- 1Department of Rehabilitation Medicine, The Third Affiliated Hospital of Sun Yat-sen University, Guangzhou, China
- 2Institute of Robotics and Intelligent Systems, School of Mechanical Engineering, Xi’an Jiaotong University, Xi’an, China
- 3Department of Rehabilitation Medicine, Shenzhen Hengsheng Hospital, Shenzhen, China
- 4Air Force Medical Center, PLA, Beijing, China
Several studies have shown the positive clinical effect of brain computer interface (BCI) training for stroke rehabilitation. This study investigated the efficacy of the sensorimotor rhythm (SMR)-based BCI with audio-cue, motor observation and multisensory feedback for post-stroke rehabilitation. Furthermore, we discussed the interaction between training intensity and training duration in BCI training. Twenty-four stroke patients with severe upper limb (UL) motor deficits were randomly assigned to two groups: 2-week SMR-BCI training combined with conventional treatment (BCI Group, BG, n = 12) and 2-week conventional treatment without SMR-BCI intervention (Control Group, CG, n = 12). Motor function was measured using clinical measurement scales, including Fugl-Meyer Assessment-Upper Extremities (FMA-UE; primary outcome measure), Wolf Motor Functional Test (WMFT), and Modified Barthel Index (MBI), at baseline (Week 0), post-intervention (Week 2), and follow-up week (Week 4). EEG data from patients allocated to the BG was recorded at Week 0 and Week 2 and quantified by mu suppression means event-related desynchronization (ERD) in mu rhythm (8–12 Hz). All functional assessment scores (FMA-UE, WMFT, and MBI) significantly improved at Week 2 for both groups (p < 0.05). The BG had significantly higher FMA-UE and WMFT improvement at Week 4 compared to the CG. The mu suppression of bilateral hemisphere both had a positive trend with the motor function scores at Week 2. This study proposes a new effective SMR-BCI system and demonstrates that the SMR-BCI training with audio-cue, motor observation and multisensory feedback, together with conventional therapy may promote long-lasting UL motor improvement.
Clinical Trial Registration: [http://www.chictr.org.cn], identifier [ChiCTR2000041119].
Introduction
Stroke is a leading cause of mortality and disability worldwide (Johnson et al., 2019; Zhou et al., 2019). Up to 66% of stroke survivors experience upper limb (UL) motor impairments, which result in functional limitations in activities of daily living and decreased life quality (Kwah et al., 2013; Morris et al., 2013).
Electroencephalography (EEG)-based sensorimotor rhythm (SMR) brain computer interface (BCI) is a novel technology that can enhance activity-dependent neuroplasticity and restore motor function for stroke survivors (Ang et al., 2014a; Lazarou et al., 2018; Jeunet et al., 2019). SMRs can be measured over the sensorimotor cortex and modulated by actual movement, motor intention, or motor imagery (MI; Frenkel-Toledo et al., 2014; Yuan and He, 2014). Task-related modulation in EEG-based SMRs is usually manifested as event-related desynchronization (ERD) or event-related synchronization (ERS) in low-frequency components [mu rhythm (8–12 Hz) and beta rhythm (13–26 Hz)] (Pfurtscheller and Lopes da Silva, 1999), which forms the basis of neural control in EEG-based SMR-BCI (Yuan and He, 2014). Furthermore, patients with stroke or spinal cord lesions can control physical or virtual devices via SMR-BCI (Prasad et al., 2010; Caria et al., 2011; Ang et al., 2014a,b; Dodakian et al., 2014; McCrimmon et al., 2014; Ono et al., 2014; Yuan and He, 2014; Ang and Guan, 2015; Bartur et al., 2015; Pichiorri et al., 2015; Zich et al., 2015; Shu et al., 2017, 2018; Barsotti et al., 2018; Biasiucci et al., 2018; Lazarou et al., 2018; Lee et al., 2018; Norman et al., 2018; Jeunet et al., 2019; Song and Kim, 2019; Chen et al., 2020; Foong et al., 2020), which raises the possibility of SMR-BCI training for stroke rehabilitation.
Several clinical studies have investigated the effect of SMR-BCI systems and demonstrated the significantly positive outcomes on motor function improvement for stroke patients (Ang and Guan, 2015). Ramos-Murguialday et al. (2013) and Ang et al. (2014a,b) stated the BCI training had better efficacy than sham-BCI for stroke rehabilitation. Besides, Cantillo-Negrete et al. (2021) investigated the clinical and physiological effects of SMR-BCI intervention and conventional therapy for upper limb stroke rehabilitation and a revealed similar positive impact of the two therapy methods. Thus, SMR-BCI training, together with conventional therapy, is a suitable therapy option for stroke recovery.
To improve the efficacy of SMR-BCI, various SMR-BCI systems combined with sensory stimulation, motor observation (MO) have been proposed. Shu et al. (2017, 2018) and Ren et al. (2020) improved the SMR-BCI performance via proprioceptive stimulation before the motor imagery (MI) task. Choi et al. (2019), Nagai and Tanaka (2019), and Fujiwara et al. (2021) found users’ ERD/ERS was enhanced when they performed MI task with motor observation. It is recognized that enhanced ERD/ERS of stroke patients, meaning enhanced motor-related cortical activation (Pfurtscheller and Lopes da Silva, 1999; Pfurtscheller et al., 2006b), can improve users’ engagement and decoding accuracy for BCI system, which could help maximize brain plasticity and restore motor and cognitive function for stroke patients (Bundy et al., 2017; Nagai and Tanaka, 2019). Furthermore, Velasco-Álvarez et al. (2013) designed an audio-cued SMR-BCI system and showed its availability.
Besides, various neuro-feedback has been added to make SMR-BCI system a closed loop for better effect on stroke recovery. Ramos-Murguialday et al. (2013) and Ang et al. (2014a,b, 2015) demonstrated that SMR-BCI with robotic feedback was the most popular feedback method and had positive efficacy for stroke rehabilitation. Pichiorri et al. (2015) and Foong et al. (2020) observed that SMR-BCI with visual feedback showed its excellence for stroke recovery. Auditory feedback may also improve SMR-BCI performance (Nijboer et al., 2008; McCreadie et al., 2013, 2014). Several researchers found the users’ ERD/ERS was improved via SMR-BCI with proprioceptive feedback (Vukelić and Gharabaghi, 2015; Barsotti et al., 2018).
For stroke patients, the ability to keep attention is weakened due to of brain damage. To enhance the ERD/ERS and maximize the efficacy of BCI training, we propose a new SMR-BCI system with audio-cue, MO, and multisensory (auditory, visual, and robotic) feedback and investigate the effectiveness of this system.
Another urgent investigation, which should be further explored, is optimal and safe exercise prescription (e.g., training intensity and duration) (Farrell et al., 2020; Luo et al., 2020). We used the definition of training intensity and duration in a review (Antje et al., 2020) as a reference: (1) training intensity (high: five times per week vs. moderate: 2–3 times per week), (2) training duration (short: 2–3 weeks vs. long: 4–8 weeks). Most of the SMR-BCI intervention proposed fell into the pattern of moderate training intensity with long training duration, involving 10 sessions (twice a week) (McCrimmon et al., 2014), 12 sessions (three times a week) (Ang et al., 2014a; Pichiorri et al., 2015; Chen et al., 2020), 18 sessions (three times a week) (Foong et al., 2020), 20 sessions (daily training exclude weekends) (Ramos-Murguialday et al., 2013; Wu et al., 2020) and 24 sessions (twice a week) (Sebastián-Romagosa et al., 2020), which have shown positive effects on stroke rehabilitation. Few studies have addressed the pattern of high training intensity with short training duration. One clinical trial involved 10 training sessions, but each session of BCI training lasted up to 40 min (Frolov et al., 2017). As our group suggests, motor function recovery and the brain networks of stroke patients could be improved significantly by 4-week SMR-BCI intervention combined with convention training compared to only conventional treatment (Wu et al., 2020), which leads us to ponder whether a high training intensity with short duration SMR-BCI intervention will get better influence. If that works, stroke patients will restore the ability to live independently faster.
As mentioned above, there are two purposes of this study. Firstly, to investigate the efficacy of non-invasive EEG-based SMR-BCI with audio-cue, MO, and multisensory (robotic, visual, and auditory) feedback, together with conventional therapy, for upper limb rehabilitation of stroke patients. Secondly, to discuss the influence of stroke rehabilitation after a high training intensity with short duration SMR-BCI intervention.
Materials and Methods
Subjects
All patients were recruited from The Third Affiliated Hospital of Sun Yat-sen University.
The following inclusion criteria were applied: (1) age between 18 and 75 years; (2) hemiparesis resulting from a unilateral brain lesion, as confirmed by magnetic resonance imaging (MRI), with a time since stroke (TSS) of 6–24 weeks before study enrollment; (3) moderate to severe hand paralysis, as determined by a Brunnstrom score <IV; (4) sufficient cognition to follow simple instructions and understand the purpose of the study (Susanto et al., 2015).
