- 1Neuropsychiatric Genetics, Trinity Center for Health Sciences, Trinity Translational Medicine Institute, St James Hospital, Dublin, Ireland
- 2Department of Brain and Cognitive Sciences, Simons Center for the Social Brain, Picower Institute for Learning and Memory, MIT, Cambridge, MA, United States
- 3Department of Neurology, Rosamund Stone Zander Translational Neuroscience Center, Boston Children’s Hospital and Harvard Medical School, Boston, MA, United States
- 4Department of Human Genetics, Emory University School of Medicine, Atlanta, GA, United States
- 5Department of Neurology, Boston Children’s Hospital, Boston, MA, United States
- 6Trinity College Institute of Neuroscience, Trinity College Dublin, Dublin, Ireland
- 7FutureNeuro, The SFI Research Centre for Chronic and Rare Neurological Diseases, Dublin, Ireland
Rett syndrome (RTT) is a devastating neurodevelopmental disorder without effective treatments. Attempts at developing targetted therapies have been relatively unsuccessful, at least in part, because the genotypical and phenotypical variability of the disorder. Therefore, identification of biomarkers of response and patients’ stratification are high priorities. Administration of Insulin-like Growth Factor 1 (IGF-1) and related compounds leads to significant reversal of RTT-like symptoms in preclinical mouse models. However, improvements in corresponding clinical trials have not been consistent. A 20-weeks phase I open label trial of mecasermin (recombinant human IGF-1) in children with RTT demonstrated significant improvements in breathing phenotypes. However, a subsequent randomised controlled phase II trial did not show significant improvements in primary outcomes although two secondary clinical endpoints showed positive changes. To identify molecular biomarkers of response and surrogate endpoints, we used RNA sequencing to measure differential gene expression in whole blood samples of participants in the abovementioned phase I mecasermin trial. When all participants (n = 9) were analysed, gene expression was unchanged during the study (baseline vs. end of treatment, T0–T3). However, when participants were subclassified in terms of breathing phenotype improvement, specifically by their plethysmography-based apnoea index, individuals with moderate-severe apnoea and breathing improvement (Responder group) displayed significantly different transcript profiles compared to the other participants in the study (Mecasermin Study Reference group, MSR). Many of the differentially expressed genes are involved in the regulation of cell cycle processes and immune responses, as well as in IGF-1 signalling and breathing regulation. While the Responder group showed limited gene expression changes in response to mecasermin, the MSR group displayed marked differences in the expression of genes associated with inflammatory processes (e.g., neutrophil activation, complement activation) throughout the trial. Our analyses revealed gene expression profiles associated with severe breathing phenotype and its improvement after mecasermin administration in RTT, and suggest that inflammatory/immune pathways and IGF-1 signalling contribute to treatment response. Overall, these data support the notion that transcript profiles have potential as biomarkers of response to IGF-1 and related compounds.
Introduction
Rett syndrome (RTT) is an X-linked neurodevelopmental disorder that affects predominantly females (∼1/9,000–1/10,000) (Kaufmann et al., 2016). The diagnosis of RTT is clinical, taking into account a phenotypic spectrum of severity. The four core diagnostic criteria that define classic/typical versus variant/atypical RTT are partial or complete loss of hand function, partial or complete loss of spoken language, impaired gait, and presence of repetitive hand movements termed hand stereotypies (Neul et al., 2010). All four criteria are required for the diagnosis of classic RTT, while atypical RTT is diagnosed when at least 2 of these 4 main criteria are present plus 5 of 11 supportive criteria (i.e., breathing disturbances, bruxism when awake, impaired sleep, tone abnormalities, peripheral vasomotor disturbances, scoliosis/kyphosis, growth retardation, small cold hands and feet, inappropriate laughing/screaming spells, diminished pain response, and intense eye communication) (Neul et al., 2010). These supportive criteria are also prevalent in classic RTT (Percy et al., 2010).
Rett syndrome is usually associated with a pathogenic mutation in the methyl-CpG binding protein 2 (MECP2) gene, particularly in those individuals with the classic presentation (Neul et al., 2010). Genotype-phenotype correlations have led to identifying groups of MECP2 mutations with different levels of severity (Cuddapah et al., 2014). MECP2 encodes the methyl CpG-binding protein 2 (MeCP2), a chromatin binder and transcription regulator (Ip et al., 2018). Abnormal expression of MeCP2 results in impaired brain development and function associated with disruption in synaptic plasticity (Kaufmann et al., 2005; Asaka et al., 2006; Blackman et al., 2012). The discovery of mutations in MECP2 (Amir et al., 1999) as the genetic abnormality associated with most cases of RTT, has led to the generation of mutant mouse models that replicate many features of the disorder (Chen et al., 2001; Guy et al., 2001; Nguyen et al., 2013; Ross et al., 2016). These mutant mouse models have become a valuable resource for the study of the molecular and cellular mechanisms underlying RTT, and for testing candidate treatments for the disorder (Katz et al., 2012). Management of RTT is mainly symptomatic (Kaufmann et al., 2016; Leonard et al., 2017); therefore, the discovery of disease-modifying therapies in models of RTT has become a priority in the field. Preclinical studies in mice have already identified several promising drugs, some of which have moved to clinical development (Tropea et al., 2009; Castro et al., 2014; Park et al., 2014; Kaufmann et al., 2019). One of the best studied candidate drugs is Insulin-like growth factor 1 (IGF-1).
Insulin-like growth factor 1 is a growth factor and signalling molecule that is involved in growth, maturation, and ageing. In the CNS, IGF-1 plays a role in developmental and mature brain synaptic plasticity (Dyer et al., 2016). IGF-1’s role in neuronal development and function presents multiple similarities to that of BDNF; however, the latter signalling molecule has limited therapeutic potential because of its inability to cross the blood-brain barrier. More recent evidence suggests that IGF-1 signalling is implicated in metabolic, homeostatic processes, which underlie synaptic plasticity and are disrupted in RTT (De Felice et al., 2014; Banerjee et al., 2016; Gazit et al., 2016; Neul et al., 2020; Crivellari et al., 2021). IGF-1 is naturally cleaved by proteases into the small tripeptide Glycine-Proline-Glutamic acid (GPE) and the larger Des (1–3) IGF-1 peptide. GPE has neuroprotective properties through different modulatory processes to those of IGF-1 (Guan and Gluckman, 2009). Both full-length IGF-1 and GPE have been shown to ameliorate features of relevance to RTT in a genetic mouse model of the disorder (Tropea et al., 2009; Castro et al., 2014). These encouraging results have led to clinical trials in RTT using either a recombinant human form of IGF-1 (i.e., rhIGF-1, mecasermin) or a modified GPE (i.e., trofinetide). Both mecasermin and trofinetide have shown safety and tolerability and initial evidence of efficacy (Pini et al., 2012, Pini et al., 2016b; Khwaja et al., 2014; Glaze et al., 2017, 2019; O’Leary et al., 2018).
The first clinical trial of mecasermin, an open label phase I pharmacokinetic and exploratory efficacy study, demonstrated that mecasermin reached the CNS compartment following a non-linear kinetics with greater distribution in the peripheral compartment (Khwaja et al., 2014). In terms of efficacy, several parameters showed improvements during a 20-weeks open label extension (OLE) of the pharmacokinetic segment. Improvements in measures of anxiety and mood during the OLE were associated with reversal of right frontal alpha band asymmetry on EEG, a biomarker of these behavioural abnormalities. Cardiorespiratory measures showed that apnoea, a characteristic and severe breathing abnormality in RTT, also improved markedly. Since these assessments were carried out by plethysmography, an objective methodology, these were considered the most meaningful clinical findings of the study (Khwaja et al., 2014). A follow up larger randomised placebo-controlled phase II trial, did not replicate these findings. However, secondary endpoints measuring stereotypic behaviour and social communication demonstrated significant improvements (O’Leary et al., 2018). One of several possible explanations for the discrepancy between the two mecasermin studies is that severity of breathing abnormality, specifically a minimum apnoea index, was not part of the inclusion criteria. This resulted in that only 14 out of 30 participants in the phase II trial presented at baseline an apnoea index high enough to demonstrate treatment efficacy.
The failure in consistently demonstrating mecasermin’s efficacy in children with RTT, in conjunction with the continuous interest in trofinetide - now reporting encouraging results following adult and paediatric trials (Glaze et al., 2017, 2019) - emphasises the importance of further characterising the mechanisms underlying therapeutic responses to IGF-1-related compounds and the need for identifying biomarkers linked to clinical improvements. The present study aimed at delineating molecular profiles associated with therapeutic responses to mecasermin in children with RTT, on the basis of data from the mecasermin phase I trial. Because this trial included two periods of drug administration and their intervening washout period, it offered the possibility of evaluating the dynamics of gene expression in response to mecasermin. For this purpose, we analysed RNA profiles on whole blood samples and correlated them with apnoea responder status (Khwaja et al., 2014). We chose the latter as measure of efficacy because of its objective nature. A link between two sets of objective parameters would provide stronger evidence for molecular factors underlying clinical responses to mecasermin and, probably, also other IGF-1-related compounds. In terms of the use of RNA profiles as biomarkers, we contemplated both that RNA profiles could serve as predictors of response (i.e., baseline levels) or as surrogate endpoints (i.e., change in levels between baseline and end of treatment). Whole blood is particularly useful in this respect due to availability and accessibility of the tissue. MECP2 is considered to be widely expressed in peripheral tissues, where it has even been shown to contribute to certain RTT symptoms, including hypoactivity, exercise fatigue, and bone abnormalities (Song et al., 2014; Ross et al., 2016). Moreover, a recent study of Mecp2 mutant mice revealed that some genes differentially expressed in blood are also altered in brain (Sanfeliu et al., 2019). These factors indicate that whole blood is a particularly relevant sample source for the aims of our study.
