00, Figure 4B). The first 20 genes were identified as potential genes. At the same time, the RF algorithm identified the top 10 genes from 52 potential genes (Figure 5C). Finally, eight common potential genes, namely, RPL3L, PLEK2, PYCRL, CD99P1, LOC100133130, MELK, LINC01101, and DLG3-AS1, were regarded as the hub genes of PD patients using the above three algorithms (Figure 4D).
Figure 4. Identification of hub genes for PD based on machine learning algorithms included (A) the Log (Lambda) value of the three genes in the LASSO model and the most proper log (Lambda) value in the LASSO model, (B) the optimum error rate of the SVM model based on one characteristic gene, (C) the RF module based on the top 20 genes, and (D) the Venn diagram showing the overlapping genes in LASSO, SVM, and RF modules.
Figure 5. (A) Nomogram predicting PD probability. (B,C) ROC curve for the GSE7621 dataset and GSE20141 dataset.
A diagnostic nomogram was successfully constructed based on the eight genes for predicting the incidence of PD (Figure 5A). The area under the curve (AUC) of the GSE7621 dataset was 1.000 (Figure 5B), and that of the GSE20141 dataset was 0.900 (Figure 5C).
To further investigate the role of hub genes in PD, we first observed their expression levels in PD patients. Interestingly, we found that the expression of PYCRL, LOC100133130, MELK, LINC01101, and DLG3-AS1 was downregulated, and the expression of RPL3L, PLEK2, and CD99P1 was upregulated in PD patients compared with the healthy samples (Figure 6A). Moreover, ROC analyses suggested RPL3L, PLEK2, PYCRL, CD99P1, LOC100133130, MELK, LINC01101, and DLG3-AS1 might be used as hub genes of PD patients (Figure 6B).
Figure 6. The expression levels and diagnostic implications of the hub genes. (A) The expression levels of hub genes in PD patients and normal samples of the dataset (number: GSE7621); (B) ROC curves of hub genes of the dataset (number: GSE7621).
To further investigate the biological functions of RPL3L, PLEK2, PYCRL, CD99P1, LOC100133130, MELK, LINC01101, and DLG3-AS1, GSEA was performed based on their ordered gene expression matrix. As shown in Figure 7, GSEA analyses revealed that RPL3L, PLEK2, PYCRL, CD99P1, LOC100133130, MELK, LINC01101, and DLG3-AS1 were mainly involved in glycosaminoglycan biosynthesis – heparan sulfate/heparin, histidine metabolism, nicotine addiction, diabetes, protein export, taste transduction, fatty acid degradation, propanoate metabolism, endocrine, and other factor–regulated calcium reabsorption, GABAergic synapse, steroid biosynthesis, synaptic vesicle cycle, carbohydrate digestion and absorption, proteasome, and asthma (Figure 7).
Figure 7. GSEA of hub genes: (A) GSEA results for CD99P1; (B) GSEA results for DLG3-AS1; (C) GSEA results for LINC01101; (D) GSEA results for LOC100133130; (E) GSEA results for MELK; (F) GSEA results for PLEAK2; (G) GSEA results for PYCRL; (H) GSEA results for RPL3L.
Parkinson's disease is the second most prevalent neurodegenerative disease and has significantly increased over the past 20 years (Cabreira and Massano, 2019). It is caused by a combination of environmental and genetic factors related to age, sex, etc (Cabreira and Massano, 2019; Cerri et al., 2019). Due to the lack of early diagnostic techniques, PD is usually not detected until later stages of complete neuronal degeneration, which often leads to delayed treatment of patients and affects the prognosis (Lotankar et al., 2017). Therefore, to improve prognosis, biomarkers are needed to detect the onset of the disease in the early stages.
In the present study, we first obtained 117 DEGs from the substantia nigra of PD, and WGCNA screened a total of 13,564 key genes for two key modules. Ninety-one target genes were screened out by intersecting DEGs with key genes. Interestingly, these 91 target genes were mostly related to the regulation of synapse structure or activity, dopamine biosynthetic process, locomotory behavior, and response to nicotine metabolism-related BPs (Figures 3A,B). Thus, we speculated that these genes might play key roles in PD by regulating the synapse structure or activity, dopamine biosynthetic process, locomotory behavior, and response to nicotine.
