- 1Department of Neurology, The First Affiliated Hospital of Yangtze University, Jingzhou, China
- 2Department of Neurology, Zhongnan Hospital of Wuhan University, Wuhan, China
- 3Qingdao Mental Health Center, Qingdao University, Qingdao, China
- 4McGovern Medical School, Houston, TX, United States
- 5Department of Radiology, The First Affiliated Hospital of Yangtze University, Jingzhou, China
- 6Department of Psychiatry, The Affiliated Brain Hospital of Guangzhou Medical University, Guangzhou, China
- 7Guangdong Engineering Technology Research Center for Translational Medicine of Mental Disorders, Guangzhou, China
Background: Although cognitive deficit is a common non-motor symptom of Parkinson’s disease (PD), the mechanism and valid biomarkers of it have not been identified. To our best knowledge, this was the first study to investigate the intrinsic dysconnectivity pattern of whole-brain functional networks in early-stage drug-naive (ESDN) PD patients and its association with cognitive deficit of PD using voxel-wise Degree Centrality (DC) approach.
Methods: A total of 53 ESDN PD patients and 53 healthy controls (HC) were recruited. Resting-state fMRI (rs-fMRI) data were acquired, and voxel-wise DC approach was applied. Electrophysiological testing at P300 amplitude was recorded. The Montreal Cognitive Assessment (MoCA) was conducted to evaluate cognitive performance.
Results: ESDN PD patients had lower MoCA scores and P300 amplitudes, but higher P300 latency, than HC (all p < 0.0001). PD patients displayed higher DC in the right inferior frontal gyrus (IFG), left medial frontal gyrus (MFG) and left precentral gyrus (PreCG); but lower DC in the left inferior parietal lobule (IPL), left inferior temporal gyrus (ITG), right occipital lobe, and right postcentral gyrus (PoCG) (pBonferroni correction < 0.0001). Interestingly, the DC values of left MFG, right PoCG and right occipital lobe were negatively associated with P300 latency but positively associated with P300 amplitudes and MoCA scores (all pBonferroni correction < 0.0001).
Conclusions: Our results indicate the cognitive deficit and abnormal intrinsic brain functional network in ESDN PD patients. The damage of Default Mode Network (DMN) may be contributes to the pathogenesis of cognitive dysfunction in ESDN PD.
Introduction
Cognitive deficit is a common, non-motor symptom in the early to moderate stages of Parkinson’s disease (PD) (Aarsland et al., 2009), even in newly diagnosed PD patients without exposure to medication (Janvin et al., 2006; Aarsland et al., 2009). The incidence of cognitive deficit in early-stage, drug-naive (ESDN) PD patients is twice as high as that of the general population’s control subjects (Aarsland et al., 2009). With further progression of the disease, up to 80% of PD patients with mild cognitive deficit may develop PD dementia (PDD) (Hely et al., 2008). At present, the diagnosis of cognitive deficit mainly relies on the patient’s clinical symptoms and their cognitive scale evaluation results, lacking of objective examination results. Clinicians rarely pay attention to the cognitive function of ESDN PD patients for no obvious symptoms of cognitive impairment. Therefore, it is particularly important to identify cognitive deficit of PD patients early.
Currently, the pathophysiological mechanism of cognitive deficit in PD has not been elucidated, and no valid biomarkers have been identified, especially in early-stage PD patients. However, with the rapid development of functional magnetic resonance imaging (fMRI) technology in recent years, there is now a method for measuring brain changes in PD patients with cognitive deficit. Accumulating studies have shown abnormal default mode networks (DMN) (van Eimeren et al., 2009; Rektorova et al., 2012) and fronto-parietal networks (Tinaz et al., 2008; Lebedev et al., 2014) in PD patients with cognitive deficit. Previous evidence demonstrated that damage of the lobo-striatal circuit was the basis of cognitive and emotional disorders in PD patients (Owen, 2004). However, the results are still inconsistent, and most studies have not eliminated the confounding effect of drug treatment.
The development of graph theory-based methods provides a promising strategy to understand the characteristics of brain functional network. The Degree Centrality (DC) method, which is based on the degrees in the graph, could depict the importance of certain brain regions in the brain network by nodes. It also enables the investigation of these neural networks by detecting the number of instantaneous functional connectivity (FC) between one region and other brain region (Buckner et al., 2009). Notably, it was demonstrated that DC abnormalities can be observed in the early stages of several neuropsychiatric disorders, including schizophrenia (Wang et al., 2018), major depressive disorder (Zhang et al., 2011), and Alzheimer’s disease (Wang et al., 2013). Although the DC method can be effectively used to investigate brain functional activity changes of PD patients (Zhong et al., 2019), the DC abnormalities and its relationship with cognitive deficit in the ESDN PD patients has not been examined.
Because PD patients are prone to cognitive impairment and changes of resting-state brain function, we have a hypothesis that the ESDN PD patients also have cognitive impairment and brain function changes, and the brain function changes is related to cognitive function changes. To our best knowledge, this was the first study to investigate the intrinsic dysconnectivity pattern of whole-brain functional networks in ESDN PD and its association with cognitive deficit of PD through voxel-wise DC approach.
Materials and Methods
Study Participants
The study was approved by the Ethics Committee of the First Affiliated Hospital of Yangtze University. The procedure was carefully explained to all participants. Signed informed consent forms were obtained from all participants.
A total of 53 PD patients were recruited from the Department of Neurology, the First Affiliated Hospital of Yangtze University from May 2015 to May 2019. The inclusion criteria was as follows: (1) met the UK PD Society Brain Bank Criteria; (2) illness duration was less than 2 years, and Hoehn and Yahr (H&Y) stage ≤ 2 at baseline; (3) did not receive any anti-PD or antidepressant medications before fMRI scans; (4) without any apparent cognitive impairment [Mini-Mental State Examination (MMSE) scores > 24] and were right-handed; All subjects were free of dementia according to their MMSE scores; and (5) All subjects did not met the Diagnostic and Statistical Manual of Mental Disorders-Fifth Edition criteria for depression/anxiety disorder, who were diagnosed using the Structured Clinical Interview for the Diagnostic and Statistical Manual of Mental Disorders-Fifth Edition and HAMA scale and HAMD scores.
A total of 53 matched healthy controls (HC) without personal or family history of neuropsychiatric disorders were recruited from the community. Furthermore, no cerebral lesions and cerebral atrophy were found by MRI. The age, sex and education were comparable between patient and control groups (all p > 0.05).
Cognitive Performance and Psychological Tests
All subjects were interviewed by neurologists. The Montreal Cognitive Assessment (MoCA) Scale was used to evaluate cognitive performance. Mini-Mental State Exam (MMSE) was applied to screen for dementia. Unified Parkinson’s Disease Rating Scale Part-III (UPDRS-III) was applied to evaluate motor symptoms. Hoehn and Yahr scale was applied to evaluate PD progression symptoms. The17-Hamilton Rating Scale for Depression (HDRS-17) and the Hamilton Anxiety Rating Scale (HAMA) were applied to assess depressive and anxiety symptoms, respectively. All raters were conducted by two neurologists with a correlation coefficient of more than 0.8.
