- 1Department of Psychiatry and Psychology, Mayo Clinic, Rochester, MN, United States
- 2Department of Health Science Research, Mayo Clinic, Rochester, MN, United States
- 3Department of Biological Sciences, Fordham University, New York, NY, United States
- 4Department of Molecular Pharmacology and Experimental Therapeutics, Mayo Clinic, Rochester, MN, United States
- 5Department of Physiology and Biomedical Engineering, Mayo Clinic, Rochester, MN, United States
- 6Mayo Clinic Alix School of Medicine, Rochester, MN, United States
Rapid cycling (RC) burdens bipolar disorder (BD) patients further by causing more severe disability and increased suicidality. Because diagnosing RC can be challenging, RC patients are at risk of rapid decline due to delayed suitable treatment. Here, we aimed to identify the differences in the circulating cell-free DNA (cfDNA) methylome between BD patients with and without RC. The cfDNA methylome could potentially be developed as a diagnostic test for BD RC. We extracted cfDNA from plasma samples of BD1 patients (46 RC and 47 non-RC). cfDNA methylation levels were measured by 850K Infinium MethylationEPIC array. Principal component analysis (PCA) was conducted to assess global differences in methylome. cfDNA methylation levels were compared between RC groups using a linear model adjusted for age and sex. PCA suggested differences in methylation profiles between RC groups (p = 0.039) although no significant differentially methylated probes (DMPs; q > 0.15) were found. The top four CpG sites which differed between groups at p < 1E-05 were located in CGGPB1, PEX10, NR0B2, and TP53I11. Gene set enrichment analysis (GSEA) on top DMPs (p < 0.05) showed significant enrichment of gene sets related to nervous system tissues, such as neurons, synapse, and glutamate neurotransmission. Other top notable gene sets were related to parathyroid regulation and calcium signaling. To conclude, our study demonstrated the feasibility of utilizing a microarray method to identify circulating cfDNA methylation sites associated with BD RC and found the top differentially methylated CpG sites were mostly related to the nervous system and the parathyroid.
Introduction
Bipolar disorder (BD) features recurrent episodes of mania/hypomania and depression, interspersed with periods of euthymia. Symptoms usually include drastic changes in energy levels, sleep, thinking, and behaviors, which can significantly disrupt the daily life of BD patients (Craddock and Sklar, 2013). A mood cycle is defined as the period from the onset of a mood episode of any polarity to the emergence of another mood episode of any polarity. Mood cycles could last for weeks to months. When four or more distinct mood episodes (manic, hypomanic, major depressive, or mixed) occur within 12 months, demarcated by a period of partial or full remission for a least 2 months or by a switch to an episode of the opposite polarity, the phenomenon is specified as rapid cycling (American Psychiatric Association, 2000). Rapid cycling affects a significant proportion of BD patients with a lifetime prevalence of 33%, particularly in those with a longer course of illness, early onset age, and substance abuse (Fountoulakis et al., 2013; Gigante et al., 2016). It has been suggested that rapid cycling represents a decline of the disorder; it is associated with more severe disability and increased suicidality and demands different treatment strategies (Fountoulakis et al., 2013). However, the diagnosis of rapid cycling may be difficult since not all rapid cyclers achieve full remissions between episodes, which may lead to misdiagnosis such as borderline personality disorder (Mackinnon and Pies, 2006) and delay appropriate treatment.
The etiology of rapid mood cycling is hitherto elusive. Female sex, BD type II, and antidepressant treatment have been associated with the risk of rapid cycling (Fountoulakis et al., 2013). Increased susceptibility to DNA damage, hypothyroidism, and insulin resistance had also been observed in BD patients with the rapid cycling feature. However, it remains unclear whether these factors are the causes of rapid cycling or the consequences of drug treatment or medical comorbidities (Buoli et al., 2017). Our group recently reported a genetic association between the glutamate transporter 2 gene SLC1A2 and rapid cycling in a group of ∼2,000 depressed patients (Veldic et al., 2019). We found the minor allele of the single nucleotide polymorphism (SNP) rs3812778, located downstream of SLC1A2, was associated with an increased risk of rapid cycling as well as increased anterior cingulate glutamate levels, implicating genetic effects on the regulation of mood fluctuation via the synaptic clearance of glutamate in this brain region (Veldic et al., 2019). While genetics informs the risks of BD and related features, state-dependent biomarkers that are associated with mood instability may help the prognosis and diagnosis of rapid cycling thus need to be identified.
Circulating cell-free DNA (cfDNA) present in plasma, urine, cerebrospinal fluid, and other bodily fluids are short DNA fragments believed to be derived from cells undergoing apoptosis and necrosis and, possibly, released by active secretion (Wan et al., 2017). In healthy individuals, cfDNA concentration is generally low and the majority of plasma cfDNA is released from cells of hematopoietic lineage and to a lesser extent from other tissues (Lui et al., 2002; Sun et al., 2015; Moss et al., 2018), but in certain physiological and clinical conditions, such as exercise, pregnancy, infection, acute trauma, and transplantation, cfDNA concentration and/or composition (in terms of the tissue/cell/donor of origin) were found to be altered (Grace et al., 2016; Wan et al., 2017; Goh et al., 2018), thus allowing cfDNA to become a potential biomarker of various conditions and diseases. For example, screening of cfDNA in maternal serum for aneuploidy and other genetic conditions of the fetus (by detecting placenta-derived cfDNA) is now widely applied (Grace et al., 2016). Serum or plasma cfDNA has demonstrated prognostic and diagnostic potential in various cancers by detecting tumor-derived cfDNA (Wan et al., 2017). Since alterations in DNA methylation have been observed in brain tissues and related cell types of various psychiatric illnesses, including posttraumatic stress disorder (Blacker et al., 2019), schizophrenia (Veldic et al., 2004; Costa et al., 2006; Guidotti et al., 2016), and BD (Guidotti et al., 2016; Ho et al., 2019), it has been proposed that DNA methylation may be involved in the etiology and progression of these illnesses. Methylation patterns in plasma cfDNA may help to determine the cell or tissue of origin (Moss et al., 2018; Huang and Wang, 2019; Liu et al., 2019). In psychiatry, recent reports on altered cfDNA abundance and methylation level after psychosocial stressor challenge (Hummel et al., 2018) and increased plasma cell-free mitochondrial DNA (cfmtDNA) in suicide attempters (Lindqvist et al., 2016) suggest cfDNA and cfmtDNA could serve as biomarkers for psychological stress response, suicidal behavior and/or depression. Since rapid cycling could be difficult to diagnose and demands immediate personalized treatment strategies to prevent further decline and to minimize the risk of suicide, the use of biomarkers would facilitate timely prescription of treatment for BD patients with rapid cycling. We conducted this study to explore the differences in the methylomic landscape of plasma cfDNA between BD patients with and without rapid cycling as foundational work for identifying BD rapid cycling diagnostic biomarkers with the use of a microarray method for cfDNA methylation detection. We hypothesized that BD patients with rapid cycling would have a different cfDNA methylomic pattern compared to BD patients without rapid cycling, which might reflect certain physiological vulnerabilities associated with this specific BD feature.
Materials and Methods
Subjects
This study included 93 participants enrolled in the Mayo Clinic Bipolar Disorder Biobank (Frye et al., 2015). The biobank was approved by the Mayo Clinic Institutional Review Board, and recruited patients aged 18–80 years old with a Diagnostic and Statistical Manual of Mental Disorders 4th Edition Text Revision (DSM-IV-TR) or DSM-5 diagnosis of bipolar I, bipolar II, bipolar NOS, or schizoaffective bipolar type. All subjects provided written informed consent for use of their data and biospecimens in future studies. Exclusion criteria included inability or unwillingness to provide written informed consent, active suicidal ideation, or active psychosis. Clinical phenotypes were confirmed by the Structured Clinical Interview for DSM-IV (First et al., 1997). Subjects included in this study had a diagnosis of BD1 in which 46 subjects had experienced rapid mood cycling (≥4 distinct mood episodes within a 12-month period) while 47 subjects who did not have such experience were regarded as non-rapid cycling controls.
Plasma Cell-Free DNA Extraction
Venous blood sample was collected in potassium EDTA tubes and was centrifuged at 145 g for 12 min with soft deceleration. The bottom 1/3 of plasma was further centrifuged at 2,300 g for 10 min, only the top 3/4 of resultant plasma was collected as platelet-poor plasma which was stored at −80°C until cfDNA extraction by QIAamp Circulating Nucleic Acid Kit (Qiagen, Hilden, Germany) according to the manufacturer’s instruction.
Genome-Wide Methylome Profiling of Plasma cfDNA by 850K MethylEPIC Array
Methylation analysis methods applied were the same as in Ho et al. (2019). About 25 ng of cfDNA was used for bisulfite conversion and methylome microarray analysis performed at the University of Minnesota Genomics Center in a single batch. Genome-wide methylomic profiling was performed on the 850K Infinium MethylationEPIC BeadChip platform (Illumina Inc., San Diego, CA, United States). Unmethylated and methylated CpGenome controls and replicates of a reference cfDNA sample were included in each array to assess inter-array consistency.
