- 1Department of Developmental Neuroscience, IRCCS Stella Maris Foundation, Pisa, Italy
- 2Department of Neuroscience, Psychology, Drug Research and Child Health NEUROFARBA, University of Florence, Florence, Italy
- 3Department of Clinical and Experimental Medicine, University of Pisa, Pisa, Italy
- 4Institute of Neuroscience, National Research Council, Pisa, Italy
Accumulating evidence suggests that functional Near-Infrared Spectroscopy (fNIRS) can provide an essential bridge between our current understanding of neural circuit organization and cortical activity in the developing brain. Indeed, fNIRS allows studying brain functions through the measurement of neurovascular coupling that links neural activity to subsequent changes in cerebral blood flow and hemoglobin oxygenation levels. While the literature offers a multitude of fNIRS applications to typical development, only recently this tool has been extended to the study of neurodevelopmental disorders (NDDs). The exponential rise of scientific publications on this topic during the last years reflects the interest to identify a “fNIRS signature” as a biomarker of high translational value to support both early clinical diagnosis and treatment outcome. The purpose of this systematic review is to describe the updating clinical applications of fNIRS in NDDs, with a specific focus on preschool population. Starting from this rationale, a systematic search was conducted for relevant studies in different scientific databases (Pubmed, Scopus, and Web of Science) resulting in 13 published articles. In these studies, fNIRS was applied in individuals with Autism Spectrum Disorder (ASD) or infants at high risk of developing ASD. Both functional connectivity in resting-state conditions and task-evoked brain activation using multiple experimental paradigms were used in the selected investigations, suggesting that fNIRS might be considered a promising method for identifying early quantitative biomarkers in the autism field.
Introduction
Functional Near-Infrared Spectroscopy (fNIRS) allows performing in vivo a continuous and non-invasive monitoring of oxygenation levels of chromophores like hemoglobin (Van de Rijt et al., 2018), thanks to the transparency of biological tissues -including the human brain- to the light in the near-infrared spectrum. Indeed, fNIRS quantifies regional changes in the concentration of oxygenated, deoxygenated, and total hemoglobin (OxyHb, DeoxyHb, and TotHb). The blood-oxygen-level-dependent (BOLD) signal detected by fNIRS provides a direct measure of modifications in the brain blood flow, which are coupled to neuronal activity by the complex process known as “neurovascular coupling” (Villringer and Chance, 1997; Logothetis and Wandell, 2004). Typically, an increase of neural activity is paralleled by a peak of OxyHb and TotHb, with a drop of DeoxyHb. fNIRS devices consist of red light-sources paired to specific detectors, which can be placed into a textile EEG-like cap to form an array of multi-distant channels. For data acquisition, the system executes the parallel reading of optical sensors. fNIRS systems give the opportunity to perform recording with different wavelengths of the near-infrared spectrum (700–1000 nm). However, for functional activation studies in humans the devices typically focus on paired wavelengths of approximately 690 and 830 nm to optimize the accuracy of OxyHb and DeoxyHb measurements (Lloyd-Fox et al., 2010). Indeed, separability between the chromophore signals seems to be optimal if one wavelength is below 720 nm and the other is above 730 nm (Uludag et al., 2004).
Although several neuroimaging methods can directly detect the electrical activity of brain circuits (EEG and MEG) or record the related hemodynamic response (fMRI), most of them have limiting factors restricting their use in the developmental time window (for instance, sensitivity to motion artifacts, requiring high grade of subject compliance). Indeed, fMRI is the gold standard for in vivo imaging of the human brain, but fNIRS stands out for its high portability, robustness to noise, relatively low costs and small size, bringing functional imaging into much more realistic environments (Cui et al., 2011; Duan et al., 2012; Scarapicchia et al., 2017). In particular, strength to motion artifacts make this technique an ideal candidate for research in preschool children (until the age of 6), even in infancy and toddlerhood (Lloyd-Fox et al., 2010). Despite lower spatial resolution and imaging depth, fNIRS offers a higher temporal resolution than fMRI. On the other hand, EEG and fNIRS share high portability, low-cost features and recording depth, but they measure different perspectives of brain activity: indeed, EEG signal reflects the bioelectrical activation of cortical neurons, while fNIRS assesses hemodynamic responses (Chiarelli et al., 2017; Berger et al., 2019). In order to obtain a more detailed picture of brain activity, fNIRS can be combined with other neuroimaging and electrophysiological methods (e.g., fMRI and EEG), without causing any measurement interference (Chen et al., 2015) and potentially gaining a multimodal data set (Yuan et al., 2010; Chiarelli et al., 2017). Notably, EEG and fNIRS use similar experimental settings (they are both scalp-located procedures), but show different temporal resolutions that might allow dissecting the electrical from the hemodynamic contribution to the recorded signal.
Although introduced into clinical care almost 40 years ago, only recently, fNIRS gained much popularity in the study of brain development and neurodevelopmental disorders (NDDs) (Greisen, 2006; Vanderwert and Nelson, 2014). FNIRS has been widely used to investigate the typical maturation of speech perception and language development, sensory and motor functions, social communication and interaction, object processing, human action processing in both toddlers and children (Lloyd-Fox et al., 2010). In contrast, the application of fNIRS in the field of NDDs is a growing research area and studies looking for a “fNIRS signature” as a brain biomarker useful for the diagnosis and the treatment outcome are still underrepresented (Gervain et al., 2011). NDDs are a heterogeneous group of complex disorders resulting from the interaction of genetic, epigenetic, neurobiological and environmental factors (from alterations in utero to postnatal environment), and characterized by early disruption in cognition, emotion, and behavior. NDDs include attention-deficit/hyperactivity disorder (ADHD), autism spectrum disorder (ASD), communication and specific learning disorders, intellectual disability (ID) and motor disorders (American Psychiatric Association, 2013). NDDs often present overlapping clinical features or may occur simultaneously in the same patient, making the differential diagnosis potentially challenging (Hansen et al., 2018). Moreover, NDDs show fairly broad behavioral phenotypes, reflecting also etiological heterogeneity of these disorders that can occur as “idiopathic” or “secondary” conditions (e.g., well documented causes of secondary ASD include monogenic disorders such as tuberous sclerosis and Fragile-X syndrome) (Muhle et al., 2004). Thus, reliable functional biomarkers would be very helpful to support early clinical diagnosis and to monitor developmental trajectories through in vivo longitudinal studies, also focused on the potential outcome of tailored intervention. Moreover functional biomarkers might help clinicians to separate behavioral symptoms of NDDs into more stable and objective phenotypes, including gender-related endophenotypes.
Clinically useful neuroimaging markers for NDDs are sorely missing yet (Vasung et al., 2019; Azhari et al., 2020), but emerging evidence suggests that fNIRS might be exploited to generate unbiased and reliable measures to explore brain functions, thus possibly driving clinicians toward a faster and more precise diagnosis (Zhang and Roeyers, 2019).
Moreover, fNIRS offers the opportunity to study cortical networks focusing not only on neuronal activation, but also on the neuromodulatory action of non-neuronal cells (such as glial cells or astrocytes that contribute to neurovascular and metabolic homeostasis of the brain). Thus, fNIRS is emerging as a fundamental tool, alone or in combination with other techniques, to dissect the key players in brain physiology and pathophysiology (Lines et al., 2020).
The main purpose of this systematic review has been to investigate the recent literature covering the use of fNIRS in the field of NDDs. We decided to focus on the preschool population in order to highlight the promising value of fNIRS as a reliable and non-invasive tool to detect brain biomarkers in clinical settings, even in populations commonly showing reduced compliance to experimental environments. This specific inclusion criterium led to narrow the focus of this review to children with idiopathic ASD or high-risk (HR) infants.
Materials and Methods
A systematic search was conducted for relevant studies in three databases (PubMed, Scopus, and Web of Science), using the following search terms that were refined from previous reviews (Liu et al., 2019; Zhang and Roeyers, 2019) and according to PICOS framework: (“fNIRS” OR “functional nirs” OR “near-infrared spectroscopy”) AND (“neurodevelopmental disorder” OR “ASD” OR “ADHD”) AND (“children” OR “toddler” OR “preschooler”).
Additionally, manual searches were conducted among the reference sections of the retrieved studies and reviews. Publication year was not restricted, and the latest database search was performed in March 2021. Reviews were not included in the selection, but were only used to collect original studies. After removing duplicate records, articles emerged from the search were required to meet pre-established criteria. Inclusion and exclusion criteria, established prior to the literature search, are outlined below.
Inclusion Criteria
(i) Studies applying fNIRS;
(ii) Studies including a clinical population with a confirmed diagnosis (according to DSM-5) or a well-established condition of risk for NDDs;
(iii) Studies including preschool children (sample mean age < 7 years).
Exclusion Criteria
(i) Articles published in languages other than English;
(ii) Meta-analyses or literature reviews;
(iii) Other reasons referring to report characteristics, as not published clinical studies (e.g., conference paper, thesis, book chapter) or studies that do not involve human participants (e.g., focused on methodological issues).
