- 1Department of Magnetic Resonance Imaging (MRI), Shaanxi Provincial People's Hospital, Xi'an, China
- 2Department of Graduate, Xi'an Medical University, Xi'an, China
- 3Department of Neurology, Shaanxi Provincial People's Hospital, Xi'an, China
- 4Department of Radiology, Beijing Tiantan Hospital, Capital Medical University, Beijing, China
Objective: To develop and validate a radiomics nomogram for predicting stroke recurrence in symptomatic intracranial atherosclerotic stenosis (SICAS).
Methods: The data of 156 patients with SICAS were obtained from the hospital database. Those with and without stroke recurrence were identified. The 156 patients were separated into a training cohort (n = 110) and a validation cohort (n = 46). Baseline clinical data were collected from our medical records, and plaque radiological features were extracted from vascular wall high-resolution imaging (VW-HRMRI). The imaging sequences included 3D-T1WI-VISTA, T2WI, and 3D-T1WI-VISTA-enhanced imaging. Least absolute shrinkage and selection operator (LASSO) analysis were used to select the radiomics features associated with stroke recurrence. Then, multiple logistic regression analysis of clinical risk factors, radiological features, and radiomics signatures were performed, and a predictive nomogram was constructed to predict the probability of stroke recurrence in SICAS. The performance of the nomogram was evaluated.
Results: Diabetes mellitus, plaque burden, and enhancement ratio were independent risk factors for stroke recurrence [odds ratio (OR) = 1.24, 95% confidence interval (CI): 1.04–3.79, p = 0.018; OR = 1.76, per 10% increase, 95% CI, 1.28–2.41, p < 0.001; and OR = 1.94, 95% CI: 1.27–3.09, p < 0.001]. Five features of 3D-T1WI-VISTA, six features of T2WI, and nine features of 3D-T1WI-VISTA-enhanced images were associated with stroke recurrence. The radiomics signature in 3D-T1WI-VISTA-enhanced images was superior to the radiomics signature of the other two sequences for predicting stroke recurrence in both the training cohort [area under the curve (AUC), 0.790, 95% CI: 0.669–0.894] and the validation cohort (AUC, 0.779, 95% CI: 0.620–0.853). The combination of clinical risk factors, radiological features, and radiomics signature had the best predictive value (AUC, 0.899, 95% CI: 0.844–0.936 in the training cohort; AUC, 0.803, 95% CI: 0.761–0.897 in the validation cohort). The C-index of the nomogram was 0.880 (95% CI: 0.805–0.934) and 0.817 (95% CI: 0.795–0.948), respectively, in the training and validation cohorts. The decision curve analysis further confirmed that the radiomics nomogram had good clinical applicability with a net benefit of 0.458.
Conclusion: The radiomics features were helpful to predict stroke recurrence in patients with SICAS. The nomogram constructed by combining clinical high-risk factors, plaque radiological features, and radiomics features is a reliable tool for the individualized risk assessment of predicting the recurrence of SICAS stroke.
Introduction
Intracranial atherosclerotic stenosis (ICAS) is a common cause of ischemic stroke, especially in Asian populations (Chimowitz et al., 2005; Wang et al., 2014). Stroke caused by ICAS is characterized by relatively severe symptoms, longer hospital stay, and a high risk of stroke recurrence (Wang et al., 2014). Even with active treatment, 12–38.2% of patients with ICAS will have a recurrent stroke within a year (Mazighi et al., 2006; Derdeyn et al., 2014; Prabhakaran et al., 2021). Effective secondary prevention can be facilitated by identification markers associated with recurrent stroke.
Stroke recurrence in ICAS is related to various factors, such as plaque burden, plaque inflammation, infarction pattern, collateral status, etc. (Kim et al., 2016; Wabnitz et al., 2019; Song et al., 2020). Plaque burden and plaque enhancement are independent risk factors for stroke recurrence, and a greater degree of stenosis is associated with a higher risk of recurrence (Ran et al., 2020; Shi et al., 2021). Vessel wall high-resolution magnetic resonance imaging (VW-HRMRI) can accurately detect plaque burden and also provide components, inflammation, and angiogenesis information (Saam et al., 2007), which are features that help the clinician assess plaque vulnerability and predict future cerebrovascular events (Underhill et al., 2010; Hosseini et al., 2013). Despite the great progress in high-resolution magnetic resonance imaging (HRMRI) evaluation of intracranial atherosclerotic plaques, limitations of imaging resolution and technical methods, complexity of intracranial arterial plaques, and subjectivity in the interpretation of plaque components and morphology make the accurate evaluation of intracranial atherosclerotic plaques challenging. There is an urgent need for automatic and reproducible quantitative methods to evaluate plaque characteristics predictive of recurrent stroke in symptomatic ICAS (SICAS).
Radiomics, which analyzes the high-throughput quantitative parameters in radiographic images, can obtain histological and biological information of whole lesions, has been shown to be useful for diagnosis and classification, and aids in the evaluation of treatment response of various tumors (Aerts et al., 2014; Yamamoto et al., 2014; Gillies et al., 2016; Kickingereder et al., 2016; Braman et al., 2017). Radiomics methods are gradually being applied for the diagnosis of intracranial atherosclerotic diseases and has been shown to be capable of distinguishing symptomatic from asymptomatic basilar atherosclerosis with good accuracy (Shi et al., 2018). In addition, the histogram features were also effective predictive parameters in the differences between culprit and non-culprit lesions (Shi et al., 2020). Radiomics methods show great potential in the evaluation of atherosclerotic diseases. However, to the best of our knowledge, there are no studies on the radiomics signature of plaques on VW-HRMRI in patients with SICAS.