The exclusion criteria were: (1) recurrent stroke; (2) other neurological, neuromuscular, orthopedic diseases; (3) shoulder or arm contracture/pain; (4) severe aphasia, dementia, psychotic symptoms, or a scalp deformity due to surgery, or those who could not undergo EEG recording for other reasons, such as involuntary movements; or (5) receiving other clinical central nervous system interventions (Susanto et al., 2015).
Based on experience, EEG acquisition devices used on women got poor signals because of their long hair, so we tried to select male patients.
From 79 potentially eligible patients, 24 stroke survivors were allocated to the intervention and received follow-up analysis (see Figure 1). Twenty-four patients were randomly assigned into one of the two groups: (1) the BCI therapy group (BCI Group, BG), and (2) the non-BCI group (Control Group, CG).
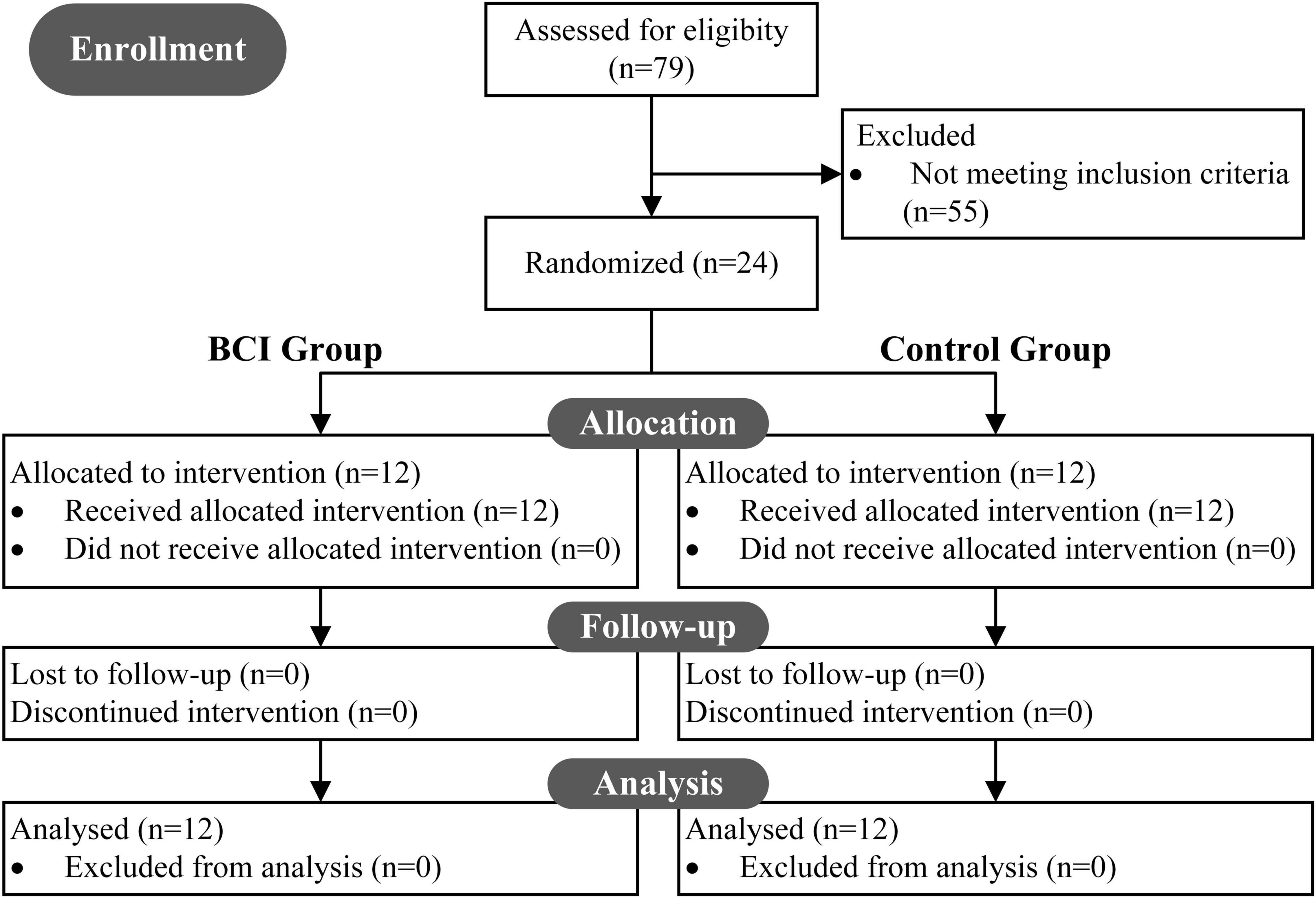
Figure 1. CONSORT diagram: a flow from recruitment through follow-up and analysis. In all, 79 patients were screened to be eligible for the study, and 55 were excluded. 24 underwent intervention and were randomly assigned to two groups (BCI group, BG; control group, CG). All 24 recruited patients received follow-up analysis.
Study Design
All subjects were recruited to receive a total of 10 training sessions, lasting for 3 h per day, 5 days per week (excluding weekends). Each training session consisted of 1-h BCI therapy and 2-h conventional treatment for the BG, while only 3-h conventional treatment for the CG.
Each SMR-BCI intervention consisted of one calibration session and about ten BCI training sessions (1 h). Conventional treatment included physiotherapy and occupational therapy involving shoulder, elbow and hand training: neuromuscular electrical stimulation, passive joint activity, strength training, stretch and Activities of Daily Living (ADL) training. Specifically, for the patients who belonged to the BG, the hand training part in conventional treatment was excluded.
The clinical measure scales were measured at three time points: at baseline (Week 0), at post-intervention (Week 2), and at follow-up week (Week 4). Notably, the patients in both groups were still hospitalized after 2 weeks of intervention and received conventional therapy.
Sensorimotor Rhythm-Brain Computer Interface System Description
The SMR-BCI system is shown in Figure 2. Patients wore EEG caps with 16 active electrodes (g.Nautilus, g.tec medical engineering GmbH, Austria) and the affected hand wore an exoskeleton hand robot (RHB-III. Shenzhen Rehab Medical Technology Co., Ltd., China). The patients were advised to avoid blinking, coughing, chewing and minimize any body movements when performing tasks.
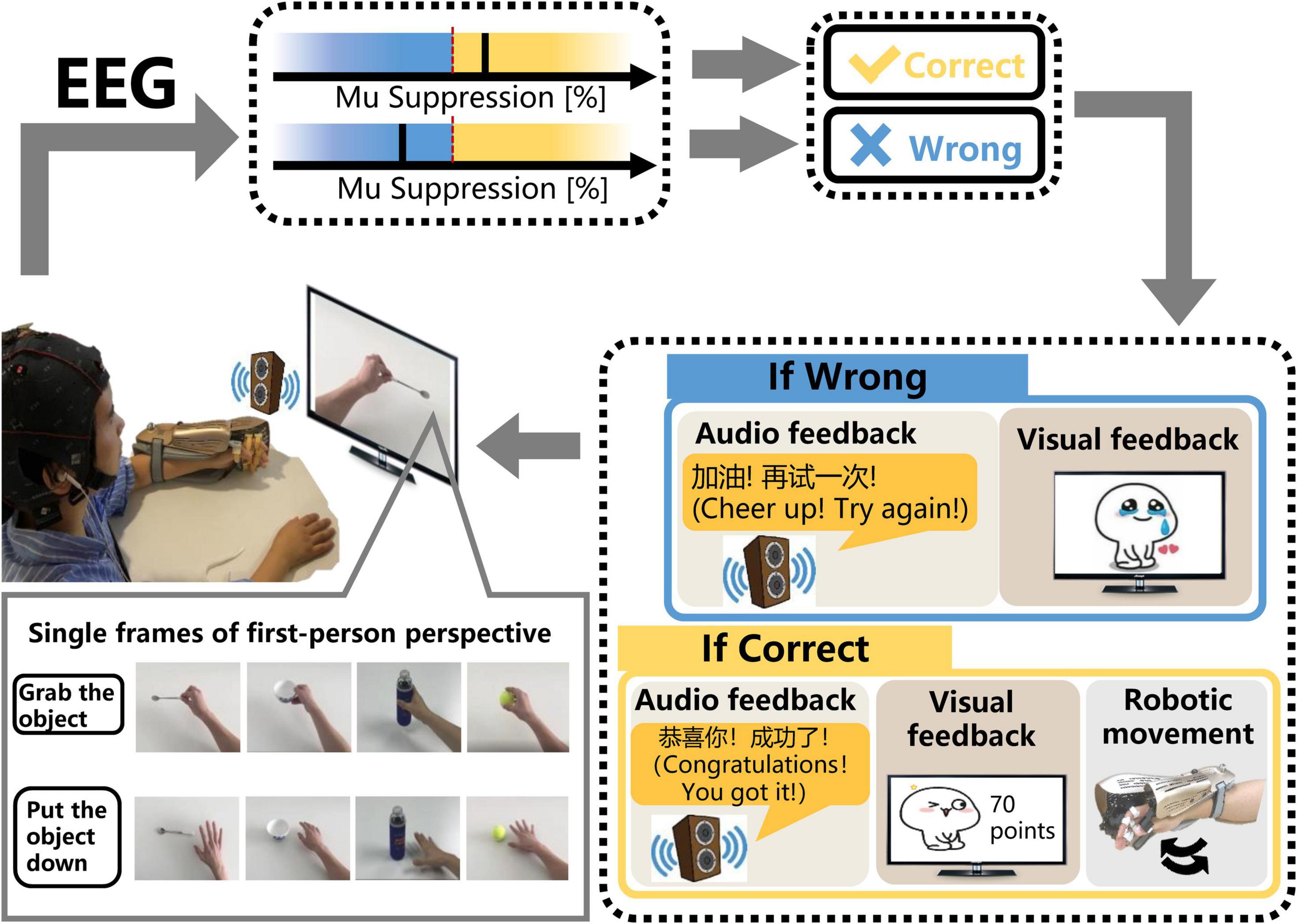
Figure 2. The schematic diagram of the SMR-BCI system. According to audio-cue, subjects imagine “grabbing the object” or “putting the object down” accompanied by observing the video. BCI system calculates the mu suppression of subjects’ EEG data and recognizes patients’ intention via comparing the mu suppression value with the threshold. If the purpose is identified correctly, the system will give multisensory (robotic, auditory, and visual) feedback. On the contrary, the system will still provide corresponding auditory and visual feedback, but the robot will maintain the previous state.