We found that individuals with RTT, severe breathing abnormalities and positive response to mecasermin, as shown by an improved apnoea index, had molecular signatures of relevance to their phenotype and treatment that can be distinguished before drug administration and, to a lesser extent, at later timepoints. This finding could assist in the design and analysis of future trials with mecasermin and other IGF-1-related compounds.
Materials and Methods
Outcome Measures and Definition of Responder
Multiple outcome measures were used for evaluating efficacy. They included a wide range of clinician- and caregiver-rated neurobehavioural assessments, several of them developed or adapted for RTT [Clinical Global Impression scales (CGI-S, CGI-I), Parent-Targetted Symptom Visual Analogue Scale (PTSVAS), Mullen Scales of Early Learning (MSEL), the Clinical Severity Scale (CSS), the Motor–Behavioural Assessment (MBA), the Rett Syndrome Behaviour Questionnaire (RSBQ), and the Anxiety, Depression, and Mood Scale (ADAMS)], and automated cardiorespiratory measures. The latter consisted of time synchronised chest respiratory inductive plethysmography and electrocardiography. While neurobehavioural assessments were performed only during the OLE, cardiorespiratory measures were collected throughout the trial. Using a wireless data-acquisition plethysmography device (BioRadio, Great Lakes Neurotechnologies, Independence, OH, United States), breathing abnormality profiles with a focus on breath holding were determined for all participants. Clinically significant apnoea, which was defined as apnoeic episodes >10 s in length, was present in five participants. Apnoea was graded as moderate–severe when these apnoeic episodes were > 5 per hour, a pattern present in 4 participants at the beginning of the study, before the multiple ascending dose (MAD)/pharmacokinetics period (Figure 1 and Table 1). All these four participants experienced a decrease in apnoea severity to mild (<5 episodes per hour) by the end of the OLE (Table 1). Details on outcome measures can be found in Khwaja et al. (2014). For the purpose of evaluating therapeutic responses, Apnoea Responder was defined as a participant who had a decrease in apnoea frequency >50% or a reduction to ≤5 apnoeic episodes per hour (the four participants mentioned above). By default, the five participants who did not fulfil the criteria for Responder (R) were assigned to the Mecasermin Study Reference (MSR) group.
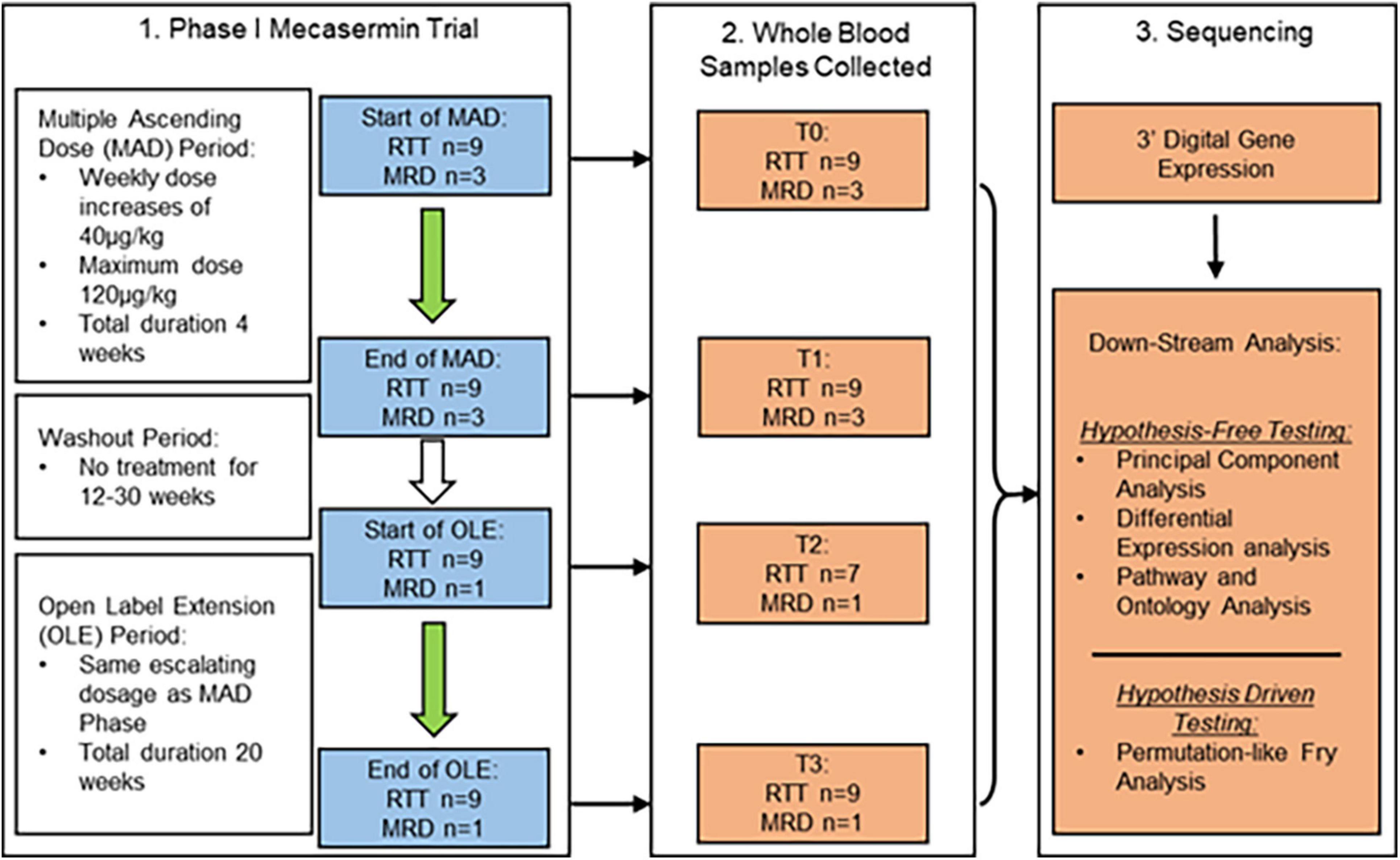
Figure 1. Study design and dosing schedule of phase I mecasermin trial in RTT. All participants (n = 12) were included in the multiple ascending dose (MAD) period while only those with RTT (n = 9; n = 7 at T2) and one with MRD progressed onto the open label extension (OLE) component. Participants were administered mecasermin twice daily by subcutaneous injection. Time points when blood sampling was performed are denoted T0 (start of MAD), T1 (end of MAD), T2 (start of OLE), and T3 (end of OLE). Whole blood samples were used for 3′-Digital Gene Expression (3′-DGE) sequencing.
Of the total 12 clinical trial participants, nine had a diagnosis of classic RTT (participants #1, #4, #5, #6, #7, #8, #9, #11, and #12) while three participants had a diagnosis of MECP2 related disorder (MRD) (participants #2, #3, and #10). Participants with MRD are characterised by having pathogenic MECP2 mutations, some of them also identified in individuals with RTT, but do not display a clinical presentation compatible with either typical or atypical RTT. They were included in the trial (Khwaja et al., 2014) in order to determine whether treatment with mecasermin could be effective in most phenotypes associated with MECP2 mutations. However, since participants with MRD did not present a breathing phenotype and were not included in the OLE period, their samples were analysed in this molecular study only as pre-treatment reference data. MECP2 mutations of each participant with classic RTT are described in Table 2. All RTT participants were included in both the MAD and OLE treatment periods, but only 1 MRD participant (participant #10) was included in the OLE phase (see Figure 1). Mutations in participants with RTT were classified according to their profile of severity as severe (R168X, R255X, deletions and insertions), intermediate (T158M) or mild (other point mutations), following the report by Cuddapah et al. (2014).
RNA Collection and Sequencing
RNA from whole blood samples was collected and extracted using PAXgene Blood RNA tubes (BD Biosciences, Radnor, PA, United States), and analysed by 3′-Digital Gene Expression (3′-DGE). Sequencing was performed at the Massachusetts Institute of Technology (MIT)’s BioMicro Center, an integrated genomics core facility. 3′-DGE was adapted from Soumillon et al. (2014) using a tag-based transcriptome sequencing method, which provides cost-effective means of generating expression data for characterising major patterns in heterogeneous samples (Soumillon et al., 2014). The sequencing read data was then combined with barcode information in a FASTQ format and mapped onto the Hg19 reference sequence using BWA. Per-gene count quantification was conducted with the End Sequence Analysis Toolkit (ESAT) for downstream differential gene expression analysis (Derr et al., 2016). Raw data will be available at the public repository Gene Expression Omnibus (GSE198856). Samples from two participant (participant #5 and participant #12) were not collected at T2 (beginning of OLE); these missing data were not imputed in the analyses.
Differential Gene Expression Analyses
Differential gene expression was quantified using EdgeR, a popular software specifically designed for analysing sequencing data from small sample sizes (Robinson et al., 2009). EdgeR was operated on an R studio environment using R statistical programming language (Venables et al., 1995). EdgeR uses an empirical Bayes estimation, based on a negative binomial model, and a quasi-likelihood F test (QLFT) to determine differential expression. QLFT is the preferable choice for comparing gene expression on small samples, as it better reflects the uncertainty of estimating gene expression dispersion (i.e., variability), resulting in a lower error rate (Chen et al., 2017).
Using QLFT, we conducted two different types of analyses to examine the drug’s effect on gene expression across the trial: (1) time-point and (2) responder status comparisons. Based on sampling, there were six possible time-point comparisons (T0–T1, T0–T2, T0–T3, T1–T2, T1–T3, and T2–T3), which were conducted on all RTT sample sets (n = 9 at T0, T1, and T3; n = 7 at T2). On the other hand, responder status comparisons contrasted R (n = 4 at T0, T1, and T3; n = 3 at T2) and MSR (n = 5 at T0, T1, and T3; n = 4 at T2) groups at each of time point.
