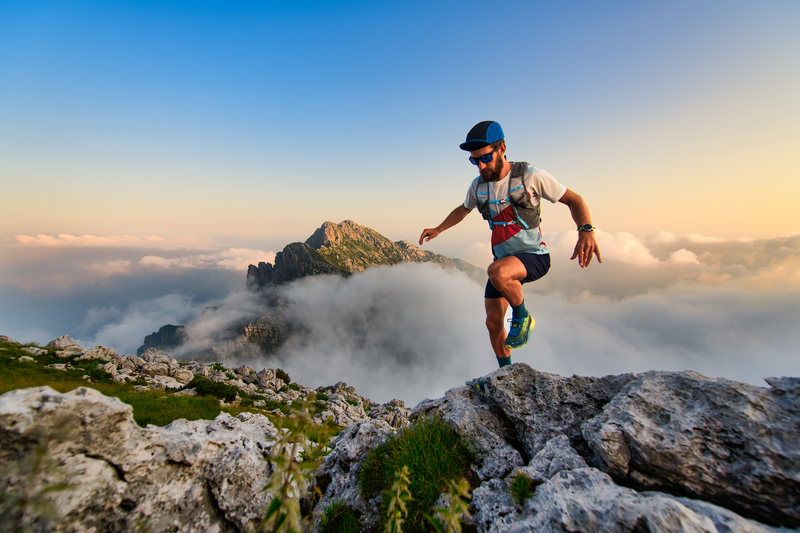
94% of researchers rate our articles as excellent or good
Learn more about the work of our research integrity team to safeguard the quality of each article we publish.
Find out more
ORIGINAL RESEARCH article
Front. Neurosci. , 19 January 2023
Sec. Translational Neuroscience
Volume 16 - 2022 | https://doi.org/10.3389/fnins.2022.1031712
This article is part of the Research Topic Advances in Theranostics of CNS Injuries and Diseases: From Basic Research to Clinical Practice View all 10 articles
A correction has been applied to this article in:
Corrigendum: Prediction of motor function in patients with traumatic brain injury using genetic algorithms modified back propagation neural network: a data-based study
Objective: Traumatic brain injury (TBI) is one of the leading causes of death and disability worldwide. In this study, the characteristics of the patients, who were admitted to the China Rehabilitation Research Center, were elucidated in the TBI database, and a prediction model based on the Fugl-Meyer assessment scale (FMA) was established using this database.
Methods: A retrospective analysis of 463 TBI patients, who were hospitalized from June 2016 to June 2020, was performed. The data of the patients used for this study included the age and gender of the patients, course of TBI, complications, and concurrent dysfunctions, which were assessed using FMA and other measures. The information was collected at the time of admission to the hospital and 1 month after hospitalization. After 1 month, a prediction model, based on the correlation analyses and a 1-layer genetic algorithms modified back propagation (GA-BP) neural network with 175 patients, was established to predict the FMA. The correlations between the predicted and actual values of 58 patients (prediction set) were described.
Results: Most of the TBI patients, included in this study, had severe conditions (70%). The main causes of the TBI were car accidents (56.59%), while the most common complication and dysfunctions were hydrocephalus (46.44%) and cognitive and motor dysfunction (65.23 and 63.50%), respectively. A total of 233 patients were used in the prediction model, studying the 11 prognostic factors, such as gender, course of the disease, epilepsy, and hydrocephalus. The correlation between the predicted and the actual value of 58 patients was R2 = 0.95.
Conclusion: The genetic algorithms modified back propagation neural network can predict motor function in patients with traumatic brain injury, which can be used as a reference for risk and prognosis assessment and guide clinical decision-making.
Traumatic brain injury (TBI) is not a one-time event but a condition, which is one of the leading causes of death and disability worldwide. This disease can develop among people of any age, often causing labor loss (Brain and Spinal Injury Center, 2021). With the development of medicine, the survival rate of patients, suffering from multi-degree TBI, increased and the number of disabilities in patients decreased, improving the quality of patients’ life and their social issues (Pickelsimer et al., 2006). It is essential to predict the functional outcome, which improves the therapeutic schedule and adjusts personal livelihood (Yu et al., 2015).