After undergoing a calibration session, including only one trial (described in the section “Sensorimotor Rhythm-Brain Computer Interface Session”), subjects performed MI training sessions according to the audio-cue and observed the corresponding video on the monitor. If the mu suppression was detected in the motor intention classification area (yellow shading), the exoskeleton hand would assist the paretic hand in grasping or opening action according to the MI task cued on the video. Once the robot was triggered, it would complete the movement regardless of the mu suppression during the motion. Then, the system provided audiovisual feedback with a training score (see Figure 2). In contrast, the robot would maintain the previous state and the BCI system would give corresponding audiovisual feedback (see Figure 2) if the mu suppression didn’t reach the threshold (60%) and was in the rest area (blue area) within 12 s. During the time of performing MI task, the BCI system would detect the mu suppression of the subject three times, each time lasting 4 s. If the mu suppression was tested above the threshold in the first 4 s, the feedback meaning “successful” would be given. If not, the BCI system would monitor the mu suppression for the next 4 s, and so on, until 12 s.
The mu suppression (Oberman et al., 2008; Sun et al., 2017) of EEG SMR recorded by electrodes was used for a brain-controlled switch. EEG acquisition and processing details are described in section “Electroencephalography Acquisition, Processing, and Analysis.”
Sensorimotor Rhythm-Brain Computer Interface Session
Before conducting MI sessions, patients first performed a calibration trial, to get the “Idle-state Potential,” where subjects were instructed to keep still at resting state with eyes closed, called “idle task.” A calibration trial lasted about 60 s. Figure 3A shows the timing of a calibration trial.
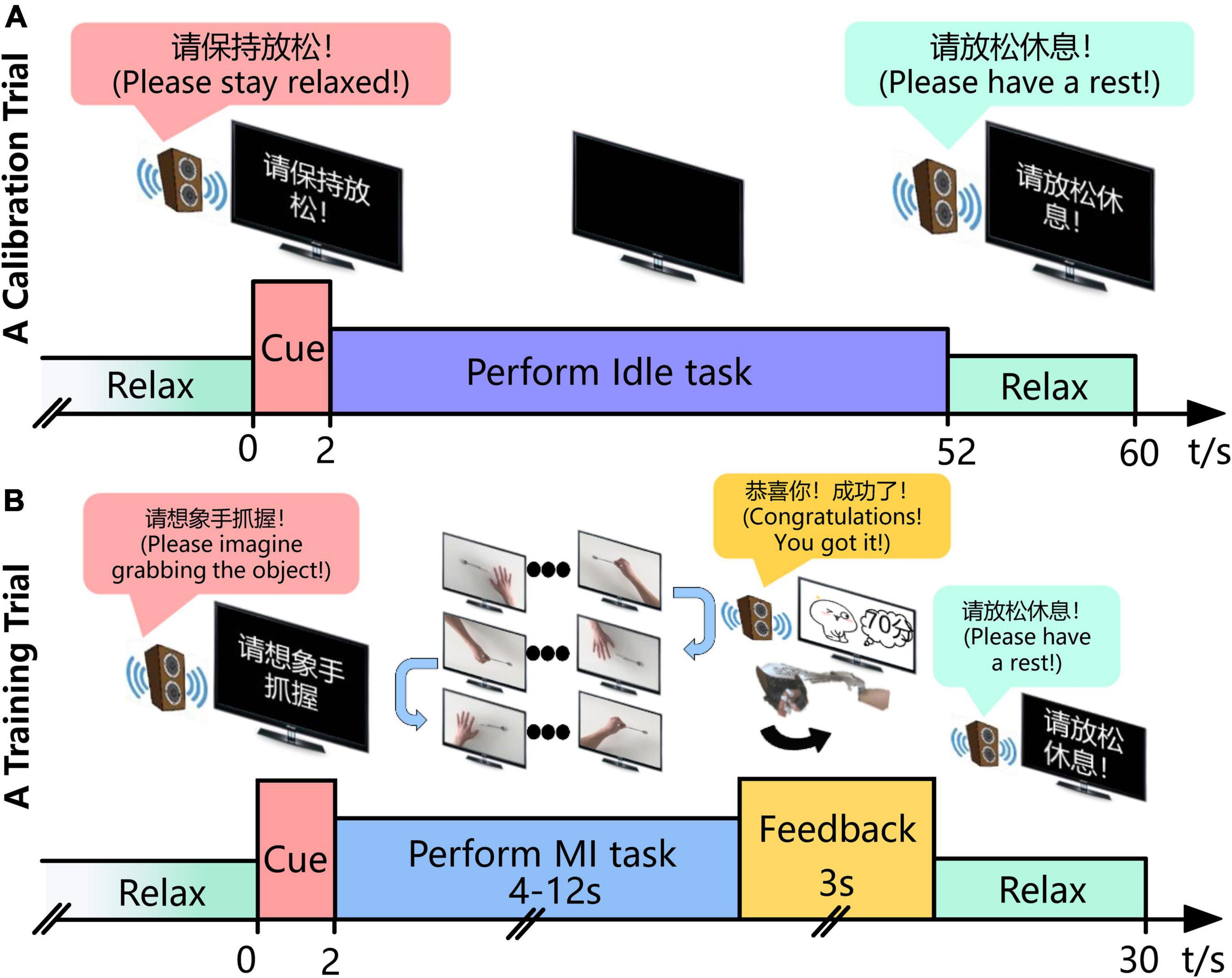
Figure 3. Experimental time course of the BCI intervention for stroke patients’ rehabilitation. (A) In the calibration trial, the patient gets an audio cue to keep still and eyes closed for 50 s and the EEG when performing the “Idle task” is collected. (B) In the training trial, the subject imagines, for instance, “grabbing the objects” according to the audio-cue and monitor from the first-person perspective, third-person perspective, or inverse first-person perspective in turn until his/her intention is recognized. Then, the system gives the corresponding auditory, visual and/or robotic feedback during the following 3 s. The MI task of “grabbing the object” and “putting the thing down” is cued alternatively if the intention is identified successfully, or it maintains the previous one.
Each MI training session completed approximately 10 runs, each consisting of 10 trials. A break of 3–5 min was given after each run. Figure 3B shows the timing of a training trial. Each trial lasted 30 s. Subjects performed the MI task according to audio-cue and the video on the monitor. In addition, the video of three perspectives (first-person perspective, third-person perspective, and inverse first-person perspective) was played in turn. Once the mu suppression of the patient exceeded the threshold, the system would give the corresponding robotic, auditory, and visual feedback.
Electroencephalography Acquisition, Processing, and Analysis
Electroencephalography was recorded using g.Nautilus headset (g.tec medical engineering GmbH, Austria), which provided 16 active electrodes placed in the international 10–20 system positioning: FP1, FP2, FC3, FZ, FC4, C1, C2, CZ, C3, C4, CP3, PZ, CP4, PO7, PO8, and POz. The reference electrode and ground electrode were located on the right mastoid and left mastoid, respectively. Impedances for all electrodes were maintained at <5 kΩ throughout the experiment. Raw EEG recordings were sampled at 256 Hz. Signals were also processed in real-time by the amplifier using an analog bandpass filter (0.5–60 Hz) and a notch filter (48–52 Hz) to remove artifacts and power line interference.