Significant gene sets identified by these comparisons were used to conduct pathway analysis using Reactome1 and Ontology analysis using Panther’s Gene Ontology database (GO).2 The gene sets were entered into these online tools filtering out any unidentified genes. Both Reactome and GO analysis use over-representation analysis to determine if a given gene set is over- or under-represented in a given pathway or ontology, with respect to a hypothetical random selection (Fabregat et al., 2018; The Gene Ontology Consortium, 2000, 2021). In both GO and Reactome analyses, significant pathways were considered those with Entities false discovery rate (FDR) values < 0.05, and the significant pathways were validated with Fry() to eliminate false positives caused by correlations between genes in the set. This function, uses operations (analogous to fractional permutations) on the gene sets to determine if a gene set was differentially expressed across randomly generated comparison sets (Wu et al., 2010; Chen et al., 2016; Muley et al., 2020; Grisaru-Tal et al., 2021). In summary, Fry(), by cross-checking the selected gene sets shuffling the data between the two compared groups, controls for false positives. This analysis was also employed to validate the pathways and ontologies that had been identified using the hypothesis-free methods. Only pathways validated with Fry() are reported in this study.
Using edgeR, two categories of analyses were performed:
(A) Hypothesis-free testing: We evaluated the differential expression of all the annotated genes expressed in all the participants (26,116 genes in total). This approach was used to detect changes in gene expression without any bias from previous studies or the literature. This type of analysis is, however, curtailed by the requirement of a high FDR due to the large number of tested genes. Hypothesis-free testing was conducted using the QLFT() function.
(B) Hypothesis-driven testing: We also used a hypothesis-driven (HD) approach for testing specific genes, based on previous research or the literature. Specifically, we tested five main classes of gene sets that have been associated to RTT pathophysiology: IGF-1 and BDNF pathways; metabolic homeostatic mechanisms, including mitochondria, protein ubiquitination, and chromatin mediated processes (Pecorelli et al., 2013); abnormal inflammatory responses (Maezawa and Jin, 2010; O’Driscoll et al., 2013; Lin et al., 2016; Zhao et al., 2017); pathways linked to the apnoea phenotype (e.g., monoamine metabolism) (Viemari et al., 2005; Toward et al., 2013; Vogelgesang et al., 2018); and autism spectrum disorder (ASD) iPSCs-IGF-1 induced genomic changes (Linker et al., 2020). To test whether these gene sets were differentially expressed across the time-point comparisons or the R vs. MSR comparisons, we used edgeR’s Fry function. Gene sets analysed with Fry were further validated by permutation analyses (Bach et al., 2020), which take into consideration control pathways with the same size of the HD gene sets and use the distribution of the p-value of the controls and the HD gene sets to confirm statistical significance. Only HD gene-sets with p-values falling into the top 5 percentile of the p-value distribution are reported in this study.
Statistical Analysis and Graphing Software
Principal component analysis (PCA) was conducted using the built-in prcomp() function in R studio, by analysing sample counts at T0, applying scaling and centring of data. For graphical representations, we used the ggplot2 package, also in R studio. All the tests were corrected for multiple testing by FDR, and differences were defined as significant with a p-value < 0.05. Analyses examining the relationship between PCs and variables of clinical significance were performed by the non-parametric Spearman rank correlation test.
Results
Cohort and Trial Design
The study is regulated by Institutional Review Board protocol number 10-08-0403, and by MTA agreement #18081. The cohort in the phase I open label mecasermin trial (Khwaja et al., 2014) included 9 participants with classic RTT and 3 with MECP2-related disorders [MRD; non-RTT clinical presentations in individuals with MECP2 mutations (Neul et al., 2010)]. Details about the cohort, including individual MECP2 mutations in participants with RTT, can be found in Table 2 and in the original publication on the trial by Khwaja et al. (2014). The study consisted of two different components: a 4-week multiple ascending dose (MAD) period and a 20-week open label extension (OLE) period. The MAD and OLE periods were separated by a variable interval of 12–30 weeks. The MAD period was focussed on assessment of safety and collection of serial pharmacokinetic data. The goal of the OLE period was to extend the evaluation of mecasermin safety and to conduct a preliminary assessment of the drug’s efficacy. Both MAD and OLE periods began with a dose of 40 μg/kg, which was increased by 40 μg/kg per week to a maximum dose of 120 μg/kg. Whole blood samples were taken from the participants at four different time points denoted T0–T3 (T0 and T1, corresponding to the beginning and end of the MAD, respectively; and T2 and T3, corresponding to the beginning and end of the OLE, respectively). Of note, for participants #5 and #12 samples were not obtained at T2. At T2 and T3 (OLE period), in addition to the cardiorespiratory evaluations described below, multiple neurobehavioral assessments were also performed (details in Khwaja et al., 2014). A schematic of the dosing schedule and study design are shown in Figure 1.
Gene Expression Profiles in Participants With Rett Syndrome and MECP2 Related Disorder
The first treatment period, the Multiple Ascending Dosage (MAD) period, intended to determine the pharmacokinetics of mecasermin. The second treatment period, the Open Label Extension (OLE), was an additional treatment segment intending to obtain additional information on safety and preliminary data on efficacy. The MAD period included, in addition to the nine participants with classic RTT, three girls with MRD. MECP2 mutations identified in each participant with RTT are described in Table 2.
We found no significant differential gene expression, calculated with edgeR, when comparing MRD and RTT groups at T0. Because the T1, T2, and T3 time points had only one MRD sample, participants with MRD were not considered for further analysis. Table 3 presents the changes in gene expression in the entire RTT cohort throughout the trial, by comparing the different sequential time points.
Gene Expression Profiles of Responder and Mecasermin Study Reference Groups at Baseline
All analyses described in this and the following sections, used only data from the participants with RTT. We used PCA to delineate the relationship between gene expression profiles at baseline (T0) in R (participants #7, #9, #11, and #12) versus MSR (participants #1, #4, #5, #6, and #8) groups. Figure 2 shows a plot of the largest principal components (PC1 and PC2) at T0 which accounted, respectively, for 44.5 and 13.1% of the variance. R (n = 4, red) and MSR (n = 5, blue) groups were divided by PC1; R to the right and MSR to the left of 0 on the PC1 axis. Participants in the MSR group were relatively close to each other with exception of one sample (participant #6), varying mainly in PC2, while the R group was more dispersed. Interestingly, although that MSR participant did not meet our stringent criteria for responder, she had a mild apnoea phenotype with episodes of the same length as those in the R group and showed an improvement in her apnoea index at the end of the OLE. As reference, the PC profile of MRD participants is intermediate between the R and MSR groups. Thus, this gene expression variance analysis showed that R and MSR groups segregated from each other before treatment with mecasermin along PC1, which is consistent with their clinical profiles and outcome. Analyses examining the relationship between gene expression profiles, measured by PC1, and variables of clinical significance, found no significant relationship between PC1 and mutation severity category (Rho = 0.52, p = 0.15). In contrast, we found a strong correlation between PC1 and apnoea index at baseline. When considering all the participants in the study (n = 9), the correlation was significant (Rho = 0.94, p = 0.00016). However, when considering only participants with apnoea index > 0 (n = 5), although the correlation was strong (Rho = 0.90), the test failed to reach significance (p = 0.08; Supplementary Figure 1).
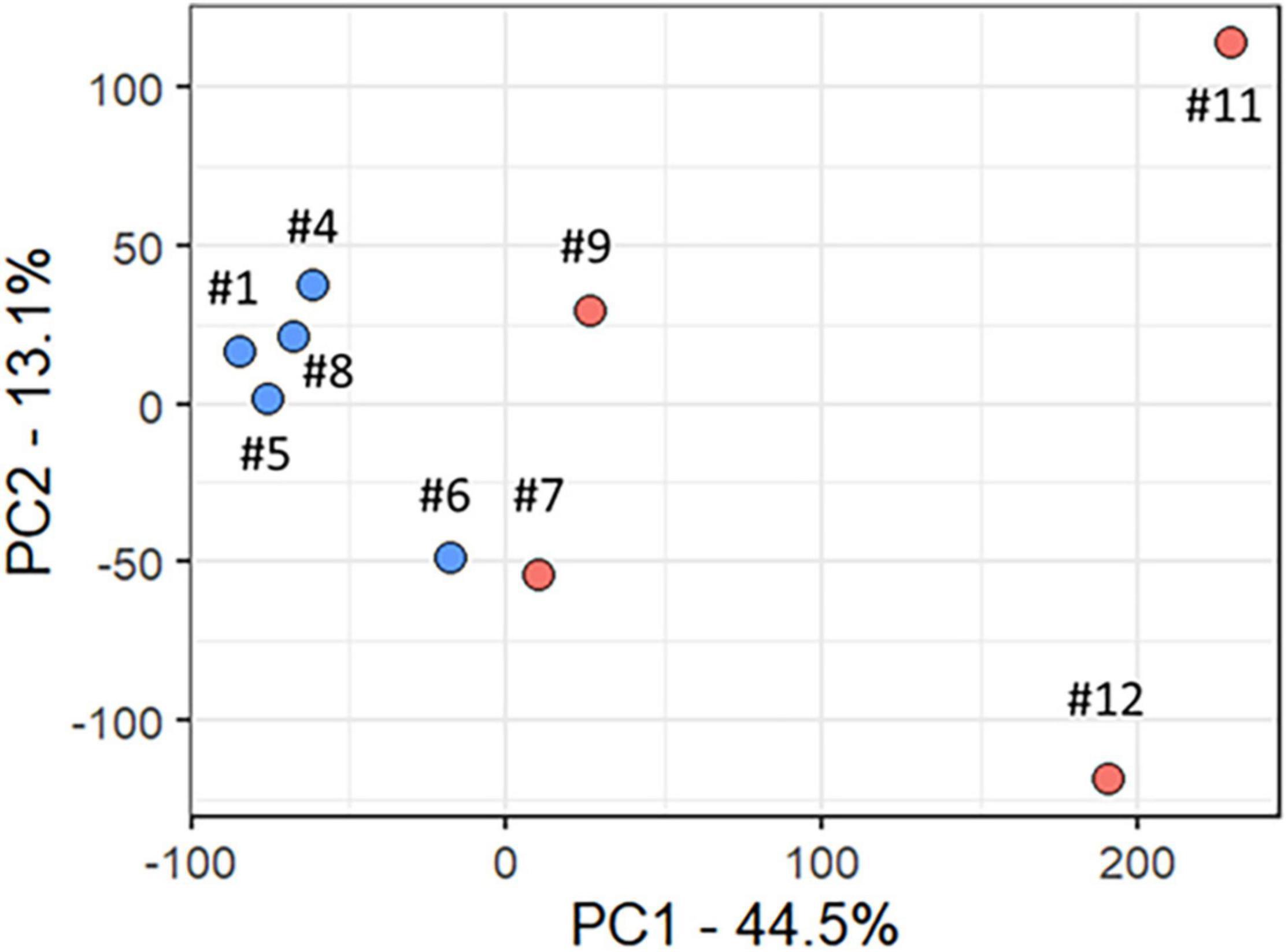
Figure 2. Principal component analysis of baseline transcript profiles in participants with RTT. Scatter plot of Principal Components 1 and 2 (PC1 and PC2), which accounted, respectively, for 44.5 and 13.1% of total variance. The Responder group is shown in red and the Mecasermin Study Reference group in blue. Note that participant #6 did not meet all criteria for the Responder group; however, she also showed an improvement in her apnoea index at the end of the trial.