In the previous studies, which predicted the mortality and GCS score, the indicators, such as GCS score, pupil response, and laboratory examination, were mainly identified in the acute phase after trauma (Roberts et al., 2004; Hukkelhoven et al., 2005; Perel et al., 2006, 2008; Rogers et al., 2010; Subaiya et al., 2012; Ercole et al., 2021). As compared to the patients in the acute phase, those in the chronic phase tended to be stable with decreased cerebral edema, and fewer acute intracranial lesions, which were favorable factors for the prediction of prognosis. However, many factors, such as rehabilitation therapies, location of the lesion, limb complications, etc., might affect the outcome of motor function.
As dysfunction is a common outcome of moderate to severe TBI. The alleviation of dysfunction and improvement of the quality of life are the primary research focus. The indicators, including GCS and pupil response, are not suitable for the prediction of outcomes in the chronic phase. Therefore, the degree of dysfunction in the patients with TBI, especially the motor function, is needed to be predicted (Perel et al., 2008; Reith et al., 2017). In the current clinical practices, the rehabilitation assessment, such as the functional independence measure (FIM), which assesses the overall prognosis of living ability, and the Fugl-Myer assessment scale (FMA), which assesses the motor function, might be used to cope with this deficiency (Ottenbacher et al., 1996; Segal et al., 1996; Zarshenas et al., 2019). The current study focused on the FMA and its effects on the upper and lower limbs.
Corticosteroid Randomization After Significant Head Injury (CRASH) and International Traumatic Brain Injury Clinical Trials Prognosis and Analysis Task (IMPACT) are relatively mature models, which can predict the outcome of TBI; their prognostic potential has been evaluated (Boyd et al., 1987; Dodds et al., 1993; Edwards et al., 2005; Rogers et al., 2010; Ercole et al., 2021). The foundation and validation of the prediction model are based on TBI clinical trials, including Collaborative European Neurotrauma Effectiveness Research in TBI (CENTER-TBI), Translational Research, and Clinical Knowledge in TBI (TRACK-TBI), and Japan Neurotrauma Database (JNTDB) (Steyerberg et al., 2008; Maeda et al., 2019; Gao et al., 2020). Based on the available data, the prediction models were built using statistical models, such as correlation, regression, non-linear fitting, and artificial neural networks (ANN) analyses. Although the previously established model could not predict FMA in the patients with dysfunction after TBI, these methods can be used to establish a prediction model.
The backpropagation (BP) neural model is one of the most common and mature models based on ANN. A large number of studies have used ANN to predict the prognosis and complications of TBI. However, these models focus on the outcome prediction, such as mortality, rather than the function prediction. This study aimed to use the BP neural network model for predicting the FMA outcome in TBI patients in the chronic phase.
In this study, the measures were retrospectively analyzed at the time of the patient’s admission to the Beijing Boai hospital and during hospitalization. The possible indicators, which were related to the motor function of the patients, were also analyzed. The demographic information of the patients, excluding their personal information, was collected. The study was approved by the Medical Ethics Committee of China Rehabilitation Research Center (No. 2021-026-1).
A total of 463 TBI patients, who were admitted to the China Rehabilitation Research Center from June 2016 to June 2020, were enrolled in this study. According to the International Statistical Classification of Diseases and Related Health Problems-tenth edition (ICD-10),the diagnosis of the hospitalized patients in this study included TBI (S06.902), traumatic intracranial hematoma (S06.806), diffuse axonal injury (S06.204), concussion (S06.001), subdural hematoma (S06.501), epidural hematoma (S06.401), traumatic subarachnoid hemorrhage (S06.601), and brain contusion and laceration (S06.201).
The criteria for the inclusion of patients in this included (1) definitive diagnosis of TBI; (2) complete availability of required data; and (3) the patients performed physical and occupying therapy during hospitalization. The criteria for the exclusion of patients from this included (1) the patients with the disorders of consciousness; (2) the patients in unstable periods; (3) the patients with dyskinesia caused by fractures or any other reason; (4) the patients, who were hospitalized for less than 4 weeks; and (5) the patients, who did not cooperate due to other reasons.