Data Processing
Data quality control and statistical analyses were performed in R software v.3.4.1 with Bioconductor package minfi (Aryee et al., 2014). No sample was excluded due to low call rates (detection p < 0.05, apart from one rapid cycling subject’s sample with p = 0.07). Considering subject samples only, one sample had a locus detection rate > 99%, 66 samples had between 95 and 98.99%, 22 samples had between 90 and 94.99%, and four samples had < 89.99% (lowest 88.41%). Probes that failed in one or more samples, had a single nucleotide polymorphism at the CpG site, or mapped to non-specific genomic locations were removed. The probes located on X and Y chromosomes were excluded from data quality control procedures but included in subsequent statistical analyses. The initial methylation data contained 865 859 probes. After quality control, 463 050 probes were available for analysis (see Supplementary Method for the numbers of probes dropped for specific reasons). Data were normalized by functional normalization (preprocessFunnorm command in minfi) which removes between-array variation by regressing out variability in the control probes on each array (Fortin et al., 2014). Probes on X and Y chromosomes were normalized according to the sex of the sample.
Multivariate Analyses
Principal component analysis (PCA) was performed on the top 2,000 most variable CpG probes considering all samples (CpG probes with the largest standard deviations in M-values) but probes located on X and Y chromosomes were excluded. Association between the clusters and clinical factors were tested by chi-square and Fisher’s exact tests for categorical variables and t-tests for continuous variables.
Estimation of Cell-Type Composition in cfDNA Samples
We used the cell-type methylomic deconvolution algorithm and reference atlas by Moss et al. (2018) to estimate the cell-type origin composition in each subject’s plasma cfDNA sample. Briefly, the method uses non-negative least squares linear regression to determine the relative contributions of various cell types to the plasma cfDNA sample. The cell-type methylomic reference atlas includes ∼8,000 CpGs which are uniquely hypermethylated and hypomethylated in a certain tissue or cell type relative to the others (a total of 26 human cell types and tissues) (Moss et al., 2018).
Identification of Differentially Methylated Positions
Differentially methylated positions (DMPs; methylation status at individual sites) were identified by the R Bioconductor package limma (Ritchie et al., 2015). Differential methylation analyses were performed comparing rapid cyclers and non-rapid cyclers using standard limma workflow with unpaired contrasts with age and sex as covariates. M-values were used for statistical analysis and β-values were reported for interpretability. Statistically significant DMP was considered at FDR-adjusted p (q-value) < 0.15 based on our previous publication using the same microarray platform (Ho et al., 2019).
Identification of Differentially Methylated Regions
Differentially methylated regions (DMRs; methylation status across a genomic region) were identified by the R Bioconductor package DMRcate (Peters et al., 2015). A DMR was annotated when a region having at least two CpG sites with FDR-adjusted p-values at default detection level within a lambda of 220 (since the lengths of most cfDNA fragments range between 120 and 220 bp). Statistically significant DMR between groups was considered at Stouffer’s p < 0.05 (Ho et al., 2019).
Gene Set Enrichment Analysis
Gene set enrichment analysis (GSEA) to determine if methylation levels of any gene sets were significantly associated with rapid cycling was performed using the missMethyl Bioconductor R package (Phipson et al., 2016). The analysis was performed on the DMPs at p < 0.05 using the Gene Ontology (GO) and Kyoto Encyclopedia of Genes and Genomes (KEGG) gene sets (Ashburner et al., 2000; Kanehisa et al., 2012). Statistically significant enrichment was considered at FDR < 0.05.
Results
Subject Characteristics
Subject characteristics are presented in Table 1. Rapid cyclers and non-rapid cyclers were not significantly different in terms of age, sex, onset age, substance abuse and dependence history, the use of lithium, mood stabilizers, and antidepressants, and the proportion of diabetic subjects. A significantly higher percentage of subjects were on antipsychotics in the rapid cycling group than the non-rapid cycling group (65.2% vs. 40.4%).
Multivariate Analysis
PCA identified a remarkable percentage of variance explained by PC1, which overshadowed the variance explained by other PCs (Figure 1A). The clustering of samples according to PC1 and PC2 was not significantly associated with technical factors (sample plate and array), sex, age, and substance abuse/dependence history (p > 0.05). No significant clustering was observed by substance abuse/dependence history (Supplementary Figure 1) or psychotropic medication class (Supplementary Figure 2).
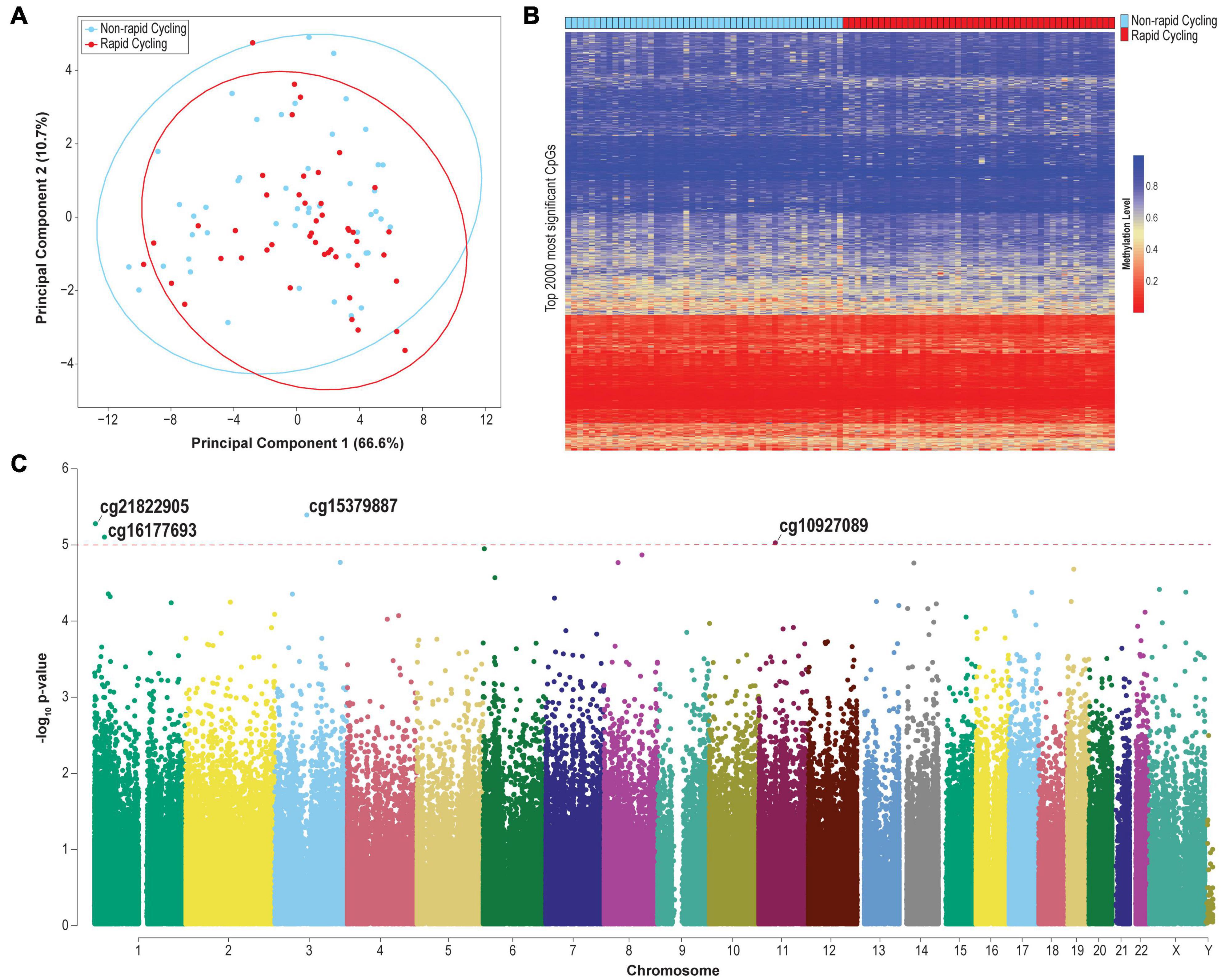
Figure 1. (A) PCA plot based on the top 2,000 most variable probes (CpG sites located on chromosomes X and Y were excluded). (B) Heatmap of the methylation levels of the top 2,000 most significant differentially methylated probes. (C) Manhattan plot of genome-wide plasma cfDNA methylomic comparison between rapid cyclers and non-rapid cyclers among bipolar disorder patients. The CpG probes where the top differentially methylated sites (p < 1E-05) are located are indicated. Red line: p = 1E-05.