Results
Study Selection
One hundred and sixty-three potentially relevant studies were identified from PubMed, 136 from Scopus, 127 from Web of Science; in addition, four articles that met the selection criteria were retrieved from screened review articles. After omitting duplicates, 224 articles were examined. On the basis of the title, a total of 52 studies that did not meet the inclusion criteria were disregarded. Finally, within the172 articles screened for abstract or full-text evaluation, a total of 13 studies were selected for this review. An in-depth detail of paper selection is shown in the PRISMA diagram (Figure 1).
Crucial information was extrapolated from included papers. Tables 1, 2 summarize the mean age of participants, the sample size and gender ratio, the experimental procedures (technical aspects of recording sessions and experimental design) used during fNIRS measurement.
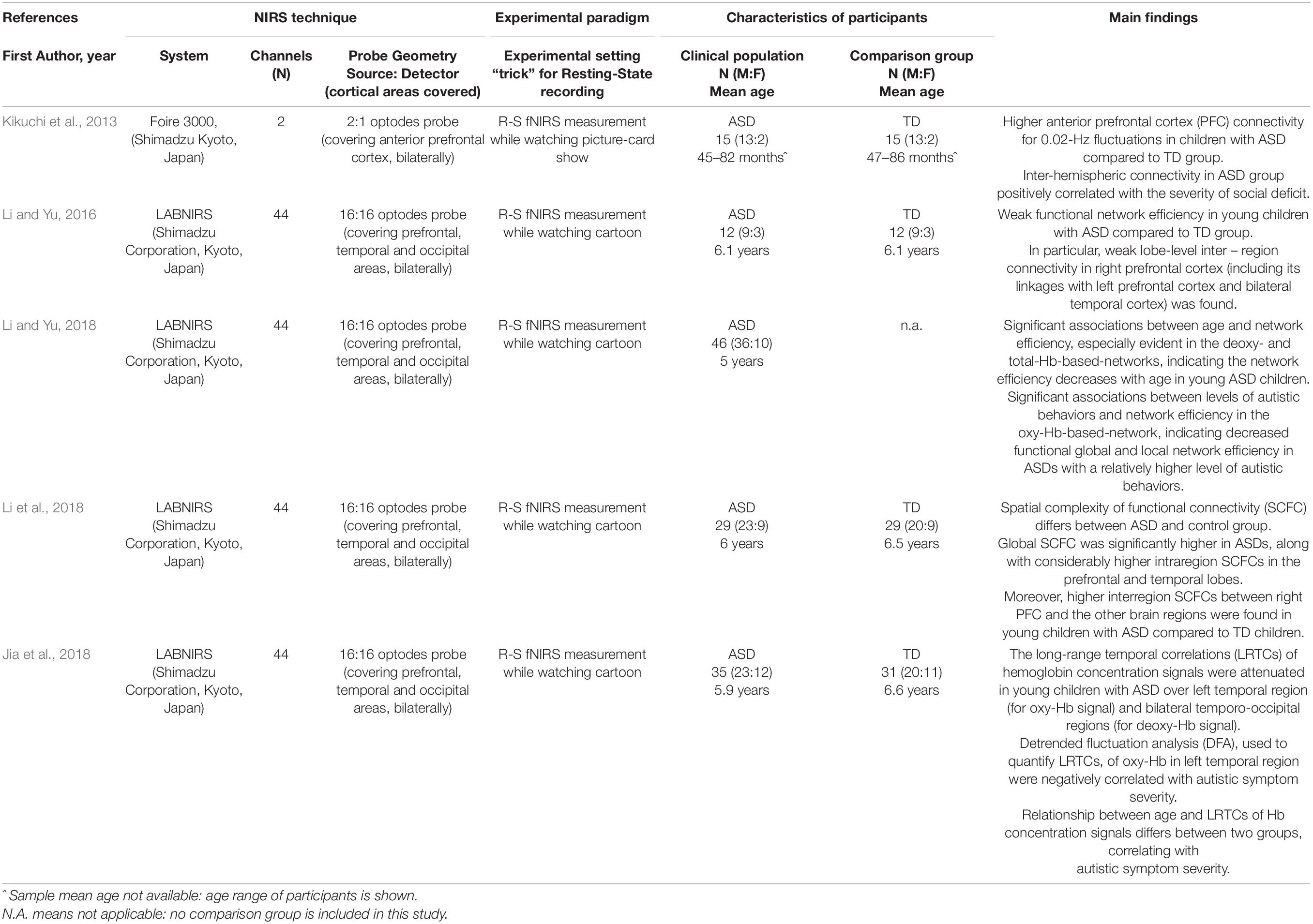
Table 1. Overview of the reviewed studies using Resting State (R-S) fNIRS recordings: references, technical issues, characteristics of participants as well as main findings are shown.
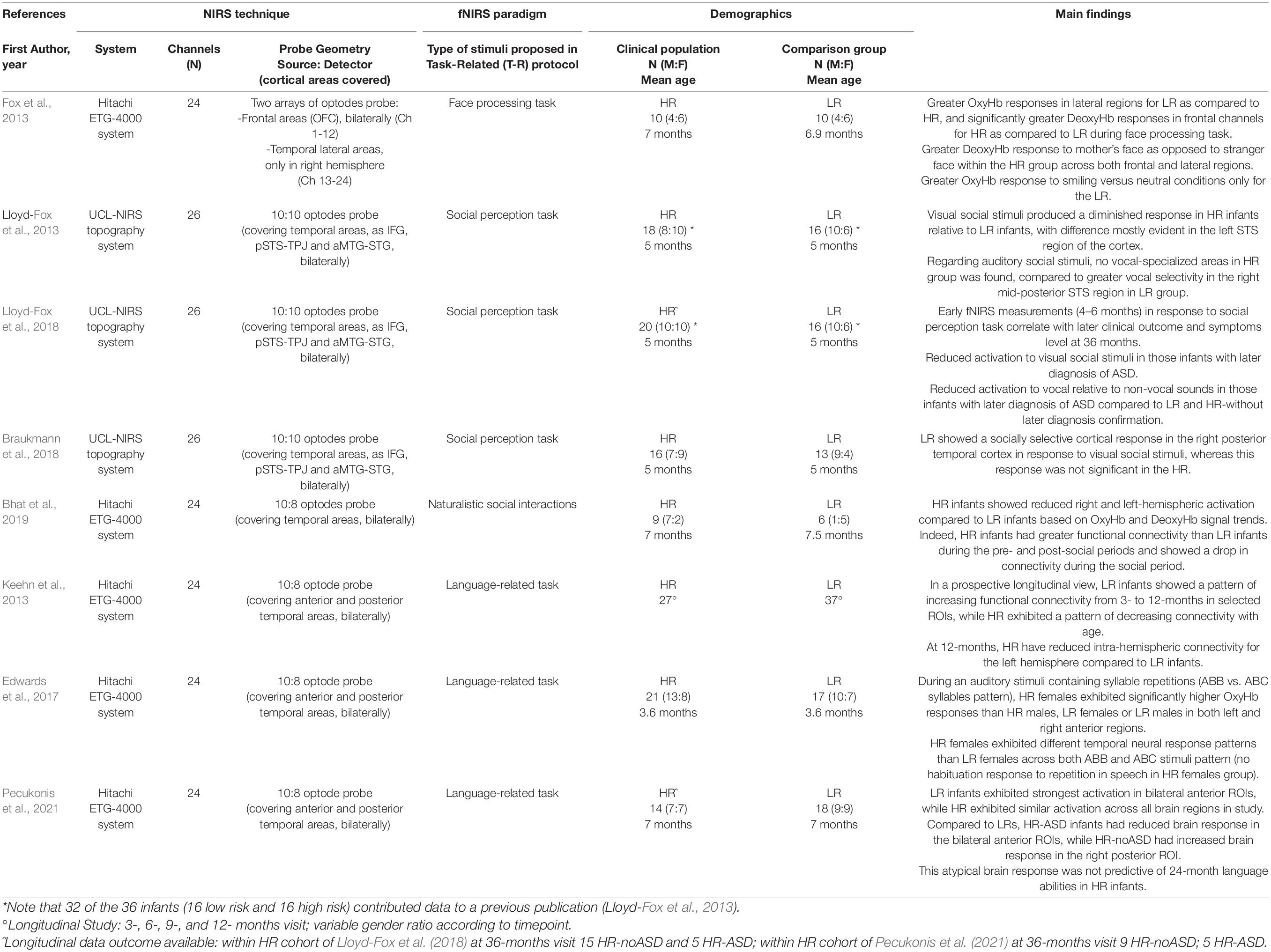
Table 2. Overview of the reviewed studies using Task-Related (T-R) fNIRS recordings: references, technical issues, characteristics of participants as well as main findings are shown.
Although the initial aim was to include different type of NDDs (see keywords used for the search), only studies focused on children with idiopathic ASD or high-risk (HR) infants (infants at high familial risk of autism) satisfied the inclusion and exclusion criteria.
Regarding other NDDs, studies on ADHD included children older than 7 years, due to the pharmacological aim of their experimental design: at the time of our last search, indeed, 55 studies focused on fNIRS and ADHD, but none of them was performed on the preschool population. Moreover, within the growing bulk of literature that uses fNIRS for evaluating the emerging language ability in infants, only two studies (Chang, 2014; Hosseini et al., 2018) focused on children with Language Disorders; however, both of them, did not meet the inclusion criteria (mean age of participants older than 7 years) and were therefore excluded from this review. fNIRS was rarely used to explore neuropsychological features in learning disorders such as Dyslexia (Zhu et al., 2012; Pecyna and Pokorski, 2013; Song et al., 2013; Sela et al., 2014; Cutini et al., 2016), but this field obviously includes only school-aged children. Application of fNIRS to Intellectual Disability (ID) is only anecdotal yet (only one scientific report found in literature database consulted) (Bembich et al., 2021).
