- 1Hefei National Research Center for Physical Sciences at the Microscale, and Department of Radiology, the First Affiliated Hospital of USTC, Division of Life Science and Medicine, University of Science & Technology of China, Hefei, China
- 2Department of Psychology, School of Humanities and Social Sciences, University of Science and Technology of China, Hefei, Anhui, China
- 3Division of Life Sciences and Medicine, School of Life Sciences, University of Science and Technology of China, Hefei, Anhui, China
- 4State Key Laboratory of Cognitive Neuroscience and Learning and IDG/McGovern Institute for Brain Research, Beijing Normal University, Beijing, China
- 5SILC Business School, Shanghai University, Shanghai, China
- 6Application Technology Center of Physical Therapy to Brain Disorders, Institute of Advanced Technology, University of Science and Technology of China, Hefei, China
- 7Biomedical Sciences and Health Laboratory of Anhui Province, University of Science and Technology of China, Hefei, China
Fueled by the development of neuroscience and artificial intelligence (AI), recent advances in the brain-inspired AI have manifested a tipping-point in the collaboration of the two fields. AI began with the inspiration of neuroscience, but has evolved to achieve a remarkable performance with little dependence upon neuroscience. However, in a recent collaboration, research into neurobiological explainability of AI models found that these highly accurate models may resemble the neurobiological representation of the same computational processes in the brain, although these models have been developed in the absence of such neuroscientific references. In this perspective, we review the cooperation and separation between neuroscience and AI, and emphasize on the current advance, that is, a new cooperation, the neurobiological explainability of AI. Under the intertwined development of the two fields, we propose a practical framework to evaluate the brain-likeness of AI models, paving the way for their further improvements.
Introduction
Artificial intelligence (AI) starts with the notion of creating Turing-powerful intelligent systems (Turing, 1936). He claimed that his desire was to build a machine to “imitate a brain” and also to “mimic the behavior of the human,” which means the likeness to both the brain and the behavior is requisite to realize such intelligent systems. For this to happen, pioneers in the field (Rosenblatt, 1958; Fukushima and Nixon, 1980; Bi and Poo, 1998; Masquelier and Thorpe, 2007) have drawn inspiration from the neurobiological representation to develop AI models. However, early models or algorithms strictly mimicking the neural processes in the brain have constantly failed to deliver satisfactory performances, such as the perceptron (Rosenblatt, 1958), Hebbian learning rules (Kempter et al., 1999), and Sigmoid (Han and Moraga, 1995). Gradually, computer scientists have strayed away from neuroscience and turned to engineering and mathematical solutions to design “outcome-driven” models. These models achieved remarkable performance in many aspects, including but not limited to object recognition (Riesenhuber and Poggio, 2000), speech and music recognition (Kell et al., 2018; Sutskever et al., 2019), and motor movement (Todorov, 2000).
Nonetheless, comparison between AI and the brain has never stopped. Once optimized performance is achieved, researchers (Yamins et al., 2014; Güçlü and van Gerven, 2015; Eickenberg et al., 2017; Zhuang et al., 2021) begin to search for the neurobiological explainability of these advanced models, that is, the similarity of the neurobiological representation of the same computational processes between AI models and the brain. The authors wish that through unraveling the neurobiological explainability of AI models, one could achieve a better understanding of the brain and thus promote the development of neuroscience (Lindsay, 2021). Interestingly, in return, the evaluation of resemblance between current AI models and the brain may also shed lights on how far away these models are to the Turing-powerful (i.e., brain-like) intelligent systems.
During the three stages (Figure 1) of AI development, the role of neuroscience has experienced a shift from the “guide,” who provides guiding principles to the design of AI models, to the “judge,” who provide references for the evaluation of AI models. In this review, we will look back to the mutual development of AI and neuroscience, and propose a framework to evaluate the brain-likeness of AI models that can serve AI development in multiple ways.