The purpose of this study was to develop and validate a nomogram constructed by combined clinical high-risk factors, plaque radiological features, and radiomics features that can be used to predict stroke recurrence in SICAS. This nomogram could be a convenient and useful clinical tool for determining treatment strategies in patients with SICAS.
Materials and Methods
Patients
The data of 468 patients with SICAS who received intracranial VW-HRMRI in our hospital between September 2017 and March 2021 were extracted from the hospital database and retrospectively analyzed. Patients were eligible for inclusion (Supplementary Figure 1) if (1) they had intracranial atherosclerotic stenosis of 50–99%; (2) they had presented with ischemic stroke or symptoms of transient ischemic attack (TIA); and (3) diffusion-weighted imaging (DWI) had showed acute infarction located in the ipsilateral intracranial atherosclerotic stenosis area. The exclusion criteria were (1) ipsilateral internal carotid artery stenosis >50% on ultrasound or magnetic resonance angiography (MRA) or computed tomography angiography (CTA); (2) stroke due to non-atherosclerotic vascular disease such as dissection, vasculitis, or moyamoya disease; (3) evidence of cardiogenic embolism; or (4) poor imaging quality. All patients underwent DWI, time-of-flight (TOF)-MRA, and VW-HRMRI within 7 days of onset of symptoms, and all received standard antithrombotic therapy and statins and secondary preventive measures (control of high blood pressure and diabetes mellitus, and advice regarding smoking cessation, exercise, and weight loss).
The 156 eligible patients were divided (in a ratio of 7:3) into a training cohort (n = 110; 67 females, 43 males; mean age, 54 years) and a validation cohort (n = 46; 13 females, 33 males; mean age, 56 years).
Baseline demographic and clinical information (sex, age, hypertension, diabetes, hyperlipidemia, smoking status, stroke history, etc.) were recorded. Stroke recurrence was confirmed by a neurologist with more than 5 years of experience; for the diagnosis of recurrence, the following conditions had to be met (Coull and Rothwell, 2004): (1) sudden onset of new focal neurological defects in the previously affected region, with symptoms lasting more than 24 h and a definite imaging evidence or (2) more than two symptoms of TIA with definite distribution in the region of the intracranial atherosclerotic stenosis, even in the absence of imaging evidence of stroke, and (3) cerebral hemorrhage, tumor, and other causes ruled out.
This retrospective study was approved by the ethics committee of Shaanxi provincial people's hospital, and all patients signed the informed consent form.
MRI Protocol
Magnetic resonance imaging was performed with a 32-channel head coil Philips 3.0T magnetic resonance scanner. The MRI sequences included T1-weighted imaging (T1WI), T2-weighted imaging (T2WI), fluid-attenuation inversion-recovery (FLAIR), and DWI. HRMRI was performed within 1 week after the onset of symptoms and included three-dimensional (3D)-TOF MRA, 3D-T1WI-volumetric isotropic TSE acquisition (VISTA), T2WI-turbo spin-echo (TSE), and 3D-T1-VISTA-enhanced imaging. The imaging parameters for each of these sequences were as follows: (1) TOF-MRA: repetition time/echo time (TR/TE) 20/3.6 ms, flip angle 18°, field of view (FOV) 180 × 180 mm2, matrix 256 × 256, layer thickness 0.5 mm; (2) 3D-T1WI-VISTA and 3D-T1WI-VISTA-enhanced imaging: TR/TE 700/16 ms, flip angle 90°, FOV 180 × 180 mm2, spatial resolution 0.5 × 0.5 × 0.5 mm3; and (3) T2WI-TSE: TR/TE 2,500/67 ms, FOV 80 × 80 mm2, matrix 256 × 256, layer thickness 2 mm. For enhanced 3D-T1WI-VISTA, gadobutrol (7.5 mL, 0.1 mmol/kg body weight) was injected intravenously and scanning was performed after 5 min; the scanning time of the whole sequence was about 30 min.
Image Analysis
The raw VW-HRMRI data were imported into MRI-PlaqueView post-processing software (VP Diagnostics, Seattle, WA, USA), and axial, coronal, and sagittal reconstructions were generated. Two senior neuroradiologists (JG and NM, each with 5 years experience in interpreting VW-HRMRI), blinded to the clinical information, independently analyzed the images for lumen size and wall thickness. The inner lumen and outer wall were manually outlined at the maximum intraluminal narrowing (MLN) site on reconstructed post-contrast T1WI images of each patient. The reference site was the nearest plaque-free segments proximal or distal to the MLN site, and the stenosis rate, enhancement ratio, plaque burden, and remodeling index (RI) were calculated using the following formulas:
(1) The stenosis rate =1 – (Ds/Dn), where Ds was the diameter of the artery where the stenosis was most obvious and Dn was the diameter of the proximal normal artery (Samuels et al., 2000).
(2) Plaque burden = (total wall area – lumen area)/total wall area (Tian et al., 2021).
(3) The enhancement ratio was calculated at the site of maximum plaque enhancement, and the signal intensity (SI) was standardized by adjacent gray matter. The enhancement ratio of plaque was calculated using the formula: (post-contrast SI of plaque/post-contrast SI of gray matter)/(pre-contrast SI of plaque/pre-contrast SI of gray matter) (Shi et al., 2018).
(4) RI = vascular areaMLN/vascular areareference. RI ≥ 1.05 was considered as positive remodeling, RI ≤ 1.05 as negative remodeling, and 0.95 < RI <1.05 as no reconstruction (Xu et al., 2010).