The clustering of samples according to PC2 was significantly associated with rapid cycling (Kruskal-Wallis test p = 0.039). Genes mapped to CpG sites with PC2 factor loading ≥ | 0.02| (n = 554 probes which mapped to 341 genes; Supplementary Table 1) include TTC12 [loading = −0.098 (top of the list); together with NCAM, ANNK1 and DRD2, forming a gene cluster which is associated with substance dependence (Mota et al., 2015)], GABBR1 (loading = −0.087; a GABAB receptor subunit), CSNK1D [loading = −0.068; related to circadian rhythm and lithium response in BD (Geoffroy et al., 2018)], and KCNC4 (loading = 0.044; a voltage-gated potassium channel). These results were not driven by CpG sites located in CpG island, shelf, or shore, since after restricting to CpG sites only within these regions, neither PC1 nor PC2 was associated with rapid cycling (p > 0.05; Supplementary Figure 3).
Cell-Type Composition in cfDNA Samples
Using the cell-type methylomic deconvolution algorithm and reference atlas by Moss et al. (2018), we estimated the distribution of cfDNA origins among 26 reference cell types/tissues (Table 2). An average of 71% of qualified probes matched the reference atlas probes of each tissue/cell type and the cfDNA tissue/cell type compositions were significantly different between our samples and Moss et al. (2018) in the left atrium, prostate, vascular endothelial cells, colon epithelial cells, lung cells, kidney, hepatocytes, bladder, and head and neck larynx (p < 0.001; Supplementary Table 2). Most of the blood cell types (monocytes, NK-cells, neutrophils, and erythrocyte progenitors) had the lowest probe overlapping rates (∼54%), while breast, pancreatic acinar cells, upper GI tract, vascular endothelial cells, hepatocytes, and cortical neurons had the highest overlapping rates (79–81%; Supplementary Table 2). Table 2 shows the comparison of estimated cfDNA tissue/cell type of origin distribution between rapid cycling groups. Overall, the most prevalent estimated cfDNA tissue/cell type of origins were blood cells (neutrophils, erythrocyte progenitors, monocytes, and lymphocytes), which accounted for 82.6% of total cfDNA origins. Estimated cfDNA origins from the left atrium (3.3%), cortical neurons (2.9%), vascular endothelial cells (2.4%), and colon epithelial cells (2.1%) were also substantially present. Between rapid cyclers and non-rapid cyclers, no significant differences in estimated cfDNA cell/tissue types of origin were found (p > Bonferroni-adjusted α = 0.05/26 = 0.001923; individual sample compositions are shown in Supplementary Figure 4), hence no adjustment in tissue/cell type origin distribution on the methylome analysis was conducted.
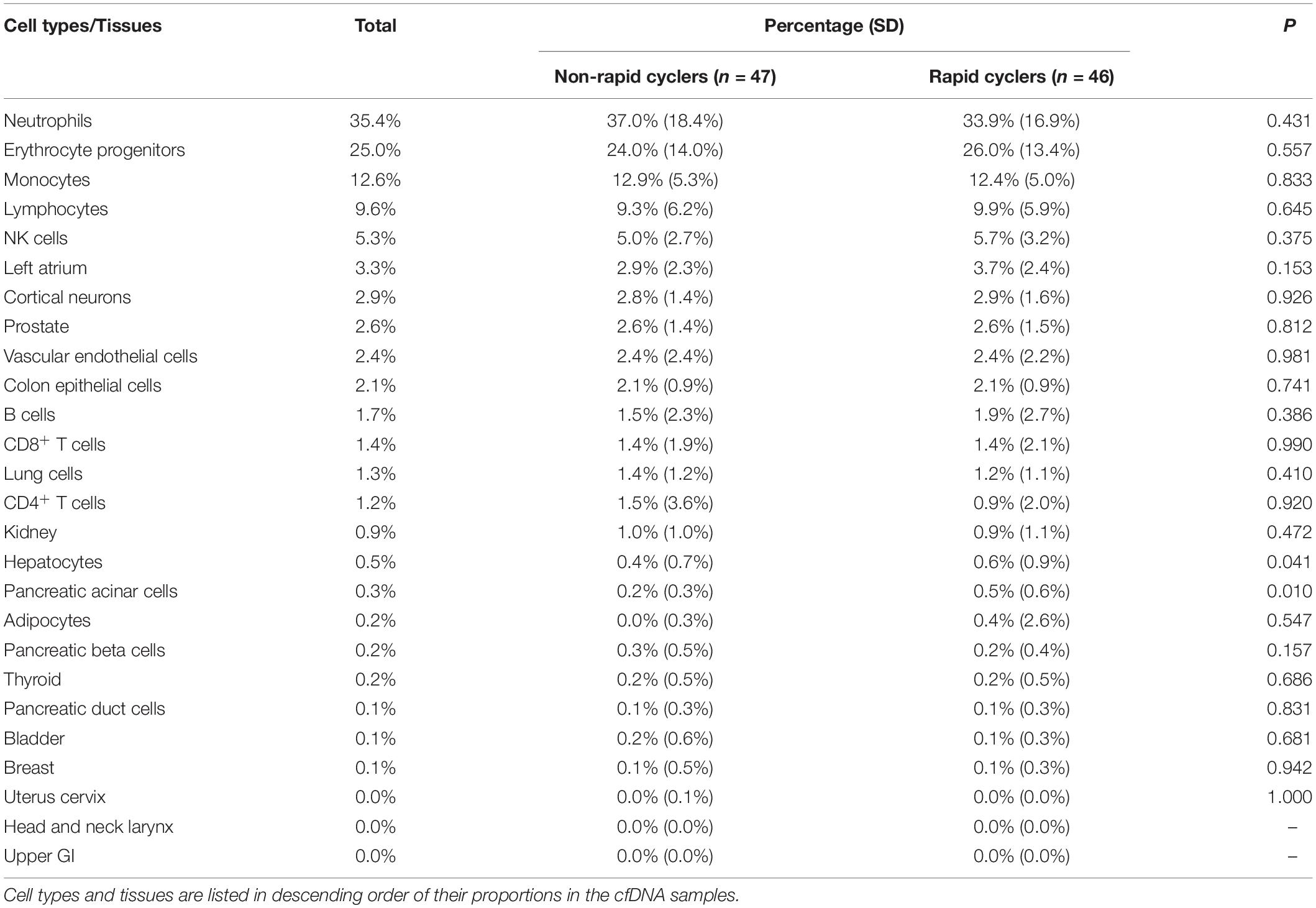
Table 2. Comparison of estimated cfDNA tissue/cell type origin distribution between BD rapid cyclers and non-rapid cyclers.
cfDNA Methylomic Differences Between Rapid Cyclers and Non-rapid Cyclers
The overall cfDNA methylomic differences between rapid cyclers and non-rapid cyclers were subtle as demonstrated in the heatmap (Figure 1B). A Manhattan plot of the genome-wide cfDNA methylomics comparison is shown in Figure 1C. No significant between-group DMPs (q > 0.15; top 2000 CpGs are listed in Supplementary Table 3) or DMRs (Stouffer p > 0.05; listed in Supplementary Table 4; top ten DMRs are shown in Supplementary Figure 5) were found. The top CpG sites with the most significant between-group differences at p < 1E-05 (cg15379887, cg21822905, cg16177693, and cg10927086) were located in CGGBP1, PEX10, NR0B2, and TP53I11. Rapid cyclers had relatively lower methylation levels in the promoter and/or 5′UTR of CGGPB1 (north CpG shore), NR0B2 (south CpG shore), and TP53I11 (north CpG shelf), while they had relatively higher methylation levels in the body of PEX10 (open sea; Figure 2).