P300 Testing
P300 is an electrophysiological indicator for cognitive performance. The P300 amplitude reflects the capability of receiving external information and the amount of resources invested. The P300 latency represents cognitive performance and advanced thinking activity (Frodl et al., 2002). We recorded the P300 using the KeyPoint Brain Electrical Physiological Instrument. The subjects received short tone stimulation in both ears with a frequency of 1/s, a duration of 10 ms, and a sensitivity of 5 μV. Two sets of trigger and stimulus systems and two independent time windows were used to detect P300. The non-target stimulus (NT) intensity was 85 dB, and the frequency was 1,000 Hz. The target stimulus (T) intensity was 95 dB, and the frequency was 2,000 Hz. The target stimulus (T) appeared randomly and was interspersed throughout the non-target stimulus, accounting for 20% of total stimuli heard. The non-target stimulus accounted for the remaining 80% of total stimuli heard. The number of non-target stimulus was 8; the number of target stimulus was 4. All subjects had normal hearing.
Imaging Acquisition
All image acquisitions were conducted by a practiced neuroradiologist via a Philips Achieva 3.0T MRI Scanner. The subjects remained motionless and stayed awake with their eyes closed, having as little thoughts as possible. All of these requisitions were to keep the subjects in a resting state (Zang et al., 2004). Firstly, normal T1-weighted and T2-weighted images were conducted to exclude obvious abnormal structures and brain atrophy after completing positional scanning. Resting-state functional images (rs-fMRI) were then obtained by scanning participants with echo-planar imaging sequence. The parameters were set as follows: 40 axial slices, matrix = 64 × 64, thickness/skip = 3/0.75 mm, echo time (TE) = 30 ms, repetition time (TR) = 2000 ms, flip angle = 90°, field of view (FOV) = 240 × 240 mm2, in-plane resolution = 3.75 × 3.75, and scan time = 8 min 6 s. Each condition comprised 240 functional volumes.
Image Data Processing
The imaging data was preprocessed with the software packages of statistical parametric mapping (SPM81) and Data Processing & Analysis for Brain Imaging (DPABI) V4.02 running on Matlab 9.4 (The MathWorks, Inc., Natick, MA, United States). The covariates including age, sex, education level, HDRS-17 score, HAMA score and MMSE score were adjusted for in SPM8. We used the MRIcroN software convert image data in DICOM format into NIFTI format3. The first 10 volumes of fMRI images were discarded and the remaining 230 image data were performed slice timing, head motion corrections, and spatial normalization into the standard Montreal Neurological Institute (MNI) space and resampled to 3 × 3 × 3 mm3 voxels using DPABI. The participants were excluded for head motion (translation head motion larger than 1.5 mm, angular motion larger than 1.5) during the whole fMRI scan. Then the images were spatial smoothed using a Gaussian kernel of 8 mm full-width at half-maximum filter.
To reduce low frequency drift, the images were band-pass filtered (0.01 Hz < f < 0.08 Hz). To reduce the influence of increasing temperature from the MRI equipment, linear trend was removed. Finally, several nuisance variables were regressed out, including head motion parameters, signals of the brain white matter and cerebrospinal fluid. Final generated images went into DC analysis.
Voxel-Based Degree Centrality (DC) Calculation
As has been reported in previous studies about DC16, we calculate the correlation coefficients between the time series of all pairs of gray matter voxels of the brain within the gray matter mask on the preprocessed rs-fMRI data using the Resting-State fMRI Data Analysis Toolkit (REST)4. The processing of the REST toolbox included the following procedures: (1) Import the preprocessed data through the button of input Data Directory; (2) Select Default Mask in the Mask option; (3) Fill Pearson’s correlation coefficient in the blank box of r(correlation) threshold as 0.259; (4) Select the button of z-transform:subtract the mean and divide by the STD within the mask; and (5) Click the button of Run to perform DC calculation. We statistically analyzed the mean Z-score DC maps.
Statistical Analysis
To examine the differences of DC value between the PD patients and health groups, we performed two sample t-test on the individual DC maps using DPABI V4.0. The resulting statistical map was set to uncorrected p < 0.001 and a minimum cluster size of 22 voxels (594 mm3), combined with a corrected threshold of p < 0.05. This was confirmed by using Monte Carlo simulations that were implemented in AlphaSim of AFNI5. We extracted the mean DC values of the clusters using the software REST V1.8.
Analyses of demographic and clinical data were done using the software SPSS 17.0 (SPSS Inc., Chicago, IL, United States). We used the method of two sample t-test to compare demographic and clinical data between the two groups. To detect the relationship between DC values and the clinical data of PD patients, we used Pearson correlation analysis.
Results
Demographics and Clinical Data
The demographics and clinical data are showed in Table 1. There were no significant differences in age, sex, education, HAMA score, HDRS score, and MMSE score between controls group and PD patients (all p > 0.05). The MoCA score and the P300 amplitudes of PD patients were significantly lower than those of controls group (pBonferroni correction < 0.0001), while the P300 latency of PD patients was significantly higher than that of controls group (pBonferroni correction < 0.0001).
Voxel-Wise Degree Centrality Value of Parkinson’s Disease Patients and Healthy Controls
As shown in Table 2, compared with the controls group, PD patients had higher DC values in the left medial frontal gyrus (MFG), right inferior frontal gyrus (IFG) and left precentral gyrus (PreCG) (all pBonferroni correction < 0.0001). PD patients had lower DC values than HC in the left inferior parietal lobule (IPL), left inferior temporal gyrus (ITG), right occipital lobe and right postcentral gyrus (PoCG) (all pBonferroni correction < 0.0001). The brain regions with abnormal DC value in PD patients were shown in Figure 1.