Over the past few decades, more and more studies have shown that the biosynthesis of dopamine and the regulation of synaptic activity and structure play an important role in the pathogenesis of PD (Latif et al., 2021; Nachman and Verstreken, 2022). In addition, increasing evidence has revealed that nicotine has protective effects on PD (Jin Jung et al., 2021; Wang et al., 2022). Furthermore, motor symptoms are one of the most important clinical manifestations of PD (Opara et al., 2017). However, we found that 91 target genes were enriched only in the calcium signaling pathway (Figures 3E,F). Calcium signaling pathways play an important role in PD (Calı̀ et al., 2014; Bohush et al., 2021). Therefore, our study may contribute to understanding the molecular mechanisms underlying PD.
Finally, we identified RPL3L, PLEK2, PYCRL, CD99P1, LOC100133130, MELK, LINC01101, and DLG3-AS1 as hub genes using LASSO logistic regression, SVM-RFE, and RF algorithms. RPL3L (ribosomal protein L3-like) is one of the four non-canonical riboprotein genes, and it encodes the 60S ribosomal protein L3-like protein that is highly expressed only in cardiac and skeletal muscles (Ganapathi et al., 2020). Recent research indicates that RPl3l overexpression impairs the growth and myogenic fusion of myotubes, and RPL3L can be used as a potential genetic marker to control neurodegeneration (Chaillou, 2019). Thus, RPL3L may play a critical role in PD by regulating function of skeletal muscle. CD99P1 (CD99 molecule pseudogene 1) is a long noncoding RNA (lncRNA) and has been revealed to be related to myofibroblast differentiation (Huang et al., 2015; Yildirim et al., 2021). Hence, CD99P1 may play a key role in PD by affecting myofibroblast differentiation. PYCRL (pyrroline-5-carboxylate reductase-like) is a pyrroline-5-carboxylate reductase linked to the conversion of ornithine to proline (De Ingeniis et al., 2012). Therefore, PYCRL may play a decisive role in PD by affecting proline biosynthesis. MELK (maternal embryonic leucine zipper kinase) is an AMP-activated protein kinase (AMPK)-related kinase (Seong and Ha, 2019). More and more studies have found that MELK is involved in the occurrence of cancer and cell metabolism by regulating the cell division cycle (Wang et al., 2018; Seong and Ha, 2019). Therefore, MELK may play a key role in PD by affecting the cell division cycle. PLEK2 (Pleckstrin-2) is a crucial mediator of cytoskeletal reorganization (Wang et al., 2021). Studies show that PLEK2 may regulate actin organization and cell spreading (Hu et al., 1999; Hamaguchi et al., 2007). Therefore, PLEK2 may play a key role in PD by regulating actin organization and cell spreading. LINC01101 is a long noncoding RNA (lncRNA) associated with progression and high-risk HPV infection (Iancu et al., 2017). But there is still no description of its pathogenesis. Notably, no studies have reported the role of RPL3L, PLEK2, PYCRL, CD99P1, LOC100133130, MELK, LINC01101, and DLG3-AS1 in PD. LOC100133130 and DLG3-AS1, as newly discovered genes, have no relevant report. Thus, further investigations are necessary.
We investigated the biological functions of RPL3L, PLEK2, PYCRL, CD99P1, LOC100133130, MELK, LINC01101, and DLG3-AS1. Interestingly, GSEA revealed that RPL3L, PLEK2, PYCRL, CD99P1, LOC100133130, MELK, LINC01101, and DLG3-AS1 were mainly involved in nicotine addiction, diabetes, protein export, taste transduction, fatty acid degradation, propanoate metabolism, endocrine and other factor-regulated calcium reabsorption, GABAergic synapse, steroid biosynthesis, synaptic vesicle cycle, carbohydrate digestion and absorption, proteasome, and asthma. Currently, an increasing number of studies have shown that the above factors significantly impact the occurrence and development of PD. For example, it has been suggested that nicotine addiction and diabetes are associated with PD (Cheong et al., 2020; Carvajal-Oliveros et al., 2021). However, their regulatory mechanisms are rarely studied to the best of our knowledge. Thus, further studies are required to explore this in the future.