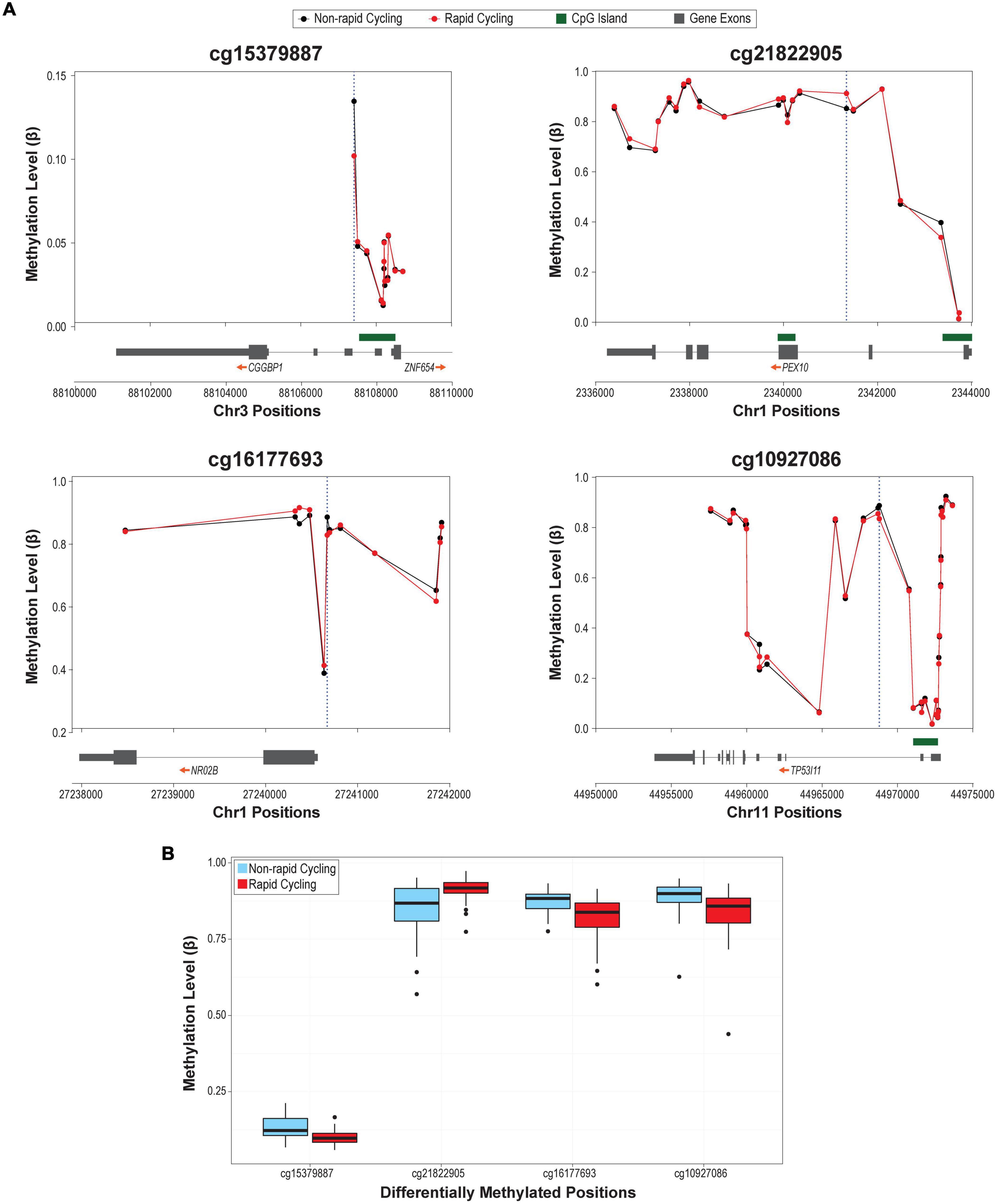
Figure 2. (A) Regional plots of the top differentially methylated probes (DMPs; p < 1E-05). Blue dotted vertical lines mark the genomic locations of the most significantly differentially methylated CpGs in that region. (B) Box plots of the methylation levels of the four DMPs differed between rapid cyclers and non-rapid cyclers at p < 1E-05.
We performed GSEA on genes with CpG sites that were different between groups at p < 0.05 (including 23,508 probes that mapped to 10,798 genes). We found significant enrichment in 26 GO gene sets, which are related to synapses, neurons, neurotransmissions, and nervous system development, and in three KEGG gene sets, which are involved in parathyroid regulation and calcium signaling (FDR < 0.05; Table 3). The list of genes and CpG sites mapped to these pathways is shown in Supplementary Table 5.
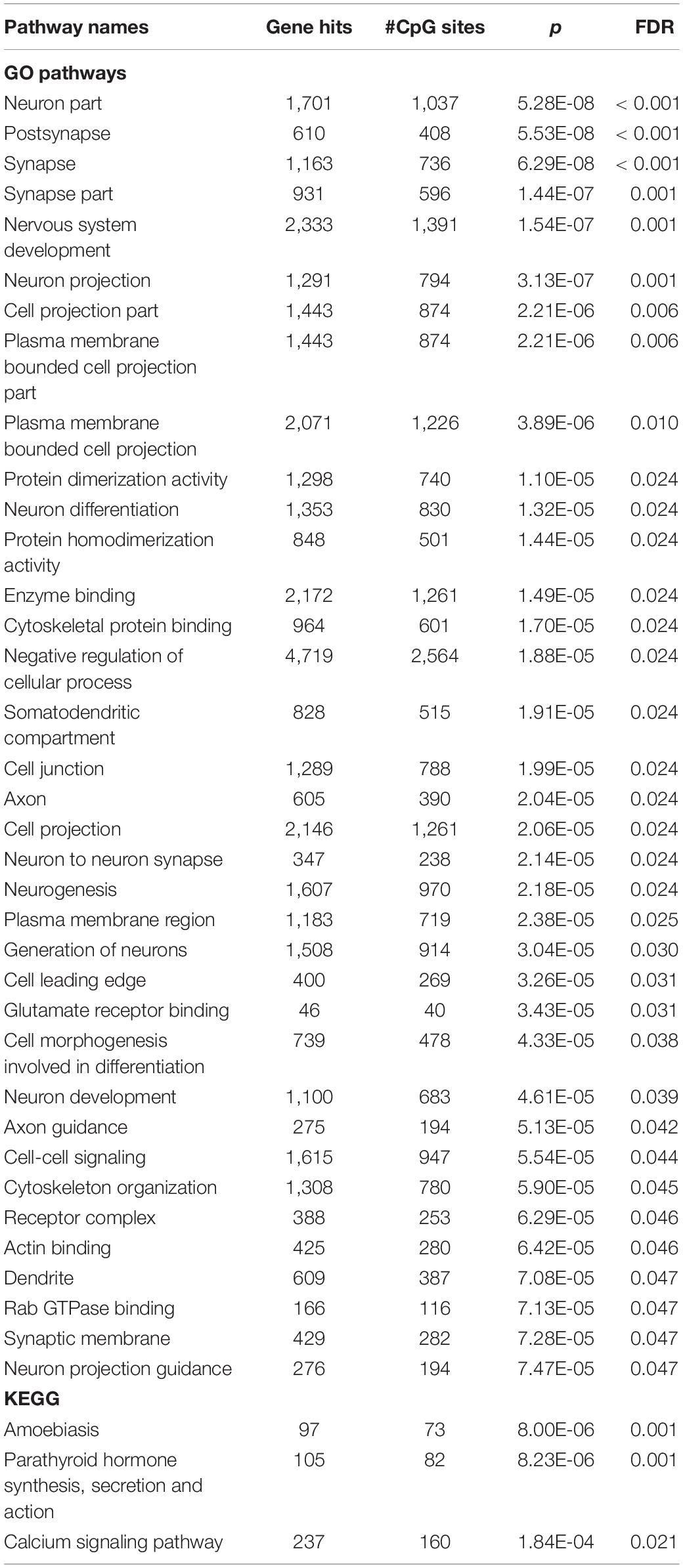
Table 3. Pathway enriched by genes with CpG sites that differed between rapid cycling groups at p < 0.05.
Leave-One-Out Cross-Validation of Top Between-Group cfDNA Methylomic Differences
We performed leave-one-out cross-validation analysis to verify that our top between-group DMPs were not driven by individuals with exceptionally high or low methylation levels. For the four DMPs with the top methylation level differences between rapid cyclers and non-rapid cyclers, we iteratively removed one individual and repeated the unpaired contrasts with adjustment of age and sex until all subjects had been excluded once. From each iteration, the log2 fold changes and p-values of the target DMPs were recorded. The log2 fold changes and p-values reported on the top four DMPs in our initial analysis were extremely close to the mean values obtained from the leave-one-out contrasts (Supplementary Table 6), thus confirming our top findings were not driven by extreme methylation values in certain samples.
Discussion
In this study, we used a microarray method to detect plasma cfDNA methylation levels to examine the cfDNA methylomic differences that were associated with rapid cycling in BD. We found global differences in methylation profiles between BD rapid cyclers and non-rapid cyclers, four CpG sites with differential methylation levels between BD rapid cyclers and non-rapid cyclers at p < 1E-05 (not genome-wide significant), and significant enrichment in pathways related to neurons and synaptic functions among the top CpG sites that differed between the groups. To our knowledge, this is the first investigation of cfDNA methylomics as potential biomarkers for BD rapid cycling, thereby marking an example of cfDNA application in psychiatric diagnosis and providing insights into amelioration of rapid mood cycling in BD.
Most cfDNA methylomic studies used sequencing-based methods such as whole-genome bisulfite sequencing, reduced representation bisulfite sequencing, and methylated CpG tandem amplification and sequencing (Zeng et al., 2018), while a few studies used microarray analysis (Gallardo-Gómez et al., 2018; Gordevicius et al., 2018). Compared to sequencing-based methods, microarrays have much lower coverage but provide an economic genome-wide platform that screens CpG sites located at gene bodies and regulatory elements (Huang and Wang, 2019) and the required amount and quality of input DNA are generally less demanding. In our study, the low locus detection rates (<99%) and high exclusion rate of CpG probes from analysis (∼45%) might be caused by insufficient input DNA, exacerbated by the biased DNA fragmentation pattern in cfDNA which can reduce coverage in transcription start sites and exon boundaries (Ma et al., 2017). In future mood disorder studies where other origins of organ damage and the presence of tumors can be excluded, strategies for increasing input cfDNA amount would be needed to increase cfDNA to boost experimental performance, such as pooling of cfDNA samples from multiple well-matched samples (Gallardo-Gómez et al., 2018).