After preprocessing, the EEG of C3/C4 electrodes covered over the primary motor cortex was used for BCI control. The signals were processed by a bandpass filtered (4th order Butterworth filter) between mu rhythm (8–12 Hz) with a Hamming window. Mu suppression reflects an ERD of the EEG caused by an increase in neural activity (Sun et al., 2017), which is used for the value of recognition in this BCI system. The mu suppression score was calculated according to the following equation (Braadbaart et al., 2013):
where muSupp represents the mu rhythm suppression value, muPtask represents the mu rhythm power of EEG while performing “MI task,” and muPidle is the mu rhythm power of EEG while performing “Idle task.”
In the offline analysis, the EEG recorded by channel C3 and channel C4 was analyzed. The segments containing gross artifacts (identified by visual inspection) were excluded for further analysis. These EEG processes were carried out in MATLAB (The MathWorks, Inc., Natick, MA, United States).
Firstly, we analyzed the mu suppression as the equation (1) and its correlation with motor function scores. The following method was used to compute the mu suppression value:
1. Bandpass filtering of 8–12 Hz on the EEG recorded during 2-week SMR-BCI training session. While the EEG on time segment 5–45 s (see Figure 3A) was analyzed for idle-state, the EEG when performing MI tasks at Week 0 and Week 2 (see Figure 3B) was investigated for MI-state.
2. Squaring the bandpass-filtered samples to obtain power samples.
3. Computing the power value when performing “Idle task” by averaging the idle-state power samples.
4. Computing the power value when performing “MI task” by averaging the MI-state power samples in one session.
5. Computing the mu suppression using the equation (1).
Outcome Measures
Fugl-Meyer Assessment-Upper Extremities (FMA-UE) assessment scale was used as the primary motor function outcome measure because of its high reliability. In this study, FMA-UE only referred to the upper extremity motor function part with a total score of 66 (Fuglmeyer et al., 1975; Page et al., 2012).
Secondary outcome measures were the Wolf Motor Function Test (WMFT; Wolf et al., 2001) and the Modified Barthel Index (MBI; Mahorney, 1965). The WMFT consists of 15 tasks (six joint-segment tasks, nine functional tasks; maximum score = 75), each of which should be performed within 120 s. The MBI is used to measure performance in ADL.
Mean change of FMA-UE and WMFT scores were compared with its estimated minimal clinically significant difference (MCID) values (Der Lee et al., 2001; Page et al., 2012) and estimated minimal detectable change (MDC) value (Lin et al., 2009), respectively.
Statistical Method
All demographic and clinical data were analyzed using SPSS version 23.0 (IBM Inc., Chicago, IL, United States). The variables tested normal (using Shapiro–Wilk test) were expressed as the mean ± standard deviation, and the two-tailed unpaired t-test was used for intergroup comparison while the two-tailed paired t-test was for intragroup comparison. Non-normally distributed data were expressed as the median with 25 and 75% quartile, and the Mann–Whitney U test was applied for intergroup comparison while the Wilcoxon ranked sum test was for intragroup comparison.
Two-way repeated measures ANOVA was performed for the functional scale scores (FMA-UE, WMFT, and MBI) with time (Week 0, Week 2, and Week 4) as the within-subject factor.
Associations between the clinical scores (at Week 2) and the mu suppression (at Week 2) were assessed using Pearson’s (if the two variables are both normally distributed), or Spearman’s correlation. Statistical significance was set at p < 0.05 for all analyses.
Results
Demographics
The demographic information of the patients (at baseline) is shown in Table 1. For demographic information, standard variables, including age and clinical scale scores, were analyzed by the parametric tests while TSS was analyzed by the non-parametric test. The chi-square test was used to identify difference in rates among the groups.
There were no significant demographic differences in age (two-tailed unpaired t-test, p = 0.054), sex (all males), and affected hand (chi-square test, p = 0.653). Similarly, there were no significant differences in stroke type (chi-square test, p = 0.386), and TSS (Mann–Whitney U test, p = 0.579). In addition, patients in the two groups had similar levels of baseline clinical scores including FMA-UE (two-tailed unpaired t-test, p = 0.795), WMFT (two-tailed unpaired t-test, p = 0.859) and MBI (two-tailed unpaired t-test, p = 0.397).
Efficacy Measurements
Two-way repeated-measures ANOVA (FMA-UE, WMFT, and MBI, by Week 4, with time as the within-subjects factor and group as the between-subjects factor) showed a significant time × group interaction on FMA-UE (F = 18.629, p < 0.01) and WMFT (F = 10.252, p = 0.001) and no significant time × group interaction on MBI (F = 0.500, p = 0.613). The results showed that time had a significant effect on FMA-UE (F = 120.626, p < 0.01), WMFT (F = 121.760, p < 0.01), and MBI (F = 17.228, p < 0.01), but no significant effect for group on FMA-UE (F = 0.005, p = 0.947), WMFT (F = 0.180, p = 0.675), and MBI (F = 1.614, p = 0.217).
Fugl-Meyer Assessment-Upper Extremities, WMFT, and MBI scores of the BG and that of the CG were significantly improved in Week 2 and Week 4. The results are shown in the Table 2. No significant differences were found between groups for clinical scores at any measurement point.
As shown in Table 3, overall improvements of outcome measure scores of the BG were higher than that of the CG, and BG had significant improvement differences in FMA-UE and WMFT changes at Week 4 (Week 0-based change) compared to the CG.
Importantly, 5.25 points and 5.55 points have been estimated to represent the minimal clinically significant difference (MCID) of FMA-UE (Cervera et al., 2018) and minimal detectable change (MDC) of WMFT (Susanto et al., 2015), respectively. At Week 4, the increase of FMA-UE and WMFT surpassed MCID and MDC for all the patients in the BG (Figure 4).
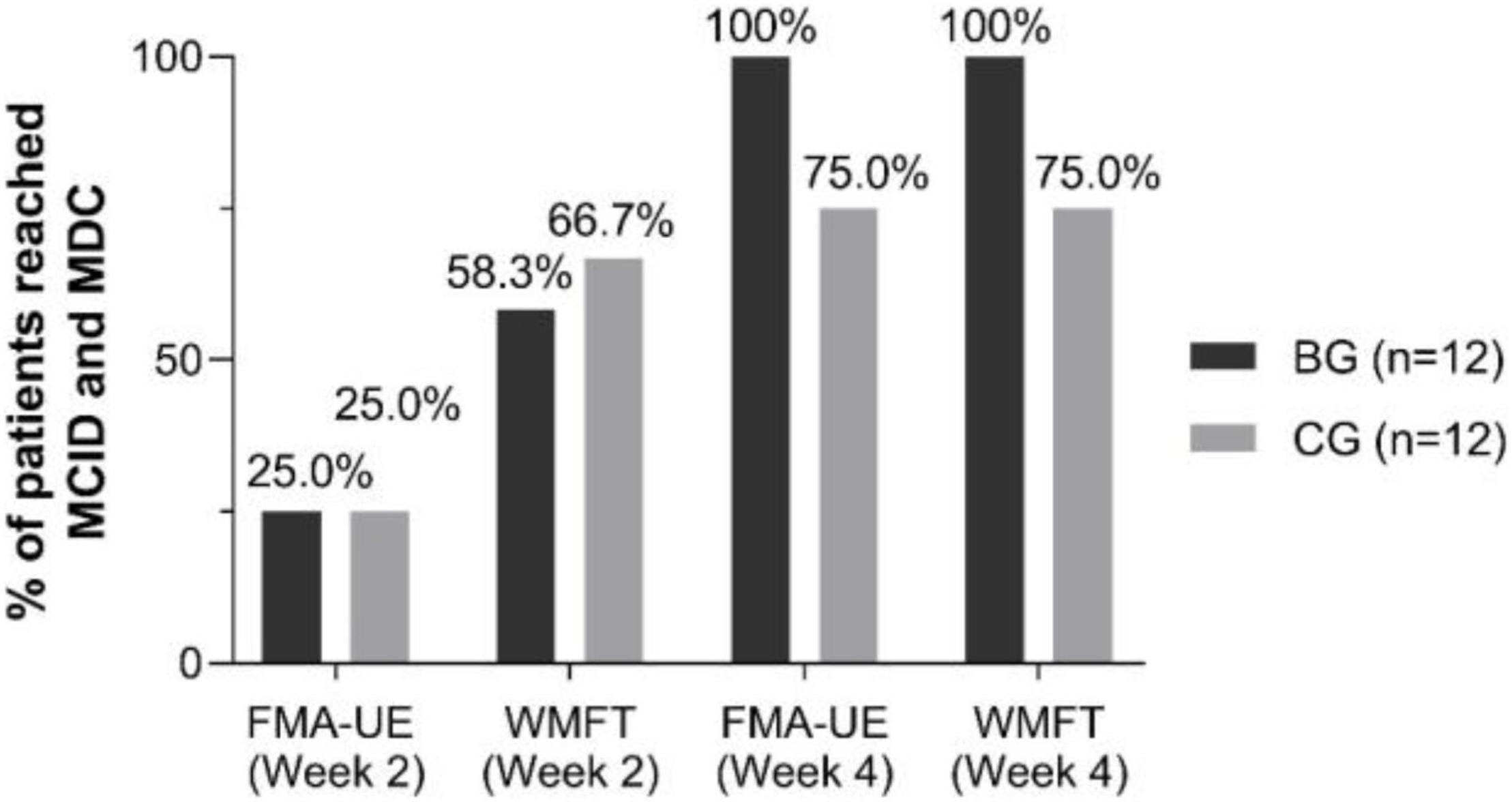
Figure 4. Percent of patients reached minimal clinically important difference (MCID) and minimal detectable change (MDC) by FMA-UE and WMFT scores in each group at Week 2 and Week 4.