Radiomics Analysis
Radiomics extracts high-throughput quantitative image features. The work flow includes plaques segmentation, feature extraction and screening, and model building and evaluation.
Plaque Segmentation
Three-dimensional manual segmentation was performed by a radiologist with 5 years of experience in VW-HRMRI using ITK-SNAP software (http://www.itksnap.org). The original image was magnified by four times and the regions of interest (ROIs) were drawn layer by layer on the 3D-T1WI-VISTA, T2WI-TSE, and 3D-T1WI-VISTA-enhanced images of each patient (Supplementary Figure 2). Then, the volume of the lesion was sketched along the boundary of the plaque to generate the 3D volume of interest (VOI).
Feature Extraction
Wavelet transform was used to filter the original data and extract high-dimensional features from different frequency scales. A total of 402 imaging features reflecting plaque heterogeneity were extracted from 3D-T1WI-VISTA, T2WI-TSE, and 3D-T1WI-VISTA-enhanced images of each patient. These features included non-texture features (such as shape, size, and intensity) and texture features [gray co-occurrence matrix (GLCM), gray-level run-length matrix (GLRLM), gray-level size zone matrix (GLSZM), and neighborhood gray-tone difference matrix (NGTDM)]. All features were extracted by Matlab 2013b (The MathWorks, Natick, MA, USA). To verify the repeatability of the extraction, 20 patients in the training group were randomly selected and re-segmented by a radiologist with 10 years of experience in the interpretation of vessel wall features in MRI, and the radiomics data were measured. Intraclass correlation coefficient (ICC) was calculated to determine the repeatability.
Clinical Features and Radiological Features
Normally distributed continuous variables were expressed as means ± standard deviation and non-normally distributed continuous variables as medians (25th−75th percentiles); comparison between the groups was performed using the independent sample t-test or the Mann–Whitney U-test, respectively. Categorical variables were summarized as counts and percentages and compared using the chi-square or Fisher exact test. Variables (clinical factors and radiological features) significantly associated (P < 0.1) with recurrent stroke in univariable analysis were included in multivariable analysis to identify the independent predictors of recurrent stroke.
Identification of Radiomics Features Related to Stroke Recurrence
Least absolute shrinkage and selection operator (LASSO) were used for eigenvalue dimension reduction to select the plaque features most strongly associated with stroke recurrence in SICAS, parameter tuning conducted by 5-fold cross-validation, based on the radiomics score (Rad score) calculated for each patient with the selected feature. The Mann–Whitney U-test was used to analyze the relationship between radiomics features and stroke recurrence in the training and validation cohorts.
Construction and Validation of the Nomogram
Plaque radiomics features, radiological features, and clinical risk factors were entered into a multivariable logistic regression model to identify the independent predictors of stroke recurrence in the training cohort. The identified predictors were then used to construct the radiomics nomogram for quantifying the risk of stroke recurrence in the training cohort. The efficacy of the nomogram was tested on the internal validation cohort; the total score of each patient was calculated and the association with stroke recurrence was examined. Finally, the C-index and calibration curve were generated from the regression model. To evaluate the clinical effectiveness of the nomogram, decision curve analysis (DCA) was used to quantify the net benefit probability under different thresholds in the training and verification cohorts.
Evaluation of the Predictive Nomogram
Receiver operating characteristic (ROC) analysis was used to assess the usefulness of the nomogram. The Delong test and Bonferroni correction were used to correct p-values for multiple comparisons. The AUC, with 95% confidence intervals (CI), sensitivity, specificity, and accuracy were calculated.
Statistical Analysis
Data analysis was done with SPSS 22 (IBM Corp., Armonk, NY, USA) and R 3.6.3 (https://cran.r-project.org/bin/windows/base/old/3.6.3/). P ≤ 0.05 indicated statistically significant difference.
Results
Comparison of Clinical and Radiological Features
Among the 156 patients in this study, the middle cerebral artery was involved in 97 patients and the basilar artery in 59 patients. Recurrence occurred in 32/110 (29.1%) patients in the training cohort and 13/46 (28.3%) in the validation cohort. Table 1 presents a comparison of clinical factors and radiological features between the training cohort and the validation cohort. Both continuous (plaque burden, plaque enhancement ratio, plaque thickness, and remodeling index) and categorical (diabetes mellitus) variables were associated with stroke recurrence in univariable analysis. In multivariable logistic regression analysis, the independent predictors of stroke recurrence were diabetes mellitus [odds ratio (OR) = 1.24, 95% CI: 1.04–3.79, p = 0.018], plaque burden (OR = 1.76, per 10% increase, 95% CI: 1.28–2.41, p < 0.001), and plaque enhancement ratio (OR = 1.94, 95% CI: 1.27–3.09, P < 0.001).
Radiomics Signature of Plaque for Predicting Stroke Recurrence
The LASSO regression model was used to extract the MRI features with the most predictive value for stroke recurrence in the training cohort; they included six features in 3D-T1WI-VISTA, nine features in T2WI, and seven features in 3D-T1WI-VISTA-enhanced imaging (Table 2). There were significant differences in rad scores for different sequences between patients with and without recurrent stroke in the training cohort: 3D-T1WI-VISTA: −0.43 ± 0.76 vs. 0.14 ± 0.71 (p < 0.001); T2WI: −0.46 ± 0.41 vs. −0.23 ± 0.37 (p < 0.002); 3D-T1WI-VISTA-enhanced imaging: −0.71 ± 0.73 vs. −0.24 ± 0.63 (p < 0.001). Among the individual sequences, the radiomics signature on 3D-T1WI-VISTA-enhanced imaging was superior to the others for predicting stroke recurrence in both the training cohort (AUC, 0.790, 95% CI: 0.669–0.894) and the validation cohort (AUC, 0.779, 95% CI: 0.620–0.853). The combination of all three sequences (3D-T1WI-VISTA, T2WI, and 3D-T1WI-VISTA-enhanced) had the best predictive value in both the training cohort (AUC, 0.813, 95% CI: 0.741–0.901) and the validation cohort (AUC, 0.778, 95% CI: 0.690–0.878) (Table 3 and Figure 1).