In this study, the data of the patient’s age, gender, course of TBI, years of education, marital status, occupation, place of residence (urban or rural), history of tobacco and alcohol use, medical history, injury location, cause of injury, lesion laterality, type of surgery, skull defect, clinical symptoms, diagnosis, tracheotomy, complications, such as epilepsy, hydrocephalus and aphasia, mental or emotional disorders, motor dysfunction, such as shoulder subluxation, shoulder-hand syndrome, disuse syndrome, and joint spasm, and rehabilitation therapies, etc., was collected. The measures, including Fugl-Meyer balance scale (FMB), Hand Practicability assessment scale, Mini-Mental State Examination Scale (MMSE), and Fugl-Meyer assessment scale (FMA), were calculated at the time of the patient’s admission to the hospital, and the FMA scores were calculated after 4 weeks of admission.
Data scoring: TBI was given scores, ranging from 1 to 5 for <1 month, 1–3 months, 3–6 months, 4 months–1 years, and >1 year, respectively. The male and female patients were indicated by 0 and 1, respectively. The presence and absence of other complications or treatments were indicated by 1 and 0, respectively. Pearson correlation was performed using SPSS 25 for determining the factors related to the outcome (4 weeks after admission), which were also included in the establishment of the prognosis model. For each input and output item, the score was recorded as P(initial), while the highest score was recorded as P(maximum). The score of each patient was calculated as P = P(initial)/P(maximum). The scores of all items were used as input in the predicting model.
The correlation analysis showed that the factors, including gender, course of TBI, rehabilitation treatment, epilepsy or hydrocephalus, aphasia, tracheotomy, concurrent dysfunction, balance, and motor function, were significantly correlated with F1 (Table 1).
This study used the neural fitting application in MATLAB 2021b software. The GA-BP neural model was applied to predict the motor function. A one-layer neural network model (Figure 1) and FMA were applied for the normalization of input and output data, respectively. To prevent over-fitting and realize external inspection, authors divided patients into two subgroups, one with 175 (75%) patients to construct and test the model, the other with 58 (25%) patients to inspect the model. The correlations between the predicted and actual values were analyzed. Then, the prediction effects were verified and the prediction differences were calculated (mapping software origin 9.1).
Figure 1. Schematic diagram of the optimal BP neural network model with 11 input nodes, 10 nodes in a hidden layer, and a single output node.
This study included a total of 463 patients (Supplementary material). Among them, a total of 233 patients were included in the analysis of the neural network model (Figure 2).
Among the patients included in this study, males were significantly more than females (348:115) but did not show significant differences in their ages, courses after TBI, and durations of hospital stay. However, the proportion of smoking and drinking among males was significantly higher as compared to the females (χ2 = 33.62, P < 0.01, OR = 6.22). Meanwhile, the incidences of concurrent diseases among males, such as hypertension, diabetes, coronary heart disease, and cerebrovascular accident were significantly higher as compared to those among the females (χ2 = 9.41, P < 0.01, OR = 1.96).
The causes of injuries among males were relatively diverse, among which, the car accident was the major cause, accounting for 47.41% of the causes of injuries. The females showed similar results; car accidents accounted for 84.35% of the causes of injuries, followed by falls (15.65%).
Most cases (98.70%) in this study were identified as severe cases (Figure 3A). The incidence of epidural hematoma and diffuse axonal injury among females was significantly higher as compared to those among the males (P < 0.01, OR = 13.14; P < 0.01, OR = 6.30), while the incidence of intracranial hematoma (and brain herniation) among females was significantly lower as compared to that among the males (χ2 = 27.78, P < 0.01, OR = 1.38; χ2 = 7.40, P < 0.01, OR = 4.18).
Figure 3. (A) Patient distribution of incidence rate of principal diagnosis. (B) Rate of the different types of surgeries after TBI. (C) Incidence rates of all the complications at the time of admission. (D) Incidence rate of all the dysfunctions at the time of admission. EDH, epidural hemorrhage; DAI, diffuse axonal injury; TICH, traumatic intracranial hemorrhage; RIH, removal of intracranial hemorrhage; PDIH, puncture drainage of intracranial hemorrhage; DS, disuse syndrome; SSL, shoulder subluxation; VTE, venous thromboembolism; SHS, shoulder-hand syndrome; HO, heterotopic ossification; USI, urinary system infection; PS, pressure sores; CI, secondary cerebral infarction after stroke; CIA, cognitive impairment assessment; DOC, disturbance of consciousness; ED, emotional disorder; E/V impairment, eyesight/vision impairment; and HD, headache or dizziness.