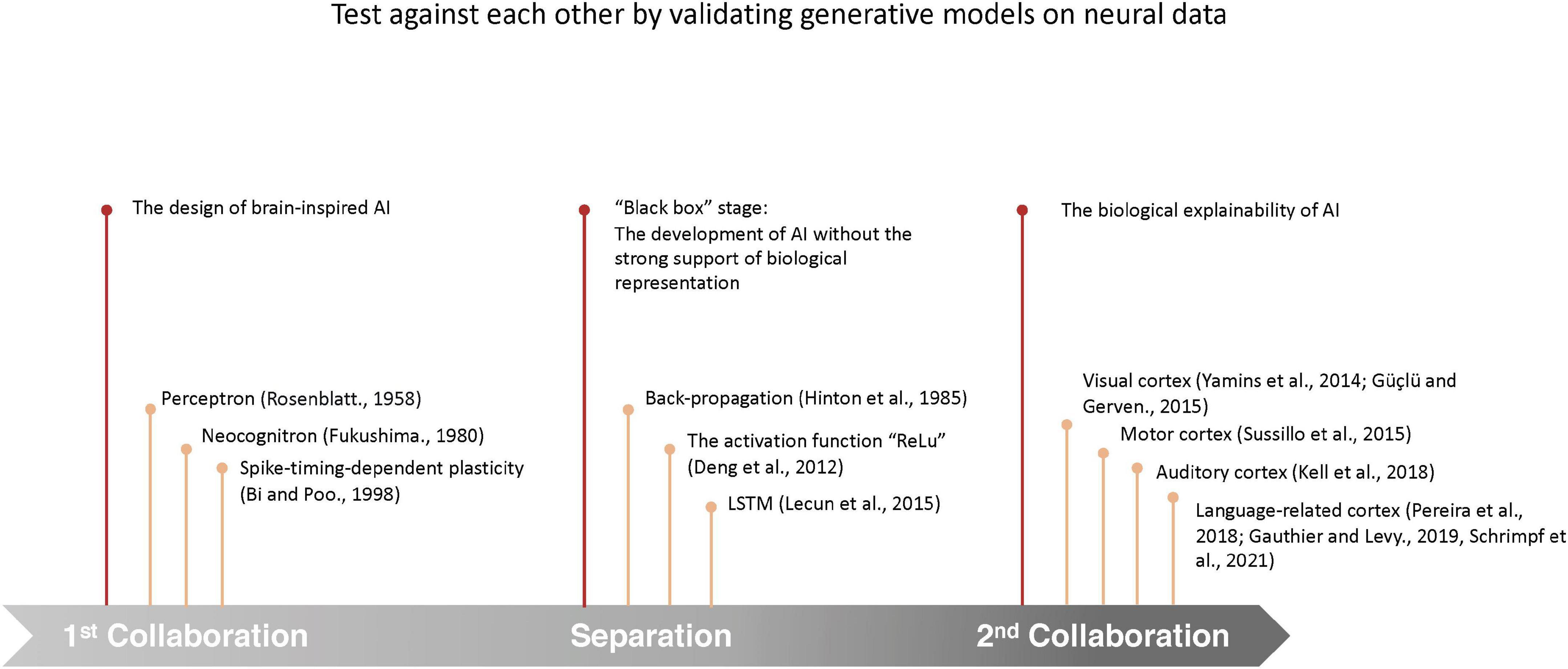
Figure 1. Timeline of the development of the interaction between neuroscience and AI. During the development, the interaction between neuroscience and AI has experienced 3 stages: (1) neuroscience guided the design of AI models; (2) implementations of engineering and mathematical tools instead of neurobiological principles in the development of AI models have led to substantial improvement in model performances, but the complex algorithms and huge parametric space behind the high performance pose a dilemma for the explanation of the underlying specific decision-making of the models, which are also called “black box” models (Arrieta et al., 2020); (3) by comparing the predicted neurobiological representation by modern AI models to the real neural processes in the brain, neurobiological explainability are provided to AI models.
The collaboration and separation of artificial intelligence and neuroscience
Brain is the most complex and efficient non-artificial intelligent system known to humans. Throughout history, the promise of creating machine intelligence with brain-like ability has been a motivation of innovation (Roy et al., 2019). One way to realize such intelligence is to scrutinize the organization principles of brain’s structures and functions and thus seek inspiration for the design of AI. Hassabis et al. (2017) stated that if a new facet of neurobiological representation were found, it would be considered as an excellent candidate for incorporation into AI. Over the years, AI models have been rapidly developed by drawing inspiration from the brain neural networks, whereas algorithms, architectures, and functions of models have benefited greatly from mimicking such neurobiological representations (e.g., neuro-synaptic framework and hierarchical structure).
In the initial collaboration between AI and neuroscience, the direct inspiration from neuroscience accelerated the start-up of AI. The earliest application was the perceptron (Rosenblatt, 1958), a simple abstract of neurons, mimicking the simple neuronal activity in visual cortex, such as the weights of synapses, the biases of the thresholds, and the activation function of the neural cells. Years later, inspired by Hubel and Wiesel’s (1962) study in the visual cortex, Fukushima and Nixon (1980) proposed an advanced model, Neocognitron, the precursor of the modern convolutional neural networks (CNN), which mimicked the organizations of neural cells in the visual cortex. Apart from the inspiration of how neurons activate, researchers also designed some brain-corresponding models (e.g., topographic maps) inspired by how brain is organized. For example, Burak and Fiete (2009) modeled the network topology of the rats entorhinal cortex to form the neural substrate for dead-reckoning.
