- 1First Hospital of Shanxi Medical University, Taiyuan, China
- 2Department of Psychiatry, First Hospital of Shanxi Medical University, Taiyuan, China
Objective: The microbiota–gut–brain axis, especially the inflammatory pathway, may play a critical role in the pathogenesis of cognitive impairment in major depressive disorder (MDD). However, studies on the microbiota-inflammatory-cognitive function axis in MDD are lacking. The aim of the present study was to analyze the gut microbiota composition and explore the correlation between gut microbiota and inflammatory factors, cognitive function in MDD patients.
Method: Study participants included 66 first-episode, drug naïve MDD patients as well as 43 healthy subjects (HCs). The composition of fecal microbiota was evaluated using16S rRNA sequencing and bioinformatics analysis. The cytokines such as hs-CRP, IL-1β, IL-6, IL-10, and TNF-α in peripheral blood were detected via enzyme linked immunosorbent assay (ELISA); assessment of cognitive functions was performed using the Color Trail Test (CTT), The Repeatable Battery for the Assessment of Neuropsychological Status (RBANS) and the Stroop Color-Word Test (SCWT).
Results: We found that compared with HCs, MDD patients had cognitive impairments and showed different α-diversity and β-diversity of gut microbiota composition. LDA effect size (LEfSe) analysis found MDD have higher Deinococcaceae and lower Bacteroidaceae, Turicibacteraceae, Clostridiaceae and Barnesiellaceae at family level. Deinococcus and Odoribacter was higher in the MDD group, however, Bacteroides, Alistipes, Turicibacter, Clostridium, Roseburia, and Enterobacter were lower at genus level. Furthermore, In MDD patients, the Bacteroidaceae and Bacteroides were both positively correlated with hsCRP, CCT1, CCT2. Alistipes was positively correlated with IL-6, Word time, Color time, Word-Color time, Color-Word time and negatively correlated with Delayed Memory, Total score and Standardized score. Turicibacteraceae and Turicibacter were both negatively correlated with IL-1β and IL-6.
Conclusion: The present findings confirm that the gut microbiota in MDD patients have altered gut microbes that are closely associated with inflammatory factors and cognitive function in MDD patients.
Introduction
Gut microbiota functions as a vital actor in the bidirectional communication between the digestive system and the central nervous system (CNS), also called the brain–gut–microbiota axis (Rhee et al., 2009). Increasingly, gut microbiota appears to influence brain functions through the autoimmune and endocrinology pathways (Huang et al., 2019). In recent decades, researchers have demonstrated the presence of bidirectional communication between the gut and brain via the brain–gut–microbiota axis (Yong et al., 2019; Yang et al., 2020; Liu et al., 2021). Some cross-sectional studies have found altered gut microbes in individuals with mental disorders (Dickerson et al., 2017; Bruce-Keller et al., 2018), particularly in major depressive disorder (MDD) (Sanada et al., 2020).
A study conducted by Naseribafrouei et al. (2014) analyzed the state of gut microbiota in individuals with MDD and healthy controls (HCs), and reported that when Bacteroidales, Oscillibacter, and Alistipes were present in higher levels, the Lachnospiraceae were linked with depressive symptoms. Other researchers studied the gut microbiota present in active-MDD (A-MDD) subjects, responding-MDD (R-MDD) subjects, and HCs (Jiang et al., 2015). They discovered that MDD patients had increased Proteobacteria, Bacteroidetes, and Actinobacteria, as well as decreased Faecalibacterium. Additionally, Faecalibacterium was negatively associated with depressive symptom severity (Jiang et al., 2015). Zheng et al. (2016) found higher levels of Actinobacteria and Bacteroidetes and lower levels of Firmicutes in MDD patients; they also found that transferring MDD feces into germ-free (GF) mice resulted in depressive phenotypes in recipient mice.
Gut microbiota plays a significant role in the development of the gut’s immune system, and host–microbe interactions impact the gut’s immunological homeostasis (Furusawa et al., 2013). When gut microbiota is disturbed, microbial-associated molecular patterns—those such as lipopolysaccharide (LPS) and bacterial lipoprotein—can activate immune cells and toll-like receptors (TLR) to trigger the release of pro-inflammatory cytokines. The effect is to increase the permeability of the gut–blood barrier and blood–brain barrier (BBB), and to regulate the function and behavior of the CNS (Petra et al., 2015). As such, gut microbiota may affect CNS function by inducing an inflammatory response. In addition, the vagus nerve (VN) can connect the CNS to the enteric nervous system (ENS), which can directly facilitate immunoregulatory signals to the brain and gut (Breit et al., 2018).
Cognitive deficits are present in patients with neuropsychiatric disorders. Impaired cognitive function has been described in the MDD patients. Numerous studies, reviews, and meta-analyses show a considerable impairment in inhibition, shifting, verbal working memory, visuospatial working memory, and verbal fluency (Ahern and Semkovska, 2016). Gut microbiota is closely related to cognitive function. Studies conducted using animal models have uncovered mutual interaction between the brain and gut microbiota in the development of Alzheimer’s disease (AD). It has been found that GF mice showed non-spatial and working memory impairment when studied alongside specific pathogen free (SPF) mice (Gareau et al., 2011). Desbonnet et al. (2015) found that antibiotic treatment significantly reduced the abundance and diversity of intestinal microbiota in weaned mice, and that non-spatial memory also declined on the Novel Object Recognition Test (NORT). Clinical trials have confirmed the presence of increased Escherichia, Shigella, Bacteroides, and Ruminococcus, in addition to the decreased E. rectale, Bifidobacterium, and Dialister in the feces of the cognitively impaired elderly, or in AD patients (Cattaneo et al., 2017; Vogt et al., 2017). After undergoing fecal microbiota transplantation from a healthy donor, AD patients displayed swift improvement in both memory and mood (Hazan, 2020). These studies locate a causal relationship between gut microbial composition and mental cognition (Chen et al., 2021). However, the mechanism by which gut microbiota affects cognitive function remains unclear. Some scholars have proposed inflammation may be responsible for the cognitive impairment of several chronic diseases, such as depression and insomnia (Strawbridge et al., 2015; Lamers et al., 2019). Depression has been associated with an increase in cytokines secretion (Zou et al., 2018), which can also lead to cognitive impairment affecting attention, learning, and memory (Misiak et al., 2018).
In conclusion, gut microbiota may participate significantly in the occurrence and development of cognitive dysfunction. However, previous studies on the relationship between gut microbes and cognitive function have focused on dementia patients or mouse models, and few studies have explored the connection in MDD patients. In light of these findings and the limitations of previous studies, we hypothesize that compared with HCs, changes in the gut microbiota could be seen in the first-episode untreated MDD group. Furthermore, dysbiosis of gut microbiota may be related to inflammatory factors and cognitive function in MDD patients.
Materials and Methods
Participants
A total of 66 first-episode, drug-naive MDD subjects between 18 and 55 years of age were taken from the inpatient and outpatient units of the First Hospital of Shanxi Medical University Department of Psychiatry during the period of December 2019 to July 2021. Each patient’s symptom was in accordance with the criteria for MDD presented by the Diagnostic and Statistical Manual of Mental Disorders-Fourth Edition (DSM-IV). We used HAMD-17 to measure the severity of depression, and scores of 17 or higher were accepted for the study (Hamilton, 1960). In order to screen for manic or hypomanic episodes, or for other psychiatric disorders, we used the Mini International Neuropsychiatric Interview (MINI) (Sheehan et al., 1998). Subjects found with the following conditions were excluded from the study: (1) autoimmune diseases, heart diseases, hepatobiliary and gastrointestinal diseases, blood diseases, diabetes, neurological diseases, mental retardation, or other psychiatric diseases; (2) pregnancy or lactation; (3) treatment in the last month with antibiotics or anti-inflammatory agents; (4) or had taken probiotics in the past 2 months. To recruit HCs, advertisements were used throughout the local community, with a total of 43 HCs gathered. HCs underwent the same exclusion criteria as MDD subjects. Participants from both groups submitted written informed consent to participate. This research project was approved by the Research Ethics Review Board of First Hospital of Shanxi Medical University in Taiyuan, China.
Cognitive Function Assessment
We implemented the Polish version of the Color Trail Test (CTT) created by Łojek and Stańczak (Tyburski et al., 2020) to evaluate participants’ cognitive flexibility. The CTT is a culture-free form of the Trail Making Test (Reichenberg, 2010) and is made up of two parts: (a) CTT 1, during which the participants are asked to connect a series of 25 numbered circles randomly printed on paper, and (b) CTT 2, during which the participants are asked to connect circles numbered from 1 to 25, and that alternate in color between pink and yellow.
The Repeatable Battery for the Assessment of Neuropsychological Status (RBANS) (Randolph et al., 1998) is frequently implemented to assess cognitive function in cases of dementia, and it consists of 12 subtests. These measure five indices: Immediate Memory, Visuospatial/Constructional, Language, Attention, and Delayed Memory. The indices are scored using a composite score; as such, this study analyzes subtest scores as well as composite scores. To measure the battery, individual subtest scores were converted into age-corrected index scores, and a sum of the index scores was valued as the total score.