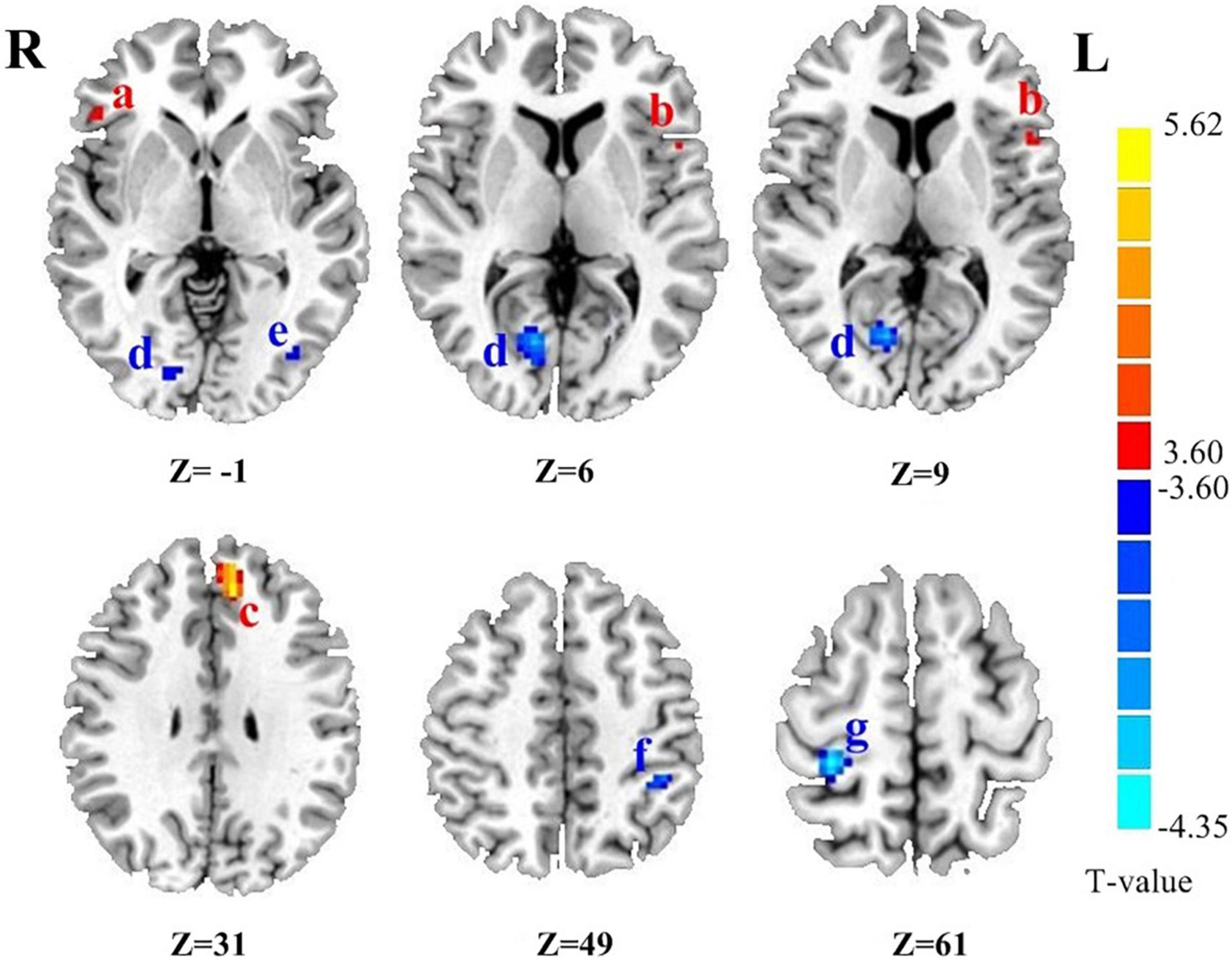
Figure 1. The brain regions with abnormal DC value in PD patients. (a) Right inferior frontal gyrus. (b) Left precentral gyrus. (c) Left medial frontal gyrus. (d) Right occipital lobe. (e) Left inferior temporal lobe gyrus. (f) Left inferior parietal lobule. (g) Right postcentral gyrus. Z is the coordinates of the Montreal Neurological Institute. The color scale indicates the magnitudes of T-values.
Correlation Analysis Results
There were negatively association between the DC values of left MFG and P300 latency (r = −0.828, pBonferroni correction < 0.0001), and there were positively association between the DC values of left MFG and P300 amplitudes (r = 0.838, pBonferroni correction < 0.0001) and MoCA scores (r = 0.855, pBonferroni correction < 0.0001) in ESDN PD patients (Figures 2A–C). No significant correlations between the DC value of left MFG and P300 latency (r = −0.095, pBonferroni correction = 0.497), P300 amplitudes (r = −0.029, pBonferroni correction = 0.834) and MoCA scores (r = −0.119, pBonferroni correction = 0.397) in HC were found (Figures 2E–G). Figure 2D showed the abnormal DC value in left MFG of the brain.
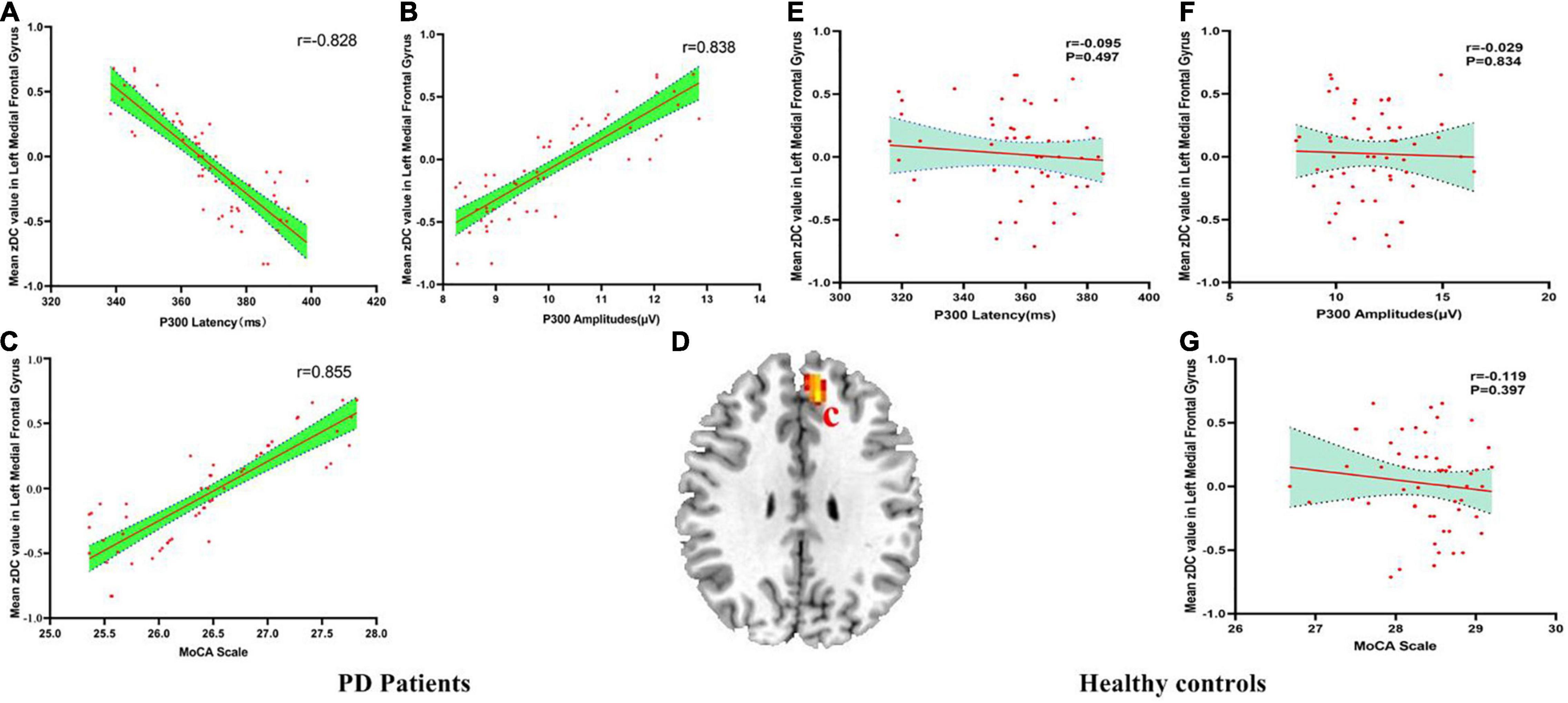
Figure 2. (A–G) The correlation results between mean zDC value in left medial frontal gyrus and P300 latency, P300 amplitudes and MoCA scale in PD patients and healthy controls. DC, Degree Centrality. The brain region in the red Alphabet c was left medial frontal gyrus.