Characteristics of Participants
As mentioned above, all studies included in this review applied fNIRS in preschool children with ASD, a clinical condition characterized by socio-communicative impairment associated with restricted interests and repetitive behaviors, or HR infants (younger siblings of children with ASD). Only four studies included more than 40 participants (Keehn et al., 2013; Jia et al., 2018; Li and Yu, 2018; Li et al., 2018); in most cases subjects were equally distributed between the clinical population and the control group. Interestingly, the cohorts included both males and females in all studies, but the two populations were not homogeneously distributed in terms of sample size, except for two investigations (Lloyd-Fox et al., 2018; Pecukonis et al., 2021).
Five studies included in this review (Kikuchi et al., 2013; Li and Yu, 2016, 2018; Jia et al., 2018; Li et al., 2018) comprised ASD children with a mean age was between 3 and 7 years and compared the ASD group with age- and gender-matched typically developing (TD) peers in resting state condition (see Table 1). It is to note that all selected studies included children with an average IQ score and no comorbidity with ADHD.
The remaining eight studies (Fox et al., 2013; Keehn et al., 2013; Lloyd-Fox et al., 2013, 2018; Edwards et al., 2017; Braukmann et al., 2018; Bhat et al., 2019; Pecukonis et al., 2021) focused on infants/toddlers (children younger than 3 years old), and compared fNIRS data between HR group and in Low-Risk groups (LR; condition defined as subjects with no family history of ASD) in task-related study designs (see Table 2). Of these studies, only two (Lloyd-Fox et al., 2018; Pecukonis et al., 2021) analyzed the correlation between the fNIRS brain responses of infants during the first year of life and their diagnostic outcome at 24 or 36 months.
fNIRS Measurement: Technical Issues and Experimental Design
A total of four different fNIRS recording systems were employed in the reviewed studies (Foire 3000, LABNIRS, Hitachi ETG-4000 system, UCL-NIRS topography system). The number of channels used ranged from a minimum of 2 (Kikuchi et al., 2013) to a maximum of 44 (Li and Yu, 2016, 2018; Jia et al., 2018; Li et al., 2018). The remaining investigations used an intermediate number of channels (24–26 pairs of optodes), but the density of montages may be considered comparable among almost all studies, due to the younger age of the cohort investigated and the relatively smaller dimension of the probe. Despite the variability of probe geometry, in all cases optodes placement followed the international 10–20 EEG system.
Within the 13 articles included, eight studies explored the brain activation evoked by specific tasks (task-related paradigm or T-R; an overview of experimental procedures is reported in Table 2) and the remaining five investigated functional brain organization in resting-state (R-S) condition (an overview of experimental procedures is reported in Table 1). Considering the significant difficulties to remain still under stimulation-free conditions for young children, all resting-state data were recorded while participants watched a cartoon or, only in one case, a picture-card show (Kikuchi et al., 2013) in order to optimize subject compliance. Papers exploring functional cortical activation in task-related condition can be sub-grouped into two categories that reflect the nature of the task proposed: (i) social stimuli, including social perception (Lloyd-Fox et al., 2013, 2018; Braukmann et al., 2018), face processing (Fox et al., 2013) and naturalistic social interactions (Bhat et al., 2019); (ii) language-related stimuli (Keehn et al., 2013; Edwards et al., 2017; Pecukonis et al., 2021).
Discussion
Papers systematically selected for this review allow discussing some research questions that might pave the way toward the fNIRS application in the field of NDDs. The final selection, mainly based on age criteria, focused our attention on ASD and HR populations. This field is characterized by huge heterogeneity both regarding the phenotypic and the neurobiological level, and a great amount of literature is devoted to uncover biomarkers dissecting sub-phenotypes and supporting the clinical assessment (Loth et al., 2016; Lombardo et al., 2019). Indeed, while an early detection of ASD is highly recommended to receive the services and supports the children need to reach their full potential (Dawson et al., 2010; Hyman et al., 2020), a final diagnosis is still obtained approximately during the third year of life (Salomone et al., 2016). However, neuroimaging and electrophysiological evidence consistently suggested that ASD-related atypical brain pattern in cortical regions crucial for socio-communicative skills (such as fronto-temporal areas) could be detected before the full-blown expression of symptoms (Conti et al., 2015; Ha et al., 2015; O’Reilly et al., 2017; Pagnozzi et al., 2018).
Is “fNIRS Signature” Useful as a Brain Biomarker for Autism Spectrum Disorder?
Resting-state fNIRS measurements have been proposed as auxiliary indexes for the objective assessment of ASD in both young (Xu et al., 2021) and adult population (Yanagisawa et al., 2016).
Regarding preschoolers, Kikuchi et al. (2013) first demonstrated an aberrant functional connectivity between the right and the left anterior prefrontal cortex (aPFC) in young children with ASD under resting state conditions. Notably, they found a significantly higher inter-hemispheric connectivity in the ASD compared to the TD group, reinforcing previous data about the aberrant cortical organization at structural level in ASD children (McCaffery and Deutsch, 2005; Courchesne et al., 2011). The higher inter-hemispheric connectivity in ASD reported by Kikuchi et al. (2013) was positively correlated with the severity of social deficit, as scored with the Autism Diagnostic Observation Schedule (Lord et al., 2012). It is to note that the anterior prefrontal cortex has already been implicated in ASD pathophysiology in previous structural and functional studies, as being involved in social perception and processing abilities that are altered in the ASD condition (Uddin et al., 2013; Pagnozzi et al., 2018).
In contrast, studies from Li and Yu (2016, 2018) and Li et al. (2018) showed weaker functional network efficiency in young children with ASD compared to TD peers, mainly in short- and long-range connectivity of the right prefrontal cortex (Li and Yu, 2016). Moreover, a subsequent study of the same research group (Li et al., 2018) showed an inverse association between network efficiency, age (especially evident in the Deoxy- and TotHb-based networks) and severity of autistic behaviors (especially evident in the OxyHb-based-network) in high-functioning ASD subjects. Statistically significant findings were also obtained when comparing ASD and TD groups through spatial complexity of functional connectivity (SCFC) analysis (Li et al., 2018).
Other approaches to analyze resting state recordings also confirmed that fNIRS might be a candidate tool to investigate the pathophysiological mechanism of ASD, looking at the temporal dynamics of neuronal oscillations (Jia et al., 2018). Specifically, the long-range temporal correlations (LRTCs) of hemoglobin concentration signals were studied by quantifying a detrended fluctuation analysis (DFA) exponent. Comparing data between ASD and TD group, significant differences were found over the left temporal region for OxyHb signal, and over bilateral temporo-occipital regions for DeoxyHb signals, suggesting a shift-to-randomness of brain oscillations in children with ASD. Moreover, the relationship between age and DFA exponents revealed that this association could be modulated by autism. Furthermore, the DFA exponents of OxyHb in the left temporal region were negatively correlated with autistic symptom severity. Between-group differences remained significant also looking at correlation coefficients between age and DFA.
Altogether, these results confirm that fNIRS can detect a different pattern of functional connectivity in the brain of ASD children. Accordingly, the considerable bulk of prospective studies focused on neurostructural and neurofunctional measures of brain development validated the concept of ASD as characterized by early altered connectivity patterns (Bosl et al., 2011; Wolff et al., 2012; Solso et al., 2016). In particular, early social brain network alterations were previously reported in structural (Shen et al., 2018) and functional MRI (Emerson et al., 2017) as well in observational studies (Elsabbagh and Johnson, 2016). However, no resting-state fNIRS data are yet available in cohorts younger than 3 years, thus restricting the predictive value of resting state analyses so far. Moreover, the mathematical algorithms used for the interpretation of resting-state data are very complex to be applied in the clinical setting and the limited coherence among models still prevents from drawing conclusive interpretations.
Is “fNIRS Signature” Useful as an Early Predictor in “At Risk” Population?
Younger siblings of children with ASD are considered as HR infants because around 20% of them receive a diagnosis of ASD at the age of 3 years (Ozonoff et al., 2011) and a further 20–30% exhibit other neurodevelopmental problems (Messinger et al., 2013). In addition, a high rate of behavioral traits overlapping with those observed in children with an ASD diagnosis (referred as the Broader Autism Phenotype) has been documented in the sibling population (Charman et al., 2017). Thus, the prospective study of the HR population allows detecting behavioral risk signs or biomarkers of NDDs at a very early age, prior to the full clinical manifestation (Zwaigenbaum et al., 2015).
Studies on younger siblings of children with ASD compared HR infants with infants without familial history of ASD or NDDs (LR infants), regardless their developmental outcome or differentiating HR infants with or without a later diagnosis of ASD. In this review, we included both types of studies as we are interested in understanding how fNIRS signal can be used as a potential biomarker of atypical development, and not only as an ASD related outcome.