Gene Expression Profiles of Responder and Mecasermin Study Reference Groups Throughout the Trial
In order to determine how the R and MSR groups reacted differentially to IGF-1 treatment, we performed gene expression analysis at different time-points throughout the trial. We compared gene expression between the two groups at T0, T1, T2, and T3. Such comparisons revealed a large number of changes (Supplementary Table 1) with the greatest difference in gene expression observed at T0, as presented in detail in the previous section, and relatively higher overall expression in the R group with respect to the MSR group (3,693 upregulated, 221 downregulated). Similar patterns, with relatively higher expression in R versus MSR groups, were also observed at T1 (113 upregulated, 34 downregulated) and T2 (66 upregulated, 16 downregulated). However, the direction of these differences was reversed at T3 (2 upregulated, 28 downregulated). Thus, the analyses revealed decreasing differential gene expression between the R and MSR groups, from baseline to end of treatment (T0–T3), confirming the existence of different “molecular” subcohorts at the beginning of the trial. The two groups, identified by their genomic profiles, appeared to respond differently to mecasermin treatment, with the MSR group showing more changes (Table 4).
In order to identify pathways differentially regulated in R versus MSR groups at different periods of the trial, the list of differentially expressed genes was input into Reactome (pathway database) and Gene Ontology (GO). Results were controlled for false positives using Fry() (EdgeR). GO found significantly enriched ontologies only at T0 (all upregulated in the R group). The top 50 validated GO gene sets are depicted in Table 5, while the full list is reported in Supplementary File 2. Validated pathways in Reatcome, at different time points, are shown below in Table 6.
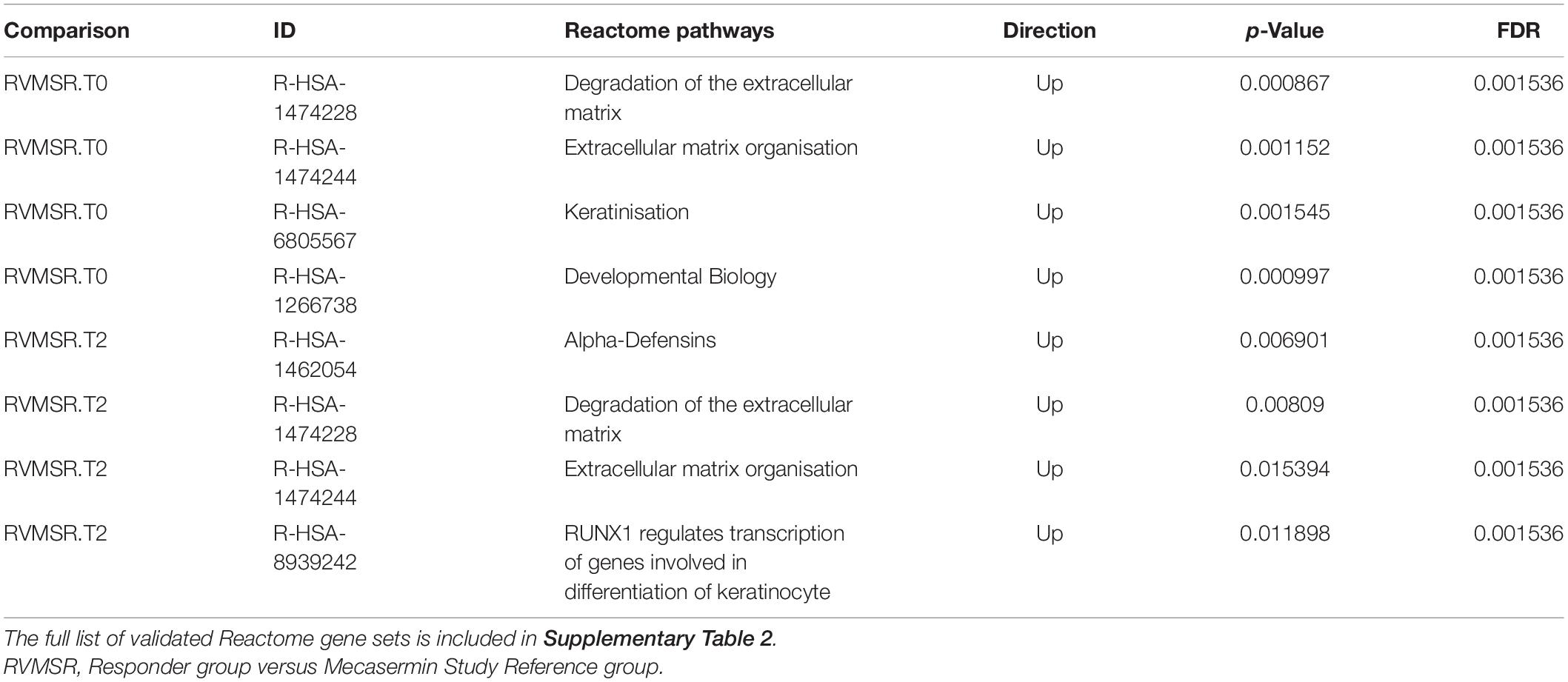
Table 6. Validated differentially regulated Reactome pathways in R and MSR groups throughout the trial.
Preliminary Assessment of Mechanisms Underlying Response to Mecasermin in Rett Syndrome: Hypothesis-Free Analysis
In order to ascertain mechanisms underlying the response to mecasermin in RTT, we examined differential gene expression in the entire RTT cohort (n = 9; n = 7 at T2) at different intervals (T0–T1, T0–T2, T0–T3, T1–T2, T1–T3, and T2–T3). There were no significant differences between T0 and T1 (MAD period), T0 and T2, T0 and T3 (entire trial), or T1 and T3. During the off-treatment period (T1–T2), two related genes TMEM176A and TMEM176B showed a reduction in levels (Table 3). In the interval corresponding to the OLE period (T2–T3), we found 4 differentially expressed genes (i.e., increased expression): ERVMER34-1, RRM2, CENPF, and the abovementioned TMEM176B. Thus, mecasermin treatment induced limited changes in gene expression that were mainly present during the OLE period (Supplementary Material), most likely due to the heterogeneity of the population. Therefore, we included separate comparisons of gene expression patterns across the different study intervals in the R (n = 4; n = 3 at T2) and MSR (n = 5; n = 4 at T2) groups.
The R group showed only two differentially expressed genes across all study intervals: HLA-DRB5 and SMCR5. HLA-DRB5 encodes the major histocompatibility complex class II DRβ5, SMCR5 is the non-coding Smith-Magenis Syndrome Chromosome Region Candidate 5 gene. In the R group, HLA-DRB5 decreased significantly from T0 to T1 (MAD period) and from T0 to T2, but it increased significantly during the OLE (T2–T3). In contrast, in the MSR group HLA-DRB5 expression decreased only during the OLE (T2–T3).
Many of the genes differentially expressed along the study in the MSR group have roles in the immune system. Among these immune function genes are the TMEM176 genes, which are associated with maintenance of dendritic cell immaturity (Condamine et al., 2010). TMEM176A is differentially expressed in the MSR group between T1 and T2 (p < 0.01), while TMEM176B is differentially expressed between T1 and T2 (p < 0.01) and T2 and T3 (p < 0.02). Fold change levels are reported in Supplementary Table 1. The MSR group also showed increases in several defensin-α genes. Defensins are antimicrobial and cytotoxic peptides involved in host defence, which are stored in granules (azurophils). During phagocytosis, these granules fuse into phagocytic vacuoles and contribute to antimicrobial response (Ganz, 2003). The increases in defensin gene expression mainly represent changes during the OLE (T2 to T3), but also between T0 and T3 and T1 and T3 (all p-values < 0.001; for detailed fold changes see Supplementary Table 1).