There were negatively association between the DC values of right occipital lobe and P300 latency (r = −0.637, pBonferroni correction < 0.0001), and there were positively association between the DC values of right occipital lobe and P300 amplitudes (r = 0.575, pBonferroni correction < 0.0001) and MoCA scores (r = 0.671, pBonferroni correction < 0.0001) in PD patients (Figures 3A–C). No significant correlations between the DC value of right occipital lobe and P300 latency (r = −0.032, pBonferroni correction = 0.820), P300 amplitudes (r = −0.020, pBonferroni correction = 0.889) and MoCA scores (r = −0.193, pBonferroni correction = 0.166) in HC were found (Figures 3E–G). Figure 3D showed the abnormal DC value in right occipital lobe of the brain.
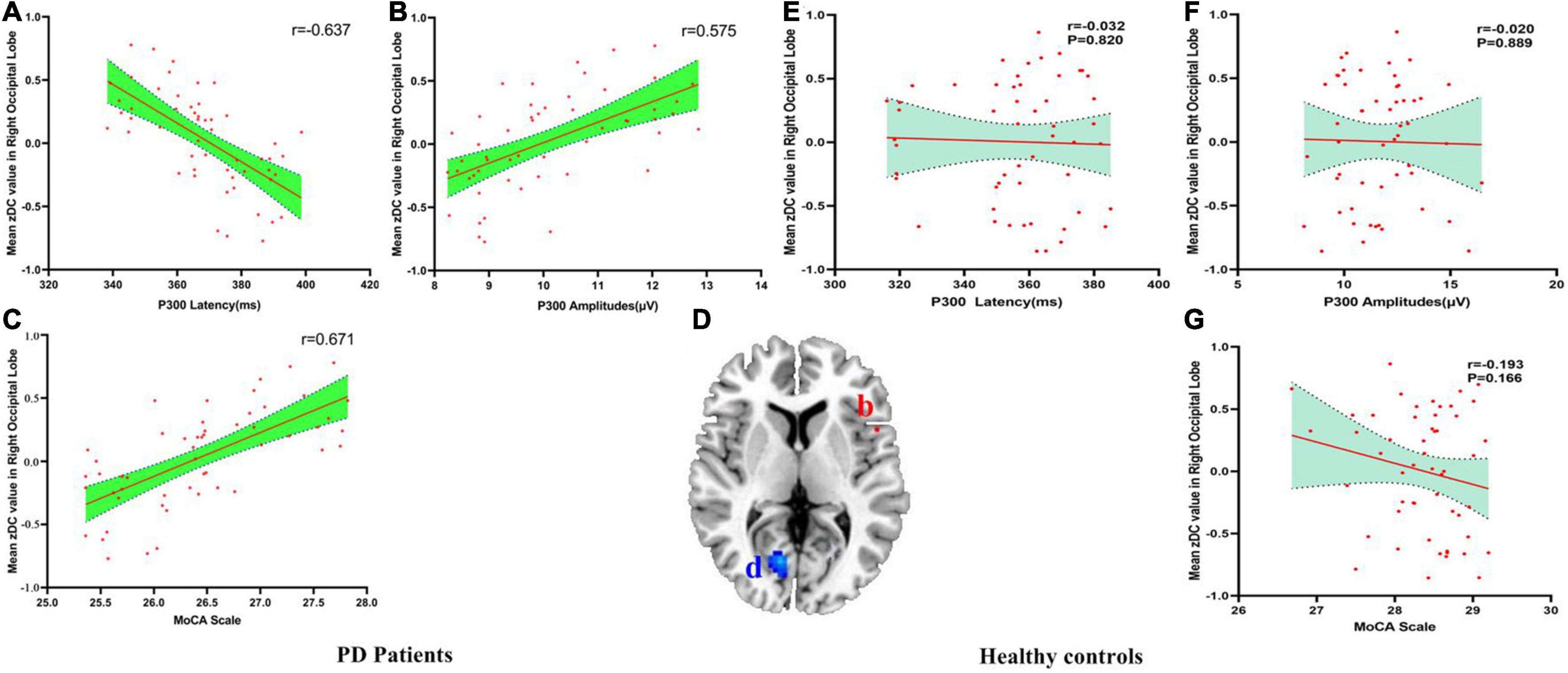
Figure 3. (A–G) The correlation results between mean zDC value in right occipital lobe and P300 latency, P300 amplitudes and MoCA scale in PD patients and healthy controls. DC, Degree Centrality. The brain region in the red Alphabet b was left precentral gyrus. The brain region in the blue Alphabet d was right occipital lobe.
There were negatively association between the DC values of right PoCG and P300 latency (r = −0.613, pBonferroni correction < 0.0001), and there were positively association between the DC values of right PoCG and P300 amplitudes (r = 0.560, pBonferroni correction < 0.0001) and MoCA scores (r = 0.618, pBonferroni correction < 0.0001) in PD patients (Figures 4A–C). No significant correlations between the DC value of right PoCG and P300 latency (r = −0.139, pBonferroni correction = 0.322), P300 amplitudes (r = 0.069, pBonferroni correction = 0.621) and MoCA scores (r = −0.086, pBonferroni correction = 0.538) in HC were found (Figures 4E–G). Figure 4D showed the abnormal DC value in right PoCG of the brain.