Although AI is profoundly inspired by the neurobiological representation of the brain, surprisingly, these brain-mimicking models have never achieved a satisfactory performance, likely due to their over-simplification of the real neural system. For instance, Hebbian learning, a neurobiologically schemed method, fail to produce models with adequate performance as it does not take into consideration of the synapse’s downstream effect on the network output (Lillicrap et al., 2020). Gradually, researchers (Rumelhart et al., 1986; Hinton et al., 2012; Lecun et al., 2015) started to turn to engineering and mathematical solutions to maximize model performance regardless of its underlying neurobiological relevance. In these works, the authors replaced the former neurobiological schemed methods with back-propagation, an algorithm without a prior neurobiological relevance, and solved the low-efficiency problem of synaptic modification (Lillicrap et al., 2020). Moreover, replacing the former neurobiologically inspired Sigmoid function (Han and Moraga, 1995) with the activation function ReLu (Deng et al., 2010) has been demonstrated to substantially improve the performance of deep neural networks (DNNs) since Krizhevsky et al. (2012). Given such superior performances, are these models operated in anyway similar to the most efficient system we ever know, the brain, despite they are not strictly structured to follow any neurobiological principles?
Neurobiological explainability of artificial intelligence
Despite of the turning of design principles from mimicking neurobiological representation of the brain to optimizing performance with tools from engineering and mathematics, AI and neuroscience have never really grown apart. With the rapid development of AI, researchers (Yamins et al., 2014; Güçlü and van Gerven, 2015; Eickenberg et al., 2017; Zhuang et al., 2021) believe that these advanced models are capable to promote the development of neuroscience in return. In specific, they advocate for seeking for the neurobiological explanations for AI models as an alternative way to better understand the organization principles of the brain.
Early studies exploring the neurobiological explainability of AI models have mainly focused on visual recognition. Yamins et al. (2014) first examined the similarity between real brain activities and predicted activations from CNN model. The authors trained CNN model to match human performances on various visual recognition tasks. The results showed that the third and the fourth (top) layer of the model could effectively predict the inferior temporal activity recorded with functional MRI during image recognition. Other findings (Cadieu et al., 2014; Khaligh-Razavi and Kriegeskorte, 2014; Güçlü and van Gerven, 2015) also confirmed that deep neural network (DNN) models trained for visual recognition have remarkable predictability for the neural responses in the human visual system as well. Moreover, Cichy et al. (2016) found that the predicted brain activities by DNN trained for object categorization are highly resemble to the brain activations recorded via both fMRI and MEG during the same cognitive process, not only in the physical space domain (i.e., matching the hierarchical topography in the human ventral and dorsal visual streams), but also in the temporal domain (i.e., matching the time course over visual processing).
In addition to visual recognition, models designed for other utilities also showed similar predicted neurobiological representations with the real activities in the corresponding neural systems. A recent heavily focused area is the neurobiological explainability of AI models for language processing, including syntax processing (Gauthier and Levy, 2019), semantic processing (Pereira et al., 2016; de Heer et al., 2017), and comprehension (Schrimpf et al., 2021). Adding to these evidence, highly corresponded mappings between predicted (by the AI) and recorded (in the brain) neurobiological representations have also been found in other cognitive systems, such as the auditory system (Kell et al., 2018), the motor system (Sussillo et al., 2015), and even the hippocampal formation (Whittington et al., 2021).
Quantify the progress toward Turing-powerful intelligence
Such demonstration of neurobiological explainability of AI models has opened the door for new contributions from neuroscience, to provide alternative tools to quantitatively evaluate the progress we made toward the Turing-powerful intelligent systems. Normally, evaluation to the distance to such intelligent systems concentrates at the behavioral level, where the performance of models would be evaluated, such as model-model comparison and model-to-human behavior comparison. However, the neurobiological explainability gives us cues to evaluate the brain-likeness of the models, which mainly focused on whether they can solve the same problems as the brain. Evaluation at the both behavioral and neurobiological gives us a more comprehensive insight to evaluate the distance to the Turing-powerful intelligent systems. Besides, the improvements of the algorithm can also indicate the advancement toward the Turing-powerful intelligent systems. To further elaborate on this new role of neuroscience in AI development, here, we capitalize on the Marr’s (1982) widely recognized computational framework, and discuss such applications in three levels.
Evaluation at the computational level
In Marr’s theory, the first level, computational level, concerns the problems that models can solve. The evaluation of the performance of the models can be categorized into two ways: model-to-model comparison and model-to-human behavior comparison. The model-to-model comparison literally compares performances of different models for the same task. For instance, Xu et al. (2021) compared supervised models to unsupervised models and found that the latter trained with 10 min of labeled data, could rival the best supervised model trained with 960 h of labeled data. The model-to-human behavior comparison contrasts AI performance to human performance during the same task. For instance, Rajalingham et al. (2018) compared the ANNs’ (Artificial neural networks) accuracy in the visual categorization task with the behavioral results from 1,477 primates (1,472 humans and 5 monkeys), and evaluated that the models could not achieve the human-like behavioral performance.