The Stroop Color-Word Test (SCWT) was used to measure inhibitory functions (Stroop, 1935). We used the classical version of the SCWT to evaluate cognitive inhibition (dominant verbal response; Stroop, 1935). In the first task (word), a patient is asked to rapidly read color words aloud (e.g., green, blue, yellow, red); the colors are printed in black ink on a standard white A4 sheet. The second task (color) requires the patient to verbally identify the colors of rectangles printed on a standard white A4 sheet. For the third task (word-color), the patient is asked to quickly read aloud the color words printed in mismatched colors (e.g., text reading “yellow” but written in red). In the fourth task (color-word), the patient must quickly name the color in which each word is printed, ignoring its word information. Researchers verbally expressed to the patients the instructions before each of the tasks. Based on Stroop (1935), the naming time was scored in all tests.
Collection of Fecal and Blood Samples
After patients completed the SCWT, we collected stool samples (2 g) and immediately froze them at −80°C for the gut microbiota assay. To identify gut microbial communities, we implemented the 16S ribosomal RNA (16S rRNA) gene sequence-based approach. We collected 10 ml of blood using normal aseptic techniques, and we separated the plasma using centrifugation at 3500 × g at 4°C for 10 min. We stored it at −80°C to measure inflammatory parameters, including hs-CRP, IL-1β, IL-6, IL-10, and TNF-α via enzyme-linked immunosorbent assay (ELISA).
Fecal Sample Collection, DNA Extraction, 16S rRNA Gene Sequencing, and Bioinformatics Data Analysis
The collection of fecal DNA was implemented by the use of the QIAamp® DNA Stool Mini Kit (Qiagen, Hilden, Germany), following the maker’s instructions. By utilizing a NanoDrop One spectrophotometer (Thermo Fisher Scientific, Fitchburg, WI, United States), we quantified the DNA and then measured the integrity and size using 0.8% agarose gel electrophoresis. In order to amplify the 16S rRNA gene of bacteria, we utilized isolated DNA following the 338F universal primers (5′-ACTCCTACGGG AGGCAGCA-3′) and 806R (5′-GGACTACHVGGGTWTCTAAT-3′), focusing on the hypervariable regions V3-V4 of bacterial 16S rRNA. The Illumina Novaseq PE250 platform by Personal Biotechnology, Co., Ltd. was used to conduct PCR amplification and preparation of sequencing library (Shanghai, China).
The open-source software QIIME 2 (version: 2019.1) was used to process the 16S rRNA raw sequencing data (Bolyen et al., 2019). We demultiplexed the sequences and removed the V3/V4 primers with cutadapt (v2.8). We also implemented the DADA2 plugin package for the amplicon workflow: quality filtering, sequence truncation, denoise (error correction), sample inference, merging of paired-end reads, chimera identification and removal, singletons removal, and dereplication of sequences into amplicon sequence variants (ASV) with 100% sequence similarity (Callahan et al., 2016). Any OTUs found to have a frequency of <0.1% of the total number of reads were excluded. A Naive-Bayes classifier trained against the SILVA 132 database (Quast et al., 2013) was used to perform taxonomic classification, targeting the V3/V4 region of the 16S rRNA. Using QIIME 2 (version: 2019.1), we calculated the alpha and beta diversity metrics. Alpha diversity indices of the Abundance-based Shannon index, Simpson index, Observed Species index, Chao index, Faith’s PD index, Pielou’s evenness index, and Good’s coverage index were taken based on rarefied sequence count. An unsupervised principal coordinates analysis (PCoA) of Jaccard dissimilarity was used to conduct beta diversity analysis in order to identify variances of microbiome composition profiles at the ASV level. Linear discriminant analysis effect size (LEfSe) analysis (Segata et al., 2011) was implemented to locate distinguishing taxa in MDD and HCs at multiple levels, and to conceptualize the results in a cladogram and bar plot. We used Spearman’s correlation coefficient to create the correlation matrix among microbiome composition in addition to cytokines and cognitive function. The online genescloud platform1 was used to generate a heat map of the correlation matrix. To find which gut microbiota could act as biomarkers for discriminating between MDD patients and HCs at the genus/ASV level, we applied a Random Forest (RF) and Boruta machine learning algorithm (Speiser et al., 2019), and the region below the receiver operating characteristic curve (AUC) was utilized to measure the classification performance.
Statistical Analyses
All of the analyses carried out in this study were undertaken using IBM SPSS Statistics Version 23.0 (SPSS-23). Two-sample t-tests were conducted to uncover distinctions in age, total years of education, total HAMD-17 scores, and the cognitive function scores of participants. X2 was used to estimate group differences in gender. The threshold of statistical significance was P < 0.05 (two-tailed). To determine the correlation coefficient between the gut microbiota and inflammatory parameters and clinical symptoms, either Pearson or Spearman correlation analyses were implemented.
Results
Clinical Characteristics and Inflammatory Parameters
We uncovered no significant discrepancies in the age, gender, or BMI of the MDD patients and HCs (P > 0.05). Patients with MDD had significantly fewer years of education than HCs (P < 0.001). The MDD group had higher total HAMD-17 scores than the HC group (P < 0.001). MDD patients spent significantly longer at the CCT1 and CCT2 stages than HCs (P < 0.05). In RBANS, healthy subjects scored significantly higher on Immediate Memory, Language, Attention, Delayed Memory, Total score, and Standardized score than MDD patients (P < 0.05). The score of Visuospatial/Constructional was not significantly different between the two groups (P > 0.05). In the Stroop Color/Word task, the MDD group showed longer Word time, Color time, and Color-Word time (P < 0.05); however, we uncovered no significant difference between the groups’ Word-Color times (P > 0.05).
We detected higher levels of hs-CRP in the MDD group than in the HCs group (P < 0.05); however, IL-1β, IL-6, IL-10, and TNF-α did not demonstrate any statistically noteworthy differences between the two groups (P > 0.05) (Table 1).
Gut Microbiota Composition Between Major Depressive Disorder Patients and Healthy Control
We analyzed the microbiomes of 81 total participants (40 MDD and 41 HC) whose stool samples were collected and obtained 5,661,964 high-quality reads across all samples (The raw data is available on NCBI, under the accession number PRJNA776170). These reads were clustered into 37,900 ASVs at 99% sequence similarity. The Venn diagram shows that 4623 ASVs were found in the two groups, while 16,442 and 16,835 ASVs were unique to MDD patients and HCs, respectively (Figure 1). At the level of phylum, these ASVs are mainly concentrated in Firmicutes, Bacteroidetes, Proteobacteria, and Actinobacteria.
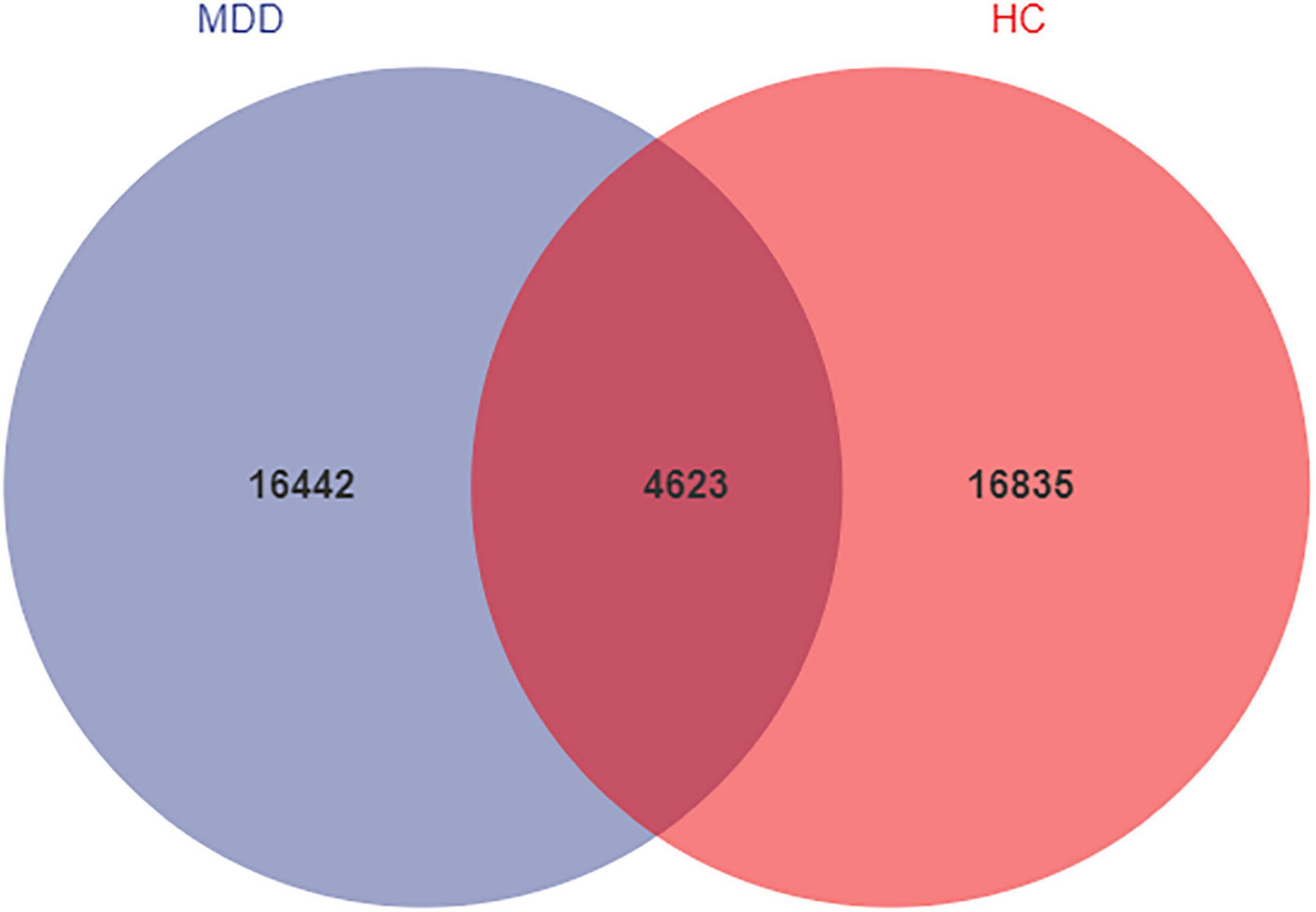
Figure 1. Venn diagram of ASV in MDD and HCs. The blue and red colors represent MDD group and HC group, respectively. The overlapping area between the color blocks indicates the common ASV between two groups, and the number of each block indicates the number of ASV.