In all, 117 DEGs were screened between the substantia nigra of PD and healthy samples. Of these, RPL3L, PLEK2, PYCRL, CD99P1, LOC100133130, MELK, LINC01101, and DLG3-AS1 were identified as hub genes of patients with PD based on WGCNA and machine learning algorithms. Therefore, our study contributes to the understanding of PD and helps in improving the diagnosis and treatment of PD. However, further studies are needed to investigate the roles of hub genes.
The original contributions presented in the study are included in the article/supplementary material, further inquiries can be directed to the corresponding author.
Ethical review and approval was not required for the study on human participants in accordance with the local legislation and institutional requirements. Written informed consent from the patients/participants or patients/participants' legal guardian/next of kin was not required to participate in this study in accordance with the national legislation and the institutional requirements.
YY conceived of the presented idea. XH developed the theory, performed the computations, and supervised the findings of this work. CW and YW verified the analytical methods. XX and CL contributed in the revision of the article. All authors discussed the results and contributed to the final manuscript.
This work was funded by Research Project Supported by Shanxi Scholarship Council of China (2020-169), Fund Program for the Scientific Activities of Selected Returned Overseas Professionals in Shanxi Province (20210023), and Fundamental Research Program of Shanxi Province (202203021211017).
We are grateful to everyone who has helped us with this paper. Our sincere gratitude and appreciation go first to our supervisor, whose suggestions and encouragement gave us much insight into these translation studies. Studying under his guidance and supervision has been a great privilege and joy. Furthermore, it is our honor to benefit from his personality and diligence, which we will treasure our whole life. Our gratitude to him knows no bounds. We are also extremely grateful to all our friends and classmates who have provided us with assistance and companionship in preparing this paper. In addition, many thanks go to our family for their unfailing love and unwavering support. Finally, we are grateful to all those who devoted much time to reading this thesis and giving us much advice, which will benefit us in our later studies.
The authors declare that the research was conducted in the absence of any commercial or financial relationships that could be construed as a potential conflict of interest.
All claims expressed in this article are solely those of the authors and do not necessarily represent those of their affiliated organizations, or those of the publisher, the editors and the reviewers. Any product that may be evaluated in this article, or claim that may be made by its manufacturer, is not guaranteed or endorsed by the publisher.
Bindas, A. J., Kulkarni, S., Koppes, R. A., and Koppes, A. N. (2021). Parkinson's disease and the gut: models of an emerging relationship. Acta Biomater. 132, 325–344. doi: 10.1016/j.actbio.2021.03.071
Bloem, B. R., Okun, M. S., and Klein, C. (2021). Parkinson's disease. Lancet. 397, 2284–2303. doi: 10.1016/S0140-6736(21)00218-X
Bohush, A., Leśniak, W., Weis, S., and Filipek, A. (2021). Calmodulin and its binding proteins in Parkinson's disease. Int. J. Mol. Sci. 22. doi: 10.3390/ijms22063016
Brundin, P., Strecker, R. E., Lindvall, O., Isacson, O., Nilsson, O. G., Barbin, G., et al. (1987). Intracerebral grafting of dopamine neurons. Experimental basis for clinical trials in patients with Parkinson's disease. Ann. N. Y. Acad. Sci. 495, 473–96. doi: 10.1111/j.1749-6632.1987.tb23695.x