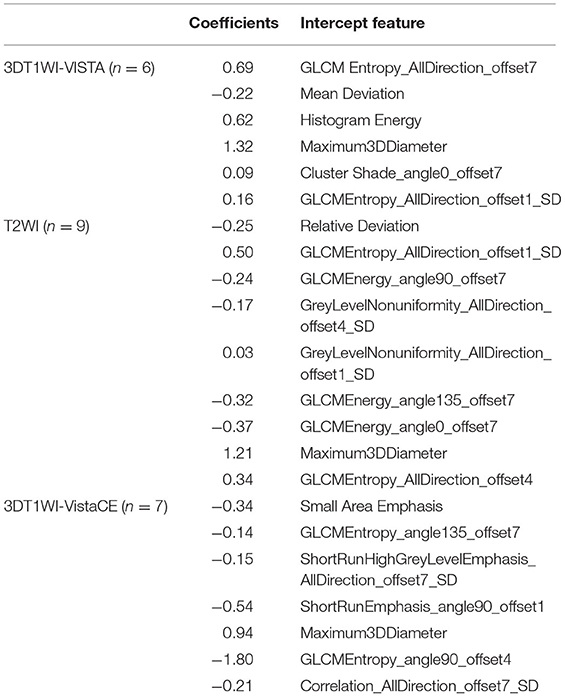
Table 2. Effective intercept features for the 3DT1WI-VISTA, T2WI, and 3DT1WI-VISTA-enhanced images in SICAS.
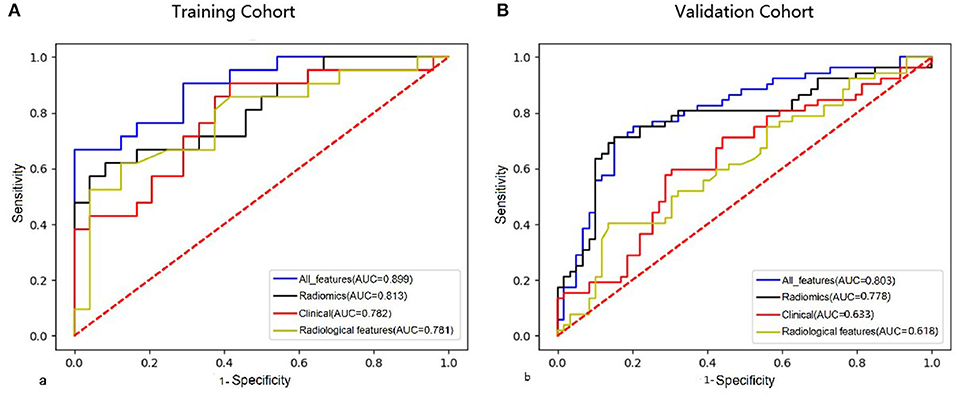
Figure 1. Comparison of ROC curves of clinical features, radiological features, combined radiomics features, and all features in the training cohort (A) and validation cohort (B).
Predictive Model for Stroke Recurrence
The nomogram for prediction of recurrent stroke included clinical risk factors, radiological features, and radiomics signature (Figure 1 and Supplementary Figure 3). In the training cohort, the AUC of the nomogram was 0.899 (95% CI: 0.844–0.936), the sensitivity was 0.844, the specificity was 0.917, and the accuracy was 0.822 (Table 3). In the validation cohort, the AUC was 0.803 (95% CI: 0.761–0.897), the sensitivity was 0.814, the specificity was 0.847, and the accuracy was 0.757 (Table 3). Moreover, the AUC of the nomogram with the training cohort and the validation cohort were better than clinical features (0.899 vs. 0.782, p = 0.003; 0.803 vs. 0.633; p = 0.002) and radiological features (0.899 vs. 0.781; p = 0.003; 0.803 vs. 0.618; p =0.001). However, the AUC of the nomogram model was superior to radiomics features in the training cohort (0.899 vs. 0.813; p = 0.021) and did not differ from the validation cohort (0.803 vs. 0.778; p = 0.626).
Development and Validation of the Nomogram
The C-index of the nomogram was 0.880 (95% CI: 0.805–0.934) and 0.817 (95% CI: 0.795–0.948) in the training cohort and validation cohort, respectively, indicating good accuracy in predicting stroke recurrence in SICAS (Figure 2). The calibration curve showed that the nomogram-predicted probability was close to the actual probability of stroke in the training cohort and validation cohort (p = 0.901 and p = 0.548, respectively; Figure 3). The threshold probability of the decision curve was 2% and the net profit rate was 0.458. When the threshold probability of decision curve was >22%, the nomogram-predicted probability of stroke recurrence was higher than the probability predicted by clinical risk factors or radiological features alone (Figure 4).