Alpha-diversity analysis exposed that both the Simpson index and Pielou’s evenness were lower in MDD patients than in HCs (Figure 2). These findings propose that the microbiota of MDD patients is less diverse than that of HCs.
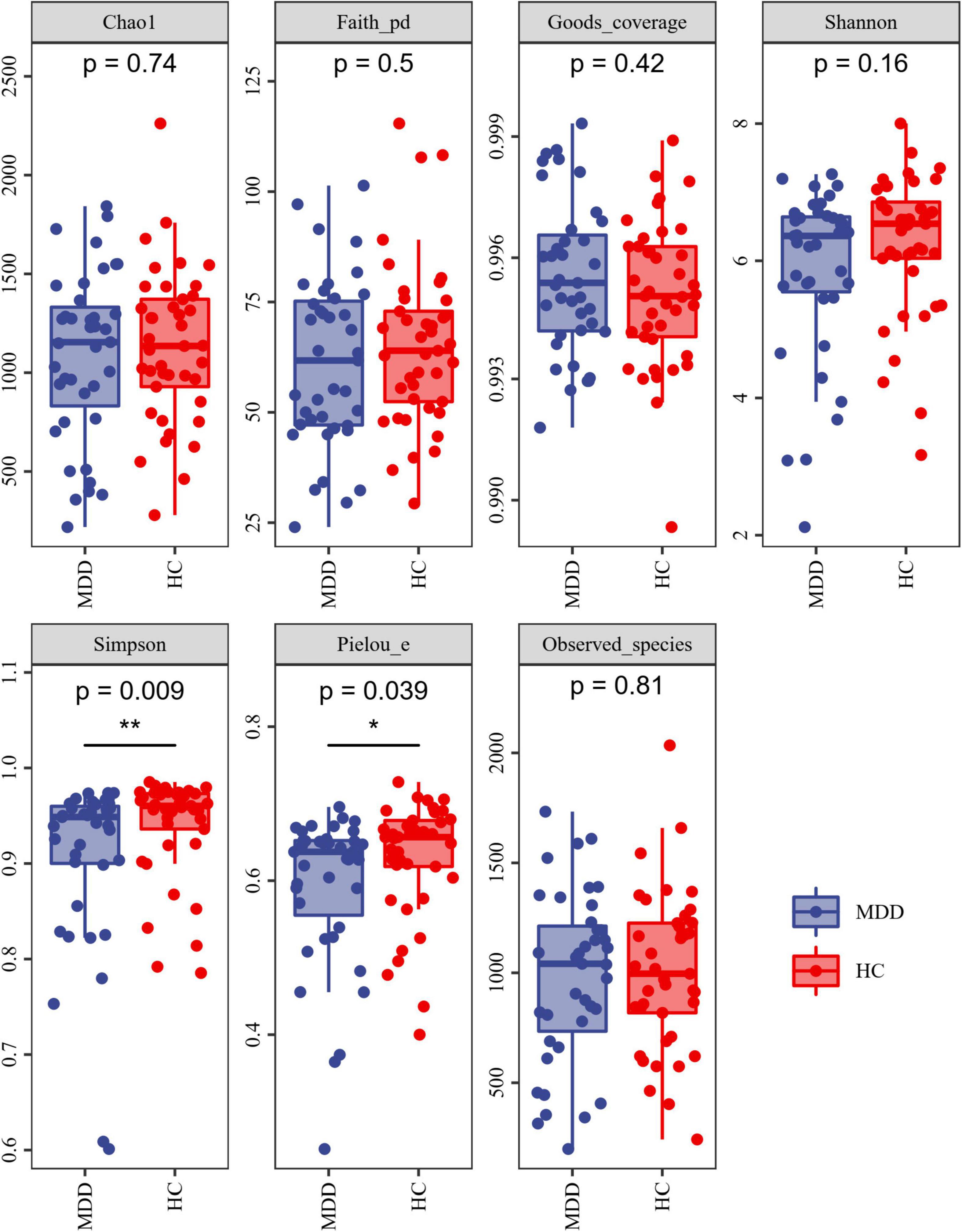
Figure 2. Alpha diversity of MDD and HCs samples. The blue and red colors represent MDD group and HC group, respectively. *P < 0.05 and **P < 0.01.
Beta diversity analysis uncovered a notable difference in bacterial community composition among MDD subjects and HCs as found by the Jaccard dissimilarity calculations (Figure 3).
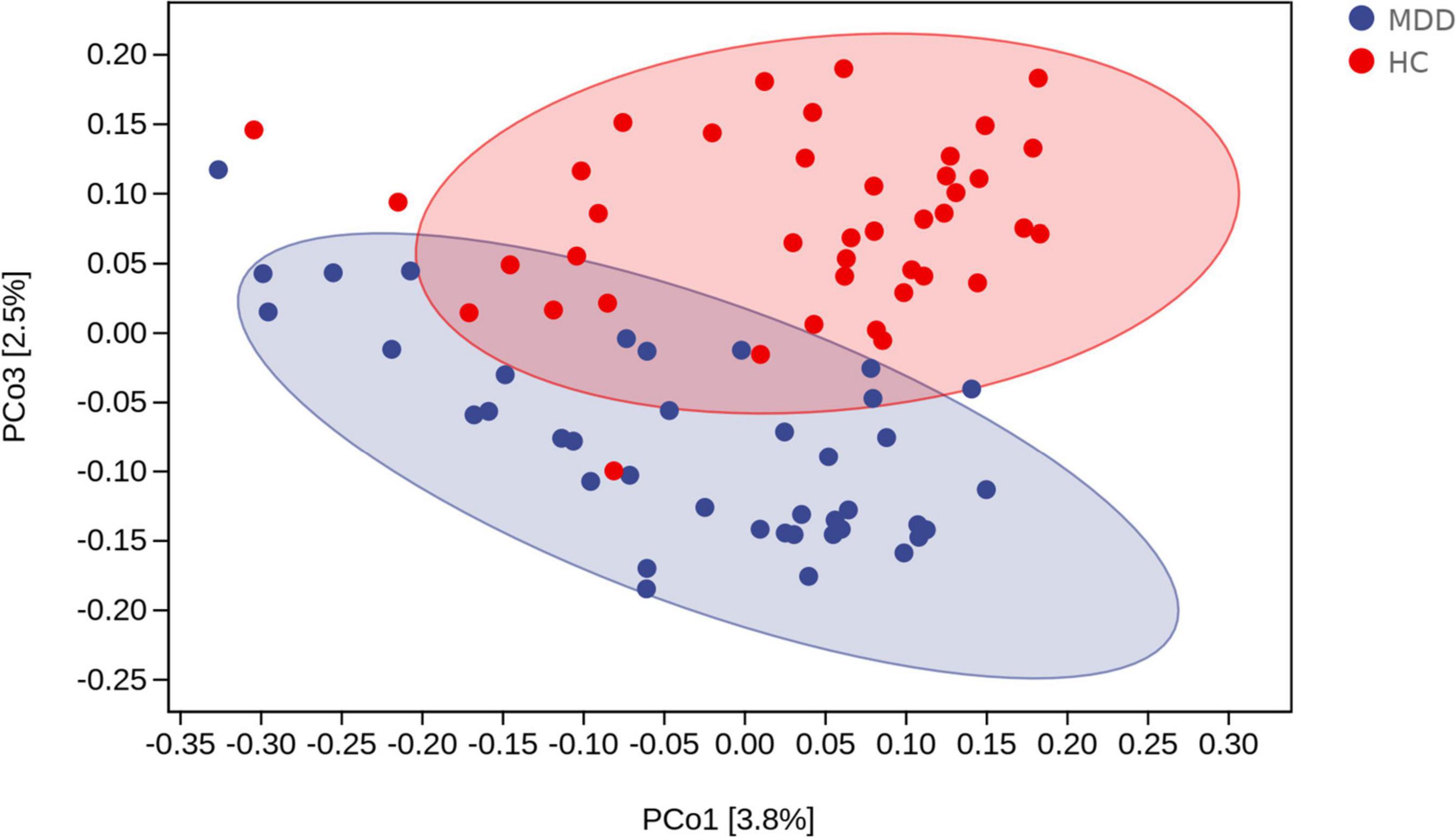
Figure 3. Beta diversity as a principal coordinate analysis (PCoA) plot based on Jaccard dissimilarity. The blue and red colors represent MDD group and HC group, respectively.