In the past decade, the number of studies exploring the applicability of fNIRS technique in HR infants has rapidly grown, focusing especially on core ASD neurocognitive domains. Accordingly, our study selection includes five papers testing social perception and three others assessing possible alterations of language development in the HR group.
With regard to social processing, starting from previous data in typically developing infants (Lloyd-Fox et al., 2012), Lloyd-Fox et al. (2013) examined whether the temporal lobe specialization for processing visual and auditory social stimuli during the first months of life differed between HR and LR infants. They found significantly diminished evoked hemodynamic responses in HR compared to LR infants in the superior temporal sulcus (STS), both in visual and auditory trials, suggesting a lack of cortical specialization to social stimuli, already within the first 6 months of life. Notably, a more recent prospective study in the same cohort of HR infants (increased by only 2 more subjects) demonstrated that the reduced activation to visual social and vocal stimuli across cortical regions of interest was specific for infants with a later diagnosis of ASD (Lloyd-Fox et al., 2018).
Moreover, Braukmann et al. (2018) provided further evidence for a social processing difference in infants at risk of autism, highlighting a reduced hemodynamic response evoked by social visual stimuli in the right posterior temporal cortex. Similarly, a reduced bilateral cortical activation in HR children compared to LR infants was reported in the social perception within the infant-mother dyad (Bhat et al., 2019). Of note, this is the only study using a fNIRS experimental protocol with live stimuli (face-to-face naturalistic social interaction between infant and mother).
Social behavior was also explored by Fox et al. (2013), recording fNIRS hemodynamic responses to a face perception task (facial identity and emotion perception on video clips performed by infant’s mothers) during the first year of life. This study suggested differences in patterns of functional connectivity in the frontal lobe (short- and long-range connections) between the HR and LR groups. HR infants showed significantly greater DeoxyHb responses in frontal channels and lower OxyHb responses in right temporal regions during face processing task; a significant difference between groups was detectable in response to mother’s face as to a stranger face (greater DeoxyHb response within the HR group across both frontal and lateral regions). For this cohort, no longitudinal data were available for discriminating infants with or without later ASD diagnosis and establishing the predictive value of early fNIRS recordings.
To summarize, these results suggest that socially evoked fNIRS signals might be a suitable and predictive biomarker in early assessment of HR infants; however, further studies are needed to confirm these promising findings.
Growing evidence on the validity of fNIRS for studying early aberrant functional brain networks in HR infants emerged from the language domain as well.
Keehn et al. (2013) explored functional connectivity during the first year of life in HR infants using a task-related experimental paradigm (language processing task). They found that from 3 to 12 months HR children exhibited a pattern of decreasing connectivity with reduced intra-hemispheric connectivity for the left hemisphere compared to LR infants at 12 months.
Moreover, an alteration of language processing can be detected very precociously with fNIRS: Edwards et al. (2017) analyzed precursors of language development in HR and LR 3-month-old infants: they found that HR females exhibited significantly higher OxyHb responses to auditory stimuli containing syllable repetitions than HR males, LR females or LR males in both left and right anterior brain regions. It is worth noting that HR females showed different temporal response patterns with respect to LR females across different type of auditory pattern (repeating vs. non-repeating syllabic sequences) presented randomly (speech-like stimuli), indicating a potential gender endophenotype in ASD. Using the same experimental protocol, Pecukonis et al. (2021) performed a longitudinal study on functional specialization of language-related brain regions during the first year of life in HR and LR group, suggesting a possible role of language-evoked fNIRS measurements to predict the diagnostic outcome of infants at 24 months.
Altogether, these data demonstrate that fNIRS studies on siblings can provide a unique window into the earliest neurobiological atypical trajectories and have the potential to shed light on possible ASD-related endophenotypes and biomarkers of brain function. In particular, studies correlating fNIRS brain responses in the first year of life to the diagnostic outcome at 24–36 months (Lloyd-Fox et al., 2018; Pecukonis et al., 2021) highlight that atypical brain responses are detectable in ASD infants as early as 6 months, thus indicating that fNIRS might be the an useful tool to early predict ASD.
Is Hemispheric Asymmetry an Informative Index in fNIRS Recordings?
Theoretical speculations about possible left-hemisphere dysfunction (Fein et al., 1984) or predominant right-hemisphere impairment (Happé, 1999) have historical roots in autism research. Many reports of atypical hemispheric asymmetries in ASD came from anatomical (Herbert et al., 2005) and functional imaging studies (Kleinhans et al., 2008), and typically focused on the abnormal lateralization of language domain (Just et al., 2004), even at very early stages of infants’ development (Eyler et al., 2012). Nevertheless, it is only in the last decade that attention on functional asymmetries related to non-verbal processing and the possibility to consider atypical brain asymmetry as a candidate for clinically meaningful stratification in ASD has grown (Floris et al., 2021). Indeed, recent evidence from resting-state fMRI studies supported the hypothesis that atypical rightward asymmetry shift may be a pervasive feature of functional brain organization in ASD, affecting not only the language network, but also the sensorimotor function and higher cognitive domains (Cardinale et al., 2013). Task-related – fNIRS studies focusing on hemispheric asymmetry in ASD individuals are still very limited and evidence reported are quite inconsistent (Doi and Shinohara, 2017). No systematic findings on hemispheric asymmetry in ASD population during resting-state fNIRS recording are currently available. Even in the studies included in this review, hemispheric asymmetry was not highlighted as a primary research purpose, allowing us to prompt only a few speculations on this topic.
Functional near-infrared spectroscopy studies on language perception and processing seem to confirm previous literature about ASD atypical lateralization for the language domain, with a reduced functional connectivity in the left hemisphere of HR infants compared to the LR peers, both at 6- (Pecukonis et al., 2021) and 12-months of age (Keehn et al., 2013). In contrast, Edwards and colleagues (Edwards et al., 2017) found left- or even hyper-lateralization of neural activity in 3-month-old HR females in response to a language auditory task, speculating about a gender-specific ASD endophenotype related to language processing. However, the limited spatial resolution of fNIRS prevent these results from being conclusive.
Similarly, task-related fNIRS studies of social processing were not consistent in defining hemispheric asymmetry. Indeed, Lloyd-Fox et al. (2013, 2018) found cortical activation patterns in response to social vs. non-social stimuli showing maximal differences between HR and LR groups in the left STS region. Moreover, Braukmann et al. (2018) detected different evoked responses in the right temporal region between groups. In contrast other studies, despite reporting cortical hypoactivation in HR both in naturalistic social interactions (Bhat et al., 2019) and face processing (Fox et al., 2013), did not highlight a clear hemispheric asymmetry of responses.
Finally, resting-state fNIRS studies found a hypoactivation with rightward asymmetry shift in the prefrontal cortical area of ASD preschool children (Li and Yu, 2016; Li et al., 2018). Only two studies reported ASD-related alterations bilaterally (Kikuchi et al., 2013; Jia et al., 2018). However, these results could be influenced by technical aspects, such as the different number of channels used.
Even if no systematic assessment of hemispheric asymmetry has been performed with fNIRS in preschoolers, these findings and evidence from other neuroimaging technique such as structural (Conti et al., 2016) and functional MRI (Müller et al., 2011) would encourage studying more in depth atypical lateralization in ASD population, also at early stage.
Could fNIRS Be the Right Tool to Dissect the Vascular Contribution to ASD Condition?
Over the last years of research, the biological dimension of ASD has been extensively investigated both in the preclinical (Ebert and Greenberg, 2013; Del Pino et al., 2018) and the clinical settings (Billeci et al., 2016; Bralten et al., 2018). However, most studies only focused on neuronal mechanisms underlying ASD.
Preclinical studies on animal models can offer important insights about specific mechanisms underlying the pathophysiology of ASD (Bartz et al., 2008). Recent studies suggested the presence of structural and functional neurovascular abnormalities in ASD. Indeed, an early dysfunction of endothelial cells, altered angiogenesis and impaired vasodilation reactivity have been shown in a mouse model of 16p11.2 deletion ASD syndrome (Ouellette et al., 2020). Similarly, cerebrovascular deficiencies have been recognized in a mouse model of Creatine Transporter Deficiency, an inherited metabolic condition characterized by intellectual disability and autistic-like features (Mazziotti et al., 2020). The vascular hypothesis is also supported by clinical studies. Indeed, postmortem analysis of ASD brains suggested an impairment of cerebral angiogenesis (Azmitia et al., 2016) and resting-state imaging highlighted the alteration of cerebral blood flow in distinct brain regions (Jann et al., 2015; Bjørklund et al., 2018). Interestingly, it has been speculated that an exacerbated inflammatory response might be one of the possible causes of the deteriorated integrity of the blood-brain barrier (Fiorentino et al., 2016; Kumar and Sharma, 2016) and of the defective blood flow documented in the ASD brain (Morgan et al., 2010; Bjørklund et al., 2018). Moreover, there is evidence of glial dysfunction in ASD (Petrelli et al., 2016), with the alteration of astrocyte population (Fatemi et al., 2008; Edmonson et al., 2014; Wang et al., 2021) potentially contributing to the deregulation of neurovascular homeostasis.