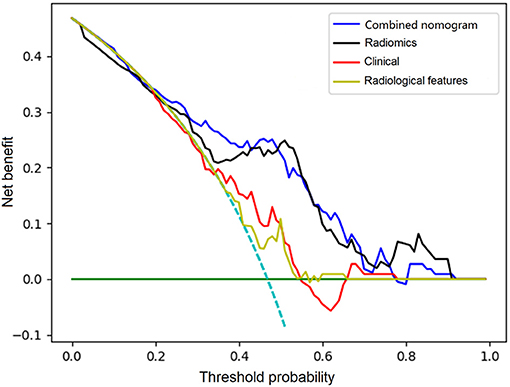
Figure 4. Comparison of decision curves analysis for clinical features, radiological features, combined radiomics features, and the constructed nomogram for prediction of stroke recurrence in SICAS.
Interobserver Consistency
There was good interobserver agreement in radiomics signature extraction and quantitative feature of plaque image (ICC, 0.761–0.898).
Discussion
This study showed that diabetes mellitus, plaque burden, and plaque enhancement ratio are independent predictors of stroke recurrence in SICAS; moreover, radiomics features of plaque on HRMRI had a high sensitivity for identifying the risk of recurrent stroke. The predictive nomogram constructed using high-risk clinical factors, radiological features, and radiomics features of plaques showed a high accuracy for the personalized prediction of stroke recurrence. These findings were helpful for early clinical intervention in people with a high risk of stroke recurrence.
Plaque enhancement ratio and plaque burden have been previously shown to be significantly associated with stroke recurrence in SICAS (Ran et al., 2020; Shi et al., 2020; Xiao et al., 2022). Plaque enhancement, a characteristic of vulnerable plaque, is related to plaque inflammation, neovascularization, and vascular endothelial permeability (Moulton et al., 2003; Marnane et al., 2012; Millon et al., 2012; Qiao et al., 2014). Our study confirmed that both plaque enhancement ratio and plaque burden, rather than degree of stenosis, are major predictors of stroke recurrence in SICAS. Several studies from coronary/intracranial artery disease have suggested the limitation of intraluminal imaging in predicting future vascular events, and the presence of stenosis may not significantly increase the risk of future vascular events (Kim et al., 2016; Mortensen et al., 2020; Ran et al., 2020). In a word, it is not enough to use the degree of stenosis to identify real high-risk patients with stroke recurrence in SICAS who may benefit from preventive treatment. The radiomics features of plaque were better than the conventional HRMRI plaque image characteristics for predicting recurrent stroke in SICAS. Among all HRMRI sequences, 3D-T1WI-VISTA-enhanced images had the highest predictive value. The combination of 3D-T1WI-VISTA, T2WI, and 3D-T1WI-VISTA-enhanced images had an excellent value for predicting stroke recurrence in the training and validation cohorts. Thus, the present study showed that radiomics can excavate quantitative texture and non-texture features that cannot be distinguished with the naked eye.
In our study, 11 radiomics signatures were associated with recurrent stroke in SICAS when combining constructing radiomics signatures. Stroke recurrence in atherosclerotic stenosis has been shown to be closely related to the morphological characteristics of plaque (Roquer et al., 2011; Altaf et al., 2014; Deng et al., 2020). This is consistent with our study, which found plaque thickness (in the validation cohort) and positive plaque remodeling (in the training cohort) to be more in the recurrence group than in the non-recurrence group. The finding that Maximum_3D_Diameter is based on morphology radiomics feature, which is related to SICAS recurrence, supports this deduction. Combined with the radiomics model, Histogram Energy represents the degree of gray-scale dispersion, which is related to stroke recurrence in SICAS; it may indicate that the heterogeneity of plaques (lipid-rich necrotic core, inflammation, and hemorrhage) in the SICAS recurrence group is more significant than the non-recurrence group (Salem et al., 2012). A recent study has also demonstrated that the coefficient of variation of histogram analysis was capable of differentiating culprit and non-culprit lesions for ICAS (Shi et al., 2021). Compared with non-culprit lesions, culprit lesions had a larger coefficient of variation as a consequence of a larger difference between the minimum and maximum intensity value and/or smaller mean intensity value on T1WI. The advantage of the radiomics method is that it can provide more information than conventional imaging (Gillies et al., 2016). In the present study, stroke recurrence in SICAS was associated with nine other texture features; these radiomics signatures cannot be easily identified and understood by an observer, and it is still a big challenge to explain the relationship between these radiomics signatures and pathology (Tran et al., 2012), which raises the researchers' attention to the radiomics method in plaques.
In the prediction model of stroke recurrence, we included clinical risk factors, radiological features, and radiomics signature. The radiomics predictive model is better than clinical and radiological features in the prediction of stroke recurrence. The use of combined radiomics features can improve the prediction efficiency of stroke recurrence in SICAS. In addition, the nomogram has higher prediction performance and good calibration in the training cohort (C-index 0.880) and the validation cohort (C-index 0.817). Therefore, the nomogram is helpful for clinicians to provide decision-making basis for individualized treatment of stroke recurrence in SICAS.
Diabetes mellitus, along with other biomarkers, could be used to predict the risk of stroke recurrence in SICAS. Our results are consistent with those of previous studies and suggest that diabetes may be an independent risk factor for stroke recurrence (Shou et al., 2015). Therefore, to avoid the serious consequences of stroke recurrence, diabetes mellitus as a risk factor should be considered for the treatment and recovery of stroke patients.
This study has some limitations. First, the use of VOI segmentation in this study may have led to subjective errors and affected the accuracy of radiomics analysis. Second, this was a single-center retrospective study with a relatively small sample size, so selection bias cannot be ruled out.
In conclusion, radiomics features are potential biomarkers for predicting stroke recurrence in SICAS. The nomogram constructed by combining clinical high-risk factors, plaque radiological features, and radiomics features is helpful to the individualized risk assessment of predicting the stroke recurrence of SICAS.