Electroencephalography Results
The mu suppression values of bilateral cortex were compared before and after MI-BCI training (Figure 5A). No significant change in the bilateral hemisphere was found after BCI training [Ipsilesional hemisphere, muSuppWeek0 = 45.7735 ± 28.0009, muSuppWeek2 = 56.8294 (47.9067, 60.6983), Wilcoxon ranked sum test, p = 0.875; Contralesional hemisphere, muSuppWeek0 = 62.4475 (21.4197, 72.9325), muSuppWeek2 = 53.8142 ± 25.9915, Wilcoxon ranked sum test, p = 0.388]. Also, there was no significant difference in mu suppression between hemispheres (Week 0: Mann–Whitney U test, p = 0.670; Week 2: Mann–Whitney U test, p = 0.768).
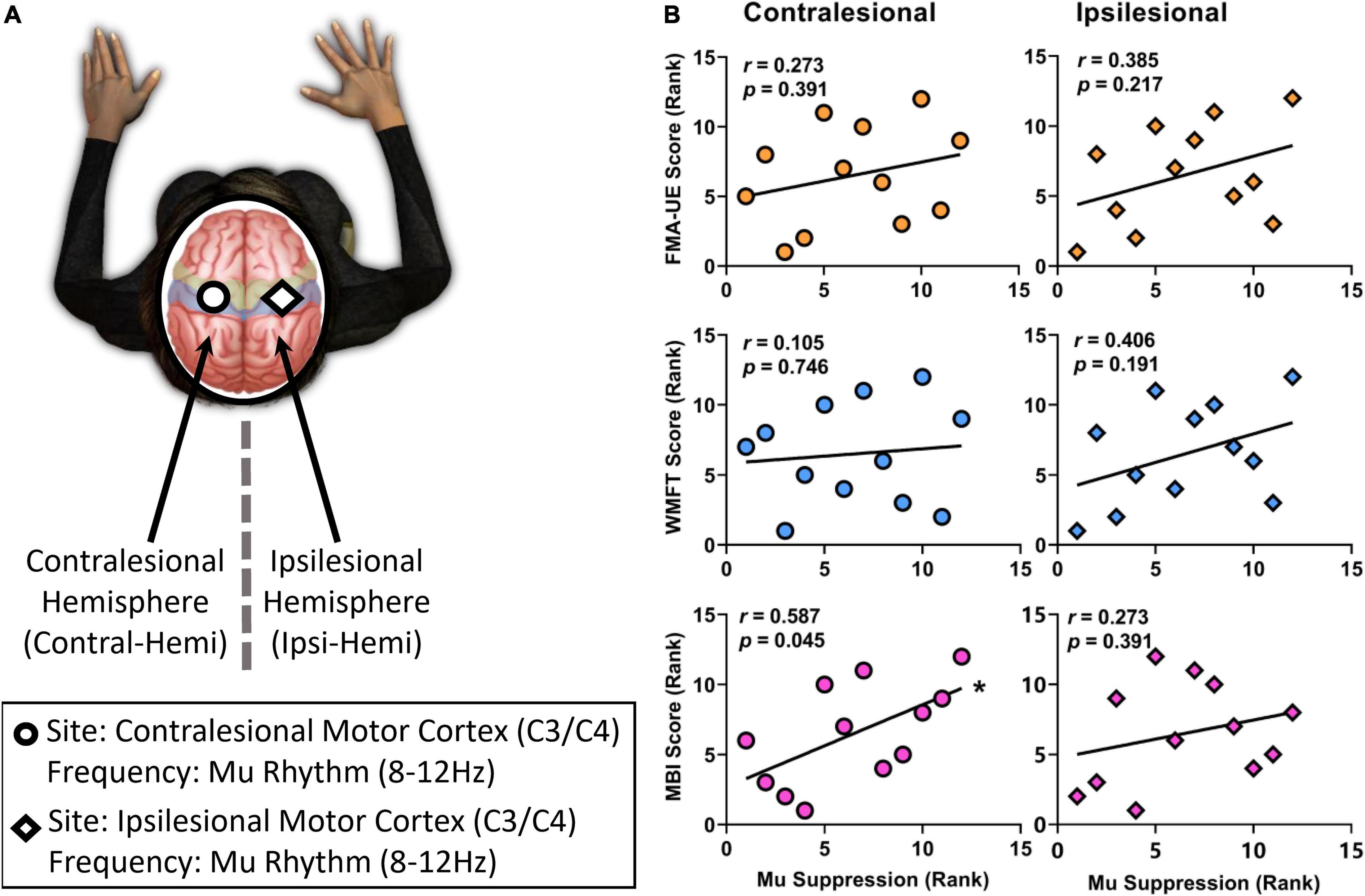
Figure 5. The correlation between the ranked mu suppression value and the ranked clinical scale scores (FMA-UE, WMFT, MBI scores). (A) Analyses were performed using EEG activity at the frequency used for BCI control but an electrode in the contralesional hemisphere, at the frequency used for BCI control but an electrode in the ipsilesional hemisphere. (B) While there was a significantly positive correlation between mu suppression of contralesional hemisphere and MBI scores after 2-week BCI intervention, others showed no significantly positive trend. Significant (p < 0.05) changes are indicated with an *.
Correlation analysis was performed to assess the relationship between the ranked mu suppression (Week 2) and the ranked clinical scale scores (Week 2) in the BG (Figure 5B). After 2-week comprehensive rehabilitation, including BCI and conventional interventions, the mu suppression of contralesional hemisphere had a significantly positive correlation with MBI scores (Pearson r = 0.587, p = 0.045). While there was no significant correlation between mu suppression of contralesional hemisphere and FMA-UE (Pearson r = 0.273, p = 0.391), or WMFT (Pearson r = 0.105, p = 0.746), they had a positive correlation trend. On the ipsilesional motor cortex, there was also a positive correlation trend between mu suppression and motor function (FMA-UE: Pearson r = 0.385, p = 0.217; WMFT: Pearson r = 0.406, p = 0.191; MBI: Pearson r = 0.273, p = 0.391). Pearson’s r was used for all variables (two-tailed tests).
Discussion
This study presents the results from a clinical study investigating the efficacy of the SMR-BCI with audio-cue, motor observation, and multisensory (robotic, auditory, and visual) feedback compared with conventional therapy for upper limb stroke rehabilitation.
In terms of clinical scale scores (FMA-UE, WMFT, and MBI score), upper limb motor functional improvement was observed in both groups after 2-week intervention. This result is consistent with previous evidence demonstrating the effectiveness of BCI intervention for stroke patients’ UL motor function recovery (Cervera et al., 2018; Wu et al., 2020) and a randomized controlled multicenter trial with post-stroke patients (in subacute and chronic phase), which showed significant improvements of FMA-UE scores in BCI and control group (Frolov et al., 2017). The clinical scale scores of the BG had a significantly greater motor function improvement than the CG at the follow-up week rather than Week 2. Similarly, the percentage of patients reaching MCID and MDC in the BG was greater than that in the CG at Week 4 instead of Week 2. This phenomenon may reflect the effectiveness of the long-term clinical effects of SMR-BCI intervention for post-stroke patients (Wu et al., 2020). BCI training added to conventional therapy may enhance motor functioning of the upper extremity and brain function recovery in patients after a stroke (Kruse et al., 2020). Compared to conventional interventions, we suggest BCI-based training for motor recovery of the upper limbs in patients with stroke (Nojima et al., 2021).