Overall, the differential gene expression analyses between different periods of the trial revealed a significant change only in the MSR group. At all intervals, we found several differentially expressed genes: 13 genes in T0–T1, 24 genes in T0–T2, 28 genes in T0–T3, 49 genes in T1–T2, 27 genes in T1–T3, and 37 genes in T2–T3. Table 4 summarises interval comparisons in all three groups under analysis: all participants with RTT, R group, and MSR group. A full list of results is included in Supplementary Table 1.
All the differentially expressed genes identified in the entire cohort were also found in the MSR group, suggesting that the differences in the entire cohort were mainly driven by the former. Therefore, all subsequent analyses of differential gene expression were carried out separately in the R and MSR groups. We used the differentially expressed genes identified in these two groups to perform pathway and ontology analyses using Reactome and GO. The significant results from these analyses were then validated using the edgeR’s Fry function. The validated results, all in the MSR group, are: Mitotic Cell Cycle Process (GO:1903047) upregulated at T3 versus T2, Non-sense mediated Decay (NMD) (R-HSA-927802), downregulated at T2 versus T1, and NMD enhanced by exon Junction Complex (R-HSA-975957) upregulated at T2 versus T1.
Preliminary Assessment of Mechanisms Underlying Response to Mecasermin in Rett Syndrome: Hypothesis-Driven Testing
We then tested if mechanisms previously associated to RTT pathogenesis were different between R and MSR groups, and if they were modulated by the administration of Mecasermin. We retrieved the corresponding gene sets in GO and then tested the hypothesis using permutation analyses in R (edgeR’s Fry function). This analysis showed significant results in R versus MSR comparisons, at T0, T1, and T2.
All the results of hypothesis-driven testing are included in Table 7. The analysis reveals that most of the gene sets were differentially expressed between R and MSR groups at T0, except for the BDNF receptor signalling pathway. At T1, which corresponded to the end of the MAD period, the IGF-1 receptor signalling pathway was differentially expressed. At T2, a number of signalling pathways were differentially expressed including PI3K, BDNF receptor, dopamine receptor, and serotonin receptor. At T3, there were no significantly different gene sets when R and MSR groups were compared. Results of R vs. MSR group analyses are shown in Table 7.
The same sets of genes were then examined within each group (R and MSR) at different intervals. We found that in the R group only the IGF-1 receptor signalling pathway was differentially expressed from T1 to T2, while in the MSR group Response to Chronic IGF-1 treatment in ASD iPSCs was significantly different between T1 and T2 (Table 7).
In summary, both hypothesis-driven and hypothesis-free analyses demonstrated that the RTT cohort was not homogeneous at baseline, and in its molecular response to mecasermin treatment. Figure 3 summarises the results of the gene expression analyses in relationship with changes in the apnoea index.
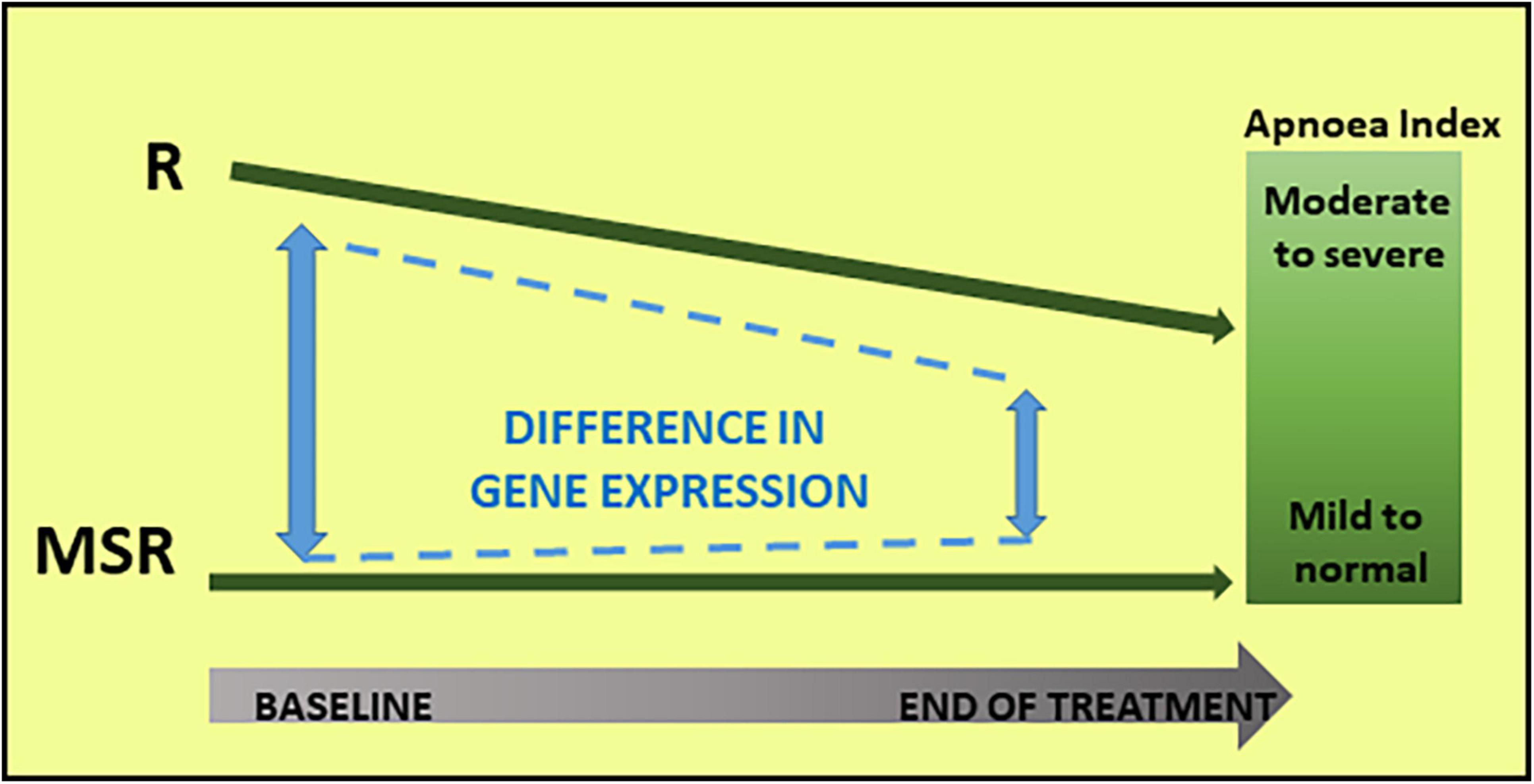
Figure 3. Molecular and phenotypic changes in Responder (R) and Mecasermin Study Reference (MSR) groups throughout the study. Based on their breathing phenotype, the cohort was divided into two groups: Responder and MSR groups. The R group, including participants with moderate to severe breath holding phenotype, responded to mecasermin administration by decreasing their apnoea index. Because of their virtual lack of breath holding phenotype, those in the MSR group experienced minimal changes. In parallel to these changes in breathing phenotype in the R group, there was a decrease in group differences in transcript profiles (i.e., significant at baseline) throughout the study.
Discussion
Molecular Biomarkers and Response to Mecasermin Treatment
The present study aimed at identifying RNA profiles associated with therapeutic responses to mecasermin in children with RTT. For this purpose, we used samples from a mecasermin phase I trial, which explored in a preliminary fashion clinical response to the compound in a study including two periods of drug administration. Of the two positive clinical endpoints, we selected breathing abnormalities because it included an objective measure: plethysmography. We defined as Responder to the drug an individual who had moderate to severe apnoea index at baseline, and significantly improved breath holding (i.e., apnoea index) based on plethysmographic evaluations. We then compared the gene expression profiles of Responders with the rest of the cohort, which we termed MSR group, throughout the trial. We also evaluated changes within each of the two groups. Although not definitive because of the lack of a non-responder group, our analyses showing differences between R and MSR groups in gene expression that included IGF-1- and breathing phenotype-related genes at baseline suggest that RNA profiles may be able to identify individuals with RTT more likely to respond to IGF-1-like compounds.
Comparisons between T0 and T1 (first drug administration), T1–T2 (washout drug-free period), T2–T3 (second drug administration), and T0–T3 (entire trial), allowed examination of baseline gene expression and its changes in response to single and repeated mecasermin exposure. Comparisons at baseline between classic RTT and MRD groups, both included in the original study (Khwaja et al., 2014), revealed similar gene expression profiles. PC profiles placed MRD participants between the R and MSR RTT groups, as expected from patients with some RTT features but no apnoea as reported (Khwaja et al., 2014). Thus, molecular phenotypical profiles before mecasermin administration were in general correspondence with clinical phenotypes. Since the MRD group was not included in the OLE period, we focussed our gene expression analyses on the classic RTT cohort. These analyses demonstrated marked pre-treatment R versus MSR differences that diminished over time. Changes in the MSR group between T0 and T2 indicate that their gene expression profiles were modified by mecasermin treatment. The latter hypothesis is supported by the clinical changes reported by Pini et al. (2014) in a single-case study, where the authors conducted two periods of mecasermin administration (6 and 4 months, respectively) separated by a washout period of 2 years. Both administration cycles led to moderate decreases in impairments (e.g., hand wringing, bruxism, apnoea) and increases in abilities (e.g., reaching, pointing, gesturing). However, improvements were not maintained between treatment cycles and outcome profiles differed between cycles. These results support dynamic and partially compensatory responses after mecasermin administration, which could be reflected in gene expression profiles.
The main differences between R and MSR groups were observed at baseline (T0) while the main changes during the course of the trial occurred between the end of the MAD period and the beginning of the OLE period (T1–T2) and throughout the OLE (T2–T3), predominantly in the MSR group. Genes found to be differentially expressed regulate cell cycle processes and, in particular, immune responses (e.g., TMEM176A, TMEM176B). These were discrete changes of variable direction, suggesting both intrinsic RTT pathogenetic processes as well as the effects of the intervention under study.