Cabreira, V., and Massano, J. J. A. M. P. (2019). Doença de Parkinson: revisão clínica e atualização. Parkinson's disease: clinical review and update. Acta. Med. Port. 32:661–670. doi: 10.20344/amp.11978
Calì, T., Ottolini, D., and Brini, M. (2014). Calcium signaling in Parkinson's disease. Cell Tissue Res. 357, 439–54. doi: 10.1007/s00441-014-1866-0
Carvajal-Oliveros, A., Domínguez-Baleón, C., Zárate, R. V., Campusano, J. M., Narváez-Padilla, V., Reynaud, E., et al. (2021). Nicotine suppresses Parkinson's disease like phenotypes induced by Synphilin-1 overexpression in Drosophila melanogaster by increasing tyrosine hydroxylase and dopamine levels. Sci. Rep. 11, 9579. doi: 10.1038/s41598-021-88910-4
Cerri, S., Mus, L., and Blandini, F. (2019). Parkinson's disease in women and men: what's the difference? J. Parkinsons Dis. 9, 501–515. doi: 10.3233/JPD-191683
Chaillou, T. (2019). Ribosome specialization and its potential role in the control of protein translation and skeletal muscle size. J. Appl. Physiol. 127, 599–607. doi: 10.1152/japplphysiol.00946.2018
Cheong, J. L. Y., de Pablo-Fernandez, E., Foltynie, T., and Noyce, A. J. (2020). The association between type 2 diabetes mellitus and Parkinson's disease. J. Parkinsons Dis. 10, 775–789. doi: 10.3233/JPD-191900
Collier, T. J., Kanaan, N. M., and Kordower, J. H. (2017). Aging and Parkinson's disease: different sides of the same coin? Mov. Disord. 32, 983–990. doi: 10.1002/mds.27037
Cutler, A., Cutler, D., and Stevens, J. (2011). “Random forests,” in Ensemble Machine Learning, eds C. Zhang and Y. Q. Ma, Vol. 45 (New York, NY: Springer), 157–176. doi: 10.1007/978-1-4419-9326-7_5
De Ingeniis, J., Ratnikov, B., Richardson, A. D., Scott, D. A., Aza-Blanc, P., et al. (2012). Functional specialization in proline biosynthesis of melanoma. PLoS ONE. 7, e45190. doi: 10.1371/journal.pone.0045190
Deng, H., Wang, P., and Jankovic, J. (2018). The genetics of Parkinson disease. Ageing Res. Rev. 42, 72–85. doi: 10.1016/j.arr.2017.12.007
Di Stefano, A., and Marinelli, L. (2021). Advances in Parkinson's disease drugs. Biomolecules. (2021) 11. doi: 10.3390/biom11111640
Dorszewska, J., Kowalska, M., Prendecki, M., Piekut, T., Kozłowska, J., Kozubski, W., et al. (2021). Oxidative stress factors in Parkinson's disease. Neural Regen. Res. 16, 1383–1391. doi: 10.4103/1673-5374.300980
Friedman, J., Hastie, T., and Tibshirani, R. (2010). Regularization paths for generalized linear models via coordinate descent. J. Stat. Softw. 33, 1–22. doi: 10.18637/jss.v033.i01
Ganapathi, M., Argyriou, L., Martínez-Azorín, F., Morlot, S., Yigit, G., Lee, T. M., et al. (2020). Bi-allelic missense disease-causing variants in RPL3L associate neonatal dilated cardiomyopathy with muscle-specific ribosome biogenesis. Hum. Genet. 139, 1443–1454. doi: 10.1007/s00439-020-02188-6
Hamaguchi, N., Ihara, S., Ohdaira, T., Nagano, H., Iwamatsu, A., Tachikawa, H., et al. (2007). Pleckstrin-2 selectively interacts with phosphatidylinositol 3-kinase lipid products and regulates actin organization and cell spreading. Biochem. Biophys. Res. Commun. 361, 270–5. doi: 10.1016/j.bbrc.2007.06.132
Hu, M. H., Bauman, E. M., Roll, R. L., Yeilding, N., and Abrams, C. S. (1999). Pleckstrin 2, a widely expressed paralog of pleckstrin involved in actin rearrangement. J. Biol. Chem. 274, 21515–8. doi: 10.1074/jbc.274.31.21515
Hu, Q., and Wang, G. (2016). Mitochondrial dysfunction in Parkinson's disease. Transl. Neurodegener. 5, 14. doi: 10.1186/s40035-016-0060-6
Huang, C., Yang, Y., and Liu, L. (2015). Interaction of long noncoding RNAs and microRNAs in the pathogenesis of idiopathic pulmonary fibrosis. Physiol. Genomics. 47, 463–9. doi: 10.1152/physiolgenomics.00064.2015
Huang, M. L., Hung, Y. H., Lee, W. M., Li, R. K., and Jiang, B. R. (2014). SVM-RFE based feature selection and Taguchi parameters optimization for multiclass SVM classifier. ScientificWorldJournal. 2014, 795624. doi: 10.1155/2014/795624