We believe that the potential vascular contribution in the etiology of ASD should be explored more in-depth, since the interplay with the vascular system is crucial for the proper maturation and function of neuronal networks (Andreone et al., 2015; Segarra et al., 2018). In this framework, the combined analysis of hemodynamic responses measured with fNIRS and bioelectrical signals obtained through EEG recordings might allow dissecting vascular vs. neuronal aspects of ASD.
Conclusion
This review highlights theoretical and methodological advantages of fNIRS that encourage its application for identifying quantitative biomarkers in NDDs, and in particular in the autism field. Even if available papers in preschoolers are yet not conclusive to let us claim a “fNIRS signature” of autism, we believe that the use of this technique as an auxiliary diagnostic tool is very promising. Consistently, we recently reported that the variability of fNIRS visually evoked hemodynamic responses correlates with autistic traits in typically developing children, setting the background for testing the diagnostic value of fNIRS visual measurements in the ASD and HR clinical population (Mazziotti et al., 2022).
Nevertheless, some critical technical issues should be taken into account, because they might limit the interpretation of data and the comparison of results among different studies. First, the availability of reliable methods to detect and remove motion artifacts is fundamental for applying fNIRS in a very young population. Only a few of the studies reviewed (Edwards et al., 2017; Bhat et al., 2019; Pecukonis et al., 2021) systematically applied motion correction algorithms to the data recorded, while most of them simply removed corrupted data (caused by large head or body movements or other unexpected behaviors) by visual inspection of experiment video recordings. This methodological heterogeneity leads not only to a considerable failure rate of fNIRS data collection, but also to a possible bias in the results. Since this topic is debated, wide agreement on subtle technical parameters to apply is still missing even if they could significantly affect data outputs (Di Lorenzo et al., 2019). Moreover, in resting-state measurements complex algorithms to extract reliable data from the fNIRS raw output are often required, representing a potential bias and limiting factor for their clinical use. Indeed, several methodological models have been applied, like functional network efficiency (Li and Yu, 2016, 2018), detrended fluctuation analysis (Jia et al., 2018), coherence analysis of spontaneous hemodynamic fluctuations (Kikuchi et al., 2013) and a combination of principal component analysis and normalized entropy (Li et al., 2018). It is to note that all resting-state studies reviewed compared ASD vs. TD cohorts (3–7-year-old children) and included in the clinical sample only high-functioning autism, probably due to recording constraints (predictable low-grade of compliance during the experimental procedure) that prevent such experimental design in younger and more impaired subjects.
On the other hand, the analysis of articles reviewed in this survey offers several cues of topics to systematically stress in the future fNIRS research within the autism field. Only one of the 13 articles included a focus on the suitability of fNIRS to highlight gender differences in HR infants (Edwards et al., 2017) and the small sample size of other studies (or the lack of a gender-balanced population) did not allow separating gender analyses. However, since consistent findings reported sexual dimorphism of specific brain regions and networks in ASD (see Mo et al., 2021 for a recent review), a specific focus on gender-related brain differences in well-powered cohorts might be an added value of next fNIRS assessments. Similarly, more weight should be given to the extrapolation of asymmetry indexes from fNIRS signals that might represent intra-subject measures potentially useful as condition biomarkers. Moreover, previous studies (Burzi et al., 2015; Jones et al., 2015) explored the importance of imitative learning during early development, focusing on differences in brain activation evoked from live (real time actions) rather than artificial stimuli (videos or photos of actions). While the others used artificial stimuli was applied (Bhat et al., 2019), while in the others artificial stimuli were used. The putative role of stimulus “quality” is still challenging to assess and it might deserve attention in future studies.
Finally, the possibility to measure hemodynamic response with fNIRS would allow the investigation of the possible role of neurovascular coupling in the pathogenesis of ASD, in particular when paired with simultaneous EEG recordings. In this framework, the evolution of hemodynamic patterns from the developing to the adult brain (Kozberg and Hillman, 2016) needs to be considered in the interpretation of typical and aberrant fNIRS signals.
Author Contributions
EC and ES equally contributed to conceptualization of the manuscript, studies selection, interpretation of results, and manuscript writing. CB contributed to systematic search of the literature. VM, VC, VD, and RM contributed to the conceptualization of the study and interpretation of results. LD, CC, and FM supervised the manuscript. LB contributed to conceptualization, interpretation of technical results, and manuscript writing. SC and RB contributed to conceptualization and supervised the manuscript. All authors have read and agreed the final version of the manuscript.
Funding
This work has been supported by grant from the IRCCS Fondazione Stella Maris (Ricerca Corrente – RC 2021: Early functional and neurobiological markers of rare diseases associated to neurodevelopmental disorders -RB- and the 5 × 1000 voluntary contributions, Italian Ministry of Health), and from the University of Pisa, “Bando PRA-Progetti di Ricerca di Ateneo” (Institutional Research Grants) 2020–2021 “Multimodal non-invasive approach in young females with autism and their parents: a feasibility study.”
Conflict of Interest
The authors declare that the research was conducted in the absence of any commercial or financial relationships that could be construed as a potential conflict of interest.
Publisher’s Note
All claims expressed in this article are solely those of the authors and do not necessarily represent those of their affiliated organizations, or those of the publisher, the editors and the reviewers. Any product that may be evaluated in this article, or claim that may be made by its manufacturer, is not guaranteed or endorsed by the publisher.
Acknowledgments
EC and SC acknowledge AIMS-2-TRIALS network.
References
American Psychiatric Association (2013). Diagnostic and Statistical Manual of Mental Disorders. Arlingon: American Psychiatric Publishing.
Andreone, B. J., Lacoste, B., and Gu, C. (2015). Neuronal and vascular interactions. Annu. Rev. Neurosci. 38, 25–46. doi: 10.1146/annurev-neuro-071714-033835
Azhari, A., Truzzi, A., Neoh, M. J., Balagtas, J. P. M., Tan, H. H., Goh, P. P., et al. (2020). A decade of infant neuroimaging research: what have we learned and where are we going? Infant. Behav. Dev. 58:101389. doi: 10.1016/j.infbeh.2019.101389
Azmitia, E. C., Saccomano, Z. T., Alzoobaee, M. F., Boldrini, M., and Whitaker-Azmitia, P. M. (2016). Persistent Angiogenesis in the Autism Brain: an Immunocytochemical Study of Postmortem Cortex. Brainstem and Cerebellum. J Autism. Dev. Disord. 46, 1307–1318. doi: 10.1007/s10803-015-2672-6
Bartz, J. A., Young, L. J., Hollander, E., Buxbaum, J. D., and Ring, R. H. (2008). “Preclinical animal models of autistic spectrum disorders,” in Animal and Translational Models for CNS Drug Discovery, Vol. 1, eds R. McArthur and F. Borsini (Amsterdam: Elsevier Inc), 353–394. https://doi.org/10.1016/B978-0-12-373861-5.00011-4
Bembich, S., Morabito, G., Simeon, V., Strajn, T., Rutigliano, R., Di Rocco, P., et al. (2021). Venipuncture activates the cerebral cortex in children with intellectual disability. Arch. Dis. Child. 106, 167–172. doi: 10.1136/archdischild-2019-318695
Berger, A., Horst, F., Müller, S., Steinberg, F., and Doppelmayr, M. (2019). Current State and Future Prospects of EEG and fNIRS in Robot-Assisted Gait Rehabilitation: a Brief Review. Front. Hum. Neurosci. 13:172. doi: 10.3389/fnhum.2019.00172
Bhat, A. N., McDonald, N. M., Eilbott, J. E., and Pelphrey, K. A. (2019). Exploring cortical activation and connectivity in infants with and without familial risk for autism during naturalistic social interactions: a preliminary study. Infant. Behav. Dev. 57:101337. doi: 10.1016/j.infbeh.2019.101337
Billeci, L., Calderoni, S., Conti, E., Gesi, C., Carmassi, C., Dell’Osso, L., et al. (2016). The Broad Autism (Endo)Phenotype: neurostructural and Neurofunctional Correlates in Parents of Individuals with Autism Spectrum Disorders. Front. Neurosci. 10:346. doi: 10.3389/fnins.2016.00346
Bjørklund, G., Kern, J. K., Urbina, M. A., Saad, K., El-Houfey, A. A., Geier, D. A., et al. (2018). Cerebral hypoperfusion in autism spectrum disorder. Acta. Neurobiol. Exp. 78, 21–29.