Mu rhythms are suppressed, and their power is attenuated, when engaging in motor activity (Gastaut, 1952), observing actions executed by someone else (Muthukumaraswamy et al., 2004), or imagining performing an action (Pfurtscheller et al., 1996, 2006a,2010). Thus, researchers usually link the mu suppression of motor cortex to the motor-relevant brain activation (Pineda, 2006). In this study, no significant difference in mu suppression value was found after 2-week BCI intervention. However, there was a positive trend between functional motor scores (FMA-UE, WMFT, and MBI) with mu suppression of bilateral hemisphere. This positive trend agrees with Bundy et al.’s (2017) study that suggests bilateral cortex activation, especially unaffected cortex activation, can contribute to the motor-relevant task of subacute stroke patients (Calautti et al., 2001). Neuronal reorganization may occur on both the ipsilesional and contralesional hemispheres during recovery to regain motor function and therefore bilateral activation for the hemiparetic side is often observed (Dodd et al., 2017). Meanwhile, this activation pattern gives a possible method to control the SMR-BCI system for stroke rehabilitation reliably via the bilateral cortex EEG-based information fusion (Bundy et al., 2017). Therefore, the unaffected hemisphere may play a role in motor recovery following stroke (Gould et al., 2021). However, there is an opinion that increased activation in the intact hemisphere may hinder reorganization in the lesioned hemisphere, which may have a negative impact on recovery. In response to the two contradictory views, a bimodal balance-recovery model that links interhemispheric balancing and functional recovery to the structural reverse was suggested (Di Pino et al., 2014). As our previous work has shown that motor function recovery and the brain networks of stroke patients could be improved significantly by 4-week SMR-BCI intervention. We conjecture that this bilateral activation, as well as the positive correlations between mu suppression and clinical scores, is a “middle state” for the patients in this study and may also mean that a 2-week training period would not be the optimal option for neurorehabilitation. This result is consistent with the conclusion that a BCI training with conventional therapy for a duration of 4 weeks or longer, with a high intensity training of five times per week, was recommend (Kruse et al., 2020). The influence of the training duration combined with the training intensity needs to be investigated further.
Several features distinguish this work from previous studies. Firstly, we developed a new SMR-BCI system for stroke rehabilitation. This BCI system showed a more vivid training experience for patients via audio-cue, motor observation, and multisensory (robotic, auditory, and visual) feedback to make subjects deeply involved in the training. Secondly, this study revealed the clinical efficacy of the SMR-BCI system we designed for post-stroke rehabilitation. Thirdly, the prescription (e.g., the intensity/frequency and the duration) of BCI training was discussed. We provided a training program (high training intensity, short training duration, and 1-h training session), which may help to develop a full picture of the clinical factor and the interaction between training duration and training intensity.
There were also several limitations to note. The small sample of participants and the absence of EEG data from the CG and follow-up week limited the further investigation of our findings on efficacy. Although the influence of patients’ age and type of lesion in motor recovery of upper extremities of post-stroke patients need further analysis (Kruse et al., 2020), there are some views that relatively younger and more hemorrhagic stroke participants in the BG may tip off the outcome measures comparison between the groups.
Conclusion
In summary, this study showed the clinical efficacy of SMR-BCI with audio-cue, MO, and multisensory (robotic, auditory, and visual) feedback for post-stroke rehabilitation. Moreover, it discussed the impact of the high training intensity BCI training with short training duration.
Clinical efficacy was measured by three clinical measure scales (FMA-UE, WMFT, and MBI), and the results showed significant improvements at Week 2 and Week 4. Notably, there was a greater improvement for patients who belonged to the BG than the CG at Week 4.
Hence, in the future, more extensive clinical trials are warranted to verify the clinical efficacy and the role of this kind of BCI system has in the rehabilitation milieu. The discussion about the interaction between training intensity and duration also motivates further research.
Data Availability Statement
The raw data supporting the conclusions of this article will be made available by the authors, without undue reservation.
Ethics Statement
The studies involving human participants were reviewed and approved by The Third Affiliated Hospital of Sun Yat-sen University, Guangzhou, China. The patients/participants provided their written informed consent to participate in this study.
Author Contributions
XL, LW, SM, ZY, ZT, LS, YZ, XW, SW, JW, and ZD worked together to complete the manuscript. XL, LW, SW, JW, and ZD contributed to conception and design of the study. LW and SM carried out the data analysis. LS carried out the experiments. ZY and ZT provided statistical assistance and support. YZ and XW provided opinions on grammar and rhetoric. All authors contributed to manuscript revision, read and approved the submitted version.
Funding
This work was supported by the Key R&D Program of Guangdong Province, China under grant 2018B030339001 and Key Realm R&D Program of Guangzhou, China under grant 202007030007.
Conflict of Interest
The authors declare that the research was conducted in the absence of any commercial or financial relationships that could be construed as a potential conflict of interest.
Publisher’s Note
All claims expressed in this article are solely those of the authors and do not necessarily represent those of their affiliated organizations, or those of the publisher, the editors and the reviewers. Any product that may be evaluated in this article, or claim that may be made by its manufacturer, is not guaranteed or endorsed by the publisher.
References
Ang, K. K., Chua, K. S. G., Phua, K. S., Wang, C., Chin, Z. Y., Kuah, C. W. K., et al. (2014a). A randomized controlled trial of eeg–based motor imagery brain-computer interface robotic rehabilitation for stroke. Clin. EEG Neurosci. 46, 310–320. doi: 10.1177/1550059414522229
Ang, K. K., and Guan, C. (2015). Brain–computer interface for neurorehabilitation of upper limb after stroke. Proc. IEEE 103, 944–953. doi: 10.1109/JPROC.2015.2415800
Ang, K. K., Guan, C., Phua, K. S., Wang, C., Zhao, L., Teo, W. P., et al. (2015). Facilitating effects of transcranial direct current stimulation on motor imagery brain-computer interface with robotic feedback for stroke rehabilitation. Arch. Phys. Med. Rehabil. 96, S79–S87. doi: 10.1016/j.apmr.2014.08.008
Ang, K. K., Guan, C., Phua, K. S., Wang, C., Zhou, L., Tang, K. Y., et al. (2014b). Brain–computer interface–based robotic end effector system for wrist and hand rehabilitation: results of a three-armed randomized controlled trial for chronic stroke. Front. Neuroeng. 7:30. doi: 10.3389/fneng.2014.00030
Antje, K., Zorica, S., Jan, T., and Corina, S. A. (2020). Effect of brain–computer interface training based on non–invasive electroencephalography using motor imagery on functional recovery after stroke – a systematic review and meta-analysis. BMC Neurol. 20:385. doi: 10.1186/s12883-020-01960-5
Barsotti, M., Leonardis, D., Vanello, N., Bergamasco, M., and Frisoli, A. (2018). Effects of continuous kinaesthetic feedback based on tendon vibration on motor imagery BCI performance. IEEE Trans. Neural Syst. Rehabil. Eng. 26, 105–114. doi: 10.1109/tnsre.2017.2739244
Bartur, G., Pratt, H., Dickstein, R., Frenkel-Toledo, S., Geva, A., and Soroker, N. (2015). Electrophysiological manifestations of mirror visual feedback during manual movement. Brain Res. 1606, 113–124. doi: 10.1016/j.brainres.2015.02.029
Biasiucci, A., Leeb, R., Iturrate, I., Perdikis, S., Al-Khodairy, A., Corbet, T., et al. (2018). Brain–actuated functional electrical stimulation elicits lasting arm motor recovery after stroke. Nat. Commun. 9:2421. doi: 10.1038/s41467-018-04673-z
Braadbaart, L., Williams, J. H. G., and Waiter, G. D. (2013). Do mirror neuron areas mediate mu rhythm suppression during imitation and action observation? Int. J. Psychophysiol. 89, 99–105.
Bundy, D. T., Souders, L., Baranyai, K., Leonard, L., Schalk, G., Coker, R., et al. (2017). Contralesional brain–computer interface control of a powered exoskeleton for motor recovery in chronic stroke survivors. Stroke 48, 1908–1915.
Calautti, C., Leroy, F., Guincestre, J. Y., and Baron, J. C. (2001). Dynamics of motor network overactivation after striatocapsular stroke: a longitudinal PET study using a fixed-performance paradigm. Stroke 32:2534.
Cantillo-Negrete, J., Carino-Escobar, R. I., Carrillo-Mora, P., Rodriguez-Barragan, M. A., Hernandez-Arenas, C., Quinzaños-Fresnedo, J., et al. (2021). Brain–computer interface coupled to a robotic hand Orthosis for stroke patients’ neurorehabilitation: a crossover feasibility study. Front. Hum. Neurosci. 15:656975. doi: 10.3389/fnhum.2021.656975
Caria, A., Weber, C., Brötz, D., Ramos, A., Ticini, L. F., Gharabaghi, A., et al. (2011). Chronic stroke recovery after combined BCI training and physiotherapy: a case report. Psychophysiology 48, 578–582. doi: 10.1111/j.1469-8986.2010.01117.x
Cervera, M. A., Soekadar, S. R., Ushiba, J., Millan, J. D. R., Liu, M., Birbaumer, N., et al. (2018). Brain–computer interfaces for post–stroke motor rehabilitation: a meta-analysis. Ann. Clin. Transl. Neurol. 5, 651–663. doi: 10.1002/acn3.544
Chen, S., Cao, L., Shu, X., Wang, H., Ding, L., Wang, S.-H., et al. (2020). Longitudinal electroencephalography analysis in subacute stroke patients during intervention of brain-computer interface with exoskeleton feedback. Front. Neurosci. 14:809. doi: 10.3389/fnins.2020.00809
Choi, H., Lim, H., Kim, J. W., Kang, Y. J., and Ku, J. (2019). Brain computer interface-based action observation game enhances mu suppression in patients with stroke. Electronics 8:1466.