Evaluation at the algorithmic level
The second level of Marr’s theory, algorithmic level, concerns the processes that models go through. During the exploration into the neurobiological explainability of models, the training methods for models displayed a positive shift, implying that models turned out to be more intelligent. First, the training methods for models in the earlier studies aimed to map the computational models into the corresponding brain activity (Mitchell et al., 2008) when receiving the same stimuli, or to use the brain responses to constrain the models (Cadieu et al., 2014). And then the artificial neural response generated from models and the unlearned brain data were compared. However, in more recent studies, researchers (Yamins et al., 2014; Kell et al., 2018; Schrimpf et al., 2021) start to train AI models with only behavioral (e.g., objects and their labels) but not any neuroimaging data. Interestingly, while the models were not optimized to fit brain signals in the first places, they can still predict the brain responses during the same cognitive process proficiently. These findings suggest that the computational processes of these models can be brain-like enough to generate neurobiological representation without explicit training.
Furthermore, the shift of paradigm from supervised to unsupervised models during the prediction of neurobiological representation can also be seen as a step-forward toward brain-like intelligence, since the latter is considered to be more similar to human learning pattern which is constantly exposed to unlabeled environments (Mitchell, 2004), which could even automatically learn the human bias from image classification (Steed and Caliskan, 2021). In earlier studies, models used to predict neurobiological representation were mostly supervised models (Cadieu et al., 2014; Yamins et al., 2014; Güçlü and van Gerven, 2015). A study even suggested that unsupervised models could not predict the brain responses (Khaligh-Razavi and Kriegeskorte, 2014). However, with the improvement of unsupervised models during the decade (Xu et al., 2021), recent studies have found evidence that unsupervised models could successfully predict the neural response as well. For instance, Zhuang et al. (2021) found that the unsupervised models achieved a high prediction accuracy in the primate ventral stream that equaled and even surpassed the performance of the best supervised model. Thus, the recent success of unsupervised models in predicting brain representation suggests that AI models have made a giant step forward on the human-like path.
Evaluation at the implementation/physical level
The last level of Marr’s theory, implementation/physical level, concerns the brain-likeness of the models.
First, instantiation (i.e., the neural representation of models) of the brain-inspired model would be an explicit and effective measure for judging success and spurring the progress to the Turing-powerful intelligence. It is an explicit measure since the layers in the models almost correspond to the hierarchical structure of the brain (Kell et al., 2018), where we can directly compared the detailed performance in each layer with the corresponding responses in the brain. If a model could highly predict the response in the brain, we consider that its corresponding parameters/weight would be vital to achieve the Turing-powerful intelligent systems (Hassabis et al., 2017). It is also an effective measure to drive models toward the goal. For example, Yamins et al. (2014) found that the top layer in the model could better predict the activity in IT cortex while other layers did not achieve the satisfactory performance. In this case, we may allocate more energy to optimize the layers that cannot successfully explain the corresponding neurobiological representation, which determines the most productive way to allocate resources.
Second, the evaluation of models from the perspective of neuroscience further supports the validation of behavioral results. Many studies have indicated that the more brain-like the model is, the better performance the model has in the task. For instance, Yamins et al. (2014) indicated that when a model highly predicts the IT (Inferior temporal) cortex, the better performance it would have in the object recognition task. And the same results were also found in another study (Khaligh-Razavi and Kriegeskorte, 2014). They compared 37 models with the human’s and monkeys’ cortex, respectively, showing that the models with more relevant correspondences with the neural representation in IT cortex have better performance in object recognition. Further, in the language model, Schrimpf et al. (2021) compared the predictability for neural response between 43 diverse language models, where they found models with high next-word predictive ability, like GPT models, have a better performance in predicting brain signals in language comprehension. Even they compared the ability of the next-word prediction of these models in another dataset, the neural predictivity still significantly correlated with the behavioral results. The parallel but highly correlated results provide us an opportunity to evaluate and further modify models from another perspective, neuroscience. Combining with the first point, it gives us a sight that we may modify the models more brain-like in order to achieve better performance.
Third, the evaluation at the behavioral level may not comprehensively explain the brain-like intelligence, as the way to process information differs in the brain and behavior (Bechara et al., 1997; Soon et al., 2008). Researchers claimed that the unconscious biases observed in the brain guided behavior before the conscious knowledge did, which means the brain signal might capture the subtle differences that were obscure at the behavioral level. Thus, the evaluation at the neurobiological level may evaluate the distance to the Turing-powerful intelligence more accurately compared to the behavioral evaluation.
Implications for the improvement of AI models
Thus far, we have reviewed the collaboration and separation between neuroscience and AI, and highlighted the significance of the current collaboration. More importantly, we propose the importance of evaluating models from the perspective of neuroscience. The evaluation tells us the closeness between the current models and the brain, which is critical to optimize models in achieving the Turing-powerful level.
To move forward, here, we present an AI-brain loop framework in which we implement the explicit evaluation from neuroscience and accurate modification in each layer and even parameters to the models (Figure 2), inspired by the human-in-the-loop (Li et al., 2014) and inception loop (Walker et al., 2019).