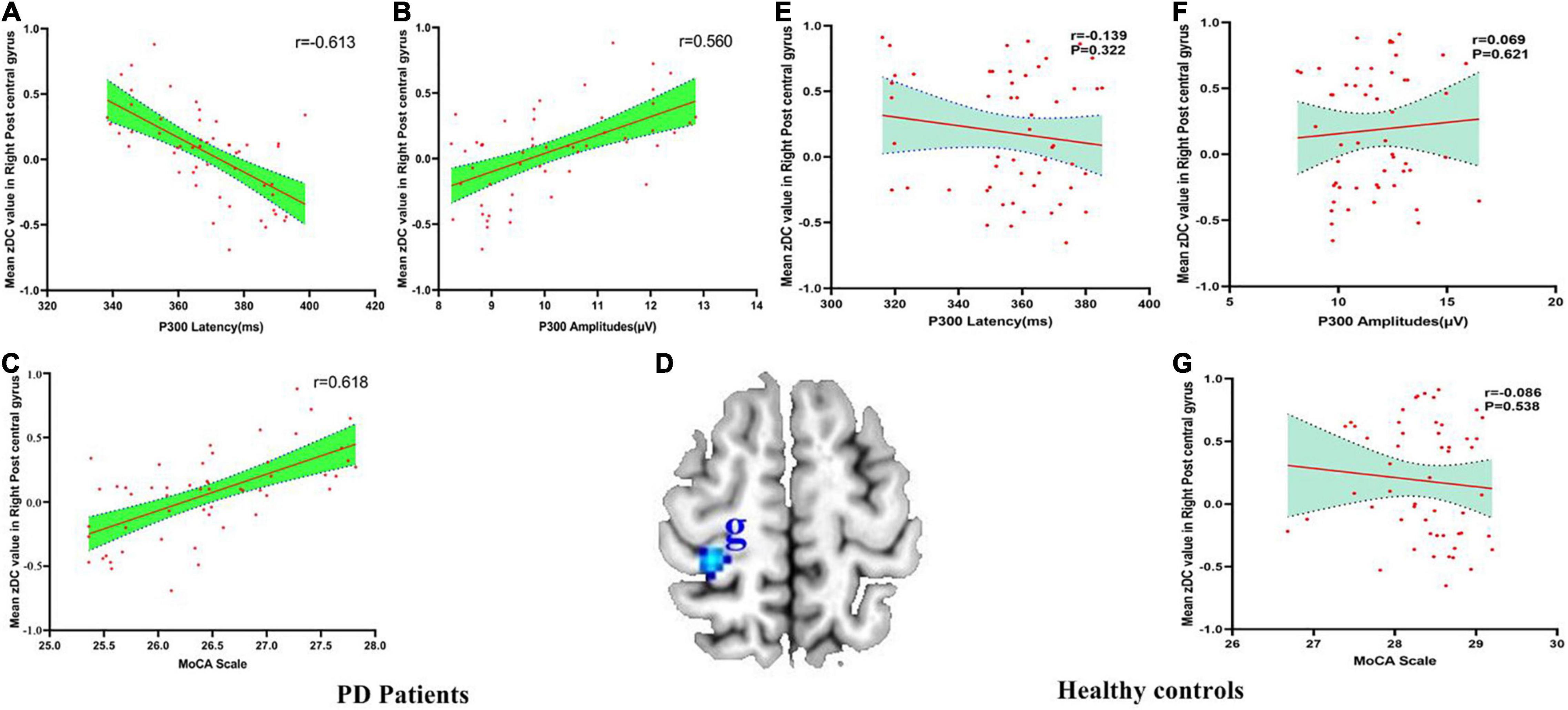
Figure 4. (A–G) The correlation between mean zDC value in the right postcentral gyrus and P300 latency, P300 amplitudes and MoCA scale in PD patients and healthy controls. DC, Degree Centrality. The brain region in the blue Alphabet g was right postcentral gyrus.
There were no associations between the DC values of the right IFG, left PreCG, left IPL, left ITG and all the clinical characteristics including age, disease course, education, onset age, HDRS score, HAMA score, MMSE score, UPDRS-III score, and H&Y score (all p > 0.05).
Discussion
The main results of this study were as follows: (1) MoCA score and P300 amplitude decreased and latency prolonged in PD patients compared with HC; (2) PD patients had higher DC values in the left MFG, right IFG, and left PreCG, but lower DC values in the left IPL, left ITG, right occipital lobe, and right PoCG compared to HC; and (3) the DC values of left MFG, right PoCG, and right occipital lobe were negatively associated with P300 latency, but positively associated with P300 amplitudes and MoCA scores in PD patients.
Our results showed that ESDN PD patients had lower cognitive performance than HC, which suggests that early stage PD patients who have never been exposed to medicine already have cognitive deficit. Furthermore, ESDN PD patients displayed decreased P300 amplitude and prolonged P300 latency, which indicates a reduced ability to understand, analyze, and respond to extrinsic information. These electrophysiological data revealed the cognitive deficit in ESDN PD patients.
The DMN is the core of the whole brain network in resting state, involving future imagination, episodic memory, emotional regulation, executive function, and other cognitive abilities (Raichle et al., 2001; He et al., 2014). Studies have found that the DMN was involved in the neural mechanism underlying cognitive deficit in PD patients (Hou et al., 2017). The greater the DC value, the more complete and faster the brain network connection and the more effective information resource utilization and information transmission (Zuo et al., 2012). In this study, the frontal lobe, which is the core region of the DMN, had brain regions with increased DC. It is well established that the frontal lobe is an important locus of mental activity as it is closely related to cognitive function, emotion, and thinking. Among the brain regions with decreased DC, the IPL, ITG and occipital lobe also belong to the DMN. Previous studies have shown lower FCs of the DMN in PD patients, which was associated with cognitive dysfunction of PD patients (Baggio et al., 2014, 2015; Hou et al., 2017). Regarding the pathogenesis of cognitive dysfunction in PD patients, some researchers believe that patients with abnormal function of the frontal-striatum displayed dysfunction in attention, memory, executive function, and speech fluency. Patients with posterior cortical deficit displayed visual, spatial, and memory abnormalities, such as naming and repetition (Jellinger, 2010; Kehagia et al., 2010). Thus, the Dual Syndrome Hypothesis about cognitive deficit in PD came into nascence: the dysfunction of the frontal-striatum dopaminergic system is associated with executive function deficit, whereas the dysfunction of the occipital, parietal, and temporal lobes’ cholinergic system is associated with the deficit of visuospatial function, memory, and attention (Kehagia et al., 2013). In this study, we found increased DC in the frontal lobe and decreased DC in the posterior cortex, both of which are consistent with previous studies and hypothesis (Jellinger, 2010; Kehagia et al., 2010; Baggio et al., 2014, 2015; Hou et al., 2017).
The MFG and IFG are key nodes in emotional regulation and cognitive control circuits (Roberts et al., 2017). The dysfunction of these brain regions already existed before significant changes in cognitive function, which resulted in the functional change of the DMN (Hou et al., 2017). Previous evidence also showed that the FC between the MFG, IFG, and other brain regions of the whole brain were weakened in PD patients (Olde Dubbelink et al., 2014). The PreCG is the main motor cortex in the brain and is responsible for the initiation and execution of movement. When performing the same motor task, PD patients needed to invest more neural activity in the PreCG to complete the nerve impulse of motor execution, which indicated that PD patients had dysfunction in the PreCG (Haslinger et al., 2001). Furthermore, the dysfunction in the PreCG and prefrontal cortex cause an absence of active movement and thinking in PD patients (Caproni et al., 2013). The non-motor symptoms of PD patients such as depression, cognitive dysfunction, and executive dysfunction may be associated with dysfunction of the PreCG and prefrontal cortex (Caproni et al., 2013). Therefore, the PreCG is thought to playa critical role in the executive ability of PD patients. Dysfunction of the PreCG will lead to difficulty with motor thoughts, motor execution, and task initiation (Caproni et al., 2013; Hou et al., 2017).