Gene Expression Profiles of Responders to Mecasermin in Rett Syndrome
Analyses of baseline (T0) transcript profiles from R and MSR groups revealed a clear separation between groups, as evidenced by a single principal component accounting for almost half of the variance in overall gene expression. The differences represented relatively higher expression in the R group with respect to the MSR group (i.e., 3,693 upregulated, 221 downregulated), a pattern that continued but markedly decreased in magnitude throughout the trial (Figure 3). Relevance of these gene expression patterns is supported by the significant correlation between principal components and apnoea index at baseline. Thus, the profile and dynamics of the differential transcript profiles seems to reflect an IGF-1 signalling “favourable” status at baseline in the R group, which facilitated selective cellular responses to IGF-1 administration exemplified by the decrease in HLA-DRB5 expression. On the other hand, mecasermin administration in the MSR group led to multiple presumably adaptive molecular changes throughout the study. These hypotheses are supported, first, by the unbiased, hypothesis-free analysis of pathways and mechanisms that revealed baseline differences in the expression of genes regulating vascular dynamics (i.e., vasoconstriction, vascular permeability), extracellular matrix, or inflammatory/immune responses. These functions are in line with the main phenotype targetted by mecasermin, namely breathing abnormalities, as well as with peripheral vasomotor disturbances commonly observed in individuals with RTT. The fact that the participant in the MSR group with a milder breathing phenotype and improvement had a gene expression profile similar to the R group, underscores the relationship between transcript patterns and clinical profiles and outcomes. Hypothesis-driven analyses discussed below provided additional support to the notion that the identified changes in gene expression were in response to IGF-1 administration.
Mechanisms Underlying Positive Response to Mecasermin in Rett Syndrome
To get additional insight into the mechanisms underlying positive response to mecasermin, we examined gene expression dynamics throughout the study using hypothesis-driven analyses. We selected pathways and mechanisms that have been implicated in the pathophysiology of RTT, such as MAPK and PI3K signalling (Tropea et al., 2009; Mellios et al., 2014), BDNF (Zhou et al., 2006), metabolic abnormalities in RTT such as mitochondrial dysfunction (Shulyakova et al., 2017; Shovlin and Tropea, 2018), and immunological function (Maezawa and Jin, 2010; Shovlin and Tropea, 2018). We also considered pathways associated to monoamine modulation (Viemari et al., 2005; Toward et al., 2013; Pini et al., 2016a; Vogelgesang et al., 2018) and IGF-1 signalling (Linker et al., 2020) since these are relevant to the breathing phenotype and its treatment with mecasermin. These analyses confirmed the involvement of inflammatory and immune responses, but also revealed differences in monoamine- and metabolism/homeostasis-related genes. Underscoring mecasermin’s mechanism of action, differential gene expression dynamics between the R and MSR groups demonstrated distinct transcript profiles related to IGF-1 signalling. Indeed, the main differences between the R and MSR groups at T0, involved the BDNF cellular pathway as well as previously reported gene expression changes in response to IGF-1 treatment in ASD iPSCs. As in the hypothesis-free analyses, most differences between the R and MSR groups were found at T0. Nonetheless, IGF-1, BDNF, and apnoea-related genes were also differential at later time points suggesting continuous action of IGF-1 on target pathways. This molecular dynamics resembles the effects of mecasermin on brain activity in participants with RTT, as previously reported by us (Keogh et al., 2020). The reason for the MSR group’s greater changes in gene expression, in response to mecasermin, is unknown and deserves further examination since these molecular changes may disclose key events associated with response to IGF-1 and related compounds.
Potential of RNA Profiles as Molecular Signatures of Response to Mecasermin and Related Compounds
This preliminary study supports the potential of gene expression profiles as biomarkers in RTT drug trials. Although our data only revealed gene expression patterns in participants with severe breathing phenotype who also improved after mecasermin administration, and no treatment response comparison group (i.e., severe apnoea without improvement) was available, the nature and evolution of the expression profiles (i.e., correlated with apnoea index at baseline, higher expression of IGF-1 signalling and monoamine modulation genes) suggest that they were treatment related. This and the fact that other informative genes in this study are in line with our current knowledge of RTT pathogenesis (e.g., immune and metabolic mechanisms) underscore the relevance of the findings. Nonetheless, follow up investigations need to address response to treatment more directly. Ideally, studies with larger samples or other IGF-1 related drugs following the course of clinical responses will elucidate whether RNA profiles could become surrogate endpoints, and will provide additional validation of the reported results (i.e., qPCR).
Our findings encourage similar assessments for other drugs under preclinical and clinical investigation in RTT. While other aspects of study design, including dosage, length, and endpoints, continue to be critical for the successful outcome of drug trials in RTT, cohort selection for all candidate treatments for RTT could be improved by molecular profiling. Whether the present data will lead to a re-examination of the therapeutic potential of IGF-1 treatment will depend on follow up supportive studies. Nonetheless, ongoing RTT studies with trofinetide may benefit of the reported data.
Although the present study used an objective measure of clinical outcome, breathing patterns by plethysmography, we acknowledge several limitations. These included small sample size, wide age range, and the limited nature of the molecular investigations. Indeed, proteomics or metabolomics studies could provide additional insights into the molecular mechanisms associated with clinical outcomes. Another limitation is the use of whole blood for RNA analysis, which could be influenced by the individual’s inflammatory/immunological status and its associated variability in cell types. Analyses of RNA expression in different cell types could have been more informative, but they were not feasible in the present study. Nonetheless, we consider the reported data the first step for identifying blood-based biomarkers in drug trials of IGF-1-related compounds in RTT. Future investigations will ideally assess the correlation between blood biomarkers with brain activity and other biomarkers, as well as with a wider range of clinical endpoints. Preclinical studies in animal models will be helpful for studying the correlation between changes in candidate biomarkers and other neurologic parameters that are also measurable in humans, such as motor function and sensory processing (e.g., prepulse inhibition of the startle response) (Kaufmann et al., 2019).
Data Availability Statement
The datasets presented in this study can be found in online repositories. The names of the repository/repositories and accession number(s) can be found below: National Center for Biotechnology Information (NCBI) BioProject database under accession number GSE198856.
Ethics Statement
The studies involving human participants were reviewed and approved by the Boston Children’s Hospital Institutional Review Board (IRB-10-08-0403). Written informed consent to participate in this study was provided by the participants’ legal guardian/next of kin.
Author Contributions
SS analysed the data, prepared the figures and tables, and contributed to the preparation of the manuscript. CD performed some experiments and contributed to the discussion of the data. LS contributed to data collection. SB contributed to data analysis. MSa contributed to data collection, discussion of the results, and preparation of the manuscript. MSu contributed to the discussion of the data and the preparation of the manuscript. WK contributed to the planning of the experiment, collection of the data, and preparation of the manuscript. DT contributed to the planning of the experiment, data analysis, and preparation of the manuscript. All authors contributed to the article and approved the submitted version.
Funding
This work was supported by the International Rett Syndrome Foundation (awards 2907 and 3507) (DT, WK, and MSu), the Boston Children’s Hospital Intellectual and Developmental Disabilities Research Center P30 HD18655/U54 HD090255, Harvard Catalyst–The Harvard Clinical and Translational Science Center (National Institutes of Health Grant 1 UL1 RR 025758-01), and NIH Grant MH085802 (MS). DT was an investigator in the FutureNeuro Research Centre supported by Science Foundation Ireland (SFI) under Grant Number 16/RC/3948 and co-funded under the European Regional Development Fund and by FutureNeuro industry partners and supported by Lejeune Foundation (1935-2020), and Meath Foundation (2019 research grant –coPI).
Conflict of Interest
WK was the Chief Scientific Officer of Anavex Life Sciences.
The remaining authors declare that the research was conducted in the absence of any commercial or financial relationships that could be construed as a potential conflict of interest.
Publisher’s Note
All claims expressed in this article are solely those of the authors and do not necessarily represent those of their affiliated organizations, or those of the publisher, the editors and the reviewers. Any product that may be evaluated in this article, or claim that may be made by its manufacturer, is not guaranteed or endorsed by the publisher.
Acknowledgments
We thank all the families of the patients with Rett Syndrome who took part in the study.
Supplementary Material
The Supplementary Material for this article can be found online at: https://www.frontiersin.org/articles/10.3389/fnins.2022.868008/full#supplementary-material
Footnotes
References
Amir, R. E., Van den Veyver, I. B., Wan, M., Tran, C. Q., Francke, U., and Zoghbi, H. Y. (1999). Rett syndrome is caused by mutations in X-linked MECP2, encoding methyl-CpG-binding protein 2. Nat. Genet. 23, 185–188. doi: 10.1038/13810
Asaka, Y., Jugloff, D. G. M., Zhang, L., Eubanks, J. H., and Fitzsimonds, R. M. (2006). Hippocampal synaptic plasticity is impaired in the Mecp2-null mouse model of Rett syndrome. Neurobiol. Dis. 21, 217–227. doi: 10.1016/j.nbd.2005.07.005
Bach, S., Ryan, N. M., Guasoni, P., Corvin, A. P., El-Nemr, R. A., Khan, D., et al. (2020). Methyl - CpG - binding protein 2 mediates overlapping mechanisms across brain disorders. Sci. Rep. 10:22255. doi: 10.1038/s41598-020-79268-0
Banerjee, A., Rikhye, R. V., Breton-Provencher, V., Tang, X., Li, C., Li, K., et al. (2016). Jointly reduced inhibition and excitation underlies circuit-wide changes in cortical processing in Rett syndrome. Proc. Natl. Acad. Sci. U.S.A. 113, E7287–E7296. doi: 10.1073/pnas.1615330113
Blackman, M. P., Djukic, B., Nelson, S. B., and Turrigiano, G. G. (2012). A Critical and Cell-Autonomous Role for MeCP2 in Synaptic Scaling Up. J. Neurosci. 32, 13529–13536. doi: 10.1523/JNEUROSCI.3077-12.2012
Castro, J., Garcia, R. I., Kwok, S., Banerjee, A., Petravicz, J., Woodson, J., et al. (2014). Functional recovery with recombinant human IGF1 treatment in a mouse model of Rett Syndrome. Proc. Natl. Acad. Sci. U.S.A. 111, 9941–9946. doi: 10.1073/pnas.1311685111
Chen, R. Z., Akbarian, S., Tudor, M., and Jaenisch, R. (2001). Deficiency of methyl-CpG binding protein-2 in CNS neurons results in a Rett-like phenotype in mice. Nat. Genet. 27, 327–331. doi: 10.1038/85906
Chen, Y., Lun, A. T. L., and Smyth, G. K. (2016). From reads to genes to pathways: differential expression analysis of RNA-Seq experiments using Rsubread and the edgeR quasi-likelihood pipeline. F1000Res. 5:1438. doi: 10.12688/f1000research.8987.1
Chen, Y., Mccarthy, D., Robinson, M., and Smyth, G. K. (2017). edgeR: Differential Expression Analysis of Digital Gene Expression Data User’s Guide. Available online at: http://www.bioconductor.org/packages/release/bioc/vignettes/edgeR/inst/doc/edgeRUsersGuide.pdf (accessed December, 2019).