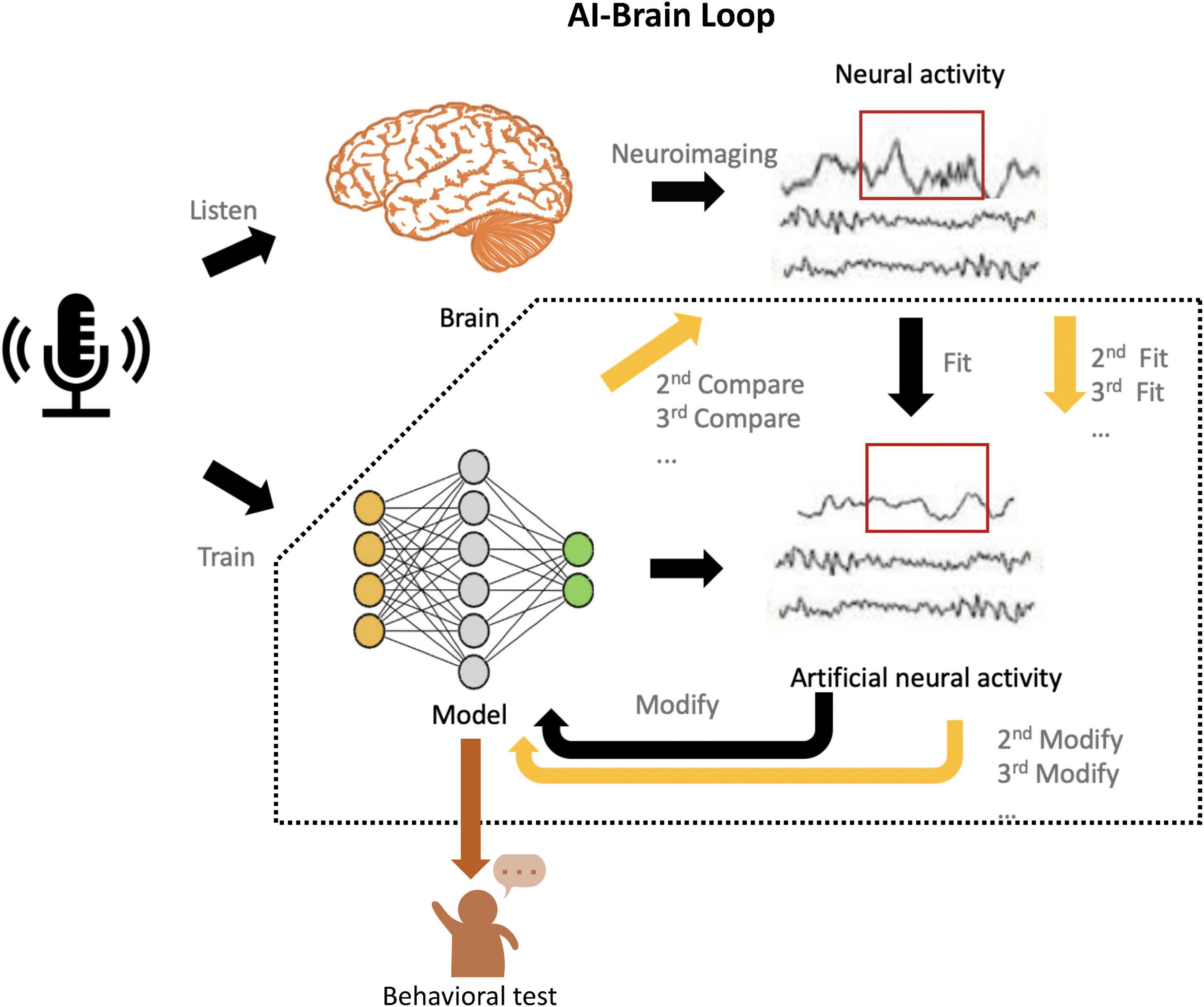
Figure 2. Schematic of the AI-Brain loop. First, present the same task (e.g., audio recognition) to human subjects and AI models, which are subsequently trained for this task. Second, record the neural activities in the brain by neuroimaging techniques (e.g., fMRI., EEG., MEG., ECoG), and predict neural responses with these trained AI models. Third, compare the recorded neural activity and the artificial neural activity generated by models. Fourth, use the artificial neural activity to fit the neural activity by modifying the corresponding layers or parameters. Fifth, implement the behavioral evaluation of model and see whether the performance achieve the human-like level. If not, implement the continuous fit until it achieves the both human-like and brain-like level.