Some reports also demonstrated that PD patients with cognitive deficit had decreased gray matter volume (GMV) in the bilateral frontotemporal region, MFG, IFG, and cingulate gyrus (Sang et al., 2014). The loss of GMV in these brain regions was associated with cognitive deficit (Sang et al., 2014). This could be explained by a decrease of GMV leading to a decrease of FC and efficiency of brain network connection in PD with cognitive deficit (Baggio et al., 2014, 2015). However, contrary to previous reports, our results showed an increased DC in the frontal lobe region. Since all patients in this study were ESDN PD patients, they may have a compensatory response to the dysfunction of DMN and frontal-striatum in the brain, which sustains their executive function, emotional control, working memory, and other cognitive functions in a transient state of balance. So we have a hypothesis that the compensatory mechanisms exist in certain brain regions in ESDN PD patients. The increased DC value in the frontal lobe further indicates that the frontal lobe plays a key role in the network of cognitive function in PD patients with cognitive deficit. Therefore, increased DC in the frontal lobe may be a characteristic imaging marker of PD patients with cognitive deficit. However, further longitudinal research is needed to clarify this hypothesis.
The IPL is an integral part of the DMN, relating to pain perception, touch, taste, cognition, and spatial sensory processing. Neuroimaging studies have shown that the IPL was involved in a wide range of behavioral and sensory cognitive activities (Igelstrom and Graziano, 2017). The structural MRI study revealed that early onset PD patients had a wide range of GMV loss in the frontal, parietal, and temporal lobes, as well as decreased intensity of neural activity in these brain regions (Sang et al., 2014). Yang found that there was increased regional homogeneity (ReHo) in parietal lobules, but decreased ReHo in the PoCG in the early stages of PD, which suggests that increased ReHo was the compensatory response to the decreased functional activity of the PoCG (Yang et al., 2013). Other studies reported that the mean amplitude of low-frequency fluctuations (mALFF) of PD patients was significantly increased in the bilateral prefrontal lobe, parietal lobe, and lateral cortex of the temporal lobe (Liu et al., 2009). Our study showed a decreased DC in the left IPL, which is consistent with the developmental trend of cognitive deficit in PD patients. However, the subtype of cognitive deficit may be different from that of the selected patients in previous studies, resulting in different brain regions for compensation (Mallol et al., 2007).
The PoCG is an important sensory brain region that is mainly responsible for integrating various somatosensory stimuli information to achieve correct recognition of objects and external stimuli. It also accepts expressive projections that are closely related to emotional expression and social cooperation. Our study found decreased DC in the PoCG, suggesting a decrease in the ability to transmit information in the whole brain network, which was consistent with previous reports. For example, previous studies have found that the FC between the right PoCG, right PreCG, right precuneus, and the putamen were decreased in early onset PD patients without medication. These decreased FC were then associated with cognitive dysfunction (Liu et al., 2009; Sang et al., 2014). A meta-analysis emphasized that the left PoCG plays a critical role in non-motor symptoms of PD, and it may become a potential target for clinical intervention (Ji et al., 2018).
In this study, we found decreased DC in the right occipital lobe and left ITG, suggesting that PD patients already had visual-related cognitive dysfunction and episodic memory disorders. Previous evidence has shown that the right occipital lobe is related to spatial recognition of visual symbols, integration of visual information, and perception (Ramirez-Ruiz et al., 2007). The occipital lobe and lingual gyrus are involved in visual recognition, language, movement, abstract concepts, and consolidation of episodic memory (Kukolja et al., 2016). The increased ReHo value in the occipital lobe and temporal lobe in PD patients with sleep behavior disorder indicated the enhanced coordination and consistency of the neural activities in these two brain regions (Wang et al., 2010). A PET study found decreased metabolism in the occipital and temporal lobes in early-onset PD patients (Wu et al., 2014). These results suggest that there may be a synergistic effect between the occipital and temporal lobes in visual information processing in PD patients, which may be related to the termination of the visual radiation located in the temporal lobe. Some studies have also found that the reduction of FC in the occipital and parietal lobes was a key pathophysiological factor for the cognitive decline of PD patients with cognitive deficit (Jellinger, 2010; Baggio et al., 2014; Olde Dubbelink et al., 2014). Structural MRI studies have reported a decreased GMV, mainly in the occipital, temporal, and frontal lobes, in PD patients with cognitive deficit (Ramirez-Ruiz et al., 2007). Furthermore, PD patients with cognitive deficit have cortical atrophy in the left temporal cortex (Janvin et al., 2006). The decrease of GMV caused the decline of functional activity and efficiency of the FC in the corresponding brain regions, which lead to the decreased DC value.
In this study, we investigated the correlation between the DC values in all abnormal brain regions and clinical characteristics. We found that the DC values of the left MFG, the right PoCG, and the right occipital lobe were positively associated with MoCA scores and P300 amplitudes, but negative correlated with P300 latency. Our results indicated that the efficiency of brain FC in the frontal lobe, PoCG, and occipital lobe were associated with the cognitive function of PD patients, which was consistent with previous studies (Ramirez-Ruiz et al., 2007; Caproni et al., 2013; Yang et al., 2013; Baggio et al., 2014; Hou et al., 2017; Ji et al., 2018). We did not find a correlation between the DC values and age, education, age of onset, course of disease, HDRS score, HAMA score, MMSE score, UPDRS-III score, and H&Y score. The results in this study of ESDN PD patients indicated that the pathological characteristics of the natural disease process of PD patients impacts cognition, rather than the secondary changes caused by long-term anti-PD treatment or the neurotoxic damage to the brain caused by the chronic disease course. This correlation provides a reference and a new perspective for revealing the pathogenesis of cognitive deficit in PD patients.
There were several limitations of this study. This was a cross-sectional study, but PD is a progressive disease; thus, the dynamic changes of the DC value of these abnormal brain regions cannot be defined at present. Further longitudinal studies using multimodal neuroimaging techniques are needed to better understand the pathologic mechanisms in-depth. Further studies can also expand the sample size to explore the mechanism of brain dysfunction in patients with different subtypes and stages.
Conclusion
This study indicates that cognitive deficit and abnormal intrinsic brain functional network exists in ESDN PD patients. The damage of the DMN may be contributes to the pathogenesis of cognitive dysfunction in ESDN PD.
Data Availability Statement
The raw data supporting the conclusions of this article will be made available by the authors, without undue reservation.
Ethics Statement
The studies involving human participants were reviewed and approved by the Ethics Committee of the First Affiliated Hospital of Yangtze University. The patients/participants provided their written informed consent to participate in this study.
Author Contributions
JZ, LZ, and ZL: conception and design. JZ, DS, QT, and JH: administrative support. LZ, TY, and QT: provision of study materials or patients. LZ, TY, DS, and JH: collection and assembly of data. LZ and TY: data analysis and interpretation. ZL and YC: literatures collection and data clean. JZ, LZ, TY, ZL, and DZ: manuscript writing. All authors final approval of manuscript.