Iancu, I. V., Anton, G., Botezatu, A., Huica, I., Nastase, A., Socolov, D. G., et al. (2017). LINC01101 and LINC00277 expression levels as novel factors in HPV-induced cervical neoplasia. J. Cell. Mol. Med. 21, 3787–3794. doi: 10.1111/jcmm.13288
Jin Jung, Y., Choi, H., and Oh, E. (2021). Effects of particulate matter and nicotine for the MPP+-induced SH-SY5Y cells: implication for Parkinson's disease. Neurosci. Lett. 765, 136265. doi: 10.1016/j.neulet.2021.136265
Kalia, L. V., and Lang, A. E. (2015). Parkinson's disease. Lancet. 386, 896–912. doi: 10.1016/S0140-6736(14)61393-3
Langfelder, P., and Horvath, S. (2008). WGCNA: an R package for weighted correlation network analysis. BMC Bioinformatics 9, 559. doi: 10.1186/1471-2105-9-559
Latif, S., Jahangeer, M., Maknoon Razia, D., Ashiq, M., Ghaffar, A., Akram, M., et al. (2021). Dopamine in Parkinson's disease. Clin. Chim. Acta. 522, 114–126. doi: 10.1016/j.cca.2021.08.009
Lesnick, T. G., Papapetropoulos, S., Mash, D. C., Ffrench-Mullen, J., Shehadeh, L., de Andrade, M., et al. (2007). A genomic pathway approach to a complex disease: axon guidance and Parkinson disease. PLoS Genet. 3, e98. doi: 10.1371/journal.pgen.0030098
Lindvall, O., Rehncrona, S., Gustavii, B., Brundin, P., Astedt, B., Widner, H., et al. (1988). Fetal dopamine-rich mesencephalic grafts in Parkinson's disease. Lancet 2, 1483–4. doi: 10.1016/S0140-6736(88)90950-6
Liu, D., Fan, Y. B., Tao, X. H., Pan, W. L., Wu, Y. X., Wang, X. H., et al. (2021). Mitochondrial quality control in sarcopenia: Updated overview of mechanisms and interventions. Aging Dis. 12, 2016–2030. doi: 10.14336/AD.2021.0427
Lotankar, S., Prabhavalkar, K. S., and Bhatt, L. K. (2017). Biomarkers for Parkinson's disease: recent advancement. Neurosci. Bull. 33, 585–597. doi: 10.1007/s12264-017-0183-5
Manto, M., and Marmolino, D. (2009). Cerebellar ataxias. Curr. Opin. Neurol. 22, 419–29. doi: 10.1097/WCO.0b013e32832b9897
Mobed, A., Razavi, S., Ahmadalipour, A., Shakouri, S. K., and Koohkan, G. (2021). Biosensors in Parkinson's disease. Clin. Chim. Acta. 518, 51–58. doi: 10.1016/j.cca.2021.03.009
Mullin, S., and Schapira, A. (2015). The genetics of Parkinson's disease. Br. Med. Bull. 114, 39–52. doi: 10.1093/bmb/ldv022
Nachman, E., and Verstreken, P. (2022). Synaptic proteostasis in Parkinson's disease. Curr. Opin. Neurobiol. 72, 72–79. doi: 10.1016/j.conb.2021.09.001
Opara, J., Małecki, A., Małecka, E., and Socha, T. (2017). Motor assessment in Parkinson's disease. Ann. Agric. Environ. Med. 24, 411–415. doi: 10.5604/12321966.1232774
Pan, L., Meng, L., He, M., and Zhang, Z. (2021). Tau in the pathophysiology of Parkinson's disease. J. Mol. Neurosci. 71, 2179–2191. doi: 10.1007/s12031-020-01776-5
Pan-Montojo, F., and Reichmann, H. (2014). Considerations on the role of environmental toxins in idiopathic Parkinson's disease pathophysiology. Transl. Neurodegener. 3, 10. doi: 10.1186/2047-9158-3-10
Poujois, A., and Woimant, F. (2018). Wilson's disease: a 2017 update. Clin. Res. Hepatol. Gastroenterol. 42, 512–520. doi: 10.1016/j.clinre.2018.03.007
Robin, X., Turck, N., Hainard, A., Tiberti, N., Lisacek, F., Sanchez, J. C., et al. (2011). pROC: an open-source package for R and S+ to analyze and compare ROC curves. BMC Bioinformatics 12, 77. doi: 10.1186/1471-2105-12-77
Seong, H. A., and Ha, H. (2019). Thr55 phosphorylation of p21 by MPK38/MELK ameliorates defects in glucose, lipid, and energy metabolism in diet-induced obese mice. Cell Death Dis. 10, 380. doi: 10.1038/s41419-019-1616-z
Su, X., and Federoff, H. J. (2014). Immune responses in Parkinson's disease: interplay between central and peripheral immune systems. Biomed Res. Int. 2014, 275178. doi: 10.1155/2014/275178
Suykens, J. A. K., and Vandewalle, J. J. N. P. L. (2004). Least squares support vector machine classifiers. Neural Process. Lett. 9, 293–300.