The estimated proportions of tissues and cell types that contributed to the cfDNA in our samples were slightly different from those reported by Moss et al. (2018), which may be due to differences in participants’ demographic and clinical characteristics, the cfDNA preparation procedures employed, and the quantity of input cfDNA applied onto the EPIC arrays. Participants in Moss et al. (2018) were healthy individuals aged 19–30 years and ≥ 75 years, while our BD participants were mainly middle-aged (20–79 years; mean 45 years). Moreover, they used pooled cfDNA samples generated by mixing cfDNA samples of several subjects (7–19 samples/pool) classified by age and sex until the sample reaching 250 ng cfDNA which was the recommended input DNA amount of the Illumina methylomic arrays (Moss et al., 2018). Moss et al. (2018) evaluated the performance of the deconvolution algorithm using 100 and 50 ng of cfDNA, i.e., amounts much less than manufacturer-recommended 250 ng, and found their predicted tissue/cell type distributions correlated with the distributions obtained with the recommended amount at r > 0.99 and > 0.90, respectively. Therefore, we projected that the reproducibility of applying the Moss et al. (2018) method to our microarray data would be lower but still satisfactory. Relative to the cfDNA tissue/cell type of origin proportions in healthy individuals reported by Moss et al. (2018), our data obtained in BD patients were largely similar for most tissues/cell types but dissimilar in some notably, for example, we found a significantly higher proportion of vascular endothelial cells (Supplementary Table 2). These differences may be attributed to BD medications, medical comorbidities of BD such as the increased risk of cardiovascular diseases (Goldstein et al., 2020), and/or the aforementioned technical differences between studies that affected the performance of the tissue/cell type estimation.
The top differentially methylated sites between rapid cyclers and non-rapid cyclers were CGGBP1, which encodes for CGG triplet repeat-binding protein 1, a nuclear protein that selectively binds to unmethylated CGG trinucleotide repeats. The hypomethylation in a CpG site which is located in the CGGBP1 5′UTR and at the north shore of a CpG island implies an upregulation of gene expression (Martino and Saffery, 2015). CGGBP1 is expressed ubiquitously across human tissues. It was found to regulate gene transcription and participate in cell growth and proliferation with cytoprotective properties (Singh and Westermark, 2015). Its specific roles in transcription regulation include, but are not limited to, shielding unmethylated CGG repeats from methylation (Deissler et al., 1996), histone modification (Stelzl et al., 2005; Patel et al., 2019), and acting as a trans-regulator of RNA polymerase II-transcribed genes (Agarwal et al., 2016). CGGBP1 deficiency results in cell cycle arrest at the S phase and G2/M phase in normal cells (Singh and Westermark, 2011). Notably, CGGBP1 as a D-box-containing protein (D-box is a cis-regulatory element known to associate with zenith expression of genes during the “active phase”) has been found to demonstrate circadian variations in its expression level in intestinal epithelial cells in response to an unusual restricted feeding schedule in mice, thus demonstrating that CGGBP1 as a component of the peripheral circadian clock (Mukherji et al., 2015). Reduced CGGBP1 gene expression had been reported in the peripheral blood of patients with post-traumatic stress disorder (Uddin et al., 2011). Therefore, altered CGGBP1 may be a critical indicator of the misalignment between the central and peripheral circadian clocks which could progressively induce metabolic syndrome (Mukherji et al., 2015) and increase the risk of mood disorders (Baron and Reid, 2014). However, due to the scarcity of studies on the association between CGGBP1 and psychiatric disorders, the involvement of CGGBP1 in mood disorders is yet to be identified.
PEX10 encodes peroxisomal biogenesis factor 10, a ubiquitously expressed peroxisomal matrix protein. Peroxisomes are organelles that are critical for fatty acid and phospholipid metabolisms, such as very-long-chain fatty acid catabolism and the biosynthesis of docosahexaenoic acid (DHA) and plasmalogens which are essential for normal brain development and functioning. Peroxisome dysfunction has been associated with neurodegenerative disorders (Jo and Cho, 2019) and mood disorders (McNamara et al., 2010). Lower erythrocyte DHA level was observed in BD1 patients and found to be associated with the onset of the first mania episode (McNamara et al., 2015) and increased risk of BD1 development (McNamara et al., 2016a), but contrasting results have also been reported (McNamara et al., 2016b). Therefore, the association between peroxisomal dysfunction and BD remains uncertain. PEX10 mutations can cause Zellweger syndrome (Okumoto et al., 1998). Although PEX10 has not been extensively studied in the brain, Pex10 deficiency in mice resulted in reduced Schwann cell count and poor integrity of axon and synapse in the spinal cord (Hanson et al., 2014). Here, we found a CpG site in PEX10 gene body was hypermethylated in the rapid cycling group, implying a potentially altered PEX10 gene expression level may be associated with BD rapid cycling. For example, the altered PEX10 levels may affect fatty and phospholipid acid metabolisms, which are important to brain health, thereby precipitating mood instability. However, it is unclear which tissue was responsible for this difference and whether the observed changes were functionally significant.
NR0B2 encodes nuclear receptor subfamily 0 group B member 2 (a.k.a. short heterodimer partner), an orphan nuclear receptor that interacts with receptors of estrogen, retinol, bile acid, and thyroid hormone to suppress the transcriptional activity of these nuclear receptors (Seol et al., 1997; Ma and Patti, 2014) as well as with peroxisome proliferator-activated receptor (PPAR) α and γ (Kassam et al., 2001; Nishizawa et al., 2002). NR0B2 is mostly expressed in the gastrointestinal tract (stomach, duodenum, small intestine, and colon) and liver, and to a lesser extent in the gall bladder, spleen, pancreas, and heart. NR0B2 is involved in the regulation of bile acid synthesis (Yang et al., 2014), bile salt secretion (Neimark et al., 2004), inflammation (Maguire et al., 2017; Zhou et al., 2018), insulin secretion and pancreatic β cell survival (Suh et al., 2004; Noh et al., 2013), as well as lipid metabolism in liver and brown adipocytes (Wang et al., 2005; Khristi et al., 2019). Our finding of a lower methylation level in the promoter region of NR0B2 suggests that an increase in NR0B2 expression in some tissues may be related to BD rapid cycling. Since NR0B2 is involved in lipid and glucose metabolisms and inflammation, dysfunctionality in these processes may be associated with the risk of rapid mood cycling, this coincides with the higher rates of rapid cycling observed in BD patients with diabetes mellitus or insulin resistance (Ruzickova et al., 2003; Calkin et al., 2015).
TP53I11 encodes tumor protein P53 inducible protein 11 and are expressed ubiquitously in human tissues. It has been studied almost exclusively in oncology and information on its association with mood disorders is absent. TP53I11 was found to promote apoptosis by binding to DNA (Liang et al., 2004; Xiong et al., 2007) as well as inhibit tumor metastasis (Xiao et al., 2019). Its association with BD rapid cycling remains to be uncovered.
In the list of genes with top differentially methylated CpG sites (p < 0.05), nearly all significantly enriched GO gene sets were related to the structure, generation, and development of neurons as well as the structure and functioning of synapses, suggesting that plasma cfDNA may contain brain-derived DNA and those methylation levels could reflect the presence of rapid cycling feature in BD. However, this postulation needs empirical support from tissue-specific methylome analyses and also proof that neuronal and synaptic dysfunctions contribute to rapid cycling in BD. Among various neurotransmitter systems, the “glutamate receptor binding” pathway was significantly enriched, thereby reiterating the importance of the glutamatergic system in regulating rapid mood cycling in BD found in previous studies (Michael et al., 2009; Veldic et al., 2019). Another interesting enriched gene set was “Rab GTPase binding” as Rab GTPases are critical players in vesicular trafficking between the cell body and axonal presynaptic and dendritic postsynaptic terminals and in synaptic vesicle docking and exocytosis at the presynapse, regulating neuronal development and polarization, presynaptic neurotransmitter release, and postsynaptic membrane composition (Mignogna and D’Adamo, 2018). Two significantly enriched KEGG gene sets, “parathyroid hormone, synthesis, secretion and action” and “calcium signaling pathway,” pointed toward calcium homeostasis and the effects of calcium on various cell types, such as muscle contraction and long-term potentiation and depression related to learning and memory. Although the relationship between parathyroid hormone and mood is unclear, parathyroid hormone can cross the blood-brain barrier and parathyroid receptors are found throughout the human brain (Lourida et al., 2015). A recent study reported that serum parathyroid levels of BD patients were correlated positively with the total number of mood episodes and suicide attempts but negatively with the age of onset (Steardo et al., 2020). Notably and paradoxically, the use of lithium is known to induce hyperparathyroidism and hypercalcemia (Albert et al., 2013).