A linear discriminant analysis (LDA) effect size (LEfSe) test was adopted to investigate the microbiota discrepancies between MDD patients and HCs. At the family level, the relative abundance of Deinococcaceae was significantly higher in MDD patients; however, the amount of Bacteroidaceae, Turicibacteraceae, Clostridiaceae, and Barnesiellaceae were significantly higher in HCs (Figure 4). At the genus level, the relative abundance of Deinococcus and Odoribacter was significantly higher in MDD patients; however, the abundance of Bacteroides, Alistipes, Turicibacter, Clostridium, Roseburia, and Enterobacter were much higher in HCs (Figure 4).
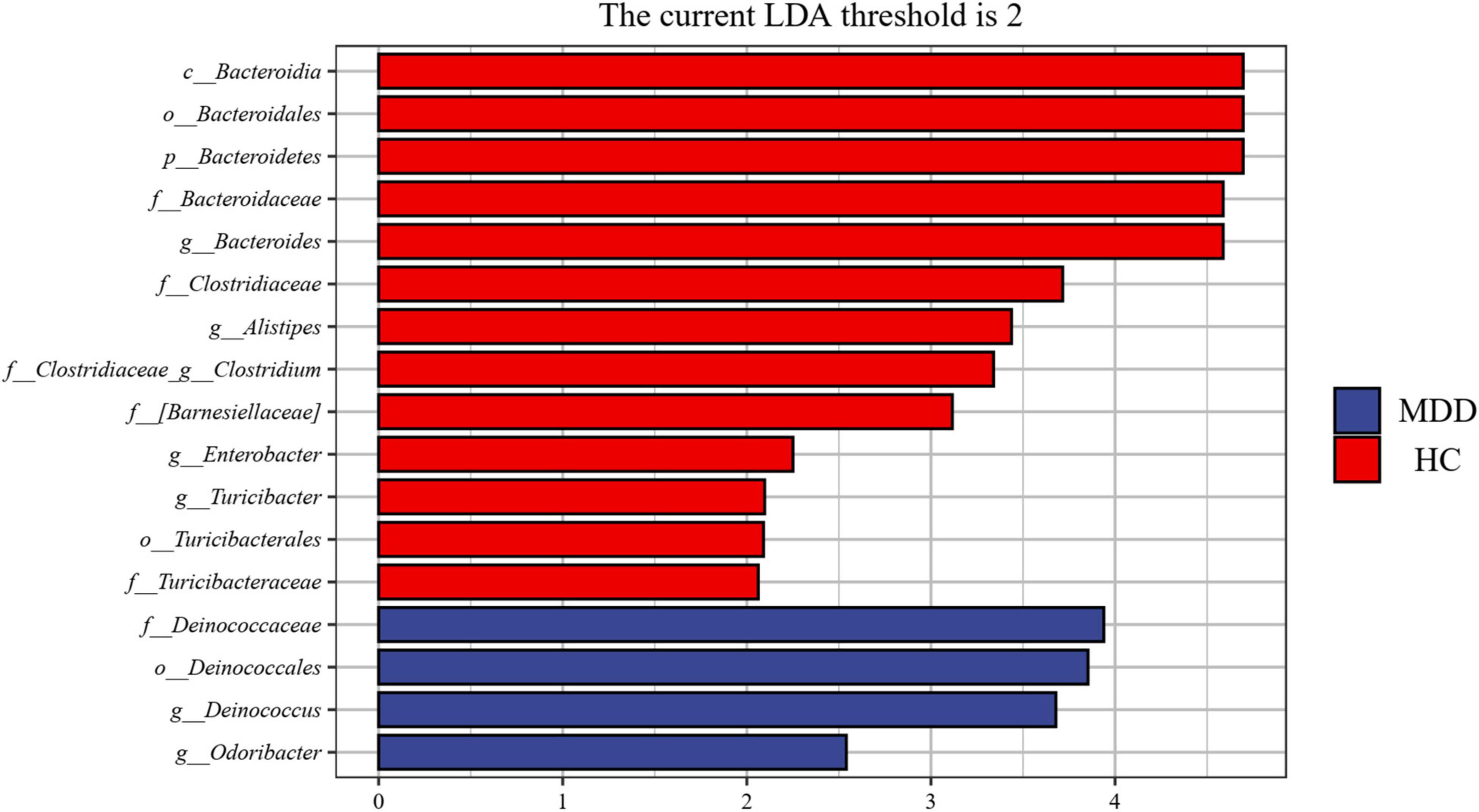
Figure 4. Taxonomic biomarkers found by LEfSe in MDD and HC. Blue and red colors represent MDD and HC enriched family or genus, respectively. Only taxa with p < 0.05 and LDA score (log2) are shown.
To determine the biomarkers for discriminating between MDD patients and HCs at the genus level, we applied a RF and Boruta machine-learning algorithm, which found 20 important genera. We then executed a stepwise regression analysis based on the relative abundance of altered genus. This examination revealed that the most pronounced discrepancies between MDD and HC subjects were due to the relative abundance of Turicibacter, Clostridium, Bacteroides, Sphingomonas, and SMB53. The ROC analysis showed that the AUC was 0.656, 0.633, 0.635, 0.601, and 0.596, separately. When combining these indicators, the AUC was 0.859. At the ASV level, and while employing the same method, we found the most significant discrepancies between MDD and HC subjects were due to the relative abundance of ASV-24439 (Faecalibacterium prausnitzii), ASV-40152 (unidentified_Bacteroides) and ASV-57529 (unidentified_Roseburia). The ROC analysis showed that the AUC was 0.780, 0.805, and 0.768, separately. When combining the three indicators, the AUC was 0.963 (Figure 5).
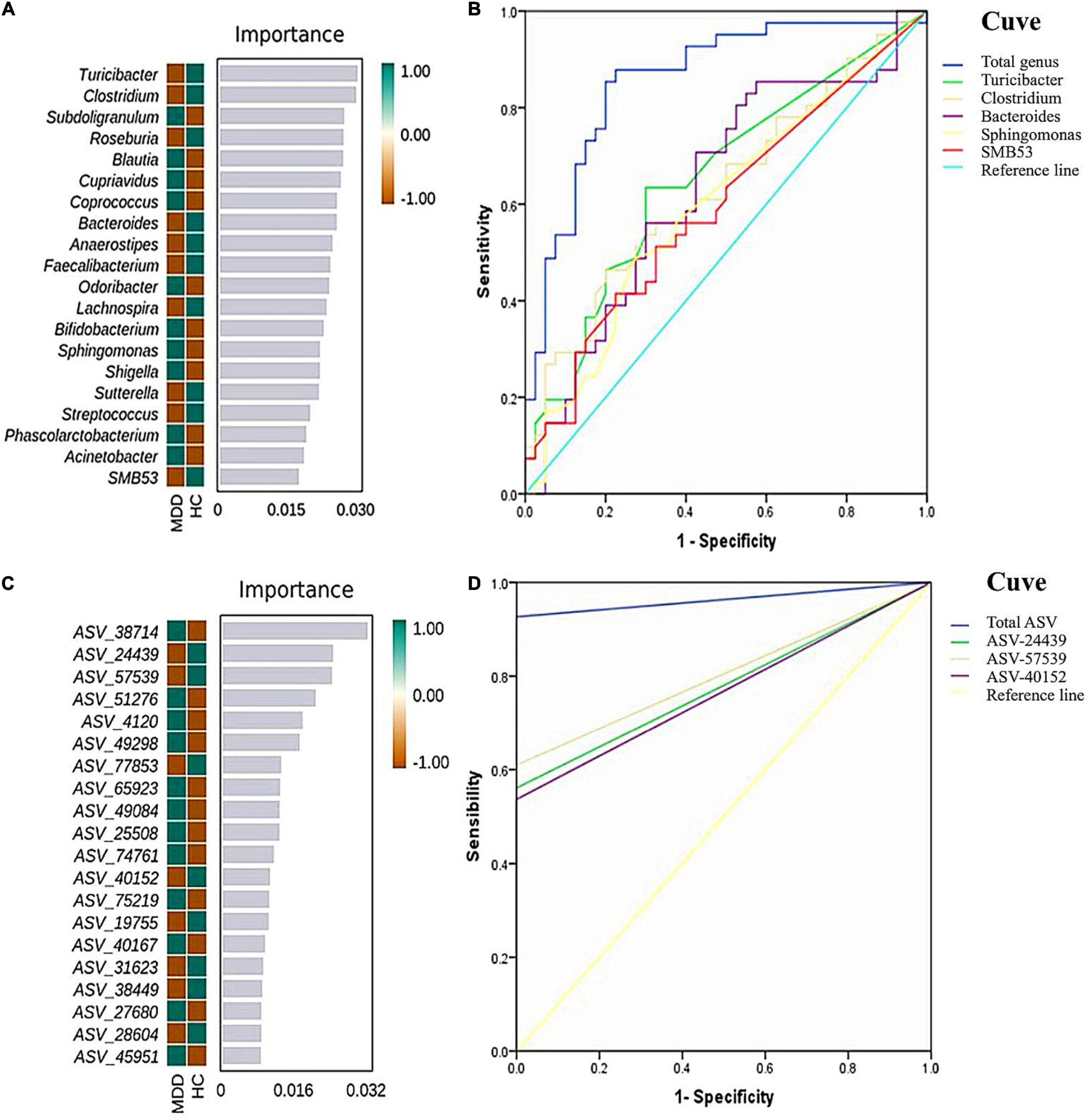
Figure 5. Random forest (RF) classification for gut microbiota in the two groups. The important scores of the confirmed attributes are presented at the genus level (A) and ASVs level (C), respectively. The receiver operating characteristic (ROC) curves were made by single and combined (total genus/ASVs) microorganism at the genus level (B) and ASVs level (D), respectively.