Tibshirani, R. (1996). Regression shrinkage and selection via the lasso. J. R. Stat. Soc. Series B Methodol. 58, 267–288. doi: 10.1111/j.2517-6161.1996.tb02080.x
Vascellari, S., and Manzin, A. (2021). Parkinson's disease: a prionopathy? Int. J. Mol. Sci. 22. doi: 10.3390/ijms22158022
Vivekanantham, S., Shah, S., Dewji, R., Dewji, A., Khatri, C., Ologunde, R., et al. (2015). Neuroinflammation in Parkinson's disease: role in neurodegeneration and tissue repair. Int. J. Neurosci. 125, 717–25. doi: 10.3109/00207454.2014.982795
Wang, C., Zhou, C., Guo, T., Huang, P., Xu, X., Zhang, M., et al. (2022). Association between cigarette smoking and Parkinson's disease: a neuroimaging study. Ther. Adv. Neurol. Disord. 15, 17562864221092566. doi: 10.1177/17562864221092566
Wang, J., He, Z., Sun, B., Huang, W., Xiang, J., Chen, Z., et al. (2021). Pleckstrin-2 as a prognostic factor and mediator of gastric cancer progression. Gastroenterol. Res. Pract. 2021, 5527387. doi: 10.1155/2021/5527387
Wang, Y., Li, Y. M., Baitsch, L., Huang, A., Xiang, Y., Tong, H., et al. (2018). Correction: MELK is an oncogenic kinase essential for mitotic progression in basal-like breast cancer cells. eLife. 7:e36414. doi: 10.7554/eLife.36414
Yildirim, M., Oztay, F., Kayalar, O., and Tasci, A. E. (2021). Effect of long noncoding RNAs on epithelial-mesenchymal transition in A549 cells and fibrotic human lungs. J. Cell. Biochem. 122, 882–896. doi: 10.1002/jcb.29920
Yu, G., Wang, L. G., Han, Y., and He, Q. Y. (2012). clusterProfiler: an R package for comparing biological themes among gene clusters. OMICS. 16, 284–7. doi: 10.1089/omi.2011.0118
Zhang, J., Huang, C., Liu, Z., Ren, S., Shen, Z., Han, K., et al. (2022). Screening of potential biomarkers in the peripheral serum for steroid-induced osteonecrosis of the femoral head based on WGCNA and machine learning algorithms. Dis. Markers. 2022, 2639470. doi: 10.1155/2022/2639470
Keywords: Parkinson's disease, identification, bioinformatics analysis, hub genes, GEO
Citation: Yang Y, Wang Y, Wang C, Xu X, Liu C and Huang X (2022) Identification of hub genes of Parkinson's disease through bioinformatics analysis. Front. Neurosci. 16:974838. doi: 10.3389/fnins.2022.974838
Received: 22 June 2022; Accepted: 15 September 2022;
Published: 08 November 2022.
Edited by:
Patrick Lewis, Royal Veterinary College (RVC), United KingdomReviewed by:
Megha Murthy, University College London, United KingdomCopyright © 2022 Yang, Wang, Wang, Xu, Liu and Huang. This is an open-access article distributed under the terms of the Creative Commons Attribution License (CC BY). The use, distribution or reproduction in other forums is permitted, provided the original author(s) and the copyright owner(s) are credited and that the original publication in this journal is cited, in accordance with accepted academic practice. No use, distribution or reproduction is permitted which does not comply with these terms.
*Correspondence: Xintao Huang, eGludGFvaEBob3RtYWlsLmNvbQ==
†These authors have contributed equally to this work and share first authorship
Disclaimer: All claims expressed in this article are solely those of the authors and do not necessarily represent those of their affiliated organizations, or those of the publisher, the editors and the reviewers. Any product that may be evaluated in this article or claim that may be made by its manufacturer is not guaranteed or endorsed by the publisher.
Research integrity at Frontiers
Learn more about the work of our research integrity team to safeguard the quality of each article we publish.