Data Availability Statement
The original contributions presented in the study are included in the article/Supplementary Material, further inquiries can be directed to the corresponding author/s.
Ethics Statement
The studies involving human participants were reviewed and approved by the Ethics Committee of Shaanxi Provincial People's Hospital. The patients/participants provided their written informed consent to participate in this study.
Author Contributions
MT, JG, and NM drafted the manuscript and designed the experiment. XY and ZZ performed the statistical analysis. XinZ, JH, XS, XL, and LL collected the data. XL provided technical support. XiaZ contributed to the design of the experiment and also revised the manuscript. All authors contributed to the article and approved the submitted version.
Funding
This research was supported by the Shanxi Provincial Key Research and Development Project of Shaanxi Province of China (2021SF-064).
Conflict of Interest
The authors declare that the research was conducted in the absence of any commercial or financial relationships that could be construed as a potential conflict of interest.
Publisher's Note
All claims expressed in this article are solely those of the authors and do not necessarily represent those of their affiliated organizations, or those of the publisher, the editors and the reviewers. Any product that may be evaluated in this article, or claim that may be made by its manufacturer, is not guaranteed or endorsed by the publisher.
Supplementary Material
The Supplementary Material for this article can be found online at: https://www.frontiersin.org/articles/10.3389/fnins.2022.851353/full#supplementary-material
Supplementary Figure 1. Flow chart of the study eligible patients.
Supplementary Figure 2. Plaque outline presentation. T2WI shows MCA atherosclerotic plaque of M1 segment of right middle cerebral artery (A). Plaque were drawn layer by layer on the T2WI-TSE images (B). The volume of the lesion was sketched along the boundary of the plaque to generate the 3D volume of interest (VOI) (C).
Supplementary Figure 3. An example of using the nomogram to illustrate the individual risk of stroke recurrence in SICAS. A 38-years-old male was showed paroxysmal dysphasia with right limb weakness for 1 week. MRA demonstrates stenosis and plaque of M1 segment of left middle cerebral artery, the plaque burden was 0.83 (A,B, yellow line). The enhancement ratio of plaque was 3.3 (C,D, blue line). No diabetes history (purple line), Rad_score (green line). The values on the Points scale intersected by the lines were added to obtain total points (28 + 16 + 0 + 62 = 106). The total points >82 points, considered as a high-risk patient. The graph revealed that the risk of stroke recurrence in SICAS was over 82% by drawing a vertical line on the Total points scale. Cerebral infarction recurred in the distribution of the left middle cerebral artery after followed up for 6 months.
Abbreviations
ICAS, intracranial atherosclerotic stenosis; VW-HRMRI, vessel wall high-resolution magnetic resonance imaging; HRMRI, high-resolution magnetic resonance imaging; SICAS, symptomatic intracranial atherosclerotic stenosis; AUC, area under the curve; TIA, transient ischemic attack; DWI, diffusion-weighted imaging; MRA, magnetic resonance angiography; CTA, computed tomography angiography; TOF, time-of-flight; T1WI, T1-weighted imaging; T2WI, T2-weighted imaging; FLAIR, fluid-attenuation inversion-recovery; 3D, three-dimensional; TSE, turbo spin-echo; VISTA, volumetric isotropic T2W acquisition; FOV, field of view; TR/TE, repetition time/echo time; WASID, warfarin-Aspirin Symptomatic Intracranial Disease; SI, signal intensity; RI, remodeling index; VOI, volume of interest; ROI, region of interest; GLCM, gray co-occurrence matrix; GLRLM, gray-level run-length matrix; GLSZM, gray-level size zone matrix; NGTDM, neighborhood gray-tone difference matrix; ICC, intraclass correlation coefficient; LASSO, least absolute shrinkage and selection operator; Rad score, radiomics score; DCA, decision curve analysis; ROC, receiver operating characteristic; CI, confidence interval; OR, odds ratio.
References
Aerts, H. J., Velazquez, E. R., Leijenaar, R. T., Parmar, C., Grossmann, P., Carvalho, S., et al. (2014). Decoding tumour phenotype by noninvasive imaging using a quantitative radiomics approach. Nat. Commun. 5:4006. doi: 10.1038/ncomms5006
Altaf, N., Kandiyil, N., Hosseini, A., Mehta, R., MacSweeney, S., and Auer, D. (2014). Risk factors associated with cerebrovascular recurrence in symptomatic carotid disease: a comparative study of carotid plaque morphology, microemboli assessment and the European Carotid Surgery Trial Risk Model. J. Am. Heart Assoc. 3:e000173. doi: 10.1161/JAHA.113.000173