Relationship Between Microbial Biomarkers and Inflammatory Parameters, Depressive Symptoms, and Cognitive Function
To identify the key microbiota, we performed correlation analysis and found that relative abundance of Clostridiaceae and Turicibacter were negatively correlated with the total score HAMD-17 in all subjects (Figure 6). In MDD patients, the relative abundance of Bacteroidaceae and Bacteroides were both positively correlated with hsCRP and cognitive function, such as CCT1 and CCT2. The relative abundance of Alistipes was positively correlated with IL-6, Word time, Color time, Word-Color time, and Color-Word time, and negatively correlated with Delayed Memory, Total score, and Standardized score. The relative abundance of Deinococcus was positively correlated with the Color-Word time, and negatively correlated with Visuospatial/Constructional, Language, Delayed Memory, Total score, and Standardized score in RBANS. The relative abundance of Turicibacteraceae and Turicibacter were both negatively correlated with IL-1β and IL-6 (Figure 7).
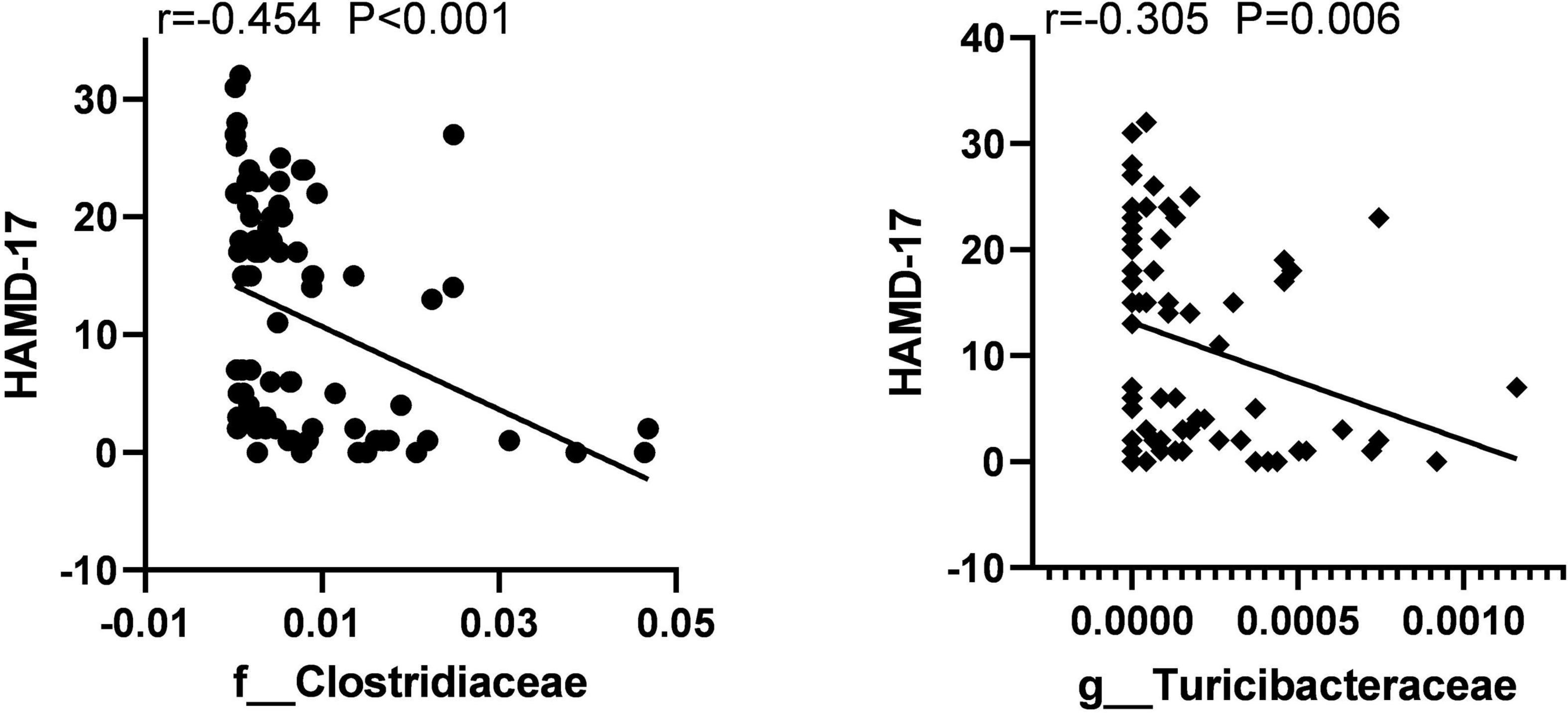
Figure 6. The scatter plot of correlation between Clostridiaceae, Turicibacteraceae abundance, and the HAMD-17 scores in two groups.
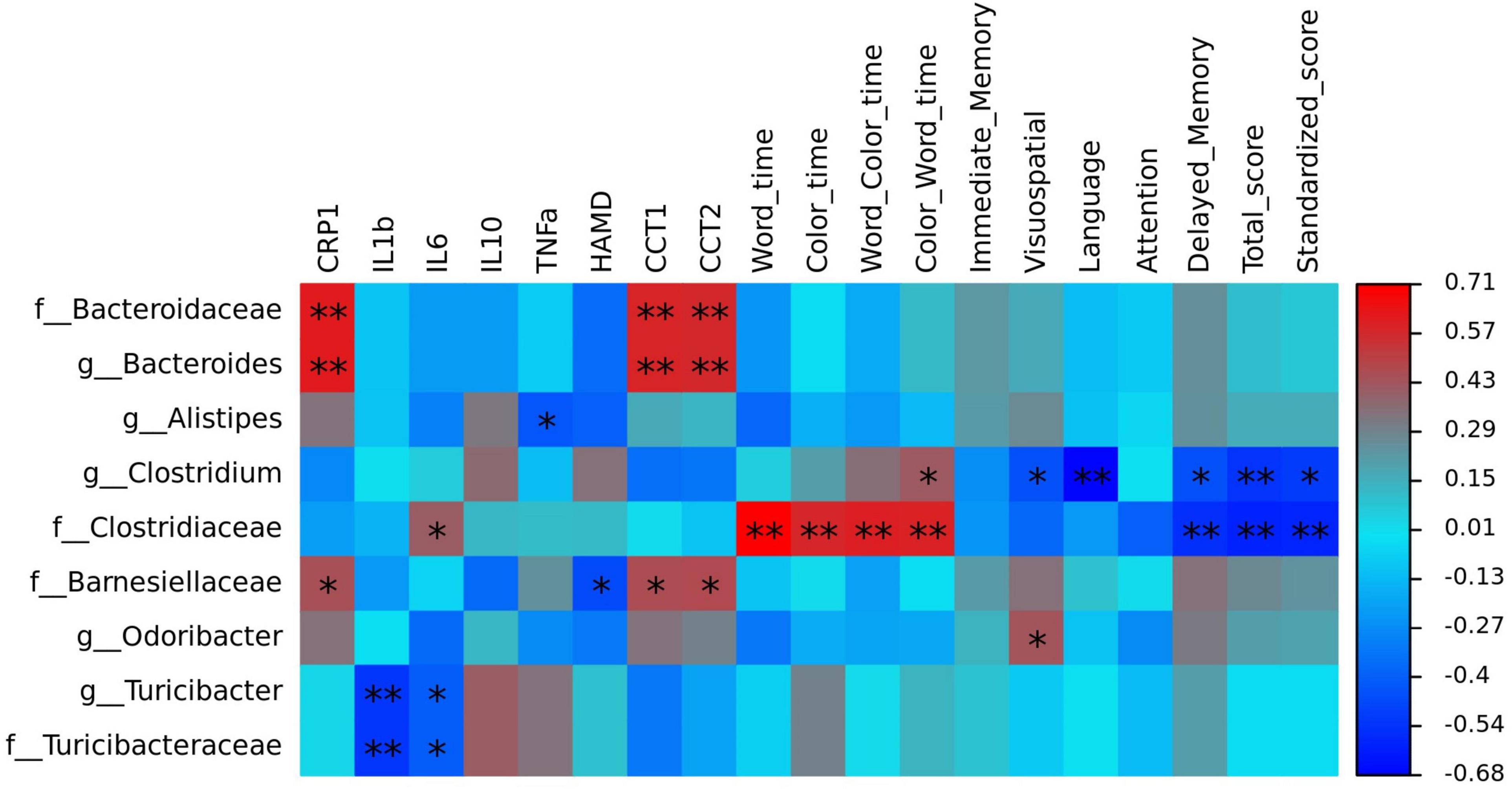
Figure 7. The heatmaps of spearman correlation coefficient matrix between significant different gut bacteria and inflammatory factors, cognitive function. Red indicates positive correlations, while blue indicates negative. *P < 0.05 and **P < 0.01. Note: HAMD, Hamilton’s Depression Scale; CRP, C-reactive protein, IL-1β, interleukin 1β; IL-6, interleukin 6; IL-10, interleukin 10; TNF-α, tumor necrosis factor α; CTT, Color Trail Test.