In this framework, we propose that the AI models trained for specific behavioral task can use neural recordings during the same task as neurobiological reference. Comparisons between recorded and model-predicted neural responses can be used to tune the parameter space of the AI models, and more realistic neurobiological representation of the models can be achieved during the process of minimizing the differences between the two. Lastly, performance of the modified models at behavioral level will be used to verify that whether models with higher brain-likeness level, but also function at the human-like level. Then we also test the modified models at the behavioral level and see whether the performance improves. Such clues of the modification are fundamental to achieve the Turing-powerful intelligent system since it echoes Turing’s claim (Turing, 1936) that models are qualified in not only “mimicking the behavior of the human,” but also “imitating the brain.” The call for Turing-powerful intelligent system asks to look beyond performance optimization, but to focus more on how to achieve higher brain resemblance in future AI models. We believe that re-introducing neuroscience back into AI development through this neurobiological explainability provides a promising opportunity to outbreaking the “black-box” dilemma suffered by most of modern AI models. By “jumping out of the box” and developing more brain-like AI through such AI-brain comparisons, we may eventually leap forward to such ultimate goal.
Author contributions
YC, ZW, XH, and XZ contributed to the conception of the study. YC and ZW wrote the first draft of the manuscript. YC, ZW, and XH wrote sections of the manuscript. YC, ZW, HG, HL, LG, XH, and XZ contributed to revise the manuscript and customized the tables. All authors contributed to manuscript revision, read, and approved the submitted final versions.
Funding
This work was supported by grants from The Chinese National Programs for Brain Science and Brain-like Intelligence Technology (2021ZD0202101), The National Natural Science Foundation of China (71942003, 32161143022, 32171080, and 31900766), Major Project of Philosophy and Social Science Research, Ministry of Education of China (19JZD010), CAS-VPST Silk Road Science Fund 2021 (GLHZ202128), Collaborative Innovation Program of Hefei Science Center, CAS (2020HSC-CIP001), and Anhui Provincial Key Research and Development Project (202004b11020013).
Acknowledgments
We thank the Bioinformatics Center of the University of Science and Technology of China, School of Life Science, for providing supercomputing resources for this project.
Conflict of interest
The authors declare that the research was conducted in the absence of any commercial or financial relationships that could be construed as a potential conflict of interest.
Publisher’s note
All claims expressed in this article are solely those of the authors and do not necessarily represent those of their affiliated organizations, or those of the publisher, the editors and the reviewers. Any product that may be evaluated in this article, or claim that may be made by its manufacturer, is not guaranteed or endorsed by the publisher.
References
Arrieta, A. B., Díaz-Rodríguez, N., Del Ser, J., Bennetot, A., Tabik, S., Barbado, A., et al. (2020). Explainable artificial intelligence (XAI): Concepts, taxonomies, opportunities and challenges toward responsible AI. Inf. Fusion 58, 82–115. doi: 10.1016/j.inffus.2019.12.012
Bechara, A., Damasio, H., Tranel, D., and Damasio, A. R. (1997). Deciding advantageously before knowing the advantageous strategy. Science 275, 1293–1295. doi: 10.1126/science.275.5304.1293
Bi, G. Q., and Poo, M. M. (1998). Synaptic modifications in cultured hippocampal neurons: Dependence on spike timing, synaptic strength, and postsynaptic cell type. J. Neurosci. 18, 10464–10472. doi: 10.1523/JNEUROSCI.18-24-10464.1998
Burak, Y., and Fiete, I. R. (2009). Accurate path integration in continuous attractor network models of grid cells. PLoS Comput. Biol. 5:e1000291. doi: 10.1371/journal.pcbi.1000291
Cadieu, C. F., Hong, H., Yamins, D. L. K., Pinto, N., Ardila, D., Solomon, E. A., et al. (2014). Deep neural networks ral the representation of primate IT cortex for core visual object recognition. PLoS Comput. Biol. 10:e1003963. doi: 10.1371/JOURNAL.PCBI.1003963