Braman, N. M., Etesami, M., Prasanna, P., Dubchuk, C., Gilmore, H., Tiwari, P., et al. (2017). Intratumoral and peritumoral radiomics for the pretreatment prediction of pathological complete response to neoadjuvant chemotherapy based on breast DCE-MRI. Breast Cancer Res. 19:57. doi: 10.1186/s13058-017-0846-1
Chimowitz, M. I., Lynn, M. J., Howlett-Smith, H., Stern, B. J., Hertzberg, V. S., Frankel, M. R., et al. (2005). Comparison of warfarin and aspirin for symptomatic intracranial arterial stenosis. New Engl. J. Med. 352, 1305–1316. doi: 10.1056/NEJMoa043033
Coull, A. J., and Rothwell, P. M. (2004). Underestimation of the early risk of recurrent stroke: evidence of the need for a standard defifinition. Stroke 35, 1925–1929. doi: 10.1161/01.STR.0000133129.58126.67
Deng, F., Mu, C., Yang, L., Li, H., Xiang, X., and Li, K. (2020). Carotid plaque magnetic resonance imaging and recurrent stroke risk: a systematic review and meta-analysis. Medicine 99:e19377. doi: 10.1097/MD.0000000000019377
Derdeyn, C. P., Chimowitz, M. I., Lynn, M. J., Fiorella, D., Turan, T. N., Janis, L. S., et al. (2014). Aggressive medical treatment with or without stenting in high-risk patients with intracranial artery stenosis (SAMMPRIS): the final results of a randomised trial. Lancet 383, 333–341. doi: 10.1016/S0140-6736(13)62038-3
Gillies, R. J., Kinahan, P. E., and Hricak, H. (2016). Radiomics: images are more than pictures, they are data. Radiology 278, 563–577. doi: 10.1148/radiol.2015151169
Hosseini, A. A., Kandiyil, N., Macsweeney, S. T., Altaf, N., and Auer, D. P. (2013). Carotid plaque hemorrhage on magnetic resonance imaging strongly predicts recurrent ischemia and stroke. Ann. Neurol. 7373, 774–784. doi: 10.1002/ana.23876
Kickingereder, P., Burth, S., Wick, A., Götz, M., Eidel, O., Schlemmer, H. P., et al. (2016). Radiomic profiling of glioblastoma: identifying an imaging predictor of patient survival with improved performance over established clinical and radiologic risk models. Radiology 280, 880–889. doi: 10.1148/radiol.2016160845
Kim, J. M., Jung, K. H., Sohn, C. H., Moon, J., Shin, J. H., Park, J., et al. (2016). Intracranial plaque enhancement from high resolution vessel wall magnetic resonance imaging predicts stroke recurrence. Int. J. Stroke 11, 171–179. doi: 10.1177/1747493015609775
Marnane, M., Merwick, A., Sheehan, O. C., Hannon, N., Foran, P., Grant, T., et al. (2012). Carotid plaque inflammation on 18F-fluorodeoxyglucose positron emission tomography predicts early stroke recurrence. Ann. Neurol. 71, 709–718. doi: 10.1002/ana.23553
Mazighi, M., Tanasescu, R., Ducrocq, X., Vicaut, E., Bracard, S., Houdart, E., et al. (2006). Prospective study of symptomatic atherothrombotic intracranial stenoses: the GESICA study. Neurology 66, 1187–1191. doi: 10.1212/01.wnl.0000208404.94585.b2
Millon, A., Boussel, L., Brevet, M., Mathevet, J. L., Canet-Soulas, E., Mory, C., et al. (2012). Clinical and histological significance of gadolinium enhancement in carotid atherosclerotic plaque. Stroke 43, 3023–3028. doi: 10.1161/STROKEAHA.112.662692
Mortensen, M. B., Dzaye, O., Steffensen, F. H., Bøtker, H. E., Jensen, J. M., Rønnow Sand, N. P., et al. (2020). Impact of plaque burden versus stenosis on ischemic events in patients with coronary atherosclerosis. J. Am. Coll. Cardiol. 76, 2803–2813. doi: 10.1016/j.jacc.2020.10.021
Moulton, K. S., Vakili, K., Zurakowski, D., Soliman, M., Butterfield, C., Sylvin, E., et al. (2003). Inhibition of plaque neovascularization reduces macrophage accumulation and progression of advanced atherosclerosis. Proc. Natl. Acad. Sci. U.S.A. 100, 4736–4741. doi: 10.1073/pnas.0730843100
Prabhakaran, S., Liebeskind, D. S., Cotsonis, G., Nizam, A., Feldmann, E., Sangha, R. S., et al. (2021). Predictors of early infarct recurrence in patients with symptomatic intracranial atherosclerotic disease. Stroke 52, 1961–1966. doi: 10.1161/STROKEAHA.120.032676
Qiao, Y., Zeiler, S. R., Mirbagheri, S., Leigh, R., Urrutia, V., Wityk, R., et al. (2014). Intracranial plaque enhancement in patients with cerebrovascular events on high-spatial-resolution MR images. Radiology 271, 534–542. doi: 10.1148/radiol.13122812
Ran, Y., Wang, Y., Zhu, M., Wu, X., Malhotra, A., Lei, X., et al. (2020). Higher plaque burden of middle cerebral artery is associated with recurrent ischemic stroke: a quantitative magnetic resonance imaging study. Stroke 51, 659–662. doi: 10.1161/STROKEAHA.119.028405
Roquer, J., Segura, T., Serena, J., Cuadrado-Godia, E., Blanco, M., García-García, J., et al. (2011). Value of carotid intima-media thickness and significant carotid stenosis as markers of stroke recurrence. Stroke 42, 3099–3104. doi: 10.1161/STROKEAHA.110.612010
Saam, T., Hatsukami, T. S., Takaya, N., Chu, B., Underhill, H., Kerwin, W. S., et al. (2007). The vulnerable, or high-risk, atherosclerotic plaque: noninvasive MR imaging for characterization and assessment. Radiology 244, 64–77. doi: 10.1148/radiol.2441051769