A few limitations need to be noted. Firstly, the blood samples from which the plasma cfDNA was derived were not collected in specific mood states (mostly during euthymia and depressed states for ethical reasons) or the change between mood states. However, obtaining biospecimens during or around the time of mood state is limited by practical and ethical reasons. Secondly, the organs and cell types responsible for the cfDNA detected in the samples could not be ascertained. We have adopted the method by Moss et al. (2018) for predicting the distribution of tissues/cell types of origin in cfDNA samples and found a substantial percentage of cfDNA with cortical neuronal origin in our samples and no significant differences in cfDNA tissues/cell types of origin distribution between groups. However, to determine that a tissue/cell type contributes to a certain cfDNA methylomic difference, methods that can detect the methylation pattern in each cfDNA fragment (e.g., sequencing) coupled with an advanced deconvolution algorithm would be required. Validation in specific tissues and cell types, such as peripheral blood mononuclear cells, are needed to confirm the origins of our findings. Thirdly, since the sample size was small, the statistical power was limited and led to the lack of significant differentially methylated sites after multiple testing correction. Lastly, in the absence of a replication experiment, in order to verify that our top findings were not due to extreme methylation values in our samples, we conducted leave-one-out cross-validation and found that our initial reported statistics were not driven by a single outlier sample. Nevertheless, replication using an independent cohort of subjects would be needed to confirm our findings.
To conclude, this study is the first investigation of circulating cfDNA methylomic differences of BD patients with rapid cycling to provide support for the diagnostic application of circulating cfDNA methylomics. We demonstrated the use of a microarray method for plasma cfDNA methylome analysis and identified potential differences associated with BD rapid cycling. The differentially methylated CpGs were mostly within or near genes involved in pathways related to the nervous system and parathyroid. Our findings need to be replicated with a larger, independent cohort and followed up with a more definitive assignment of the cfDNA methylomic signals to tissues-of-origin.
Data Availability Statement
The datasets presented in this article are not readily available because of research ethics protocol restriction. Requests to access the datasets should be directed to the corresponding author.
Ethics Statement
The studies involving human participants were reviewed and approved by the Mayo Clinic Institutional Review Board. The patients/participants provided their written informed consent to participate in this study.
Author Contributions
AM-CH and MV conceived the study. SW and BM provided advice on the study design and conducted the statistical analyses. AM-CH wrote the manuscript. MK, KR, ZS, TO, LW, and MF provided expert opinions on the study and critically reviewed the manuscript. All authors contributed to the article and approved the submitted version.
Funding
This study was funded by the Mayo Clinic Center for Individualized Medicine Epigenomics Program.
Conflict of Interest
MF was a consultant (for Mayo Clinic) to Janssen, Mitsubishi Tanabe Pharma Corporation, Myriad, Sunovion, and Teva Pharmaceuticals; none of this funding contributed to any work carried out in this study. The remaining authors declare that the research was conducted in the absence of any commercial or financial relationships that could be construed as a potential conflict of interest.
Publisher’s Note
All claims expressed in this article are solely those of the authors and do not necessarily represent those of their affiliated organizations, or those of the publisher, the editors and the reviewers. Any product that may be evaluated in this article, or claim that may be made by its manufacturer, is not guaranteed or endorsed by the publisher.
Acknowledgments
We thank the invaluable contributions of the Mayo Clinic Bipolar Biobank participants and the University of Minnesota Genomics Center for providing the methylomics service. We also thank Alejandra Cabello Arreola for clinical data extraction.
Supplementary Material
The Supplementary Material for this article can be found online at: https://www.frontiersin.org/articles/10.3389/fnins.2021.774037/full#supplementary-material
References
Agarwal, P., Enroth, S., Teichmann, M., Jernberg Wiklund, H., Smit, A., Westermark, B., et al. (2016). Growth signals employ CGGBP1 to suppress transcription of Alu-SINEs. Cell Cycle 15, 1558–1571. doi: 10.4161/15384101.2014.967094
Albert, U., De Cori, D., Aguglia, A., Barbaro, F., Lanfranco, F., Bogetto, F., et al. (2013). Lithium-associated hyperparathyroidism and hypercalcaemia: a case-control cross-sectional study. J. Affect. Disord. 151, 786–790. doi: 10.1016/j.jad.2013.06.046
American Psychiatric Association. (2000). Diagnostic and Statistical Manual of Mental Disorders, 4th Edn. Washington, D.C: APA.
Ashburner, M., Ball, C. A., Blake, J. A., Botstein, D., Butler, H., Cherry, J. M., et al. (2000). Gene ontology: tool for the unification of biology. Nat. Genet. 25, 25–29. doi: 10.1038/75556
Aryee, M. J., Jaffe, A. E., Corrada-Bravo, H., Ladd-Acosta, C., Feinberg, A. P., Hansen, K. D., et al. (2014). Minfi: a flexible and comprehensive Bioconductor package for the analysis of Infinium DNA methylation microarrays. Bioinformatics 30, 1363–1369. doi: 10.1093/bioinformatics/btu049
Baron, K. G., and Reid, K. J. (2014). Circadian misalignment and health. Int. Rev. Psychiatry 26, 139–154. doi: 10.3109/09540261.2014.911149
Blacker, C. J., Frye, M. A., Morava, E., Kozicz, T., and Veldic, M. (2019). A Review of epigenetics of PTSD in comorbid psychiatric conditions. Genes 10:140. doi: 10.3390/genes10020140
Buoli, M., Serati, M., and Altamura, A. C. (2017). Biological aspects and candidate biomarkers for rapid-cycling in bipolar disorder: a systematic review. Psychiatry Res. 258, 565–575. doi: 10.1016/j.psychres.2017.08.059
Calkin, C. V., Ruzickova, M., Uher, R., Hajek, T., Slaney, C. M., Garnham, J. S., et al. (2015). Insulin resistance and outcome in bipolar disorder. Br. J. Psychiatry 206, 52–57. doi: 10.1192/bjp.bp.114.152850
Costa, E., Dong, E., Grayson, D. R., Ruzicka, W. B., Simonini, M. V., Veldic, M., et al. (2006). Epigenetic targets in GABAergic neurons to treat schizophrenia. Adv. Pharmacol. 54, 95–117. doi: 10.1016/S1054-3589(06)54005-0
Craddock, N., and Sklar, P. (2013). Genetics of bipolar disorder. Lancet 381, 1654–1662. doi: 10.1016/S0140-6736(13)60855-7
Deissler, H., Behn-Krappa, A., and Doerfler, W. (1996). Purification of nuclear proteins from human HeLa cells that bind specifically to the unstable tandem repeat (CGG)n in the human FMR1 gene. J. Biol. Chem. 271, 4327–4334. doi: 10.1074/jbc.271.8.4327
First, M. B., Gibbon, M., Spitzer, R. L., Williams, J. B. W., and Benjamin, L. S. (1997). Structured Clinical Interview for DSM-IV axis II Personality Disorders, (SCID-II). Virginia: American Psychiatric Association
Fortin, J. P., Labbe, A., Lemire, M., Zanke, B. W., Hudson, T. J., Fertig, E. J., et al. (2014). Functional normalization of 450k methylation array data improves replication in large cancer studies. Genome Biol. 15:503. doi: 10.1186/s13059-014-0503-2
Fountoulakis, K. N., Kontis, D., Gonda, X., and Yatham, L. N. (2013). A systematic review of the evidence on the treatment of rapid cycling bipolar disorder. Bipolar Disord. 15, 115–137. doi: 10.1111/bdi.12045
Frye, M. A., McElroy, S. L., Fuentes, M., Sutor, B., Schak, K. M., Galardy, C. W., et al. (2015). Development of a bipolar disorder biobank: differential phenotyping for subsequent biomarker analyses. Int. J. Bipolar Disord. 3:30. doi: 10.1186/s40345-015-0030-4