Discussion
In our examination, we found a significant cognitive decline in MDD patients compared to healthy subjects. Our findings support the findings of previous research. MDD patients more commonly demonstrate cognitive disturbances than the general population (Pan et al., 2019). Although a disturbance of mood is the traditional definition of MDD, impaired cognitive function is often considered a result of the disorder (Austin et al., 2001). We define cognitive function as the mental processes of receiving, using, and preserving information, and these are sorted into fields like attention and memory (Ahern and Semkovska, 2017). Many researchers have also uncovered cognitive deficits in processing speed, attention, learning abilities, long-term memory, autobiographical memory, and executive function. For example, a meta-analysis of 24 studies, one comprised of 784 MDD patients, quantified moderate cognitive deficits in executive function, memory, and attention of MDD patients relative to HCs (Snyder, 2013; Rock et al., 2014). The state of knowledge at the moment proposes that cognitive impairment in MDD patients is an important determining factor of a patient’s individual functional outcomes. Cognitive impairment has been observed to remain present after MDD remission, to decline with recurrent depressive episodes, and to serve as an important predictor of relapse (Reppermund et al., 2009). Furthermore, previous research has proposed that cognitive remission should serve as a therapeutic target for restoring, functioning, and preventing relapse (Bortolato et al., 2016).
Previous studies have identified depression as linked to differences in the gut microbiota of patients, and our study contributes to this body of work (Kelly et al., 2016; Huang et al., 2018; Liśkiewicz et al., 2021; Yuan et al., 2021). Here, we found Alpha-diversity and Beta-diversity were different between MDD subjects and HCs. However, a number of studies have also reported different conclusions (Naseribafrouei et al., 2014; Jiang et al., 2015).
We discovered that gut microbiota was significantly different between MDD and HCs at the family level and genus level.
Some of our results were congruent with outcomes of previous studies. Zhang et al. (2021) found decreasing Clostridiaceae and Alistipes in MDD, and that the severity of depression correlated with bacterial composition. Zheng et al. (2016) found that the families Bacteroidaceae and Lachnospiraceae and the genera Alistipes and Clostridium XlVa were overrepresented in HCs. Another study noted that the overall content of Firmicutes was dramatically decreased in the MDD group than in the HC group; at the family level, Clostridiaceae were decreased; and at the genus level, Clostridium and Alistipes were decreased (Huang et al., 2018). However, contrary to our findings, some studies showed that the relative proportions of Bacteroides, Turicibacter, and Clostridium were increased in the depressed group, whereas Dialister was decreased (Jiang et al., 2015; Kelly et al., 2016; Liu et al., 2020).
Correlation analysis showed that Clostridiaceae and Turicibacter were negatively correlated with the total HAMD-17 score in all subjects. In MDD patients, Bacteroidaceae and Bacteroides were both positively correlated with hsCRP, CCT1, and CCT2. Alistipes was positively correlated with IL-6, Word time, Color time, Word-Color time, and Color-Word time, and negatively correlated with Delayed Memory, Total score, and Standardized score. Deinococcus was positively correlated with the Color-Word time and negatively correlated with Visuospatial/Constructional, Language, Delayed Memory, Total score, and Standardized score in RBANS. Turicibacteraceae and Turicibacter were both negatively correlated with IL-1βand IL-6.
Currently, studies on the correlation between cognitive impairment and gut microbes have focused on AD. Many studies have found that neuroinflammation plays an important role in cognitive impairment. Gut microbiota could affect intestinal permeability and trigger systemic proinflammatory cytokines. Systemic inflammation could accelerate cognitive impairment by acting synergistically (Calsolaro and Edison, 2016; Li et al., 2019). Bacteroides are commensal and Gram-negative, and are some of the most copious bacteria present in the human gastrointestinal system. Certain strains of the Bacteroidetes species are considered generally advantageous to human health because of their many abilities (Sears, 2009). However, when people experience stress such as shock, abuse, the loss of a family member, etc., certain strains of Bacteroidetes species such as Bacteroides fragilis can secrete lipopolysaccharide (LPS), bacterial amyloids, endotoxins (such as fragilysin) and exotoxins. These neurotoxins stimulates the release of TNF-α, IL-1β, IL-8, gamma interferon (IFN-γ), CXC ligand 8 (CXCL8) and other inflammatory cytokines and chemokines in various cell types, leading to inflammatory response toward these bacterial molecular pathogens (Lukiw, 2016; Alexandrov et al., 2020). Activated inflammatory responses can disrupt both the intestinal mucosal barrier as well as the BBB, and further, they can activate the microglia of CNS (Alexandrov et al., 2020). Activated microglia were found to participate in the secretion of pro-inflammatory cytokines, including IL-1β, IL-6, TNF-α, and TGF-β, thus aiding the development of cognitive impairment in individuals with neurological disorders (Giau et al., 2018). Alistipes is also pro-inflammatory bacteria. Hennezel et al. (2017) revealed that the levels of Alistipes putredinis and Alistipes finegoldii possess a moderate-to-strong correlation with IL-6, TNF-α and IL-1 production. Another study showed that the Alistipes genus participated in prompting inflammation and tumorigenesis in an IL-6-dependent manner (Moschen et al., 2016). However, there was no significant correlation between Alistipes and IL-1, IL-6 in this study, and a negative correlation between Alistipes and TNF-α. Therefore, Alistipes can aggravate cognitive impairment by other ways than inflammatory response.
We found that Clostridiaceae and Turicibacter were both negatively correlated with IL-1β, IL-6, TNF-α, and HAMD-17 scores. These bacteria participate in the production of SCFAs such as butyrate, acetic acid, and valeric acid. SCFAs demonstrate anti-inflammatory functions, regulate the differentiation of T-lymphocytes, function as energy sources for the intestinal epithelium, and act as a key regulator for proper intestinal permeability (Haghikia et al., 2015). Furthermore, butyrate can cross the BBB and disrupt the function of the hippocampus, in addition to promoting BDNF expression, which has previously been found to cause antidepressant-like effects in animal models (Soret et al., 2010). Consequently, insufficient butyrate-producing bacteria in MDD subjects may influence MDD’s pathology.
In conclusion, this study found that some increased pro-inflammatory bacteria and some decreased anti-inflammatory bacteria in MDD patients are associated with inflammatory factors and cognitive function. Gut microbiota may be the cause of cognitive impairment in patients with MDD. Environmental and genetic factors cause changes in gut microbiota, and altered gut microbiota promotes inflammatory responses in the intestinal, peripheral blood and CNS, which in turn leads to cognitive impairment. However, an altered gut microbiota can also be a result of depressive symptoms and impaired cognitive function. Changes to lifestyle, eating habits, and behavior that may cause dysbiosis of gut microbes can accompany/precede diagnosis of MDD. One limitation of this study is that we conducted only correlation analyses, thus is difficult to determine the causal relationship between gut microbes and cognitive impairment, which would require to be verified through animal experiments. In addition, an inflammation response may be one of the mechanisms of the interaction between gut microbiota and the CNS. The imbalance of neuroendocrine and metabolism should also be a concern.
Our study submits a prediction model for microbial biomarkers at the genus and ASV levels, which showed high accuracy (AUC = 0.859 and 0.963) signifying an effective classification model. According to previous studies, gut microbes can be used as biomarkers for distinguishing MDD patients form HCs. Lai et al. (2021) created an RF classification model based on genus levels with an AUC of 0.890. Further, their RF classification model based on species levels attained a higher AUC than their genus level model, achieving 0.997. It is commonly accepted that AUC scores can be taken as a ‘single number’ measure to evaluate and compare classifiers, where scores of <0.70 are considered poor, 0.70–0.79 are considered fair, 0.80–0.89 are considered good, and 0.90–1.00 are considered excellent (Cicchetti, 2001). The AUC score of the model used here was calculated as 0.86 and 0.96, thereby indicating good discrimination and robustness.
Compared with previous studies, there are many inconsistent results. The heterogeneity of the microbiome research is understood to be connected to various factors that been stated in limitation.
Our study has certain limitations. One limitation is that we used a sample size that was too small to eliminate the chance of false positives. Second, we applied 16S rRNA sequencing, which only detects taxa at the genus level. Therefore, there may have been key associations at the species or strain levels that were overlooked. Third, dietary structure is understood to significantly impact the composition and function of the gut microbiota. In the present study, we did not collect complete dietary data, which could have biased our results. Fourth, geographical distribution may be a potential confounder, which could affect the composition of gut microbiota. The generalization of our results needs additional corroboration in more regions of China, and in other countries, as well.
Conclusion
This study demonstrates that compared to HCs, MDD patients showed impaired cognitive function and had a significant abnormal gut microbiota composition, and increased CRP. Altered gut microbiota is closely associated with inflammatory factors and cognitive function in MDD patients.
Data Availability Statement
The original contributions presented in the study are publicly available. This data can be found here: https://www.ncbi.nlm.nih.gov/search/all/?term=PRJNA776170.
Ethics Statement
The studies involving human participants were reviewed and approved by Ethical Committee of the First Hospital of Shanxi Medical University. The patients/participants provided their written informed consent to participate in this study. Written informed consent was obtained from the individual(s) for the publication of any potentially identifiable images or data included in this article.
Author Contributions
KZ and NS designed the experiments. MG, YZ, HT, LL, PW, AZ, CY, and ZL participated in the collection and analysis of clinical data, stool samples, and blood samples of all subjects. PL analyzed data and wrote the manuscript. All authors contributed to the clinical data collection and assessment.