The surgery rate among all the patients was 70.19%, with little gender difference (71.84% vs. 65.22%). Most of the surgeries were performed for the removal of hematoma using craniotomy (69.54% vs. 60%), followed by hematoma puncture and drainage (2.30% vs. 5.22%) (Figure 3B). Among all the patients, undergoing surgery, 59 cases (18.15%) went through a second surgical procedure, which included puncture and drainage of hematoma.
Hydrocephalus (46.84%), epilepsy (27.87%), and shoulder subluxation (12.64%) were the most common complications among males (Figure 3C). On the other hand, among females, hydrocephalus (45.22%), shoulder subluxation (19.13%), and disuse syndrome (16.52%) were the most common complications, while the incidence of epilepsy was slightly lower among them (15.65%).
Among the types of dysfunctions, both the males and females showed higher consistencies (Figure 3D). Cognitive impairment (66.09% vs. 62.61%), dyskinesia (61.21% vs. 70.43%), and language impairment (32.76% vs. 36.52%) were the most common types dysfunctions. Bilateral dyskinesia showed the highest consistency (31.03% vs. 32.17%).
Based on their correlations to the actual FMA, all the 11 prognostic-related factors were included in the prediction model (Table 1). After constructed the mode with 175 samples, the GA-BP neural network was built and the code can be found in https://github.com/swlmed/swlmed-public. Pearson correlation between predicted and actual values of the model was R2 = 0.97 and both of these values were shown in Supplementary Figure 2. The weight of every features to neurons and neurons to output listed in Table 2.
Of all the 58 test patients, the predicted and actual FMA values are shown in Figure 4. Pearson correlation analysis was used to evaluate the differences between the predicted and actual FMA values (R2 = 0.95, P < 0.01, Figure 5).
Figure 5. Correlation between the predictive and actual FMA value of 58 patients. Prediction value = –1.40 + 1.05 × actual value, R2 = 0.95.
Backpropagation neural network is a multi-layer feed forward network trained by the error BP algorithm, proposed by Rumelhart et al. (1986). The BP network stores a large number of input-output pattern mapping correlations, describing the mapping correlation without the need for revealing the mathematical equations. Using the gradient descent method, it continuously adjusts the network through BP and minimizes the sum of squared errors of the network. Therefore, it also avoids the use of linear correlations between data. Numerous factors, affecting the degree of injury and prognosis of TBI patients, have unclear correlations among them. Currently, BP neural network is an easily applicable model (Rau et al., 2018).
Although mild TBIs account for the majority of the cases, in this study, moderate to severe TBIs accounted for the majority of the cases (98.70%). The reasons might include the lower hospitalization rate of the patients with mild TBI. Moreover, the patients with concussions mostly complaint about headaches, nervousness, inattention, forgetfulness, etc., which can be cured. The severely affected limb function is mainly manifested in the patients with moderate to severe TBI. Therefore, the improvement of motor function was mainly studied among these patients (Levin and Diaz-Arrastia, 2015; Hon et al., 2019; Silverberg et al., 2020).
In this study, correlation analysis was used to explore the prognostic factors. For this purpose, 11 prognostic-related indicators were investigated. In the current study, age was not included as a prognostic factor, as used in the previously reported mortality prediction models, affecting the survival rate of the TBI patients (Gang et al., 2019; Li et al., 2020). After involving the rehabilitation period, the focus of rehabilitation gradually changed from the central nervous system to the rehabilitation of the central-peripheral joint with the weakened effect of neuroplasticity, among which, age might play a less important role than that in the acute period. Unlike the previous prognostic prediction models, this study predicted continuous changes in the FMA, rather than categorizing the results, thereby showing relatively more refinement of the results. As compared to the real results, this prediction model had a satisfying accuracy rate (R2 = 0.95). The reliability of the results was obtained by the integration of training, validation, and test datasets.