Gallardo-Gómez, M., Moran, S., Páez de la Cadena, M., Martínez-Zorzano, V. S., Rodríguez-Berrocal, F. J., Rodríguez-Girondo, M., et al. (2018). A new approach to epigenome-wide discovery of non-invasive methylation biomarkers for colorectal cancer screening in circulating cell-free DNA using pooled samples. Clin. Epigenet. 10:53. doi: 10.1186/s13148-018-0487-y
Geoffroy, P. A., Curis, E., Courtin, C., Moreira, J., Morvillers, T., Etain, B., et al. (2018). Lithium response in bipolar disorders and core clock genes expression. World J. Biol. Psychiatry 19, 619–632. doi: 10.1080/15622975.2017.1282174
Gigante, A. D., Barenboim, I. Y., Dias, R. D., Toniolo, R. A., Mendonca, T., Miranda-Scippa, A., et al. (2016). Psychiatric and clinical correlates of rapid cycling bipolar disorder: a cross-sectional study. Braz. J. Psychiatry 38, 270–274. doi: 10.1590/1516-4446-2015-1789
Goh, S. K., Do, H. D., Muralidharan, V., Jones, R., Dobrovic, A., and Christophi, C. (2018). Donor-specific cell-free DNA as an emerging biomarker of organ rejection after liver transplantation. Transplantation 102, S182–S182. doi: 10.1097/01.tp.0000542826.68057.b6
Goldstein, B. I., Baune, B. T., Bond, D. J., Chen, P. H., Eyler, L., Fagiolini, A., et al. (2020). Call to action regarding the vascular-bipolar link: a report from the vascular task force of the international society for bipolar disorders. Bipolar Disord. 22, 440–460. doi: 10.1111/bdi.12921
Gordevicius, J., Krisciunas, A., Groot, D. E., Yip, S. M., Susic, M., Kwan, A., et al. (2018). Cell-Free DNA modification dynamics in abiraterone acetate-treated prostate cancer patients. Clin. Cancer Res. 24, 3317–3324. doi: 10.1158/1078-0432.CCR-18-0101
Grace, M. R., Hardisty, E., Dotters-Katz, S. K., Vora, N. L., and Kuller, J. A. (2016). Cell-Free DNA screening: complexities and challenges of clinical implementation. Obstet. Gynecol. Surv. 71, 477–487. doi: 10.1097/OGX.0000000000000342
Guidotti, A., Grayson, D. R., and Caruncho, H. J. (2016). Epigenetic RELN dysfunction in schizophrenia and related neuropsychiatric disorders. Front. Cell Neurosci. 10:89. doi: 10.3389/fncel.2016.00089
Hanson, M. G., Fregoso, V. L., Vrana, J. D., Tucker, C. L., and Niswander, L. A. (2014). Peripheral nervous system defects in a mouse model for peroxisomal biogenesis disorders. Dev. Biol. 395, 84–95. doi: 10.1016/j.ydbio.2014.08.026
Ho, A. M., Winham, S. J., Armasu, S. M., Blacker, C. J., Millischer, V., Lavebratt, C., et al. (2019). Genome-wide DNA methylomic differences between dorsolateral prefrontal and temporal pole cortices of bipolar disorder. J. Psychiatr. Res. 117, 45–54. doi: 10.1016/j.jpsychires.2019.05.030
Huang, J., and Wang, L. (2019). Cell-Free DNA methylation profiling analysis-technologies and bioinformatics. Cancers 11:1741. doi: 10.3390/cancers11111741
Hummel, E. M., Hessas, E., Müller, S., Beiter, T., Fisch, M., Eibl, A., et al. (2018). Cell-free DNA release under psychosocial and physical stress conditions. Transl. Psychiatry 8, 236–236. doi: 10.1038/s41398-018-0264-x
Jo, D. S., and Cho, D. H. (2019). Peroxisomal dysfunction in neurodegenerative diseases. Arch. Pharm. Res. 42, 393–406. doi: 10.1007/s12272-019-01131-2
Kanehisa, M., Goto, S., Sato, Y., Furumichi, M., and Tanabe, M. (2012). KEGG for integration and interpretation of large-scale molecular data sets. Nucleic Acids Res. 40, D109–D114. doi: 10.1093/nar/gkr988
Kassam, A., Capone, J. P., and Rachubinski, R. A. (2001). The short heterodimer partner receptor differentially modulates peroxisome proliferator-activated receptor alpha-mediated transcription from the peroxisome proliferator-response elements of the genes encoding the peroxisomal beta-oxidation enzymes acyl-CoA oxidase and hydratase-dehydrogenase. Mol. Cell. Endocrinol. 176, 49–56. doi: 10.1016/S0303-7207(01)00475-0
Khristi, V., Ratri, A., Ghosh, S., Pathak, D., Borosha, S., Dai, E., et al. (2019). Disruption of ESR1 alters the expression of genes regulating hepatic lipid and carbohydrate metabolism in male rats. Mol. Cell. Endocrinol. 490, 47–56. doi: 10.1016/j.mce.2019.04.005
Liang, X. Q., Cao, E. H., Zhang, Y., and Qin, J. F. (2004). A P53 target gene, PIG11, contributes to chemosensitivity of cells to arsenic trioxide. FEBS Lett. 569, 94–98. doi: 10.1016/j.febslet.2004.05.057
Lindqvist, D., Fernstrom, J., Grudet, C., Ljunggren, L., Traskman-Bendz, L., Ohlsson, L., et al. (2016). Increased plasma levels of circulating cell-free mitochondrial DNA in suicide attempters: associations with HPA-axis hyperactivity. Transl. Psychiatry 6:e971. doi: 10.1038/tp.2016.236
Liu, X., Ren, J., Luo, N., Guo, H., Zheng, Y., Li, J., et al. (2019). Comprehensive DNA methylation analysis of tissue of origin of plasma cell-free DNA by methylated CpG tandem amplification and sequencing (MCTA-Seq). Clin. Epigenet. 11:93. doi: 10.1186/s13148-019-0689-y
Lourida, I., Thompson-Coon, J., Dickens, C. M., Soni, M., Kuźma, E., Kos, K., et al. (2015). Parathyroid hormone, cognitive function and dementia: a systematic review. PLoS One 10:e0127574. doi: 10.1371/journal.pone.0127574
Lui, Y. Y., Chik, K. W., Chiu, R. W., Ho, C. Y., Lam, C. W., and Lo, Y. M. (2002). Predominant hematopoietic origin of cell-free DNA in plasma and serum after sex-mismatched bone marrow transplantation. Clin. Chem. 48, 421–427. doi: 10.1093/clinchem/48.3.421
Ma, H., and Patti, M. E. (2014). Bile acids, obesity, and the metabolic syndrome. Best Pract. Res. Clin. Gastroenterol. 28, 573–583. doi: 10.1016/j.bpg.2014.07.004
Ma, X., Zhu, L., Wu, X., Bao, H., Wang, X., Chang, Z., et al. (2017). Cell-free DNA provides a good representation of the tumor genome despite its biased fragmentation patterns. PLoS One 12:e0169231. doi: 10.1371/journal.pone.0169231
Mackinnon, D. F., and Pies, R. (2006). Affective instability as rapid cycling: theoretical and clinical implications for borderline personality and bipolar spectrum disorders. Bipolar Disord. 8, 1–14. doi: 10.1111/j.1399-5618.2006.00283.x
Maguire, M., Bushkofsky, J. R., Larsen, M. C., Foong, Y. H., Tanumihardjo, S. A., and Jefcoate, C. R. (2017). Diet-dependent retinoid effects on liver gene expression include stellate and inflammation markers and parallel effects of the nuclear repressor Shp. J. Nutr. Biochem. 47, 63–74. doi: 10.1016/j.jnutbio.2017.04.009
Martino, D., and Saffery, R. (2015). Characteristics of DNA methylation and gene expression in regulatory features on the Infinium 450k Beadchip. bioRxiv doi: 10.1101/032862
McNamara, R. K., Jandacek, R., Rider, T., Tso, P., Dwivedi, Y., and Pandey, G. N. (2010). Selective deficits in erythrocyte docosahexaenoic acid composition in adult patients with bipolar disorder and major depressive disorder. J. Affect. Disord. 126, 303–311. doi: 10.1016/j.jad.2010.03.015
McNamara, R. K., Jandacek, R., Tso, P., Blom, T. J., Welge, J. A., Strawn, J. R., et al. (2015). First-episode bipolar disorder is associated with erythrocyte membrane docosahexaenoic acid deficits: dissociation from clinical response to lithium or quetiapine. Psychiatry Res. 230, 447–453. doi: 10.1016/j.psychres.2015.09.035
McNamara, R. K., Jandacek, R., Tso, P., Blom, T. J., Welge, J. A., Strawn, J. R., et al. (2016a). Adolescents with or at ultra-high risk for bipolar disorder exhibit erythrocyte docosahexaenoic acid and eicosapentaenoic acid deficits: a candidate prodromal risk biomarker. Early Interv. Psychiatry 10, 203–211. doi: 10.1111/eip.12282
McNamara, R. K., Moser, A. B., Jones, R. I., Jandacek, R., Patino, L. R., Strawn, J. R., et al. (2016b). Familial risk for bipolar disorder is not associated with impaired peroxisomal function: dissociation from docosahexaenoic acid deficits. Psychiatry Res. 246, 803–807. doi: 10.1016/j.psychres.2016.10.042
Michael, N., Erfurth, A., and Pfleiderer, B. (2009). Elevated metabolites within dorsolateral prefrontal cortex in rapid cycling bipolar disorder. Psychiatry Res. 172, 78–81. doi: 10.1016/j.pscychresns.2009.01.002