Condamine, T., Le Texier, L., Howie, D., Lavault, A., Hill, M., Halary, F., et al. (2010). Tmem176B and Tmem176A are associated with the immature state of dendritic cells. J. Leukoc. Biol. 88, 507–515. doi: 10.1189/jlb.1109738
Crivellari, I., Pecorelli, A., Cordone, V., Marchi, S., Pinton, P., Hayek, J., et al. (2021). Impaired mitochondrial quality control in Rett Syndrome. Arch. Biochem. Biophys. 700:108790. doi: 10.1016/j.abb.2021.108790
Cuddapah, V. A., Pillai, R. B., Shekar, K. V., Lane, J. B., Kathleen, J., Skinner, S. A., et al. (2014). Methyl-CpG-bnding protein 2 (MECP2) mutation type is associated with disease severity in Rett Syndrome. J. Med. Genet. 51, 152–158. doi: 10.1136/jmedgenet-2013-102113.Methyl-CpG-binding
De Felice, C., Della Ragione, F., Signorini, C., Leoncini, S., Pecorelli, A., Ciccoli, L., et al. (2014). Oxidative brain damage in Mecp2-mutant murine models of Rett syndrome. Neurobiol. Dis. 68, 66–77. doi: 10.1016/j.nbd.2014.04.006
Derr, A., Yang, C., Zilionis, R., Sergushichev, A., Blodgett, D. M., Redick, S., et al. (2016). End Sequence Analysis ToolKit (ESAT) expands the extractable from single cell RNA-seq experiments. Genome Res. 26, 1397–1410. doi: 10.1101/gr.207902.116
Dyer, A. H., Vahdatpour, C., Sanfeliu, A., and Tropea, D. (2016). The role of Insulin-Like Growth Factor 1 (IGF-1) in brain development, maturation and neuroplasticity. Neuroscience 325, 89–99. doi: 10.1016/j.neuroscience.2016.03.056
Fabregat, A., Korninger, F., Viteri, G., Sidiropoulos, K., Marin-Garcia, P., Ping, P., et al. (2018). Reactome graph database: efficient access to complex pathway data. PLoS Comput. Biol. 14:e1005968. doi: 10.1371/journal.pcbi.1005968
Ganz, T. (2003). Defensins: antimicrobial peptides of innate immunity. Nat. Rev. Immunol. 3, 710–720. doi: 10.1038/nri1180
Gazit, N., Vertkin, I., Shapira, I., Helm, M., Slomowitz, E., Sheiba, M., et al. (2016). IGF-1 receptor differentially regulates spontaneous and evoked transmission via mitochondria at hippocampal synapses. Neuron 89, 583–597. doi: 10.1016/j.neuron.2015.12.034
Glaze, D. G., Neul, J. L., Kaufmann, W. E., Berry-kravis, E., Condon, S., Stoms, G., et al. (2019). Double-blind, randomised, placebo-controlled study of trofinetide in pediatric Rett syndrome. Neurology 92, 1912–1925. doi: 10.1212/WNL.0000000000007316
Glaze, D. G., Neul, J. L., Percy, A., Feyma, T., Beisang, A., Yaroshinsky, A., et al. (2017). A double-blind, randomized, placebo-controlled clinical study of trofinetide in the treatment of rett syndrome. Pediatr. Neurol. 76, 37–46. doi: 10.1016/j.pediatrneurol.2017.07.002
Grisaru-Tal, S., Dulberg, S., Beck, L., Zhang, C., Itan, M., Hediyeh-zadeh, S., et al. (2021). Metastasis-entrained eosinophils enhance lymphocyte-mediated antitumor immunity. Cancer Res. 81, 5555–5571. doi: 10.1158/0008-5472.CAN-21-0839
Guan, J., and Gluckman, P. D. (2009). IGF-1 derived small neuropeptides and analogues: a novel strategy for the development of pharmaceuticals for neurological conditions. Br. J. Pharmacol. 157, 881–891. doi: 10.1111/j.1476-5381.2009.00256.x
Guy, J., Hendrich, B., Holmes, M., Martin, J. E., and Bird, A. (2001). A mouse Mecp2-null mutation causes neurological symptoms that mimic Rett syndrome. Nat. Genet. 27, 322–326. doi: 10.1038/85899
Ip, J. P. K., Mellios, N., and Sur, M. (2018). Rett syndrome: insights into genetic, molecular and circuit mechanisms. Nat. Rev. Neurosci. 19, 368–382. doi: 10.1038/s41583-018-0006-3
Katz, D. M., Berger-Sweeney, J. E., Eubanks, J. H., Justice, M. J., Neul, J. L., Pozzo-Miller, L., et al. (2012). Preclinical research in Rett syndrome: setting the foundation for translational success. Dis. Models Mech. 5, 733–745. doi: 10.1242/dmm.011007
Kaufmann, W. E., Johnston, M. V., and Blue, M. E. (2005). MeCP2 expression and function during brain development: implications for Rett syndrome’s pathogenesis and clinical evolution. Brain Dev. 27, 77–87. doi: 10.1016/j.braindev.2004.10.008
Kaufmann, W. E., Sprouse, J., Rebowe, N., Hanania, T., Klamer, D., and Missling, C. U. (2019). ANAVEX§2-73 (blarcamesine), a Sigma-1 receptor agonist, ameliorates neurologic impairments in a mouse model of Rett syndrome. Pharmacol. Biochem. Behav. 187:172796. doi: 10.1016/j.pbb.2019.172796
Kaufmann, W. E., Stallworth, J. L., Everman, D. B., and Skinner, S. A. (2016). Neurobiologically-based treatments in Rett syndrome: opportunities and challenges. Expert Opin. Orphan Drugs 4, 1043–1055. doi: 10.1080/21678707.2016.1229181
Keogh, C., Pini, G., Gemo, I., Kaufmann, W. E., and Tropea, D. (2020). Functional network mapping reveals state-dependent response to IGF1 treatment in rett syndrome. Brain Sci. 10:515. doi: 10.3390/brainsci10080515
Khwaja, O. S., Ho, E., Barnes, K. V., O’Leary, H. M., Pereira, L. M., Finkelstein, Y., et al. (2014). Safety, pharmacokinetics, and preliminary assessment of efficacy of mecasermin (recombinant human IGF-1) for the treatment of Rett syndrome. Proc. Natl. Acad. Sci. U.S.A. 111, 4596–4601. doi: 10.1073/pnas.1311141111
Leonard, H., Cobb, S., and Downs, J. (2017). Clinical and biological progress over 50 years in Rett syndrome. Nat. Rev. Neurol. 13, 37–51. doi: 10.1038/nrneurol.2016.186
Lin, P., Nicholls, L., Assareh, H., Fang, Z., Amos, T. G., Edwards, R. J., et al. (2016). Transcriptome analysis of human brain tissue identifies reduced expression of complement complex C1Q Genes in Rett syndrome. BMC Genomics 17:427. doi: 10.1186/s12864-016-2746-7
Linker, S. B., Mendes, A. P. D., and Marchetto, M. C. (2020). IGF-1 treatment causes unique transcriptional response in neurons from individuals with idiopathic autism. Mol. Autism 11:55. doi: 10.1186/s13229-020-00359-w
Maezawa, I., and Jin, L. W. (2010). Rett syndrome microglia damage dendrites and synapses by the elevated release of glutamate. J. Neurosci. 30, 5346–5356. doi: 10.1523/JNEUROSCI.5966-09.2010
Mellios, N., Woodson, J., Garcia, R. I., Crawford, B., Sharma, J., Sheridan, S. D., et al. (2014). β2-Adrenergic receptor agonist ameliorates phenotypes and corrects microRNA-mediated IGF1 deficits in a mouse model of Rett syndrome. Proc. Natl. Acad. Sci. U.S.A. 111, 9947–9952. doi: 10.1073/pnas.1309426111
Muley, V. Y., López-Victorio, C. J., Ayala-Sumuano, J. T., González-Gallardo, A., González-Santos, L., Lozano-Flores, C., et al. (2020). Conserved and divergent expression dynamics during early patterning of the telencephalon in mouse and chick embryos. Prog. Neurobiol. 186:101735. doi: 10.1016/j.pneurobio.2019.101735
Neul, J. L., Kaufmann, W. E., Glaze, D. G., Clarke, A. J., Leonard, H., Bailey, M. E. S., et al. (2010). Rett syndrome: revised diagnostic criteria and nomenlcature. Annu. Neurol. 68, 944–950. doi: 10.1002/ana.22124.Rett
Neul, J. L., Skinner, S. A., Annese, F., Lane, J., Heydemann, P., Jones, M., et al. (2020). Metabolic signatures differentiate rett syndrome from unaffected siblings. Front. Integr. Neurosci. 14:7. doi: 10.3389/fnint.2020.00007
Nguyen, M. V. C., Du, F., Felice, C. A., Shan, X., Nigam, A., Mandel, G., et al. (2013). MeCP2 is critical for maintainng mature neuronal networks and global brain anatomy during late stages of postnatal brain development and in the mature adult brain. J. Neurosci. 32, 10021–10034. doi: 10.1523/JNEUROSCI.1316-12.2012.MeCP2