In order to establish the TBI prediction model, ANN was used for predicting the prognosis and complications due to its flexibility and other advantages (DiRusso et al., 2000; Rughani et al., 2010; Hannah et al., 2020; Wang et al., 2021). ANN was superior to the TRISS model in terms of accurate mortality prediction (Matsuo et al., 2020). ANN could achieve higher accuracy than the CRASH and IMPACT models in terms of predicting mortality in patients with moderate to severe TBIs (Rau et al., 2018). ANN was more sensitive than the traditional models in predicting the mortality of the patients with severe cases (Yao et al., 2020), underwent surgery (Shi et al., 2013), mechanical ventilation (Abujaber et al., 2020a,b), septicemia (Kim et al., 2011), as well as in identifying the clinically relevant pediatric TBI (Hale et al., 2018).
The data-driven prediction models, such as CRASH and IMPACT, were based on the databases; as the database updated, their prediction accuracies improved (Hannah et al., 2020). This implied that the data-driven could be continuously improved with the refining of data. Therefore, for these models, the critical point is a database with sufficient data. Although, China collects the TBI information through various systems, such as the Nationally Representative Disease Monitoring Point System (DSP), Hospital Quality Monitoring System (HQMS), and National Injury Monitoring System (NISS), a uniform data system for medical rehabilitation (UDMSR) for the collection of data information in a unified way is currently lacking (Granger et al., 2010; Oyesanya et al., 2021). In this study, a database, containing the rehabilitation information of 463 patients, was established. The outcome measure of this study was FMA, which is one of the most commonly used indices of motor function. In the end, only 233 patients were included in this study. The information in the database was inefficient, which might be due to the following reasons. First, the patients under rehabilitation might have more comorbidities; therefore, numerous factors might affect the rehabilitation treatment. Second, the patients came from different areas in China. Therefore, it was difficult to follow up with the patients after discharge for the follow-up assessment.
This study started with the admission of patients with TBI to a rehabilitation hospital and used the functional assessment as the baseline. The characteristics of TBI were elucidated and the prediction model based on FMA was established to predict the outcome of motor dysfunction. The prediction model had low requirements, strong operation ability, and easy promotion. It had high practicability for the hospitals with fewer research resources and might serve as a reference in the risk and prognosis assessment as well as guiding the clinical decision-making.
The first limitation of this study was the small sample size obtained from a single medical center. This study lacked the indicators, which directly reflected the severity of TBI in the patients. Therefore, this might have affected the results. Second, the model could not clearly explain which factor was listed at the top. Finally, this study assumed unified rehabilitation therapies for all the patients and did not consider their differences in them.
In addition to the above-mentioned shortcomings, this study used neural network and regression models to predict the prognosis of motor function in patients with TBI and provided novel ideas for the evaluation of the patients with TBI.
The original contributions presented in this study are included in the article/Supplementary material, further inquiries can be directed to the corresponding authors.
HD contributed to the study design, literature search, figures, data extraction, data analysis, and writing. ZT and WS contributed to the figures, data extraction, data interpretation, and writing. HZ and SY contributed to the study design and data interpretation. All authors contributed to the article and approved the submitted version.
This work was supported by the National Key Research and Development Program of China (Grant No. 2018YFC2001703), the National Natural Science Foundation of China (Grant No. U1809209), and China Rehabilitation Research Center Key Project (Grant Nos. 2021ZX-02 and 2021ZX-19).
We thank all the reviewers who participated in the review and MJEditor (www.mjeditor.com) for its linguistic assistance during the preparation of this manuscript.
The authors declare that the research was conducted in the absence of any commercial or financial relationships that could be construed as a potential conflict of interest.
All claims expressed in this article are solely those of the authors and do not necessarily represent those of their affiliated organizations, or those of the publisher, the editors and the reviewers. Any product that may be evaluated in this article, or claim that may be made by its manufacturer, is not guaranteed or endorsed by the publisher.
The Supplementary Material for this article can be found online at: https://www.frontiersin.org/articles/10.3389/fnins.2022.1031712/full#supplementary-material
Abujaber, A., Fadlalla, D. G., Abdelrahman, H., Mollazehi, M., and El-Menyar, A. (2020a). Using trauma registry data to predict prolonged mechanical ventilation in patients with traumatic brain injury: Machine learning approach. PLoS One 15:e0235231. doi: 10.1371/journal.pone.0235231
Abujaber, A., Fadlalla, D. G., Abdelrahman, H., Mollazehi, M., and El-Menyar, A. (2020b). Prediction of in-hospital mortality in patients on mechanical ventilation post traumatic brain injury: Machine learning approach. BMC Med. Inform. Decis. Mak. 20:336. doi: 10.1186/s12911-020-01363-z
Boyd, C. R., Tolson, M. A., and Copes, W. S. (1987). Evaluating trauma care: The TRISS method. trauma score and the injury severity score. J. Trauma 27, 370–378.