Funding
This work was supported by the National Natural Science Foundation of China (Grant Number 81771151).
Conflict of Interest
The authors declare that the research was conducted in the absence of any commercial or financial relationships that could be construed as a potential conflict of interest.
Publisher’s Note
All claims expressed in this article are solely those of the authors and do not necessarily represent those of their affiliated organizations, or those of the publisher, the editors and the reviewers. Any product that may be evaluated in this article, or claim that may be made by its manufacturer, is not guaranteed or endorsed by the publisher.
Abbreviations
DC, Degree Centrality; DMN, default mode networks; ESDN, early-stage drug-naive; FC, functional connectivity; HAMA, Hamilton Anxiety Rating Scale; HDRS-17, The17-Hamilton Rating Scale for Depression; IFG, inferior frontal gyrus; IPL, inferior parietal lobule; ITG, inferior temporal gyrus; mALFF, mean amplitude of low-frequency fluctuations; MFG, medial frontal gyrus; MMSE, Mini-Mental State Exam; MoCA, Montreal Cognitive Assessment; PD, Parkinson’s disease; PoCG, postcentral gyrus; PPD, Parkinson’s disease dementia; PreCG, precentral gyrus; ReHo, regional homogeneity; rs-fMRI, resting-state magnetic resonance imaging; UPDRS-III, Unified Parkinson’s Disease Rating Scale Part-III.
Footnotes
- ^ http://www.fil.ion.ucl.ac.uk/spm/software/spm8
- ^ http://rfmri.org/dpabi
- ^ http://www.mccauslandcenter.sc.edu/mricro/mricron/
- ^ http://www.restfmri.net
- ^ http://afni.nimh.nih.gov
References
Aarsland, D., Bronnick, K., Larsen, J. P., Tysnes, O. B., and Alves, G. (2009). Cognitive impairment in incident, untreated Parkinson disease: the Norwegian ParkWest study. Neurology 72, 1121–1126. doi: 10.1212/01.wnl.0000338632.00552.cb
Baggio, H. C., Sala-Llonch, R., Segura, B., Marti, M. J., Valldeoriola, F., Compta, Y., et al. (2014). Functional brain networks and cognitive deficits in Parkinson’s disease. Hum. Brain Mapp. 35, 4620–4634. doi: 10.1002/hbm.22499
Baggio, H. C., Segura, B., Sala-Llonch, R., Marti, M. J., Valldeoriola, F., Compta, Y., et al. (2015). Cognitive impairment and resting-state network connectivity in Parkinson’s disease. Hum. Brain Mapp. 36, 199–212.
Buckner, R. L., Sepulcre, J., Talukdar, T., Krienen, F. M., Liu, H., Hedden, T., et al. (2009). Cortical hubs revealed by intrinsic functional connectivity: mapping, assessment of stability, and relation to Alzheimer’s disease. J. Neurosci. 29, 1860–1873. doi: 10.1523/JNEUROSCI.5062-08.2009
Caproni, S., Muti, M., Principi, M., Ottaviano, P., Frondizi, D., Capocchi, G., et al. (2013). Complexity of motor sequences and cortical reorganization in Parkinson’s disease: a functional MRI study. PLoS One 8:e66834. doi: 10.1371/journal.pone.0066834
Frodl, T., Meisenzahl, E. M., Muller, D., Holder, J., Juckel, G., Moller, H. J., et al. (2002). P300 subcomponents and clinical symptoms in schizophrenia. Int. J. Psychophysiol. 43, 237–246. doi: 10.1016/s0167-8760(01)00182-9
Haslinger, B., Erhard, P., Kampfe, N., Boecker, H., Rummeny, E., Schwaiger, M., et al. (2001). Event-related functional magnetic resonance imaging in Parkinson’s disease before and after levodopa. Brain 124, 558–570. doi: 10.1093/brain/124.3.558
He, X., Qin, W., Liu, Y., Zhang, X., Duan, Y., Song, J., et al. (2014). Abnormal salience network in normal aging and in amnestic mild cognitive impairment and Alzheimer’s disease. Hum. Brain Mapp. 35, 3446–3464. doi: 10.1002/hbm.22414
Hely, M. A., Reid, W. G., Adena, M. A., Halliday, G. M., and Morris, J. G. (2008). The Sydney multicenter study of Parkinson’s disease: the inevitability of dementia at 20 years. Mov. Disord. 23, 837–844. doi: 10.1002/mds.21956
Hou, Y., Luo, C., Yang, J., Ou, R., Liu, W., Song, W., et al. (2017). Default-mode network connectivity in cognitively unimpaired drug-naive patients with rigidity-dominant Parkinson’s disease. J. Neurol. 264, 152–160. doi: 10.1007/s00415-016-8331-9
Igelstrom, K. M., and Graziano, M. S. A. (2017). The inferior parietal lobule and temporoparietal junction: a network perspective. Neuropsychologia 105, 70–83. doi: 10.1016/j.neuropsychologia.2017.01.001
Janvin, C. C., Larsen, J. P., Aarsland, D., and Hugdahl, K. (2006). Subtypes of mild cognitive impairment in Parkinson’s disease: progression to dementia. Mov. Disord. 21, 1343–1349. doi: 10.1002/mds.20974
Jellinger, K. A. (2010). Cognitive impairment and dementia in Parkinson’s disease. Eur. J. Neurol. 17:e64.
Ji, G. J., Hu, P., Liu, T. T., Li, Y., Chen, X., Zhu, C., et al. (2018). Functional connectivity of the corticobasal ganglia-thalamocortical network in parkinson disease: a systematic review and meta-analysis with cross-validation. Radiology 287, 973–982. doi: 10.1148/radiol.2018172183
Kehagia, A. A., Barker, R. A., and Robbins, T. W. (2010). Neuropsychological and clinical heterogeneity of cognitive impairment and dementia in patients with Parkinson’s disease. Lancet Neurol. 9, 1200–1213. doi: 10.1016/S1474-4422(10)70212-X
Kehagia, A. A., Barker, R. A., and Robbins, T. W. (2013). Cognitive impairment in Parkinson’s disease: the dual syndrome hypothesis. Neurodegener. Dis. 11, 79–92. doi: 10.1159/000341998
Kukolja, J., Goreci, D. Y., Onur, O. A., Riedl, V., and Fink, G. R. (2016). Resting-state fMRI evidence for early episodic memory consolidation: effects of age. Neurobiol. Aging 45, 197–211. doi: 10.1016/j.neurobiolaging.2016.06.004
Lebedev, A. V., Westman, E., Simmons, A., Lebedeva, A., Siepel, F. J., Pereira, J. B., et al. (2014). Large-scale resting state network correlates of cognitive impairment in Parkinson’s disease and related dopaminergic deficits. Front. Syst. Neurosci. 8:45. doi: 10.3389/fnsys.2014.00045
Liu, B., Chen, J., Liu, X., Long, Y., Chen, Z. G., and Ning-Na, L. I. (2009). Observation on brain default mode network in patients with Parkinson disease. Chin. J. Med. Imaging Technol. 25, 1156–1159.