Cichy, R. M., Khosla, A., Pantazis, D., Torralba, A., and Ola, A. (2016). Comparison of deep neural networks to spatio-temporal cortical dynamics of human visual object recognition reveals hierarchical correspondence. Sci. Rep. 6:27755. doi: 10.1038/srep27755
de Heer, W. A., Huth, A. G., Griffiths, T. L., Gallant, J. L., and Theunissen, F. E. (2017). The hierarchical cortical organization of human speech processing. J. Neurosci. 37, 6539–6557. doi: 10.1523/JNEUROSCI.3267-16.2017
Deng, J., Dong, W., Socher, R., Li, L.-J., Li, K., and Fei-Fei, L. (2010). “ImageNet: A large-scale hierarchical image database,” in Proceedings of the 2009 IEEE conference on computer vision and pattern recognition, (Miami, FL: IEEE), 248–255. doi: 10.1109/CVPR.2009.5206848
Eickenberg, M., Gramfort, A., Varoquaux, G., and Thirion, B. (2017). Seeing it all: Convolutional network layers map the function of the human visual system. Neuroimage 152, 184–194. doi: 10.1016/J.NEUROIMAGE.2016.10.001
Fukushima, T., and Nixon, J. C. (1980). Analysis of reduced forms of biopterin in biological tissues and fluids. Anal. Biochem. 102, 176–188. doi: 10.1016/0003-2697(80)90336-X
Gauthier, J., and Levy, R. P. (2019). “Linking artificial and human neural representations of language,” in Proceedings of the 2019 conference on empirical methods in natural language processing and the 9th international joint conference on natural language processing (EMNLP-IJCNLP), (Hong Kong: Association for Computational Linguistics), 529–539. doi: 10.18653/V1/D19-1050
Güçlü, U., and van Gerven, M. A. J. (2015). Deep neural networks reveal a gradient in the complexity of neural representations across the ventral stream. J. Neurosci. 35, 10005–10014. doi: 10.1523/JNEUROSCI.5023-14.2015
Han, J., and Moraga, C. (1995). The influence of the sigmoid function parameters on the speed of backpropagation learning. Lect. Notes Comput. Sci. 930, 195–201. doi: 10.1007/3-540-59497-3_175/COVER
Hassabis, D., Kumaran, D., Summerfield, C., and Botvinick, M. (2017). Neuroscience-inspired artificial intelligence. Neuron 95, 245–258. doi: 10.1016/j.neuron.2017.06.011
Hinton, G. E., Srastava, N., Krizhevsky, A., Sutskever, I., and Salakhutdinov, R. R. (2012). Improving neural networks by preventing co-adaptation of feature detectors. arX [Preprint] doi: 10.48550/arx.1207.0580
Hubel, D. H., and Wiesel, T. N. (1962). Recepte fields, binocular interaction and functional architecture in the cat’s visual cortex. J. Physiol. 160, 106–154. doi: 10.1113/JPHYSIOL.1962.SP006837
Kell, A. J. E., Yamins, D. L. K., Shook, E. N., Norman-Haignere, S. V., and McDermott, J. H. (2018). A task-optimized neural network replicates human auditory behavior, predicts brain responses, and reveals a cortical processing hierarchy. Neuron 98, 630–644.e16. doi: 10.1016/J.NEURON.2018.03.044
Kempter, R., Gerstner, W., and van Hemmen, J. L. (1999). Hebbian learning and spiking neurons. Phys. Rev. E 59:4498. doi: 10.1103/PhysRevE.59.4498
Khaligh-Razavi, S. M., and Kriegeskorte, N. (2014). Deep supervised, but not unsupervised, models may explain IT cortical representation. PLoS Comput. Biol. 10:e1003915. doi: 10.1371/JOURNAL.PCBI.1003915
Krizhevsky, A., Sutskever, I., and Hinton, G. E. (2012). ImageNet classification with deep convolutional neural networks. Adv. Neural Inf. Process. Syst. 25, 1097–1105.
Lecun, Y., Bengio, Y., and Hinton, G. (2015). Deep learning. Nature 521, 436–444. doi: 10.1038/nature14539
Li, W., Sadigh, D., Sastry, S. S., and Seshia, S. A. (2014). Synthesis for human-in-the-loop control systems. Lect. Notes Comput. Sci. 8413, 470–484. doi: 10.1007/978-3-642-54862-8_40/COVER
Lillicrap, T. P., Santoro, A., Marris, L., Akerman, C. J., and Hinton, G. (2020). Backpropagation and the brain. Nat. Rev. Neurosci. 21, 335–346. doi: 10.1038/s41583-020-0277-3
Lindsay, G. W. (2021). Convolutional neural networks as a model of the visual system: Past, present, and future. J. Cogn. Neurosci. 33, 2017–2031. doi: 10.1162/JOCN_A_01544
Marr, D. (1982). Vision: A computational investigation into the human representation and processing of visual information. New York, NY: W.H. Freeman and Company.