Brain and Spinal Injury Center (2021). TRACK-TBI: Transforming research and clinical knowledge in TBI. San Francisco: University of California. [Accessed 2021 Oct 15]. https://tracktbi.ucsf.edu.
DiRusso, S. M., Sullivan, T., Holly, C., Cuff, S. N., and Savino, J. (2000). An artificial neural network as a model for prediction of survival in trauma patients: Validation for a regional trauma area. J. Trauma 49, 212–20;discussion220–3. doi: 10.1097/00005373-200008000-00006
Dodds, T. A., Martin, D. P., Stolov, W. C., and Deyo, R. A. (1993). A validation of the functional independence measurement and its performance among rehabilitation inpatients. Arch. Phys. Med. Rehabil. 75, 531–536. doi: 10.1016/0003-9993(93)90119-u
Edwards, P., Arango, M., Balica, L., Cottingham, R., El-Sayed, H., Farrell, B., et al. (2005). Final results of MRC CRASH, a randomised placebo-controlled trial of intravenous corticosteroid in adults with head injury-outcomes at 6 months. Lancet 365, 1957–1959. doi: 10.1016/S0140-6736(05)66552-X
Ercole, A., Dixit, A., Nelson, D. W., Bhattacharyay, S., Zeiler, F. A., Nieboer, D., et al. (2021). Imputation strategies for missing baseline neurological assessment covariates after traumatic brain injury: A CENTER-TBI study. PLoS One 16:e0253425. doi: 10.1371/journal.pone.0253425
Gang, M. C., Hong, K. J., Shin, S. D., Song, K. J., Ro, Y. S., Kim, T. H., et al. (2019). New prehospital scoring system for traumatic brain injury to predict mortality and severe disability using motor Glasgow Coma Scale, hypotension, and hypoxia: a nationwide observational study. Clin. Exp. Emerg. Med. 6, 152–159. doi: 10.15441/ceem.18.027
Gao, G., Wu, X., Feng, J., Hui, J., Mao, Q., Lecky, F., et al. (2020). Clinical characteristics and outcomes in patients with traumatic brain injury in China: A prospective, multicentre, longitudinal, observational study. Lancet Neurol. 19, 670–677. doi: 10.1016/s1474-4422(20)30182-4
Granger, V., Markello, S., Graham, J., Deutsch, A., Reistetter, T., and Ottenbacher, K. J. (2010). The uniform data system for medical rehabilitation: report of patients with traumatic brain injury discharged from rehabilitation programs in 2000-2007. Am. J. Phys. Med. Rehabil. 89, 265–278. doi: 10.1097/PHM.0b013e3181d3eb20
Hale, T., Stonko, D. P., Lim, J., Guillamondegui, O. D., Shannon, C. N., and Patel, M. B. (2018). Using an artificial neural network to predict traumatic brain injury. J. Neurosurg. Pediatr. 23, 219–226. doi: 10.3171/2018.8.PEDS18370
Hannah, L., Radabaugh, J. B., Dietrich, W. D., and Helen, M. (2020). Bramlett, odelia schwartz and dilip sarkar: Development and evaluation of machine learning models for recovery prediction after treatment for traumatic brain injury. Ann. Int. Conf. IEEE Eng. Med. Biol. Soc. 2020, 2416–2420. doi: 10.1109/EMBC44109.2020.9175658
Hon, K. L., Leung, A. K. C., and Torres, A. R. (2019). Concussion: A global perspective. Semin. Pediatr. Neurol. 30, 117–127. doi: 10.1016/j.spen.2019.03.017
Hukkelhoven, C. W., Steyerberg, E., Dik, J., Habbema, F., Habbema, J., Farace, E., et al. (2005). Predicting outcome after traumatic brain injury: development and validation of a prognostic score based on admission characteristics. J. Neurotrauma 22, 1025–1039. doi: 10.1089/neu.2005.22.1025
Kim, S., Kim, W., and Park, R. W. (2011). A comparison of intensive care unit mortality prediction models through the use of data mining techniques. Healthc. Inform. Res. 17, 232–243. doi: 10.4258/hir.2011.17.4.232
Levin, H. S., and Diaz-Arrastia, R. R. (2015). Diagnosis, prognosis, and clinical management of mild traumatic brain injury. Lancet Neurol. 14, 506–517. doi: 10.1016/s1474-4422(15)00002-2