Der Lee, J. H. V., De Groot, V., Beckerman, H., Wagenaar, R. C., Lankhorst, G. J., and Bouter, L. M. (2001). The intra- and interrater reliability of the action research arm test: a practical test of upper extremity function in patients with stroke. Arch. Phys. Med. Rehabil. 82, 14–19.
Di Pino, G., Pellegrino, G., Assenza, G., Capone, F., Ferreri, F., Formica, D., et al. (2014). Modulation of brain plasticity in stroke: a novel model for neurorehabilitation. Nat. Rev. Neurol 10, 597–608. doi: 10.1038/nrneurol.2014.162
Dodakian, L., Campbell Stewart, J., and Cramer, S. C. (2014). Motor imagery during movement activates the brain more than movement alone after stroke: a pilot study. J. Rehabil. Med. 46, 843–848. doi: 10.2340/16501977-1844
Dodd, K. C., Nair, V. A., and Prabhakaran, V. (2017). Role of the Contralesional vs. Ipsilesional Hemisphere in stroke recovery. Front. Hum. Neurosci. 11:469. doi: 10.3389/fnhum.2017.00469
Farrell, J. W. III, Merkas, J., and Pilutti, L. A. (2020). The effect of exercise training on gait, balance, and physical fitness asymmetries in persons with chronic neurological conditions: a systematic review of randomized controlled trials. Front. Physiol. 11:585765. doi: 10.3389/fphys.2020.585765
Foong, R., Ang, K. K., Quek, C., Guan, C., Phua, K. S., Kuah, C. W. K., et al. (2020). Assessment of the efficacy of EEG-Based MI-BCI with visual feedback and EEG correlates of mental fatigue for upper–limb stroke rehabilitation. IEEE Trans. Biomed. Eng. 67, 786–795. doi: 10.1109/TBME.2019.2921198
Frenkel-Toledo, S., Bentin, S., Perry, A., Liebermann, D. G., and Soroker, N. (2014). Mirror–neuron system recruitment by action observation: effects of focal brain damage on mu suppression. Neuroimage 87, 127–137.
Frolov, A. A., Mokienko, O., Lyukmanov, R., Biryukova, E., Kotov, S., Turbina, L., et al. (2017). Post–stroke rehabilitation training with a motor–imagery–based brain–computer interface (BCI)–controlled hand exoskeleton: a randomized controlled multicenter trial. Front. Neurosci. 11:400. doi: 10.3389/fnins.2017.00400
Fuglmeyer, A. R., Jaasko, L., Leyman, I., Olsson, S., and Steglind, S. (1975). The post–stroke hemiplegic patient. 1. A method for evaluation of physical performance. Scand. J. Rehabil. Med. 7, 13–31.
Fujiwara, K., Shibata, M., Awano, Y., Shibayama, K., Iso, N., Matsuo, M., et al. (2021). A method for using video presentation to increase the vividness and activity of cortical regions during motor imagery tasks. Neural Regen. Res. 16, 2431–2437. doi: 10.4103/1673-5374.313058
Gastaut, H. (1952). Electrocorticographic study of the reactivity of rolandic rhythm. Rev. Neurologique 87, 176–182.
Gould, L., Kress, S., Neudorf, J., Gibb, K., Persad, A., Meguro, K., et al. (2021). An fMRI, DTI and neurophysiological examination of atypical organization of motor cortex in ipsilesional hemisphere following post–stroke recovery. J. Stroke Cerebrovasc. Dis. 30:105593. doi: 10.1016/j.jstrokecerebrovasdis.2020.105593
Jeunet, C., Glize, B., McGonigal, A., Batail, J.-M., and Micoulaud-Franchi, J.-A. (2019). Using EEG–based brain computer interface and neurofeedback targeting sensorimotor rhythms to improve motor skills: theoretical background, applications and prospects. Neurophysiol. Clin. 49, 125–136. doi: 10.1016/j.neucli.2018.10.068
Johnson, C. O., Minh, N., Roth, G. A., Nichols, E., Alam, T., Abate, D., et al. (2019). Global, regional, and national burden of stroke, 1990-2016: a systematic analysis for the global burden of disease study 2016. Lancet Neurol. 18, 439–458. doi: 10.1016/s1474-4422(19)30034-1
Kruse, A., Suica, Z., Taeymans, J., and Schuster-Amft, C. (2020). Effect of brain–computer interface training based on non-invasive electroencephalography using motor imagery on functional recovery after stroke – a systematic review and meta-analysis. BMC Neurol. 20:385.
Kwah, L. K., Harvey, L. A., Diong, J., and Herbert, R. D. (2013). Models containing age and NIHSS predict recovery of ambulation and upper limb function six months after stroke: an observational study. J. Physiother. 59, 189–197. doi: 10.1016/S1836-9553(13)70183-8
Lazarou, I., Nikolopoulos, S., Petrantonakis, P. C., Kompatsiaris, I., and Tsolaki, M. (2018). EEG-based brain–computer interfaces for communication and rehabilitation of people with motor impairment: a novel approach of the 21st century. Front. Hum. Neurosci. 12:14. doi: 10.3389/fnhum.2018.00014
Lee, B. J. B., Williams, A., and Ben-Tzvi, P. (2018). Intelligent object grasping with sensor fusion for rehabilitation and assistive applications. IEEE Trans. Neural. Syst. Rehabil. Eng. 26, 1556–1565. doi: 10.1109/TNSRE.2018.2848549
Lin, K.-c, Hsieh, Y.-w, Wu, C.-y, Chen, C.-l, Jang, Y., and Liu, J.-s (2009). Minimal detectable change and clinically important difference of the wolf motor function test in stroke patients. Neurorehabil. Neural Repair 23, 429–434. doi: 10.1177/1545968308331144
Luo, L., Meng, H., Wang, Z., Zhu, S., Yuan, S., Wang, Y., et al. (2020). Effect of high-intensity exercise on cardiorespiratory fitness in stroke survivors: a systematic review and meta-analysis. Ann. Phys. Rehabil. Med. 63, 59–68. doi: 10.1016/j.rehab.2019.07.006
McCreadie, K. A., Coyle, D. H., and Prasad, G. (2013). Sensorimotor learning with stereo auditory feedback for a brain–computer interface. Med. Biol. Eng. Comput. 51, 285–293. doi: 10.1007/s11517-012-0992-7
McCreadie, K. A., Coyle, D. H., and Prasad, G. (2014). Is sensorimotor BCI performance influenced differently by mono, stereo, or 3–D auditory feedback? IEEE Trans. Neural. Syst. Rehabil. Eng. 22, 431–440. doi: 10.1109/tnsre.2014.2312270
McCrimmon, C. M., King, C. E., Wang, P. T., Cramer, S. C., Nenadic, Z., and Do, A. H. (2014). Brain-controlled functional electrical stimulation for lower–limb motor recovery in stroke survivors. Annu. Int. Conf. IEEE Eng. Med. Biol. Soc. 2014, 1247–1250. doi: 10.1109/embc.2014.6943823
Morris, J. H., van Wijck, F., Joice, S., and Donaghy, M. (2013). Predicting health related quality of life 6 months after stroke: the role of anxiety and upper limb dysfunction. Disabil. Rehabil. 35, 291–299. doi: 10.3109/09638288.2012.691942
Muthukumaraswamy, S. D., Johnson, B. W., and Mcnair, N. A. (2004). Mu rhythm modulation during observation of an object-directed grasp. Cogn. Brain Res. 19, 195–201.