Bosl, W., Tierney, A., Tager-Flusberg, H., and Nelson, C. (2011). EEG complexity as a biomarker for autism spectrum disorder risk. BMC Med. 9:18. doi: 10.1186/1741-7015-9-18
Bralten, J., van Hulzen, K. J., Martens, M. B., Galesloot, T. E., Arias Vasquez, A., Kiemeney, L. A., et al. (2018). Autism spectrum disorders and autistic traits share genetics and biology. Mol. Psychiatry 23, 1205–1212. doi: 10.1038/mp.2017.98
Braukmann, R., Lloyd-Fox, S., Blasi, A., Johnson, M. H., Bekkering, H., Buitelaar, J. K., et al. (2018). Diminished socially selective neural processing in 5-month-old infants at high familial risk of autism. Eur. J. Neurosci. 47, 720–728. doi: 10.1111/ejn.13751
Burzi, V., Marchi, V., Boyd, R. N., Mazziotti, R., Moscarelli, M., Sgherri, G., et al. (2015). Brain representation of action observation in human infants. Dev. Med. Child. Neurol. 57(Suppl. 2), 26–30. doi: 10.1111/dmcn.12693
Cardinale, R. C., Shih, P., Fishman, I., Ford, L. M., and Müller, R. A. (2013). Pervasive rightward asymmetry shifts of functional networks in autism spectrum disorder. JAMA Psychiatry 70, 975–982. doi: 10.1001/jamapsychiatry.2013.382
Chang, S. E. (2014). Research updates in neuroimaging studies of children who stutter. Semin. Speech Lang. 35, 67–79. doi: 10.1055/s-0034-1382151
Charman, T., Young, G. S., Brian, J., Carter, A., Carver, L. J., Chawarska, K., et al. (2017). Non-ASD outcomes at 36 months in siblings at familial risk for autism spectrum disorder (ASD): a baby siblings research consortium (BSRC) study. Autism Res. 10, 169–178. doi: 10.1002/aur.1669
Chen, L. C., Sandmann, P., Thorne, J. D., Herrmann, C. S., and Debener, S. (2015). Association of Concurrent fNIRS and EEG Signatures in Response to Auditory and Visual Stimuli. Brain Topogr. 28, 710–725. doi: 10.1007/s10548-015-0424-8
Chiarelli, A. M., Zappasodi, F., Di Pompeo, F., and Merla, A. (2017). Simultaneous functional near-infrared spectroscopy and electroencephalography for monitoring of human brain activity and oxygenation: a review. Neurophotonics. 4:041411. doi: 10.1117/1.NPh.4.4.041411
Conti, E., Calderoni, S., Gaglianese, A., Pannek, K., Mazzotti, S., Rose, S., et al. (2016). Lateralization of Brain Networks and Clinical Severity in Toddlers with Autism Spectrum Disorder: a HARDI Diffusion MRI Study. Autism Res. 9, 382–392. doi: 10.1002/aur.1533
Conti, E., Calderoni, S., Marchi, V., Muratori, F., Cioni, G., and Guzzetta, A. (2015). The first 1000 days of the autistic brain: a systematic review of diffusion imaging studies. Front. Hum. Neurosci. 9:159. doi: 10.3389/fnhum.2015.00159
Courchesne, E., Campbell, K., and Solso, S. (2011). Brain growth across the life span in autism: age-specific changes in anatomical pathology. Brain Res. 1380, 138–145. doi: 10.1016/j.brainres.2010.09.101
Cui, X., Bray, S., Bryant, D. M., Glover, G. H., and Reiss, A. L. (2011). A quantitative comparison of NIRS and fMRI across multiple cognitive tasks. Neuroimage. 54, 2808–2821. doi: 10.1016/j.neuroimage.2010.10.069
Cutini, S., Szűcs, D., Mead, N., Huss, M., and Goswami, U. (2016). Atypical right hemisphere response to slow temporal modulations in children with developmental dyslexia. Neuroimage. 143, 40–49. doi: 10.1016/j.neuroimage.2016.08.012
Dawson, G., Rogers, S., Munson, J., Smith, M., Winter, J., Greenson, J., et al. (2010). Randomized, controlled trial of an intervention for toddlers with autism: the Early Start Denver Model. Pediatrics. 125, e17–e23. doi: 10.1542/peds.2009-0958
Del Pino, I., Rico, B., and Marín, O. (2018). Neural circuit dysfunction in mouse models of neurodevelopmental disorders. Curr. Opin. Neurobiol. 48, 174–182. doi: 10.1016/j.conb.2017.12.013
Di Lorenzo, R., Pirazzoli, L., Blasi, A., Bulgarelli, C., Hakuno, Y., Minagawa, Y., et al. (2019). Recommendations for motion correction of infant fNIRS data applicable to multiple data sets and acquisition systems. Neuroimage. 200, 511–527. doi: 10.1016/j.neuroimage.2019.06.056
Doi, H., and Shinohara, K. (2017). fNIRS Studies on Hemispheric Asymmetry in Atypical Neural Function in Developmental Disorders. Front. Hum. Neurosci. 11:137. doi: 10.3389/fnhum.2017.00137
Duan, L., Zhang, Y. J., and Zhu, C. Z. (2012). Quantitative comparison of resting-state functional connectivity derived from fNIRS and fMRI: a simultaneous recording study. Neuroimage. 60, 2008–2018. doi: 10.1016/j.neuroimage.2012.02.014
Ebert, D. H., and Greenberg, M. E. (2013). Activity-dependent neuronal signalling and autism spectrum disorder. Nature. 493, 327–337. doi: 10.1038/nature11860
Edmonson, C., Ziats, M. N., and Rennert, O. M. (2014). Altered glial marker expression in autistic post-mortem prefrontal cortex and cerebellum. Mol. Autism. 5:3. doi: 10.1186/2040-2392-5-3
Edwards, L. A., Wagner, J. B., Tager-Flusberg, H., and Nelson, C. A. (2017). Differences in Neural Correlates of Speech Perception in 3 Month Olds at High and Low Risk for Autism Spectrum Disorder. J. Autism. Dev. Disord. 47, 3125–3138. doi: 10.1007/s10803-017-3222-1
Elsabbagh, M., and Johnson, M. H. (2016). Autism and the Social Brain: the First-Year Puzzle. Biol Psychiatry. 80, 94–99. doi: 10.1016/j.biopsych.2016.02.019
Emerson, R. W., Adams, C., Nishino, T., Hazlett, H. C., Wolff, J. J., Zwaigenbaum, L., et al. (2017). Functional neuroimaging of high-risk 6-month-old infants predicts a diagnosis of autism at 24 months of age. Sci. Transl. Med. 9:eaag2882. doi: 10.1126/scitranslmed.aag2882
Eyler, L. T., Pierce, K., and Courchesne, E. (2012). A failure of left temporal cortex to specialize for language is an early emerging and fundamental property of autism. Brain 135(Pt 3), 949–960. doi: 10.1093/brain/awr364
Fatemi, S. H., Folsom, T. D., Reutiman, T. J., and Lee, S. (2008). Expression of astrocytic markers aquaporin 4 and connexin 43 is altered in brains of subjects with autism. Synapse 62, 501–507. doi: 10.1002/syn.20519
Fein, D., Humes, M., Kaplan, E., Lucci, D., and Waterhouse, L. (1984). The question of left hemisphere dysfunction in infantile autism. Psychol. Bull. 95, 258–281. doi: 10.1037//0033-2909.95.2.258
Fiorentino, M., Sapone, A., Senger, S., Camhi, S. S., Kadzielski, S. M., Buie, T. M., et al. (2016). Blood-brain barrier and intestinal epithelial barrier alterations in autism spectrum disorders. Mol. Autism. 7:49. doi: 10.1186/s13229-016-0110-z
Floris, D. L., Wolfers, T., Zabihi, M., Holz, N. E., Zwiers, M. P., Charman, T., et al. (2021). EU-AIMS Longitudinal European Autism Project Group. Atypical Brain Asymmetry in Autism-A Candidate for Clinically Meaningful Stratification. Biol. Psychiatry Cogn. Neurosci. Neuroimaging. 6, 802–812. doi: 10.1016/j.bpsc.2020.08.008
Fox, S. E., Wagner, J. B., Shrock, C. L., Tager-Flusberg, H., and Nelson, C. A. (2013). Neural processing of facial identity and emotion in infants at high-risk for autism spectrum disorders. Front. Hum. Neurosci. 7:89. doi: 10.3389/fnhum.2013.00089
Gervain, J., Mehler, J., Werker, J. F., Nelson, C. A., Csibra, G., Lloyd-Fox, S., et al. (2011). Near-infrared spectroscopy: a report from the McDonnell infant methodology consortium. Dev. Cogn. Neurosci. 1, 22–46. doi: 10.1016/j.dcn.2010.07.004
Greisen, G. (2006). Is near-infrared spectroscopy living up to its promises? Semin. Fetal. Neonatal. Med. 11, 498–502. doi: 10.1016/j.siny.2006.07.010
Ha, S., Sohn, I. J., Kim, N., Sim, H. J., and Cheon, K. A. (2015). Characteristics of Brains in Autism Spectrum Disorder: structure, Function and Connectivity across the Lifespan. Exp. Neurobiol. 24, 273–284. doi: 10.5607/en.2015.24.4.273
Hansen, B. H., Oerbeck, B., Skirbekk, B., and Petrovski, B. É, and Kristensen, H. (2018). Neurodevelopmental disorders: prevalence and comorbidity in children referred to mental health services. Nord J. Psychiatry 72, 285–291. doi: 10.1080/08039488.2018.1444087
Happé, F. (1999). Autism: cognitive deficit or cognitive style? Trends Cogn. Sci. 3, 216–222. doi: 10.1016/s1364-6613(99)01318-2