Li, X., Lü, C., Wang, J., Wan, Y., Dai, S. H., Zhang, L., et al. (2020). Establishment and validation of a model for brain injury state evaluation and prognosis prediction. Chin. J. Traumatol. 23, 284–289. doi: 10.1016/j.cjtee.2020.08.006
Maeda, Y., Ichikawa, R., Misawa, J., Shibuya, A., Hishiki, T., Maeda, T., et al. (2019). External validation of the TRISS, CRASH, and IMPACT prognostic models in severe traumatic brain injury in Japan. PLoS One 14:e0221791. doi: 10.1371/journal.pone.0221791
Matsuo, K., Aihara, H., Nakai, T., Morishita, A., Tohma, Y., and Kohmura, E. (2020). Machine learning to predict in-hospital morbidity and mortality after traumatic brain injury. J. Neurotrauma 37, 202–210. doi: 10.1089/neu.2018.6276
Ottenbacher, K. J., Hsu, Y., Granger, C. V., and Fiedler, R. C. (1996). The reliability of the functional independence measure: A quantitative review. Arch. Phys. Med. Rehabil. 77, 1226–1232. doi: 10.1016/s0003-9993(96)90184-7
Oyesanya, T. O., Moran, T. P., Espinoza, T. R., and Wright, D. W. (2021). Regional variations in rehabilitation outcomes of adult patients with traumatic brain injury: A uniform data system for medical rehabilitation investigation. Arch. Phys. Med. Rehabil. 102, 68–75. doi: 10.1016/j.apmr.2020.07.011
Perel, P., Arango, M., Clayton, T., Edwards, P., Komolafe, E., Poccock, S., et al. (2008). Predicting outcome after traumatic brain injury: Practical prognostic models based on large cohort of international patients. BMJ 336, 425–429. doi: 10.1136/bmj.39461.643438.25
Perel, P., Edwards, P., Wentz, R., and Roberts, I. (2006). Systematic review of prognostic models in traumatic brain injury. BMC Med. Inform. Decis. Mak. 6:38. doi: 10.1186/1472-6947-6-38
Pickelsimer, E. E., Selassie, A., Gu, J., and Langlois, J. A. (2006). A population-based outcomes study of persons hospitalized with traumatic brain injury: Operations of the South Carolina traumatic brain injury follow-up registry. J. Head Trauma Rehabil. 21, 491–504. doi: 10.1097/00001199-200611000-00004
Rau, C. S., Kuo, P. J., Chien, P. C., Huang, C. Y., Hsieh, H. Y., and Hsieh, C. H. (2018). Mortality prediction in patients with isolated moderate and severe traumatic brain injury using machine learning models. PLoS One 13:e0207192. doi: 10.1371/journal.pone.0207192
Reith, F., Lingsma, H., Gabbe, B., Lecky, F., Roberts, I., and Maas, A. (2017). Differential effects of the glasgow coma scale score and its components: An analysis of 54,069 patients with traumatic brain injury. Injury 48, 1932–1943. doi: 10.1016/j.injury.2017.05.038
Roberts, I., Yates, D., Sandercock, P., Farrell, B., Wasserberg, J., Lomas, G., et al. (2004). Effect of intravenous corticosteroids on death within 14 days in 10008 adults with clinically significant head injury (MRC CRASH trial): Randomised placebo-controlled trial. Lancet 364, 1321–1328. doi: 10.1016/S0140-6736(04)17188-2
Rogers, S. C., Campbell, B., Saleheen, H., Borrup, K., and Lapidus, G. (2010). Using trauma registry data to guide injury prevention program activities. J. Trauma 69(4 Suppl), S209–S213. doi: 10.1097/TA.0b013e3181f1e9fe
Rughani, A. I., Dumont, T. M., Lu, Z., Bongard, J., Horgan, M. A., Penar, P. L., et al. (2010). Use of an artificial neural network to predict head injury outcome. J. Neurosurg. 113, 585–590. doi: 10.3171/2009.11.JNS09857
Rumelhart, D. E., Hinton, G. E., and Williams, R. J. (1986). Learning representations by back-propagating errors. Nature 323, 533–536. doi: 10.1038/323533a0