Funding
This study was financially supported by the National Natural Science Youth Fund Project (82001802 and 81601192).
Conflict of Interest
The authors declare that the research was conducted in the absence of any commercial or financial relationships that could be construed as a potential conflict of interest.
Publisher’s Note
All claims expressed in this article are solely those of the authors and do not necessarily represent those of their affiliated organizations, or those of the publisher, the editors and the reviewers. Any product that may be evaluated in this article, or claim that may be made by its manufacturer, is not guaranteed or endorsed by the publisher.
Footnotes
References
Ahern, E., and Semkovska, M. (2016). Cognitive functioning in the first-episode of major depressive disorder: a systematic review and meta-analysis. Neuropsychology 31, 52–72. doi: 10.1037/neu0000319
Ahern, E., and Semkovska, M. (2017). Cognitive functioning in the first-episode of major depressive disorder: a systematic review and meta-analysis. Neuropsychology 31, 52–72.
Alexandrov, P. N., Hill, J. M., Zhao, Y., Bond, T., Taylor, C. M., Percy, M. E., et al. (2020). Aluminum-induced generation of lipopolysaccharide (LPS) from the human gastrointestinal (GI)-tract microbiome-resident Bacteroides fragilis. J. Inorg. Biochem. 203:110886. doi: 10.1016/j.jinorgbio.2019.110886
Austin, M. P., Mitchell, P., and Goodwin, G. M. (2001). Cognitive deficits in depression: possible implications for functional neuropathology. Br. J. Psychiatry 178, 200–206. doi: 10.1192/bjp.178.3.200
Bolyen, E., Rideout, J. R., Dillon, M. R., Bokulich, N. A., Abnet, C. C., Al-Ghalith, G. A., et al. (2019). Author correction: reproducible, interactive, scalable and extensible microbiome data science using QIIME 2. Nat. Biotechnol. 37:1091. doi: 10.1038/s41587-019-0252-6
Bortolato, B., Miskowiak, K. W., Köhler, C. A., Maes, M., Fernandes, B. S., Berk, M., et al. (2016). Cognitive remission: a novel objective for the treatment of major depression. BMC Med. 14:9. doi: 10.1186/s12916-016-0560-3
Breit, S., Kupferberg, A., Rogler, G., and Hasler, G. (2018). Vagus nerve as modulator of the brain-gut axis in psychiatric and inflammatory disorders. Front. Psychiatry 9:44. doi: 10.3389/fpsyt.2018.00044
Bruce-Keller, A. J., Salbaum, J. M., and Berthoud, H. R. (2018). Harnessing gut microbes for mental health: getting from here to there. Biol. Psychiatry 83, 214–223. doi: 10.1016/j.biopsych.2017.08.014
Callahan, B. J., McMurdie, P. J., Rosen, M. J., Han, A. W., Johnson, A. J., and Holmes, S. P. (2016). DADA2: high-resolution sample inference from Illumina amplicon data. Nat. Methods 13, 581–583. doi: 10.1038/nmeth.3869
Calsolaro, V., and Edison, P. (2016). Neuroinflammation in Alzheimer’s disease: current evidence and future directions. Alzheimer’s Dement. 12, 719–732. doi: 10.1016/j.jalz.2016.02.010
Cattaneo, A., Cattane, N., Galluzzi, S., Provasi, S., Lopizzo, N., Festari, C., et al. (2017). Association of brain amyloidosis with pro-inflammatory gut bacterial taxa and peripheral inflammation markers in cognitively impaired elderly. Neurobiol. Aging 49, 60–68. doi: 10.1016/j.neurobiolaging.2016.08.019
Chen, Y., Xu, J., and Chen, Y. (2021). Regulation of neurotransmitters by the gut microbiota and effects on cognition in neurological disorders. Nutrients 13:2099. doi: 10.3390/nu13062099
Cicchetti, D. V. (2001). The precision of reliability and validity estimates re-visited: distinguishing between clinical and statistical significance of sample size requirements. J. Clin. Exp. Neuropsychol. 23, 695–700. doi: 10.1076/jcen.23.5.695.1249
Desbonnet, L., Clarke, G., Traplin, A., Sullivan, O., Crispie, F., Moloney, R. D., et al. (2015). Gut microbiota depletion from early adolescence in mice: implications for brain and behaviour. Brain Behav. Immun. 48, 165–173. doi: 10.1016/j.bbi.2015.04.004
Dickerson, F., Severance, E., and Yolken, R. (2017). The microbiome, immunity, and schizophrenia and bipolar disorder. Brain Behav. Immun. 62, 46–52. doi: 10.1016/j.bbi.2016.12.010
Furusawa, Y., Obata, Y., Fukuda, S., Endo, T. A., Nakato, G., Takahashi, D., et al. (2013). Commensal microbe-derived butyrate induces the differentiation of colonic regulatory T cells. Nature 504, 446–450. doi: 10.1038/nature12721
Gareau, M. G., Wine, E., Rodrigues, D. M., Cho, J. H., Whary, M. T., Philpott, D. J., et al. (2011). Bacterial infection causes stress-induced memory dysfunction in mice. Gut 60, 307–317. doi: 10.1136/gut.2009.202515
Giau, V. V., Wu, S. Y., Jamerlan, A., An, S., Kim, S. Y., and Hulme, J. (2018). Gut microbiota and their neuroinflammatory implications in Alzheimer’s disease. Nutrients 10:1765. doi: 10.3390/nu10111765
Haghikia, A., Jörg, S., Duscha, A., Berg, J., Manzel, A., Waschbisch, A., et al. (2015). Dietary fatty acids directly impact central nervous system autoimmunity via the small intestine. Immunity 43, 817–829. doi: 10.1016/j.immuni.2015.09.007
Hamilton, M. (1960). A rating scale for depression. J. Neurol. Neurosurg. Psychiatry 23, 56–62. doi: 10.1136/jnnp.23.1.56
Hazan, S. (2020). Rapid improvement in Alzheimer’s disease symptoms following fecal microbiota transplantation: a case report. J. Int. Med. Res. 48:300060520925930. doi: 10.1177/0300060520925930
Hennezel, E., Abubucker, S., Murphy, L. O., and Cullen, T. W. (2017). Total lipopolysaccharide from the human gut microbiome silences toll-like receptor signaling. mSystems 2:e00046-17. doi: 10.1128/mSystems.00046-17
Huang, T. T., Lai, J. B., Du, Y. L., Xu, Y., Ruan, L. M., and Hu, S. H. (2019). Current understanding of gut microbiota in mood disorders: an update of human studies. Front. Genet. 10:98. doi: 10.3389/fgene.2019.00098
Huang, Y., Shi, X., Li, Z., Shen, Y., Shi, X., Wang, L., et al. (2018). Possible association of Firmicutes in the gut microbiota of patients with major depressive disorder. Neuropsychiatric Dis. Treatment 14, 3329–3337. doi: 10.2147/NDT.S188340
Jiang, H., Ling, Z., Zhang, Y., Mao, H., Ma, Z., Yin, Y., et al. (2015). Altered fecal microbiota composition in patients with major depressive disorder. Brain Behav. Immun. 48, 186–194. doi: 10.1016/j.bbi.2015.03.016
Kelly, J. R., Borre, Y., Brien, C., Patterson, E., El Aidy, S., Deane, J., et al. (2016). Transferring the blues: depression-associated gut microbiota induces neurobehavioural changes in the rat. J. Psychiatr. Res. 82, 109–118. doi: 10.1016/j.jpsychires.2016.07.019
Lai, W. T., Deng, W. F., Xu, S. X., Zhao, J., Xu, D., Liu, Y. H., et al. (2021). Shotgun metagenomics reveals both taxonomic and tryptophan pathway differences of gut microbiota in major depressive disorder patients. Psychol. Med. 51, 90–101. doi: 10.1017/S0033291719003027
Lamers, F., Milaneschi, Y., Smit, J. H., Schoevers, R. A., Wittenberg, G., and Penninx, B. (2019). Longitudinal association between depression and inflammatory markers: results from the netherlands study of depression and anxiety. Biol. Psychiatry 85, 829–837. doi: 10.1016/j.biopsych.2018.12.020
Li, B., He, Y., Ma, J., Huang, P., Du, J., Cao, L., et al. (2019). Mild cognitive impairment has similar alterations as Alzheimer’s disease in gut microbiota. Alzheimer’s Dement. 15, 1357–1366. doi: 10.1016/j.jalz.2019.07.002
Liśkiewicz, P., Kaczmarczyk, M., Misiak, B., Wroński, M., Ba̧ba-Kubiś, A., Skonieczna-Żydecka, K., et al. (2021). Analysis of gut microbiota and intestinal integrity markers of inpatients with major depressive disorder. Prog. Neuro-psychopharmacol. Biol. Psychiatry 106:110076. doi: 10.1016/j.pnpbp.2020.110076
Liu, R. T., Rowan-Nash, A. D., Sheehan, A. E., Walsh, R., Sanzari, C. M., Korry, B. J., et al. (2020). Reductions in anti-inflammatory gut bacteria are associated with depression in a sample of young adults. Brain Behav. Immun. 88, 308–324. doi: 10.1016/j.bbi.2020.03.026