Herbert, M. R., Ziegler, D. A., Deutsch, C. K., O’Brien, L. M., Kennedy, D. N., Filipek, P. A., et al. (2005). Brain asymmetries in autism and developmental language disorder: a nested whole-brain analysis. Brain 128(Pt 1), 213–226. doi: 10.1093/brain/awh330
Hosseini, R., Walsh, B., Tian, F., and Wang, S. (2018). An fNIRS-Based Feature Learning and Classification Framework to Distinguish Hemodynamic Patterns in Children Who Stutter. IEEE Trans Neural Syst. Rehabil. Eng. 26, 1254–1263. doi: 10.1109/TNSRE.2018.2829083
Hyman, S. L., Levey, S. E., and Myers, S. M. (2020). Council on Children with Disabilities, Section on Developmental and Behavioral Pediatrics. Identification, Evaluation, and Management of Children With Autism Spectrum Disorder. Peditarics. 145:e20193447. doi: 10.1542/peds.2019-3447
Jann, K., Hernandez, L. M., Beck-Pancer, D., McCarron, R., Smith, R. X., Dapretto, M., et al. (2015). Altered resting perfusion and functional connectivity of default mode network in youth with autism spectrum disorder. Brain Behav. 5:e00358. doi: 10.1002/brb3.358
Jia, H., Li, Y., and Yu, D. (2018). Attenuation of long-range temporal correlations of neuronal oscillations in young children with autism spectrum disorder. Neuroimage Clin. 20, 424–432. doi: 10.1016/j.nicl.2018.08.012
Jones, E. J., Venema, K., Lowy, R., Earl, R. K., and Webb, S. J. (2015). Developmental changes in infant brain activity during naturalistic social experiences. Dev. Psychobiol. 57, 842–853. doi: 10.1002/dev.21336
Just, M. A., Cherkassky, V. L., Keller, T. A., and Minshew, N. J. (2004). Cortical activation and synchronization during sentence comprehension in high-functioning autism: evidence of underconnectivity. Brain 127(Pt 8), 1811–1821. doi: 10.1093/brain/awh199
Keehn, B., Wagner, J. B., Tager-Flusberg, H., and Nelson, C. A. (2013). Functional connectivity in the first year of life in infants at-risk for autism: a preliminary near-infrared spectroscopy study. Front. Hum. Neurosci. 7:444. doi: 10.3389/fnhum.2013.00444
Kikuchi, M., Yoshimura, Y., Shitamichi, K., Ueno, S., Hiraishi, H., Munesue, T., et al. (2013). Anterior prefrontal hemodynamic connectivity in conscious 3- to 7-year-old children with typical development and autism spectrum disorder. PLoS One 8:e56087. doi: 10.1371/journal.pone.0056087
Kleinhans, N. M., Müller, R. A., Cohen, D. N., and Courchesne, E. (2008). Atypical functional lateralization of language in autism spectrum disorders. Brain Res. 1221, 115–125. doi: 10.1016/j.brainres.2008.04.080
Kozberg, M., and Hillman, E. (2016). Neurovascular coupling and energy metabolism in the developing brain. Prog Brain Res. 225, 213–242. doi: 10.1016/bs.pbr.2016.02.002
Kumar, H., and Sharma, B. (2016). Memantine ameliorates autistic behavior, biochemistry & blood brain barrier impairments in rats. Brain Res Bull. 124, 27–39. doi: 10.1016/j.brainresbull.2016.03.013
Li, Y., Jia, H., and Yu, D. (2018). Novel analysis of fNIRS acquired dynamic hemoglobin concentrations: application in young children with autism spectrum disorder. Biomed. Opt. Express. 9, 3694–3710. doi: 10.1364/BOE.9.003694
Li, Y., and Yu, D. (2016). Weak network efficiency in young children with Autism Spectrum Disorder: evidence from a functional near-infrared spectroscopy study. Brain Cogn. 108, 47–55. doi: 10.1016/j.bandc.2016.07.006
Li, Y., and Yu, D. (2018). Variations of the Functional Brain Network Efficiency in a Young Clinical Sample within the Autism Spectrum: a fNIRS Investigation. Front. Physiol. 9:67. doi: 10.3389/fphys.2018.00067
Lines, J., Martin, E. D., Kofuji, P., Aguilar, J., and Araque, A. (2020). Astrocytes modulate sensory-evoked neuronal network activity. Nat. Commun. 11:3689. doi: 10.1038/s41467-020-17536-3
Liu, T., Liu, X., Yi, L., Zhu, C., Markey, P. S., and Pelowski, M. (2019). Assessing autism at its social and developmental roots: a review of Autism Spectrum Disorder studies using functional near-infrared spectroscopy. Neuroimage 185, 955–967. doi: 10.1016/j.neuroimage.2017.09.044
Lloyd-Fox, S., Blasi, A., and Elwell, C. E. (2010). Illuminating the developing brain: the past, present and future of functional near infrared spectroscopy. Neurosci. Biobehav. Rev. 34, 269–284. doi: 10.1016/j.neubiorev.2009.07.008
Lloyd-Fox, S., Blasi, A., Elwell, C. E., Charman, T., Murphy, D., and Johnson, M. H. (2013). Reduced neural sensitivity to social stimuli in infants at risk for autism. Proc. Biol. Sci. 280:20123026. doi: 10.1098/rspb.2012.3026
Lloyd-Fox, S., Blasi, A., Mercure, E., Elwell, C. E., and Johnson, M. H. (2012). The emergence of cerebral specialization for the human voice over the first months of life. Soc. Neurosci. 7, 317–330. doi: 10.1080/17470919.2011.614696
Lloyd-Fox, S., Blasi, A., Pasco, G., Gliga, T., Jones, E. J. H., Murphy, D. G. M., et al. (2018). BASIS Team. Cortical responses before 6 months of life associate with later autism. Eur. J. Neurosci. 47, 736–749. doi: 10.1111/ejn.13757
Logothetis, N. K., and Wandell, B. A. (2004). Interpreting the BOLD signal. Annu. Rev. Physiol. 66, 735–769. doi: 10.1146/annurev.physiol.66.082602.092845
Lombardo, M. V., Lai, M. C., and Baron-Cohen, S. (2019). Big data approaches to decomposing heterogeneity across the autism spectrum. Mol. Psychiatry. 24, 1435–1450. doi: 10.1038/s41380-018-0321-0
Lord, C., Rutter, M., DiLavore, P., Risi, S., Gotham, K., and Bishop, S. (2012). Autism Diagnostic Observation Schedule–2nd edition (ADOS-2). Alaska: Western Psychological Corporation.
Loth, E., Murphy, D. G., and Spooren, W. (2016). Defining Precision Medicine Approaches to Autism Spectrum Disorders:Concepts and Challenges. Front. Psychiat. 7:188. doi: 10.3389/fpsyt.2016.00188
Mazziotti, R., Cacciante, F., Sagona, G., Lupori, L., Gennaro, M., Putignano, E., et al. (2020). Novel translational phenotypes and biomarkers for creatine transporter deficiency. Brain Commun. 2:fcaa089. doi: 10.1093/braincomms/fcaa089
Mazziotti, R., Scaffei, E., Conti, E., Marchi, V., Rizzi, R., Cioni, G., et al. (2022). The amplitude of fNIRS hemodynamic response in the visual cortex unmasks autistic traits in typically developing children. Transl. Psychiatry. 12:53. doi: 10.1038/s41398-022-01820-5
McCaffery, P., and Deutsch, C. K. (2005). Macrocephaly and the control of brain growth in autistic disorders. Prog. Neurobiol. 77, 38–56. doi: 10.1016/j.pneurobio.2005.10.005
Messinger, D., Young, G. S., Ozonoff, S., Dobkins, K., Carter, A., Zwaigenbaum, L., et al. (2013). Beyond autism: a baby siblings research consortium study of high-risk children at three years of age. J. Am. Acad. Child Adolesc. Psychiat. 52, 300.e–308.e. doi: 10.1016/j.jaac.2012.12.011
Mo, K., Sadoway, T., Bonato, S., Ameis, S. H., Anagnostou, E., Lerch, J. P., et al. (2021). Sex/gender differences in the human autistic brains: a systematic review of 20 years of neuroimaging research. Neuroimage Clin. 32:102811. doi: 10.1016/j.nicl.2021.102811
Morgan, J. T., Chana, G., Pardo, C. A., Achim, C., Semendeferi, K., Buckwalter, J., et al. (2010). Microglial activation and increased microglial density observed in the dorsolateral prefrontal cortex in autism. Biol. Psychiatry. 68, 368–376. doi: 10.1016/j.biopsych.2010.05.024
Muhle, R., Trentacoste, S. V., and Rapin, I. (2004). The genetics of autism. Pediatrics 113, e472–e486. doi: 10.1542/peds.113.5.e472
Müller, R. A., Shih, P., Keehn, B., Deyoe, J. R., Leyden, K. M., and Shukla, D. K. (2011). Underconnected, but how? A survey of functional connectivity MRI studies in autism spectrum disorders. Cereb. Cortex. 21, 2233–2243. doi: 10.1093/cercor/bhq296
O’Reilly, C., Lewis, J. D., and Elsabbagh, M. (2017). Is functional brain connectivity atypical in autism? A systematic review of EEG and MEG studies. PLoS One 12:e0175870. doi: 10.1371/journal.pone.0175870