Segal, E., Gillard, M., and Schall, R. R. (1996). Telephone and in-person proxy agreement between stroke patients and caregivers for the functional independence measure. Am. J. Phys. Med. Rehabil. 75, 208–212. doi: 10.1097/00002060-199605000-00013
Shi, H. Y., Hwang, S. L., Lee, K. T., and Lin, C. L. (2013). In-hospital mortality after traumatic brain injury surgery: A nationwide population-based comparison of mortality predictors used in artificial neural network and logistic regression models. J. Neurosurg. 118, 746–752. doi: 10.3171/2013.1.JNS121130
Silverberg, N. D., Iaccarino, M. A., Panenka, W. J., Iverson, G. L., McCulloch, K. L., Dams-O’Connor, K., et al. (2020). Management of concussion and mild traumatic brain injury: A synthesis of practice guidelines. Arch. Phys. Med. Rehabil. 101, 382–393. doi: 10.1016/j.apmr.2019.10.179
Steyerberg, E. W., Mushkudiani, N., Perel, P., Butcher, I., Lu, J., McHugh, G., et al. (2008). Predicting outcome after traumatic brain injury: development and international validation of prognostic scores based on admission characteristics. PLoS Med. 5:e165;discussione165. doi: 10.1371/journal.pmed.0050165
Subaiya, S., Roberts, I., Komolafe, E., and Perel, P. (2012). Predicting intracranial hemorrhage after traumatic brain injury in low and middle-income countries: A prognostic model based on a large, multi-center, international cohort. BMC Emerg. Med. 12:17. doi: 10.1186/1471-227X-12-17
Wang, X., Zhong, J., Lei, T., Chen, D., Wang, H., Zhu, L., et al. (2021). An artificial neural network prediction model for posttraumatic epilepsy: Retrospective cohort study. J. Med. Internet Res. 23:e25090. doi: 10.2196/25090
Yao, R. Q., Jin, X., Wang, G. W., Yu, Y., Wu, G. S., Zhu, Y. B., et al. (2020). A machine learning-based prediction of hospital mortality in patients with postoperative sepsis. Front. Med. (Lausanne) 7:445. doi: 10.3389/fmed.2020.00445
Yu, J., Tam, H. M., and Lee, T. M. (2015). Traumatic brain injury rehabilitation in Hong Kong: A review of practice and research. Behav. Neurol. 2015:274326. doi: 10.1155/2015/274326
Keywords: TBI, back propagation neural network, prediction model, FMA, database
Citation: Dang H, Su W, Tang Z, Yue S and Zhang H (2023) Prediction of motor function in patients with traumatic brain injury using genetic algorithms modified back propagation neural network: a data-based study. Front. Neurosci. 16:1031712. doi: 10.3389/fnins.2022.1031712
Received: 30 August 2022; Accepted: 30 December 2022;
Published: 19 January 2023.
Edited by:
Bo Li, Sun Yat-sen University, ChinaReviewed by:
Weitao Man, Tsinghua University, ChinaCopyright © 2023 Dang, Su, Tang, Yue and Zhang. This is an open-access article distributed under the terms of the Creative Commons Attribution License (CC BY). The use, distribution or reproduction in other forums is permitted, provided the original author(s) and the copyright owner(s) are credited and that the original publication in this journal is cited, in accordance with accepted academic practice. No use, distribution or reproduction is permitted which does not comply with these terms.
*Correspondence: Shouwei Yue, c2hvdXdlaXlAc2R1LmVkdS5jbg==; Hao Zhang,
Y3JyY3poMjAyMEAxNjMuY29t
Disclaimer: All claims expressed in this article are solely those of the authors and do not necessarily represent those of their affiliated organizations, or those of the publisher, the editors and the reviewers. Any product that may be evaluated in this article or claim that may be made by its manufacturer is not guaranteed or endorsed by the publisher.
Research integrity at Frontiers
Learn more about the work of our research integrity team to safeguard the quality of each article we publish.