Mignogna, M. L., and D’Adamo, P. (2018). Critical importance of RAB proteins for synaptic function. Small GTPases 9, 145–157. doi: 10.1080/21541248.2016.1277001
Moss, J., Magenheim, J., Neiman, D., Zemmour, H., Loyfer, N., Korach, A., et al. (2018). Comprehensive human cell-type methylation atlas reveals origins of circulating cell-free DNA in health and disease. Nat. Commun. 9:5068. doi: 10.1038/s41467-018-07466-6
Mota, N. R., Rovaris, D. L., Kappel, D. B., Picon, F. A., Vitola, E. S., Salgado, C. A., et al. (2015). NCAM1-TTC12-ANKK1-DRD2 gene cluster and the clinical and genetic heterogeneity of adults with ADHD. Am. J. Med. Genet. B Neuropsychiatr. Genet. 168, 433–444. doi: 10.1002/ajmg.b.32317
Mukherji, A., Kobiita, A., Damara, M., Misra, N., Meziane, H., Champy, M. F., et al. (2015). Shifting eating to the circadian rest phase misaligns the peripheral clocks with the master SCN clock and leads to a metabolic syndrome. Proc. Natl. Acad. Sci. U.S.A. 112, E6691–E6698. doi: 10.1073/pnas.1519807112
Neimark, E., Chen, F., Li, X., and Shneider, B. L. (2004). Bile acid-induced negative feedback regulation of the human ileal bile acid transporter. Hepatology 40, 149–156. doi: 10.1002/hep.20295
Nishizawa, H., Yamagata, K., Shimomura, I., Takahashi, M., Kuriyama, H., Kishida, K., et al. (2002). Small heterodimer partner, an orphan nuclear receptor, augments peroxisome proliferator-activated receptor gamma transactivation. J. Biol. Chem. 277, 1586–1592. doi: 10.1074/jbc.M104301200
Noh, J. R., Hwang, J. H., Kim, Y. H., Kim, K. S., Gang, G. T., Kim, S. W., et al. (2013). The orphan nuclear receptor small heterodimer partner negatively regulates pancreatic beta cell survival and hyperglycemia in multiple low-dose streptozotocin-induced type 1 diabetic mice. Int. J. Biochem. Cell Biol. 45, 1538–1545. doi: 10.1016/j.biocel.2013.05.004
Okumoto, K., Itoh, R., Shimozawa, N., Suzuki, Y., Tamura, S., Kondo, N., et al. (1998). Mutations in PEX10 is the cause of zellweger peroxisome deficiency syndrome of complementation group B. Hum. Mol. Genet. 7, 1399–1405. doi: 10.1093/hmg/7.9.1399
Patel, D., Patel, M., Datta, S., and Singh, U. (2019). CGGBP1 regulates CTCF occupancy at repeats. Epigenet. Chromatin 12:57. doi: 10.1186/s13072-019-0305-6
Peters, T. J., Buckley, M. J., Statham, A. L., Pidsley, R., Samaras, K., Lord, V. R., et al. (2015). De novo identification of differentially methylated regions in the human genome. Epigenet. Chromatin 8:6. doi: 10.1186/1756-8935-8-6
Phipson, B., Maksimovic, J., and Oshlack, A. (2016). missMethyl: an R package for analyzing data from Illumina’s HumanMethylation450 platform. Bioinformatics 32, 286–288. doi: 10.1093/bioinformatics/btv560
Ritchie, M. E., Phipson, B., Wu, D., Hu, Y., Law, C. W., Shi, W., et al. (2015). limma powers differential expression analyses for RNA-sequencing and microarray studies. Nucleic Acids Res. 43:e47. doi: 10.1093/nar/gkv007
Ruzickova, M., Slaney, C., Garnham, J., and Alda, M. (2003). Clinical features of bipolar disorder with and without comorbid diabetes mellitus. Can. J. Psychiatry 48, 458–461. doi: 10.1177/070674370304800705
Seol, W., Chung, M., and Moore, D. D. (1997). Novel receptor interaction and repression domains in the orphan receptor SHP. Mol. Cell. Biol. 17, 7126–7131. doi: 10.1128/MCB.17.12.7126
Singh, U., and Westermark, B. (2011). CGGBP1 is a nuclear and midbody protein regulating abscission. Exp. Cell Res. 317, 143–150. doi: 10.1016/j.yexcr.2010.08.019
Singh, U., and Westermark, B. (2015). CGGBP1–an indispensable protein with ubiquitous cytoprotective functions. Ups. J. Med. Sci. 120, 219–232. doi: 10.3109/03009734.2015.1086451
Steardo, L. Jr., Luciano, M., Sampogna, G., Carbone, E. A., Caivano, V., et al. (2020). Clinical severity and calcium metabolism in patients with bipolar disorder. Brain Sci. 10:417. doi: 10.3390/brainsci10070417
Stelzl, U., Worm, U., Lalowski, M., Haenig, C., Brembeck, F. H., Goehler, H., et al. (2005). A human protein-protein interaction network: a resource for annotating the proteome. Cell 122, 957–968. doi: 10.1016/j.cell.2005.08.029
Suh, Y. H., Kim, S. Y., Lee, H. Y., Jang, B. C., Bae, J. H., Sohn, J. N., et al. (2004). Overexpression of short heterodimer partner recovers impaired glucose-stimulated insulin secretion of pancreatic beta-cells overexpressing UCP2. J. Endocrinol. 183, 133–144. doi: 10.1677/joe.1.05675
Sun, K., Jiang, P., Chan, K. C. A., Wong, J., Cheng, Y. K. Y., Liang, R. H. S., et al. (2015). Plasma DNA tissue mapping by genome-wide methylation sequencing for noninvasive prenatal, cancer, and transplantation assessments. Proc. Natl. Acad. Sci. U.S.A. 112, E5503–E5512. doi: 10.1073/pnas.1508736112
Uddin, M., Galea, S., Chang, S. C., Aiello, A. E., Wildman, D. E., de los Santos, R., et al. (2011). Gene expression and methylation signatures of MAN2C1 are associated with PTSD. Dis. Markers 30, 111–121. doi: 10.1155/2011/513659
Veldic, M., Caruncho, H. J., Liu, W. S., Davis, J., Satta, R., Grayson, D. R., et al. (2004). DNA-methyltransferase 1 mRNA is selectively overexpressed in telencephalic GABAergic interneurons of schizophrenia brains. Proc. Natl. Acad. Sci. U.S.A. 101, 348–353. doi: 10.1073/pnas.2637013100
Veldic, M., Millischer, V., Port, J. D., Ho, A. M., Jia, Y. F., Geske, J. R., et al. (2019). Genetic variant in SLC1A2 is associated with elevated anterior cingulate cortex glutamate and lifetime history of rapid cycling. Transl. Psychiatry 9:149. doi: 10.1038/s41398-019-0483-9
Wan, J. C. M., Massie, C., Garcia-Corbacho, J., Mouliere, F., Brenton, J. D., Caldas, C., et al. (2017). Liquid biopsies come of age: towards implementation of circulating tumour DNA. Nat. Rev. Cancer 17, 223–238. doi: 10.1038/nrc.2017.7
Wang, L., Liu, J., Saha, P., Huang, J., Chan, L., Spiegelman, B., et al. (2005). The orphan nuclear receptor SHP regulates PGC-1alpha expression and energy production in brown adipocytes. Cell Metab. 2, 227–238. doi: 10.1016/j.cmet.2005.08.010
Xiao, T., Xu, Z., Zhou, Y., Zhang, H., Geng, J., Liang, Y., et al. (2019). Loss of TP53I11 enhances the extracellular matrix-independent survival by promoting activation of AMPK. IUBMB Life 71, 183–191. doi: 10.1002/iub.1949
Xiong, X. F., Li, H., and Cao, E. H. (2007). PIG11 protein binds to DNA in sequence-independent manner in vitro. Biochem. Biophys. Res. Commun. 358, 29–34. doi: 10.1016/j.bbrc.2007.04.048
Yang, F., He, Y., Liu, H. X., Tsuei, J., Jiang, X., Yang, L., et al. (2014). All-trans retinoic acid regulates hepatic bile acid homeostasis. Biochem. Pharmacol. 91, 483–489. doi: 10.1016/j.bcp.2014.08.018
Zeng, H., He, B., Yi, C., and Peng, J. (2018). Liquid biopsies: DNA methylation analyses in circulating cell-free DNA. J. Genet. Genom. 45, 185–192. doi: 10.1016/j.jgg.2018.02.007
Keywords: bipolar disorder, rapid cycling, plasma, cell-free DNA, methylomics, microarray
Citation: Ho AM-C, Winham SJ, McCauley BM, Kundakovic M, Robertson KD, Sun Z, Ordog T, Webb LM, Frye MA and Veldic M (2021) Plasma Cell-Free DNA Methylomics of Bipolar Disorder With and Without Rapid Cycling. Front. Neurosci. 15:774037. doi: 10.3389/fnins.2021.774037
Received: 10 September 2021; Accepted: 01 November 2021;
Published: 30 November 2021.
Edited by:
Hector J. Caruncho, University of Victoria, CanadaReviewed by:
Carlos Spuch, Instituto de Investigación Sanitaria Galicia Sur (IISGS), SpainMihi Yang, Sookmyung Women’s University, South Korea
Copyright © 2021 Ho, Winham, McCauley, Kundakovic, Robertson, Sun, Ordog, Webb, Frye and Veldic. This is an open-access article distributed under the terms of the Creative Commons Attribution License (CC BY). The use, distribution or reproduction in other forums is permitted, provided the original author(s) and the copyright owner(s) are credited and that the original publication in this journal is cited, in accordance with accepted academic practice. No use, distribution or reproduction is permitted which does not comply with these terms.
*Correspondence: Marin Veldic, Veldic.Marin@mayo.edu