Ouellette, J., Toussay, X., Comin, C. H., Costa, L. D. F., Ho, M., Lacalle-Aurioles, M., et al. (2020). Vascular contributions to 16p11.2 deletion autism syndrome modeled in mice. Nat. Neurosci. 23, 1090–1101. doi: 10.1038/s41593-020-0663-1
Ozonoff, S., Young, G. S., Carter, A., Messinger, D., Yirmiya, N., Zwaigenbaum, L., et al. (2011). Recurrence risk for autism spectrum disorders: a Baby Siblings Research Consortium study. Pediatrics 128, e488–e495. doi: 10.1542/peds.2010-2825
Pagnozzi, A. M., Conti, E., Calderoni, S., Fripp, J., and Rose, S. E. (2018). A systematic review of structural MRI biomarkers in autism spectrum disorder: a machine learning perspective. Int. J. Dev. Neurosci. 71, 68–82. doi: 10.1016/j.ijdevneu.2018.08.010
Pecukonis, M., Perdue, K. L., Wong, J., Tager-Flusberg, H., and Nelson, C. A. (2021). Exploring the relation between brain response to speech at 6-months and language outcomes at 24-months in infants at high and low risk for autism spectrum disorder: a preliminary functional near-infrared spectroscopy study. Dev. Cogn. Neurosci. 47:100897. doi: 10.1016/j.dcn.2020.100897
Pecyna, M. B., and Pokorski, M. (2013). Near-infrared hemoencephalography for monitoring blood oxygenation in prefrontal cortical areas in diagnosis and therapy of developmental dyslexia. Adv. Exp. Med. Biol. 788, 175–180. doi: 10.1007/978-94-007-6627-3_26
Petrelli, F., Pucci, L., and Bezzi, P. (2016). Astrocytes and Microglia and Their Potential Link with Autism Spectrum Disorders. Front. Cell Neurosci. 10:21. doi: 10.3389/fncel.2016.00021
Salomone, E., Charman, T., McConachie, H., and Warreyn, P. (2016). Child’s verbal ability and gender are associated with age at diagnosis in a sample of young children with ASD in Europe. Child Care Health Dev. 42, 141–145. doi: 10.1111/cch.12261
Scarapicchia, V., Brown, C., Mayo, C., and Gawryluk, J. R. (2017). Functional Magnetic Resonance Imaging and Functional Near-Infrared Spectroscopy: insights from Combined Recording Studies. Front. Hum. Neurosci. 11:419. doi: 10.3389/fnhum.2017.00419
Segarra, M., Aburto, M. R., Cop, F., Llaó-Cid, C., Härtl, R., Damm, M., et al. (2018). Endothelial Dab1 signaling orchestrates neuro-glia-vessel communication in the central nervous system. Science 361:eaao2861. doi: 10.1126/science.aao2861
Sela, I., Izzetoglu, M., Izzetoglu, K., and Onaral, B. (2014). A functional near-infrared spectroscopy study of lexical decision task supports the dual route model and the phonological deficit theory of dyslexia. J. Learn Disabil. 47, 279–288. doi: 10.1177/0022219412451998
Shen, M. D., Nordahl, C. W., Li, D. D., Lee, A., Angkustsiri, K., Emerson, R. W., et al. (2018). Extra-axial cerebrospinal fluid in high-risk and normal-risk children with autism aged 2-4 years: a case-control study. Lancet Psychiatr. 5, 895–904. doi: 10.1016/S2215-0366(18)30294-3
Solso, S., Xu, R., Proudfoot, J., Hagler, D. J. Jr., Campbell, K., Venkatraman, V., et al. (2016). Diffusion Tensor Imaging Provides Evidence of Possible Axonal Overconnectivity in Frontal Lobes in Autism Spectrum Disorder Toddlers. Biol. Psychiat. 79, 676–684. doi: 10.1016/j.biopsych.2015.06.029
Song, R., Zhang, J., Wang, B., Zhang, H., and Wu, H. (2013). A near-infrared brain function study of Chinese dyslexic children. Neurocase 19, 382–389. doi: 10.1080/13554794.2012.690422
Uddin, L. Q., Supekar, K., and Menon, V. (2013). Reconceptualizing functional brain connectivity in autism from a developmental perspective. Front. Hum. Neurosci. 7:458. doi: 10.3389/fnhum.2013.00458
Uludag, K., Steinbrink, J., Vilringer, A., and Obrig, H. (2004). Separability and cross talk: optimizing dual wavelength combinations for near-infrared spectroscopy of the adult head. NeuroImage 22, 583–589. doi: 10.1016/j.neuroimage.2004.02.023
Van de Rijt, L. P. H., van Wanrooij, M. M., Snik, A. F. M., Mylanus, E. A. M., van Opstal, A. J., and Roye, A. (2018). Measuring Cortical Activity During Auditory Processing with Functional Near-Infrared Spectroscopy. J. Hear. Sci. 8, 9–18. doi: 10.17430/1003278
Vanderwert, R. E., and Nelson, C. A. (2014). The use of near-infrared spectroscopy in the study of typical and atypical development. Neuroimage 85(Pt 1), 264–271. doi: 10.1016/j.neuroimage.2013.10.009
Vasung, L., Abaci Turk, E., Ferradal, S. L., Sutin, J., Stout, J. N., Ahtam, B., et al. (2019). Exploring early human brain development with structural and physiological neuroimaging. Neuroimage 187, 226–254. doi: 10.1016/j.neuroimage.2018.07.041
Villringer, A., and Chance, B. (1997). Non-invasive optical spectroscopy and imaging of human brain function. Trends Neurosci. 20, 435–442. doi: 10.1016/s0166-2236(97)01132-6
Wang, Q., Kong, Y., Wu, D. Y., Liu, J. H., Jie, W., You, Q. L., et al. (2021). Impaired calcium signaling in astrocytes modulates autism spectrum disorder-like behaviors in mice. Nat. Commun. 12:3321. doi: 10.1038/s41467-021-23843-0
Wolff, J. J., Gu, H., Gerig, G., Elison, J. T., Styner, M., Gouttard, S., et al. (2012). IBIS Network. Differences in white matter fiber tract development present from 6 to 24 months in infants with autism. Am. J. Psychiat. 169, 589–600. doi: 10.1176/appi.ajp.2011.11091447
Xu, L., Sun, Z., Xie, J., Yu, J., Li, J., and Wang, J. (2021). Identification of autism spectrum disorder based on short-term spontaneous hemodynamic fluctuations using deep learning in a multi-layer neural network. Clin. Neurophysiol. 132, 457–468. doi: 10.1016/j.clinph.2020.11.037
Yanagisawa, K., Nakamura, N., Tsunashima, H., and Narita, N. (2016). Proposal of auxiliary diagnosis index for autism spectrum disorder using near-infrared spectroscopy. Neurophotonics. 3, 031413. doi: 10.1117/1.NPh.3.3.031413
Yuan, H., Liu, T., Szarkowski, R., Rios, C., Ashe, J., and He, B. (2010). Negative covariation between task-related responses in alpha/beta-band activity and BOLD in human sensorimotor cortex: an EEG and fMRI study of motor imagery and movements. Neuroimage. 49, 2596–2606. doi: 10.1016/j.neuroimage.2009.10.028
Zhang, F., and Roeyers, H. (2019). Exploring brain functions in autism spectrum disorder: a systematic review on functional near-infrared spectroscopy (fNIRS) studies. Int. J. Psychophysiol. 137, 41–53. doi: 10.1016/j.ijpsycho.2019.01.003
Zhu, D., Wang, J., and Wu, H. (2012). Working memory function in Chinese dyslexic children: a near-infrared spectroscopy study. J. Huazhong Univ. Sci. Technol. Med. Sci. 32, 141–145. doi: 10.1007/s11596-012-0025-7
Keywords: fNIRS, near-infrared spectroscopy, functional neuroimaging, preschooler, neurodevelopmental disorders, autism spectrum disorder, high-risk infant
Citation: Conti E, Scaffei E, Bosetti C, Marchi V, Costanzo V, Dell’Oste V, Mazziotti R, Dell’Osso L, Carmassi C, Muratori F, Baroncelli L, Calderoni S and Battini R (2022) Looking for “fNIRS Signature” in Autism Spectrum: A Systematic Review Starting From Preschoolers. Front. Neurosci. 16:785993. doi: 10.3389/fnins.2022.785993
Received: 29 September 2021; Accepted: 08 February 2022;
Published: 02 March 2022.
Edited by:
Marco Turi, Fondazione Stella Maris Mediterraneo Onlus, ItalyReviewed by:
Jennifer L. Bruno, Stanford University, United StatesMladen-Roko Rasin, Rutgers, The State University of New Jersey, United States
Copyright © 2022 Conti, Scaffei, Bosetti, Marchi, Costanzo, Dell’Oste, Mazziotti, Dell’Osso, Carmassi, Muratori, Baroncelli, Calderoni and Battini. This is an open-access article distributed under the terms of the Creative Commons Attribution License (CC BY). The use, distribution or reproduction in other forums is permitted, provided the original author(s) and the copyright owner(s) are credited and that the original publication in this journal is cited, in accordance with accepted academic practice. No use, distribution or reproduction is permitted which does not comply with these terms.
*Correspondence: Elena Scaffei, ZWxlbmEuc2NhZmZlaUBmc20udW5pcGkuaXQ=
†These authors have contributed equally to this work and share first authorship