O’Driscoll, C. M., Kaufmann, W. E., and Bressler, J. P. (2013). MeCP2 deficiency enhances glutamate release through NF-κB signaling in myeloid derived cells. J. Neuroimmunol. 265, 61–67. doi: 10.1016/j.jneuroim.2013.09.002
O’Leary, H. M., Kaufmann, W. E., Barnes, K. V., Rakesh, K., Kapur, K., Tarquinio, D. C., et al. (2018). Placebo-controlled crossover assessment of mecasermin for the treatment of Rett syndrome. Ann. Clin. Transl. Neurol. 5, 323–332. doi: 10.1002/acn3.533
Park, M. J., Aja, S., Li, Q., Degano, A. L., Penati, J., Zhuo, J., et al. (2014). Anaplerotic triheptanoin diet enhances mitochondrial substrate use to remodel the metabolome and improve lifespan, motor function, and sociability in MeCP2-null mice. PLoS One 9:e109527. doi: 10.1371/journal.pone.0109527
Pecorelli, A., Leoni, G., Cervellati, F., Canali, R., Signorini, C., Leoncini, S., et al. (2013). Genes related to mitochondrial functions, protein degradation, and chromatin folding are differentially expressed in lymphomonocytes of rett syndrome patients. Mediators Inflamm. 2013:137629. doi: 10.1155/2013/137629
Percy, A. K., Neul, J. L., Glaze, D. G., Motil, K. J., Skinner, S. A., Khwaja, O., et al. (2010). Brief communications: Rett syndrome diagnostic criteria: lessons from the natural history study. Ann. Neurol. 68, 951–955. doi: 10.1002/ana.22154
Pini, G., Bigoni, S., Congiu, L., Romanelli, A. M., Scusa, M. F., Di Marco, P., et al. (2016a). Rett syndrome: a wide clinical and autonomic picture. Orphanet J. Rare Dis. 11:132. doi: 10.1186/s13023-016-0499-7
Pini, G., Congiu, L., Benincasa, A., DiMarco, P., Bigoni, S., Dyer, A. H., et al. (2016b). Illness Severity, Social and Cognitive Ability, and EEG Analysis of Ten Patients with Rett Syndrome Treated with Mecasermin (Recombinant Human IGF-1). Autism Res. Treat. 2016:5073078. doi: 10.1155/2016/5073078
Pini, G., Scusa, M. F., Benincasa, A., Bottiglioni, I., Congiu, L., Vadhatpour, C., et al. (2014). Repeated insulin-like growth factor 1 treatment in a patient with rett syndrome: a single case study. Front. Pediatr. 2:52. doi: 10.3389/fped.2014.00052
Pini, G., Scusa, M. F., Congiu, L., Benincasa, A., Morescalchi, P., Bottiglioni, I., et al. (2012). IGF1 as a potential treatment for Rett syndrome: safety assessment in six Rett patients. Autism Res. Treat. 2012:679801. doi: 10.1155/2012/679801
Robinson, M. D., McCarthy, D. J., and Smyth, G. K. (2009). edgeR: a Bioconductor package for differential expression analysis of digital gene expression data. Bioinformatics 26, 139–140. doi: 10.1093/bioinformatics/btp616
Ross, P. D., Guy, J., Selfridge, J., Kamal, B., Bahey, N., Tanner, E., et al. (2016). Exclusive expression of MeCP2 in the nervous system distinguishes between brain and peripheral Rett syndrome-like phenotypes. Hum. Mol. Genet. 25, 4389–4404. doi: 10.1093/hmg/ddw269
Sanfeliu, A., Hokamp, K., Gill, M., and Tropea, D. (2019). Transcriptomic Analysis of Mecp2 mutant mice reveals differentially expressed genes and altered mechanisms in both blood and brain. Front. Psychiatry 10:278. doi: 10.3389/fpsyt.2019.00278
Shovlin, S., and Tropea, D. (2018). Transcriptome level analysis in Rett syndrome using human samples from different tissues. Orphanet J. Rare Dis. 13:113. doi: 10.1186/s13023-018-0857-8
Shulyakova, N., Andreazza, A. C., Mills, L. R., and Eubanks, J. H. (2017). Mitochondrial dysfunction in the pathogenesis of rett syndrome: implications for mitochondria-targeted therapies. Front. Cell. Neurosci. 11:58. doi: 10.3389/fncel.2017.00058
Song, C., Feodorova, Y., Guy, J., Peichl, L., Jost, K. L., Kimura, H., et al. (2014). DNA methylation reader MECP2: cell type- and differentiation stage-specific protein distribution. Epigenetics Chromatin 7:17. doi: 10.1186/1756-8935-7-17
Soumillon, M., Cacchiarelli, D., Semrau, S., van Oudenaarden, A., and Mikkelsen, T. S. (2014). Characterization of directed differentiation by high-throughput single-cell RNA-Seq. bioRxiv [Preprint]. doi: 10.1101/003236
The Gene Ontology Consortium (2000). Gene Ontology: tool for the unification of biology. Nat. Genet. 25, 25–29. doi: 10.1038/75556
The Gene Ontology Consortium (2021). The Gene Ontology resource: enriching a GOld mine. Nucleic Acids Res. 49, D325–D334. doi: 10.1093/nar/gkaa1113
Toward, M. A., Abdala, A. P., Knopp, S. J., Paton, J. F. R., and Bissonnette, J. M. (2013). Increasing brain serotonin corrects CO2 chemosensitivity in methyl-CpG-binding protein 2 (Mecp2)-deficient mice. Exp. Physiol. 98, 842–849. doi: 10.1113/expphysiol.2012.069872
Tropea, D., Giacometti, E., Wilson, N. R., Beard, C., McCurry, C., Fu, D. D., et al. (2009). Partial reversal of Rett Syndrome-like symptoms in MeCP2 mutant mice. Proc. Natl. Acad. Sci. U.S.A. 106, 2029–2034. doi: 10.1073/pnas.0812394106
Venables, P. J. W., Brookes, S. M., Griffith, D., Weiss, R., and Boyd, M. T. (1995). Abudnance of an endogenous retroviral envelope protein trophoblasts suggests a biological function. Virology 211, 589–592. doi: 10.1006/viro.1995.1442
Viemari, J. C., Roux, J. C., Tryba, A. K., Saywell, V., Burnet, H., Peña, F., et al. (2005). Mecp2 deficiency disrupts norepinephrine and respiratory systems in mice. J. Neurosci. 25, 11521–11530. doi: 10.1523/JNEUROSCI.4373-05.2005
Vogelgesang, S., Niebert, M., Bischoff, A. M., Hülsmann, S., and Manzke, T. (2018). Persistent expression of serotonin receptor 5b alters breathing behavior in male MeCP2 knockout mice. Front. Mol. Neurosci. 11:28. doi: 10.3389/fnmol.2018.00028
Wu, D., Lim, E., Vaillant, F., Asselin-Labat, M. L., Visvader, J. E., and Smyth, G. K. (2010). ROAST: rotation gene set tests for complex microarray experiments. Bioinformatics 26, 2176–2182. doi: 10.1093/bioinformatics/btq401
Zhao, D., Mokhtari, R., Pedrosa, E., Birnbaum, R., Zheng, D., and Lachman, H. M. (2017). Transcriptome analysis of microglia in a mouse model of Rett syndrome: differential expression of genes associated with microglia/macrophage activation and cellular stress. Mol. Autism 8:17. doi: 10.1186/s13229-017-0134-z
Keywords: Rett syndrome, mecasermin, insulin-like growth factor 1 (IGF1), methyl-CpG binding protein 2 (MECP2), biomarker
Citation: Shovlin S, Delepine C, Swanson L, Bach S, Sahin M, Sur M, Kaufmann WE and Tropea D (2022) Molecular Signatures of Response to Mecasermin in Children With Rett Syndrome. Front. Neurosci. 16:868008. doi: 10.3389/fnins.2022.868008
Received: 01 February 2022; Accepted: 26 April 2022;
Published: 31 May 2022.
Edited by:
Maria Vincenza Catania, Institute of Neurological Sciences (CNR), ItalyReviewed by:
Jessica L. MacDonald, Syracuse University, United StatesFerdinando Di Cunto, University of Turin, Italy
Copyright © 2022 Shovlin, Delepine, Swanson, Bach, Sahin, Sur, Kaufmann and Tropea. This is an open-access article distributed under the terms of the Creative Commons Attribution License (CC BY). The use, distribution or reproduction in other forums is permitted, provided the original author(s) and the copyright owner(s) are credited and that the original publication in this journal is cited, in accordance with accepted academic practice. No use, distribution or reproduction is permitted which does not comply with these terms.
*Correspondence: Daniela Tropea, RGFuaWVsYS5Ucm9wZWFAdGNkLmll; Walter E. Kaufmann, d2FsdGVyLmUua2F1Zm1hbm5AZW1vcnkuZWR1