Nagai, H., and Tanaka, T. (2019). Action observation of own hand movement enhances event–related desynchronization. IEEE Trans. Neural Syst. Rehabil. Eng. 27, 1407–1415. doi: 10.1109/tnsre.2019.2919194
Nijboer, F., Furdea, A., Gunst, I., Mellinger, J., McFarland, D. J., Birbaumer, N., et al. (2008). An auditory brain–computer interface (BCI). J. Neurosci. Methods 167, 43–50. doi: 10.1016/j.jneumeth.2007.02.009
Nojima, I., Sugata, H., Takeuchi, H., and Mima, T. (2021). Brain–computer interface training based on brain activity can induce motor recovery in patients with stroke: a meta–analysis. Neurorehabil. Neural Repair 36, 83–96. doi: 10.1177/15459683211062895
Norman, S. L., McFarland, D. J., Miner, A., Cramer, S. C., Wolbrecht, E. T., Wolpaw, J. R., et al. (2018). Controlling pre-movement sensorimotor rhythm can improve finger extension after stroke. J. Neural Eng. 15:14. doi: 10.1088/1741-2552/aad724
Oberman, L. M., Ramachandran, V. S., and Pineda, J. A. (2008). Modulation of mu suppression in children with autism spectrum disorders in response to familiar or unfamiliar stimuli: the mirror neuron hypothesis. Neuropsychologia 46, 1558–1565. doi: 10.1016/j.neuropsychologia.2008.01.010
Ono, T., Shindo, K., Kawashima, K., Ota, N., Ito, M., Ota, T., et al. (2014). Brain–computer interface with somatosensory feedback improves functional recovery from severe hemiplegia due to chronic stroke. Front. Neuroeng. 7:19. doi: 10.3389/fneng.2014.00019
Page, S. J., Fulk, G. D., and Boyne, P. (2012). Clinically important differences for the upper-extremity Fugl–Meyer scale in people with minimal to moderate impairment due to chronic stroke. Phys. Ther. 92, 791–798. doi: 10.2522/ptj.20110009
Pfurtscheller, G., Brunner, C., Schlögl, A., and Silva, F. (2006a). Mu rhythm (de)synchronization and EEG single–trial classification of different motor imagery tasks. Neuroimage 31, 153–159.
Pfurtscheller, G., Brunner, C., Schlogl, A., and da Silva, F. H. L. (2006b). Mu rhythm (de)synchronization and EEG single-trial classification of different motor imagery tasks. Neuroimage 31, 153–159. doi: 10.1016/j.neuroimage.2005.12.003
Pfurtscheller, G., and Lopes da Silva, F. H. (1999). Event–related EEG/MEG synchronization and desynchronization: basic principles. Clin. Neurophysiol. 110, 1842–1857. doi: 10.1016/s1388-2457(99)00141-8
Pfurtscheller, G., Scherer, R., Müller-Putz, G., and Silva, F. (2010). Short-lived brain state after cued motor imagery in naive subjects. Eur. J. Neurosci. 28, 1419–1426.
Pfurtscheller, G., Stancak, A. J., and Neuper, C. (1996). Event–related synchronization (ERS) in the alpha band – an electrophysiological correlate of cortical idling: a review. Int. J. Psychophysiol. 24, 39–46.
Pichiorri, F., Morone, G., Petti, M., Toppi, J., Pisotta, I., Molinari, M., et al. (2015). Brain–computer interface boosts motor imagery practice during stroke recovery. Ann. Neurol. 77, 851–865. doi: 10.1002/ana.24390
Pineda, J. A. (2006). The functional significance of mu rhythms: translating “seeing” and “hearing” into “doing”. Brain Res. Rev. 50, 57–68.
Prasad, G., Herman, P., Coyle, D., McDonough, S., and Crosbie, J. (2010). Applying a brain–computer interface to support motor imagery practice in people with stroke for upper limb recovery: a feasibility study. J. Neuroeng. Rehabil. 7:60. doi: 10.1186/1743-0003-7-60
Ramos-Murguialday, A., Broetz, D., Rea, M., Läer, L., Yilmaz, Ö, Brasil, F. L., et al. (2013). Brain–machine interface in chronic stroke rehabilitation: a controlled study. Ann. Neurol. 74, 100–108. doi: 10.1002/ana.23879
Ren, S., Wang, W., Hou, Z. G., Liang, X., Wang, J., and Shi, W. (2020). Enhanced motor imagery based brain– computer interface via FES and VR for lower limbs. IEEE Trans. Neural Syst. Rehabil. Eng. 28, 1846–1855. doi: 10.1109/tnsre.2020.3001990
Sebastián-Romagosa, M., Cho, W., Ortner, R., Murovec, N., Von Oertzen, T., Kamada, K., et al. (2020). Brain computer interface treatment for motor rehabilitation of upper extremity of stroke patients—a feasibility study. Front. Neurosci. 14:591435. doi: 10.3389/fnins.2020.591435
Shu, X., Chen, S., Meng, J., Yao, L., Sheng, X., Jia, J., et al. (2018). Tactile stimulation improves sensorimotor rhythm-based bci performance in stroke patients. IEEE Trans. Biomed. Eng. 66, 1987–1995. doi: 10.1109/TBME.2018.2882075
Shu, X., Yao, L., Sheng, X., Zhang, D., and Zhu, X. (2017). Enhanced motor imagery–based BCI performance via tactile stimulation on unilateral hand. Front. Hum. Neurosci. 11:585. doi: 10.3389/fnhum.2017.00585
Song, M., and Kim, J. (2019). A paradigm to enhance motor imagery using rubber hand illusion induced by visuo–tactile stimulus. IEEE Trans. Neural Syst. Rehabil. Eng. 27, 477–486. doi: 10.1109/tnsre.2019.2895029
Sun, R., Wong, W.-W., Wang, J., and Tong, R. K.-Y. (2017). Changes in electroencephalography complexity using a brain computer interface-motor observation training in chronic stroke patients: a fuzzy approximate entropy analysis. Front. Hum. Neurosci. 11:444. doi: 10.3389/fnhum.2017.00444
Susanto, E. A., Tong, R. K., Ockenfeld, C., and Ho, N. S. (2015). Efficacy of robot-assisted fingers training in chronic stroke survivors: a pilot randomized-controlled trial. J. NeuroEng. Rehabil. 12:42.
Velasco-Álvarez, F., Ron-Angevin, R., da Silva-Sauer, L., and Sancha-Ros, S. (2013). Audio–cued motor imagery–based brain–computer interface: navigation through virtual and real environments. Neurocomputing 121, 89–98. doi: 10.1016/j.neucom.2012.11.038
Vukelić, M., and Gharabaghi, A. (2015). Oscillatory entrainment of the motor cortical network during motor imagery is modulated by the feedback modality. NeuroImage 111, 1–11. doi: 10.1016/j.neuroimage.2015.01.058
Wolf, S. L., Catlin, P. A., Ellis, M., Archer, A. L., Morgan, B., and Piacentino, A. (2001). Assessing wolf motor function test as outcome measure for research in patients after stroke. Stroke 32, 1635–1639. doi: 10.1161/01.Str.32.7.1635
Wu, Q., Yue, Z., Ge, Y., Ma, D., Yin, H., Zhao, H., et al. (2020). Brain functional networks study of subacute stroke patients with upper limb dysfunction after comprehensive rehabilitation including BCI training. Front. Neurol. 10:1419. doi: 10.3389/fneur.2019.01419
Yuan, H., and He, B. (2014). Brain-computer interfaces using sensorimotor rhythms: current state and future perspectives. IEEE Trans. Biomed. Eng. 61, 1425–1435. doi: 10.1109/tbme.2014.2312397
Zhou, M. G., Wang, H. D., Zeng, X. Y., Yin, P., Zhu, J., Chen, W. Q., et al. (2019). Mortality, morbidity, and risk factors in China and its provinces, 1990–2017: a systematic analysis for the global burden of disease study 2017. Lancet 394, 1145–1158. doi: 10.1016/s0140-6736(19)30427-1
Keywords: stroke, motor imagery, brain computer interface, mu rhythm, rehabilitation
Citation: Li X, Wang L, Miao S, Yue Z, Tang Z, Su L, Zheng Y, Wu X, Wang S, Wang J and Dou Z (2022) Sensorimotor Rhythm-Brain Computer Interface With Audio-Cue, Motor Observation and Multisensory Feedback for Upper-Limb Stroke Rehabilitation: A Controlled Study. Front. Neurosci. 16:808830. doi: 10.3389/fnins.2022.808830
Received: 04 November 2021; Accepted: 27 January 2022;
Published: 11 March 2022.
Edited by:
Surjo R. Soekadar, Charité – Universitätsmedizin Berlin, GermanyReviewed by:
Pratik Yashvant Chhatbar, Duke University, United StatesPavel Bobrov, Institute of Higher Nervous Activity and Neurophysiology (RAS), Russia
Copyright © 2022 Li, Wang, Miao, Yue, Tang, Su, Zheng, Wu, Wang, Wang and Dou. This is an open-access article distributed under the terms of the Creative Commons Attribution License (CC BY). The use, distribution or reproduction in other forums is permitted, provided the original author(s) and the copyright owner(s) are credited and that the original publication in this journal is cited, in accordance with accepted academic practice. No use, distribution or reproduction is permitted which does not comply with these terms.
*Correspondence: Shan Wang, ab0782@sina.com; Jing Wang, wangpele@gmail.com; Zulin Dou, douzul@163.com
†These authors have contributed equally to this work