Masquelier, T., and Thorpe, S. J. (2007). Unsupervised learning of visual features through spike timing dependent plasticity. PLoS Comput. Biol. 3:e31. doi: 10.1371/journal.pcbi.0030031
Mitchell, T. M. (2004). “The role of unlabeled data in supervised learning,” in Language, knowledge, and representation, Vol. 99, eds J. M. Larrazabal and L. A. P. Miranda (Dordrecht: Springer), 103–111. doi: 10.1007/978-1-4020-2783-3_7
Mitchell, T. M., Shinkareva, S. V., Carlson, A., Chang, K. M., Malave, V. L., Mason, R. A., et al. (2008). Predicting human brain actity associated with the meanings of nouns. Science 320, 1191–1195. doi: 10.1126/science.1152876
Pereira, F., Gershman, S., Ritter, S., and Botvinick, M. (2016). A comparate evaluation of off-the-shelf distributed semantic representations for modelling behavioural data. Cogn. Neuropsychol. 33, 175–190. doi: 10.1080/02643294.2016.1176907
Rajalingham, R., Issa, E. B., Bashan, P., Kar, K., Schmidt, K., and DiCarlo, J. J. (2018). Large-scale, high-resolution comparison of the core visual object recognition behavior of humans, monkeys, and state-of-the-art deep artificial neural networks. J. Neurosci. 38, 7255–7269. doi: 10.1523/JNEUROSCI.0388-18.2018
Riesenhuber, M., and Poggio, T. (2000). Models of object recognition. Nat. Neurosci. 3, 1199–1204. doi: 10.1038/81479
Rosenblatt, F. (1958). The perceptron: A probabilistic model for information storage and organization in the brain. Psychol. Rev. 65, 386–408. doi: 10.1037/H0042519
Roy, K., Jaiswal, A., and Panda, P. (2019). Towards spike-based machine intelligence with neuromorphic computing. Nature 575, 607–617. doi: 10.1038/s41586-019-1677-2
Rumelhart, D. E., Hinton, G. E., and Williams, R. J. (1986). Learning representations by back-propagating errors. Nature 323, 533–536. doi: 10.1038/323533a0
Schrimpf, M., Blank, I. A., Tuckute, G., Kauf, C., Hosseini, E. A., Kanwisher, N., et al. (2021). The neural architecture of language: Integrate modeling converges on predicte processing. Proc. Natl. Acad. Sci. U.S.A. 118:e2105646118. doi: 10.1073/pnas.2105646118
Soon, C. S., Brass, M., Heinze, H. J., and Haynes, J. D. (2008). Unconscious determinants of free decisions in the human brain. Nat. Neurosci. 11, 543–545. doi: 10.1038/nn.2112
Steed, R., and Caliskan, A. (2021). “Image representations learned with unsupervised pre-training contain human-like biases,” in Proceedings of the 2021 ACM conference on fairness, accountability, and transparency, (Toronto: IEEE), 701–713. doi: 10.1145/3442188.3445932
Sussillo, D., Churchland, M. M., Kaufman, M. T., and Shenoy, K. V. (2015). A neural network that finds a naturalistic solution for the production of muscle actity. Nat. Neurosci. 18, 1025–1033. doi: 10.1038/nn.4042
Sutskever, I., Martens, J., and Hinton, G. E. (2019). “Generating text with recurrent neural networks,” in Proceedings of the 28th international conference on international conference on machine learning, Bellevue, WA, 1017–1024.
Todorov, E. (2000). Direct cortical control of muscle actation in voluntary arm movements: A model. Nat. Neurosci. 3, 391–398. doi: 10.1038/73964
Turing, A. M. (1936). On computable numbers, with an application to the Entscheidungsproblem. J. Math. 58, 345–363.
Walker, E. Y., Sinz, F. H., Cobos, E., Muhammad, T., Froudarakis, E., Fahey, P. G., et al. (2019). Inception loops discover what excites neurons most using deep predicte models. Nat. Neurosci. 22, 2060–2065. doi: 10.1038/s41593-019-0517-x
Whittington, J. C. R., Warren, J., and Behrens, T. E. J. (2021). Relating transformers to models and neural representations of the hippocampal formation. arX [Preprint] doi: 10.48550/arx.2112.04035
Xu, Q., Baevski, A., Likhomanenko, T., Tomasello, P., Conneau, A., Collobert, R., et al. (2021). “Self-training and pre-training are complementary for speech recognition,” in Proceedings of the ICASSP, IEEE international conference on acoustics, speech and signal processing, (Toronto: IEEE), 3030–3034. doi: 10.1109/ICASSP39728.2021.9414641
Yamins, D. L. K., Hong, H., Cadieu, C. F., Solomon, E. A., Seibert, D., and DiCarlo, J. J. (2014). Performance-optimized hierarchical models predict neural responses in higher visual cortex. Proc. Natl. Acad. Sci. U.S.A. 111, 8619–8624. doi: 10.1073/pnas.1403112111
Keywords: artificial intelligence, brain, brain-inspired intelligence, neurobiological explainability, AI evaluation, artificial neural network
Citation: Chen Y, Wei Z, Gou H, Liu H, Gao L, He X and Zhang X (2022) How far is brain-inspired artificial intelligence away from brain? Front. Neurosci. 16:1096737. doi: 10.3389/fnins.2022.1096737
Received: 12 November 2022; Accepted: 24 November 2022;
Published: 09 December 2022.
Edited by:
Zhiguo Zhang, Harbin Institute of Technology, ChinaReviewed by:
Yanghua Tian, The First Affiliated Hospital of Anhui Medical University, ChinaPeng Li, Shenzhen University, China
Copyright © 2022 Chen, Wei, Gou, Liu, Gao, He and Zhang. This is an open-access article distributed under the terms of the Creative Commons Attribution License (CC BY). The use, distribution or reproduction in other forums is permitted, provided the original author(s) and the copyright owner(s) are credited and that the original publication in this journal is cited, in accordance with accepted academic practice. No use, distribution or reproduction is permitted which does not comply with these terms.
*Correspondence: Li Gao, gaoli@shu.edu.cn; Xiaosong He, hexs@ustc.edu.cn; Xiaochu Zhang, zxcustc@ustc.edu.cn
†These authors have contributed equally to this work