Mallol, R., Barros-Loscertales, A., Lopez, M., Belloch, V., Parcet, M. A., and Avila, C. (2007). Compensatory cortical mechanisms in Parkinson’s disease evidenced with fMRI during the performance of pre-learned sequential movements. Brain Res. 1147, 265–271. doi: 10.1016/j.brainres.2007.02.046
Olde Dubbelink, K. T., Schoonheim, M. M., Deijen, J. B., Twisk, J. W., Barkhof, F., and Berendse, H. W. (2014). Functional connectivity and cognitive decline over 3 years in Parkinson disease. Neurology 83, 2046–2053. doi: 10.1212/WNL.0000000000001020
Owen, A. M. (2004). Cognitive dysfunction in Parkinson’s disease: the role of frontostriatal circuitry. Neuroscientist 10, 525–537. doi: 10.1177/1073858404266776
Raichle, M. E., MacLeod, A. M., Snyder, A. Z., Powers, W. J., Gusnard, D. A., and Shulman, G. L. (2001). A default mode of brain function. Proc. Natl. Acad. Sci. U.S.A. 98, 676–682.
Ramirez-Ruiz, B., Marti, M. J., Tolosa, E., Gimenez, M., Bargallo, N., Valldeoriola, F., et al. (2007). Cerebral atrophy in Parkinson’s disease patients with visual hallucinations. Eur. J. Neurol. 14, 750–756. doi: 10.1111/j.1468-1331.2007.01768.x
Rektorova, I., Krajcovicova, L., Marecek, R., and Mikl, M. (2012). Default mode network and extrastriate visual resting state network in patients with Parkinson’s disease dementia. Neurodegener. Dis. 10, 232–237. doi: 10.1159/000334765
Roberts, G., Lord, A., Frankland, A., Wright, A., Lau, P., Levy, F., et al. (2017). Functional dysconnection of the inferior frontal gyrus in young people with bipolar disorder or at genetic high risk. Biol. Psychiatry 81, 718–727. doi: 10.1016/j.biopsych.2016.08.018
Sang, W. N., Yong, H. H., Chi, W. M., Chung, E. J., Kim, E. G., Ji, K. H., et al. (2014). Analysis among cognitive profiles and gray matter volume in newly diagnosed Parkinson’s disease with mild cognitive impairment. J. Neurol. Sci. 347, 210–213. doi: 10.1016/j.jns.2014.09.049
Tinaz, S., Schendan, H. E., and Stern, C. E. (2008). Fronto-striatal deficit in Parkinson’s disease during semantic event sequencing. Neurobiol. Aging 29, 397–407. doi: 10.1016/j.neurobiolaging.2006.10.025
van Eimeren, T., Monchi, O., Ballanger, B., and Strafella, A. P. (2009). Dysfunction of the default mode network in Parkinson disease: a functional magnetic resonance imaging study. Arch. Neurol. 66, 877–883. doi: 10.1001/archneurol.2009.97
Wang, G., Wan, Y., Wang, Y., Xiao, Q., Liu, J., Ma, J. F., et al. (2010). Visual hallucinations and associated factors in Chinese patients with Parkinson’s disease: roles of RBD and visual pathway deficit. Parkinsonism Relat. Disord. 16, 695–696. doi: 10.1016/j.parkreldis.2010.08.013
Wang, H., Zhang, B., Zeng, B., Tang, Y., Zhang, T., Zhao, S., et al. (2018). Association between catechol-O-methyltransferase genetic variation and functional connectivity in patients with first-episode schizophrenia. Schizophr. Res. 199, 214–220. doi: 10.1016/j.schres.2018.04.023
Wang, J., Zuo, X., Dai, Z., Xia, M., Zhao, Z., Zhao, X., et al. (2013). Disrupted functional brain connectome in individuals at risk for Alzheimer’s disease. Biol. Psychiatry 73, 472–481. doi: 10.1016/j.biopsych.2012.03.026
Wu, P., Yu, H., Peng, S., Dauvilliers, Y., Wang, J., Ge, J., et al. (2014). Consistent abnormalities in metabolic network activity in idiopathic rapid eye movement sleep behaviour disorder. Brain 137, 3122–3128. doi: 10.1093/brain/awu290
Yang, H., Zhou, X. J., Zhang, M. M., Zheng, X. N., Zhao, Y. L., and Wang, J. (2013). Changes in spontaneous brain activity in early Parkinson’s disease. Neurosci. Lett. 549, 24–28. doi: 10.1016/j.neulet.2013.05.080
Zang, Y., Jiang, T., Lu, Y., He, Y., and Tian, L. (2004). Regional homogeneity approach to fMRI data analysis. Neuroimage 22, 394–400. doi: 10.1016/j.neuroimage.2003.12.030
Zhang, J., Wang, J., Wu, Q., Kuang, W., Huang, X., He, Y., et al. (2011). Disrupted brain connectivity networks in drug-naive, first-episode major depressive disorder. Biol. Psychiatry 70, 334–342. doi: 10.1016/j.biopsych.2011.05.018
Zhong, M., Yang, W., Huang, B., Jiang, W., Zhang, X., Liu, X., et al. (2019). Effects of levodopa therapy on voxel-based degree centrality in Parkinson’s disease. Brain Imaging Behav. 13, 1202–1219. doi: 10.1007/s11682-018-9936-7
Keywords: Degree Centrality, cognitive deficit, Parkinson’s disease, resting-state functional magnetic resonance imaging (fMRI), early-stage drug-naive
Citation: Zhang L, Yang T, Chen Y, Zheng D, Sun D, Tu Q, Huang J, Zhang J and Li Z (2022) Cognitive Deficit and Aberrant Intrinsic Brain Functional Network in Early-Stage Drug-Naive Parkinson’s Disease. Front. Neurosci. 16:725766. doi: 10.3389/fnins.2022.725766
Received: 15 June 2021; Accepted: 27 January 2022;
Published: 25 February 2022.
Edited by:
Aline Silva Miranda, Federal University of Minas Gerais, BrazilReviewed by:
Radek Marecek, Central European Institute of Technology (CEITEC), CzechiaYanqing Tang, First Affiliated Hospital of China Medical University, China
Copyright © 2022 Zhang, Yang, Chen, Zheng, Sun, Tu, Huang, Zhang and Li. This is an open-access article distributed under the terms of the Creative Commons Attribution License (CC BY). The use, distribution or reproduction in other forums is permitted, provided the original author(s) and the copyright owner(s) are credited and that the original publication in this journal is cited, in accordance with accepted academic practice. No use, distribution or reproduction is permitted which does not comply with these terms.
*Correspondence: Zezhi Li, YmlvbHBzeWNoaWF0cnlAMTI2LmNvbQ==; Junjian Zhang, eHNzc3NtQDE2My5jb20=
†These authors have contributed equally to this work