Salem, M. K., Sayers, R. D., Bown, M. J., West, K., Moore, D., Nicolaides, A., et al. (2012). Patients with recurrent ischaemic events from carotid artery disease have a large lipid core and low GSM. Eur. J. Vasc. Endovasc. Surg. 43, 147–153. doi: 10.1016/j.ejvs.2011.11.008
Samuels, O. B., Joseph, G. J., Lynn, M. J., Smith, H. A., and Chimowitz, M. I. (2000). A standardized method for measuring intracranial arterial stenosis. AJNR Am. J. Neuroradiol. 21, 643–646. doi: 10.1016/S0925-4927(99)00050-5
Shi, Z., Li, J., Zhao, M., Peng, W., Meddings, Z., Jiang, T., et al. (2020). Quantitative histogram analysis on intracranial atherosclerotic plaques: a high-resolution magnetic resonance imaging study. Stroke 51, 2161–2169. doi: 10.1161/STROKEAHA.120.029062
Shi, Z., Li, J., Zhao, M., Zhang, X., Degnan, A. J., Mossa-Basha, M., et al. (2021). Progression of plaque burden of intracranial atherosclerotic plaque predicts recurrent stroke/transient ischemic attack: a pilot follow-up study using higher-resolution MRI. J. Magn. Reson. Imaging 54, 560–570. doi: 10.1002/jmri.27561
Shi, Z., Zhu, C., Degnan, A., Tian, X., Li, J., Chen, L., et al. (2018). Identification of high-risk plaque features in intracranial atherosclerosis: initial experience using a radiomic approach. Eur. Radiol. 28, 3912–3921. doi: 10.1007/s00330-018-5395-1
Shou, J., Zhou, L., Zhu, S., and Zhang, X. (2015). Diabetes is an independent risk factor for stroke recurrence in stroke patients: a meta-analysis. J. Stroke Cerebrovasc. Dis. 24, 1961–1968. doi: 10.1016/j.jstrokecerebrovasdis.2015.04.004
Song, X. W., Zhao, X. H., Liebeskind, D. S., Wang, L. X., Xu, W. D., Xu, Y. L., et al. (2020). Incremental value of plaque enhancement in predicting stroke recurrence in symptomatic intracranial atherosclerosis. Neuroradiology 62, 1123–1131. doi: 10.1007/s00234-020-02418-8
Tian, X., Tian, B., Shi, Z., Wu, X., Peng, W. J., and Zhang, X. F. (2021). Assessment of intracranial atherosclerotic plaques using 3D black-blood MRI: comparison with 3D time-of-flight MRA and DSA. JMRI 53, 469-478. doi: 10.1002/jmri.27341
Tran, B., Dancey, J. E., Kamel-Reid, S., McPherson, J. D., Bedard, P. L., Brown, A. M. K., et al. (2012). Cancer genomics: technology, discovery, and translation. J. Clin. Oncol. 30, 647–660. doi: 10.1200/JCO.2011.39.2316
Underhill, H. R., Hatsukami, T. S., Fayad, Z. A., Fuster, V., and Yuan, C. (2010). MRI of carotid atherosclerosis: clinical implications and future directions. Nat. Rev. Cardiol. 7, 165–173. doi: 10.1038/nrcardio.2009.246
Wabnitz, A. M., Derdeyn, C. P., Fiorella, D. J., Lynn, M. J., Cotsonis, G. A., Liebeskind, D. S., et al. (2019). Hemodynamic markers in the anterior circulation as predictors of recurrent stroke in patients with intracranial stenosis. Stroke 50, 143–147. doi: 10.1161/STROKEAHA.118.020840
Wang, Y., Zhao, X., Liu, L., Soo, Y. O., Pu, Y., Pan, Y., et al. (2014). Prevalence and outcomes of symptomatic intracranial large artery stenoses and occlusions in China: the Chinese Intracranial Atherosclerosis (CICAS) Study. Stroke 45, 663–669. doi: 10.1161/STROKEAHA.113.003508
Xiao, J., Song, S. S., Schlick, K. H., Xia, S., Jiang, T., Han, T. J., et al. (2022). Disparate trends of atherosclerotic plaque evolution in stroke patients under 18-month follow-up: a 3D whole-brain magnetic resonance vessel wall imaging study. Neuroradiol J. 35, 42–52. doi: 10.1177/19714009211026920
Xu, W. H., Li, M. L., Gao, S., Ni, J., Zhou, L. X., Yao, M., et al. (2010). In vivo high-resolution MR imaging of symptomatic and asymptomatic middle cerebral artery atherosclerotic stenosis. Atherosclerosis 212, 507–511. doi: 10.1016/j.atherosclerosis.2010.06.035
Keywords: intracranial arteriosclerosis, plaques, magnetic resonance imaging, stroke, recurrence, nomogram, radiomics
Citation: Tang M, Gao J, Ma N, Yan X, Zhang X, Hu J, Zhuo Z, Shi X, Li L, Lei X and Zhang X (2022) Radiomics Nomogram for Predicting Stroke Recurrence in Symptomatic Intracranial Atherosclerotic Stenosis. Front. Neurosci. 16:851353. doi: 10.3389/fnins.2022.851353
Received: 09 January 2022; Accepted: 15 March 2022;
Published: 12 April 2022.
Edited by:
Tong Tong, Fuzhou University, ChinaReviewed by:
Shuang Xia, Tianjin First Central Hospital, ChinaYe Wu, University of North Carolina at Chapel Hill, United States
Lifang Wei, Fujian Agriculture and Forestry University, China
Copyright © 2022 Tang, Gao, Ma, Yan, Zhang, Hu, Zhuo, Shi, Li, Lei and Zhang. This is an open-access article distributed under the terms of the Creative Commons Attribution License (CC BY). The use, distribution or reproduction in other forums is permitted, provided the original author(s) and the copyright owner(s) are credited and that the original publication in this journal is cited, in accordance with accepted academic practice. No use, distribution or reproduction is permitted which does not comply with these terms.
*Correspondence: Xiaoling Zhang, enhsLjgyMkAxNjMuY29t; Xiaoyan Lei, MTMxNTIxODAyMzVAMTYzLmNvbQ==