Liu, Y., Wang, H., Gui, S., Zeng, B., Pu, J., Zheng, P., et al. (2021). Proteomics analysis of the gut-brain axis in a gut microbiota-dysbiosis model of depression. Translational Psychiatry 11:568. doi: 10.1038/s41398-021-01689-w
Lukiw, W. J. (2016). Bacteroides fragilis lipopolysaccharide and inflammatory signaling in Alzheimer’s disease. Front. Microbiol. 7:1544. doi: 10.3389/fmicb.2016.01544
Misiak, B., Beszłej, J. A., Kotowicz, K., Szewczuk-Bogusławska, M., Samochowiec, J., Kucharska-Mazur, J., et al. (2018). Cytokine alterations and cognitive impairment in major depressive disorder: from putative mechanisms to novel treatment targets. Prog. Neuro-Psychopharmacol. Biol. Psychiatry 80, 177–188. doi: 10.1016/j.pnpbp.2017.04.021
Moschen, A. R., Gerner, R. R., Wang, J., Klepsch, V., Adolph, T. E., Reider, S. J., et al. (2016). Lipocalin 2 protects from inflammation and tumorigenesis associated with gut microbiota alterations. Cell Host Microbe 19, 455–469. doi: 10.1016/j.chom.2016.03.007
Naseribafrouei, A., Hestad, K., Avershina, E., Sekelja, M., Linløkken, A., Wilson, R., et al. (2014). Correlation between the human fecal microbiota and depression. Neurogastroenterol. Motility 26, 1155–1162. doi: 10.1111/nmo.12378
Pan, Z., Park, C., Brietzke, E., Zuckerman, H., Rong, C., Mansur, R. B., et al. (2019). Cognitive impairment in major depressive disorder. CNS Spectr. 24, 22–29. doi: 10.1017/S1092852918001207
Petra, A. I., Panagiotidou, S., Hatziagelaki, E., Stewart, J. M., Conti, P., and Theoharides, T. C. (2015). Gut-Microbiota-Brain axis and its effect on neuropsychiatric disorders with suspected immune dysregulation. Clin. Ther. 37, 984–995. doi: 10.1016/j.clinthera.2015.04.002
Quast, C., Pruesse, E., Yilmaz, P., Gerken, J., Schweer, T., Yarza, P., et al. (2013). The SILVA ribosomal RNA gene database project: improved data processing and web-based tools. Nucleic Acids Res. 41, D590–D596. doi: 10.1093/nar/gks1219
Randolph, C., Tierney, M. C., Mohr, E., and Chase, T. N. (1998). The Repeatable Battery for the Assessment of Neuropsychological Status (RBANS): preliminary clinical validity. J. Clin. Exp. Neuropsychol. 20, 310–319. doi: 10.1076/jcen.20.3.310.823
Reichenberg, A. (2010). The assessment of neuropsychological functioning in schizophrenia. Dial. Clin. Neurosci. 12, 383–392. doi: 10.31887/DCNS.2010.12.3/areichenberg
Reppermund, S., Ising, M., Lucae, S., and Zihl, J. (2009). Cognitive impairment in unipolar depression is persistent and non-specific: further evidence for the final common pathway disorder hypothesis. Psychol. Med. 39, 603–614. doi: 10.1017/S003329170800411X
Rhee, S. H., Pothoulakis, C., and Mayer, E. A. (2009). Principles and clinical implications of the brain-gut-enteric microbiota axis. Nat. Rev. Gastroenterol. Hepatol. 6, 306–314. doi: 10.1038/nrgastro.2009.35
Rock, P. L., Roiser, J. P., Riedel, W. J., and Blackwell, A. D. (2014). Cognitive impairment in depression: a systematic review and meta-analysis. Psychol. Med. 44, 2029–2040. doi: 10.1017/S0033291713002535
Sanada, K., Nakajima, S., Kurokawa, S., Barceló-Soler, A., Ikuse, D., Hirata, A., et al. (2020). Gut microbiota and major depressive disorder: a systematic review and meta-analysis. J. Affect. Disord. 266, 1–13. doi: 10.1016/j.jad.2020.01.102
Sears, C. L. (2009). Enterotoxigenic Bacteroides fragilis: a rogue among symbiotes. Clin. Microbiol. Rev. 22, 349–369, Table of Contents. doi: 10.1128/CMR.00053-08
Segata, N., Izard, J., Waldron, L., Gevers, D., Miropolsky, L., Garrett, W. S., et al. (2011). Metagenomic biomarker discovery and explanation. Genome Biol. 12:R60. doi: 10.1186/gb-2011-12-6-r60
Sheehan, D. V., Lecrubier, Y., Sheehan, K. H., Amorim, P., Janavs, J., Weiller, E., et al. (1998). The Mini-International Neuropsychiatric Interview (M.I.N.I.): the development and validation of a structured diagnostic psychiatric interview for DSM-IV and ICD-10. J. Clin. Psychiatry 59(Suppl. 20), 22-33;quiz 34–57.
Snyder, H. R. (2013). Major depressive disorder is associated with broad impairments on neuropsychological measures of executive function: a meta-analysis and review. Psychol. Bull. 139, 81–132. doi: 10.1037/a0028727
Soret, R., Chevalier, J., De Coppet, P., Poupeau, G., Derkinderen, P., Segain, J. P., et al. (2010). Short-chain fatty acids regulate the enteric neurons and control gastrointestinal motility in rats. Gastroenterology 138, 1772–1782. doi: 10.1053/j.gastro.2010.01.053
Speiser, J. L., Miller, M. E., Tooze, J., and Ip, E. (2019). A comparison of random forest variable selection methods for classification prediction modeling. Expert Systems Appl. 134, 93–101. doi: 10.1016/j.eswa.2019.05.028
Strawbridge, R., Arnone, D., Danese, A., Papadopoulos, A., Herane Vives, A., and Cleare, A. J. (2015). Inflammation and clinical response to treatment in depression: a meta-analysis. Eur. Neuropsychopharmacol. 25, 1532–1543. doi: 10.1016/j.euroneuro.2015.06.007
Stroop, J. R. (1935). Studies of interference in serial verbal reactions. J. Exp. Psychol. 18, 643–662. doi: 10.1037/h0054651
Tyburski, E., Mak, M., Samochowiec, A., Plichta, P., Bielecki, M., Rek-Owodziń, K., et al. (2020). The relationship between cingulum bundle integrity and different aspects of executive functions in chronic schizophrenia. Prog. Neuro-psychopharmacol. Biol. Psychiatry 102:109955. doi: 10.1016/j.pnpbp.2020.109955
Vogt, N. M., Kerby, R. L., Dill-McFarland, K. A., Harding, S. J., Merluzzi, A. P., Johnson, S. C., et al. (2017). Gut microbiome alterations in Alzheimer’s disease. Sci. Rep. 7:13537. doi: 10.1038/s41598-017-13601-y
Yang, Z., Li, J., Gui, X., Shi, X., Bao, Z., Han, H., et al. (2020). Updated review of research on the gut microbiota and their relation to depression in animals and human beings. Mol. Psychiatry 25, 2759–2772. doi: 10.1038/s41380-020-0729-1
Yuan, X., Wang, Y., Li, X., Jiang, J., Kang, Y., Pang, L., et al. (2021). Gut microbial biomarkers for the treatment response in first-episode, drug-naïve schizophrenia: a 24-week follow-up study. Transl. Psychiatry 11:422. doi: 10.1038/s41398-021-01531-3
Yong, S. J., Tong, T., Chew, J., and Lim, W. L. (2019). Antidepressive mechanisms of probiotics and their therapeutic potential. Front. Neurosci. 13:1361. doi: 10.3389/fnins.2019.01361
Zhang, Q., Yun, Y., An, H., Zhao, W., Ma, T., Wang, Z., et al. (2021). Gut microbiome composition associated with major depressive disorder and sleep quality. Front. Psychiatry 12:645045. doi: 10.3389/fpsyt.2021.645045
Zheng, P., Zeng, B., Zhou, C., Liu, M., Fang, Z., Xu, X., et al. (2016). Gut microbiome remodeling induces depressive-like behaviors through a pathway mediated by the host’s metabolism. Mol. Psychiatry 21, 786–796. doi: 10.1038/mp.2016.44
Keywords: major depressive disorder, gut microbiome, diversity, inflammatory factors, cognitive function
Citation: Liu P, Gao M, Liu Z, Zhang Y, Tu H, Lei L, Wu P, Zhang A, Yang C, Li G, Sun N and Zhang K (2022) Gut Microbiome Composition Linked to Inflammatory Factors and Cognitive Functions in First-Episode, Drug-Naive Major Depressive Disorder Patients. Front. Neurosci. 15:800764. doi: 10.3389/fnins.2021.800764
Received: 24 October 2021; Accepted: 30 December 2021;
Published: 28 January 2022.
Edited by:
Shaohua Hu, Zhejiang University, ChinaReviewed by:
Dominik Strzelecki, Medical University of Lodz, PolandManli Huang, Zhejiang University, China
Copyright © 2022 Liu, Gao, Liu, Zhang, Tu, Lei, Wu, Zhang, Yang, Li, Sun and Zhang. This is an open-access article distributed under the terms of the Creative Commons Attribution License (CC BY). The use, distribution or reproduction in other forums is permitted, provided the original author(s) and the copyright owner(s) are credited and that the original publication in this journal is cited, in accordance with accepted academic practice. No use, distribution or reproduction is permitted which does not comply with these terms.
*Correspondence: Ning Sun, c3VubmluZ0BzeG11LmVkdS5jbg==; Kerang Zhang, YXRvbXN4bXVAdmlwLjE2My5jb20=