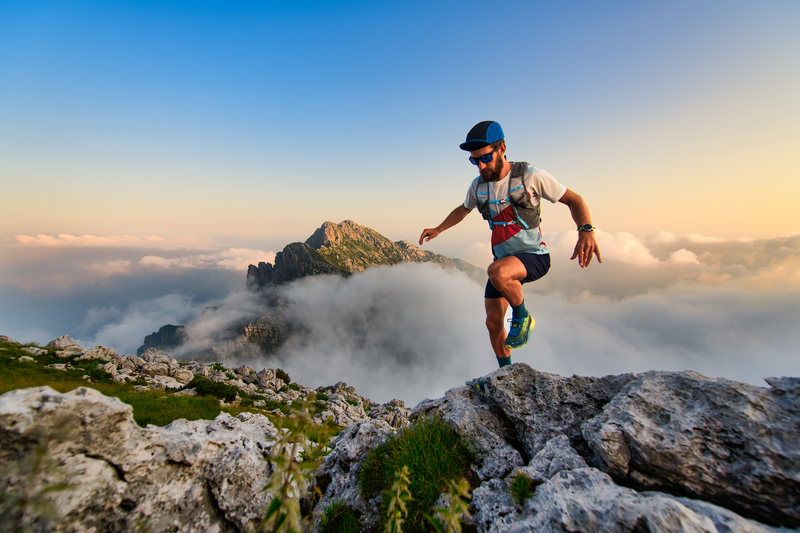
94% of researchers rate our articles as excellent or good
Learn more about the work of our research integrity team to safeguard the quality of each article we publish.
Find out more
ORIGINAL RESEARCH article
Front. Neurosci. , 20 January 2022
Sec. Sleep and Circadian Rhythms
Volume 15 - 2021 | https://doi.org/10.3389/fnins.2021.765775
This article is part of the Research Topic The underlying relationship between sleep and neurodegenerative diseases View all 9 articles
The insular cortex is a cortical regulatory area involved in dyspnea, cognition, emotion, and sensorimotor function. Previous studies reported that obstructive sleep apnea (OSA) shows insular tissue damage and abnormal functional connections for the whole insula. The insula can be divided into different subregions with distinct functional profiles, including the ventral anterior insula (vAI) participating in affective processing, dorsal anterior insula (dAI) involved in cognitive processing, and posterior insula (PI) involved in the processing of sensorimotor information. However, the functional connectivity (FC) of these insular subregions in OSA has yet to be established. Hence, the purpose of this study was to explore the resting-state FC of the insular subregions with other brain areas and its relationship with clinical symptoms of OSA. Resting-state functional magnetic resonance imaging data from 83 male OSA patients and 84 healthy controls were analyzed by whole-brain voxel-based FC using spherical seeds from six insular subregions, namely, the bilateral vAI, dAI, and PI, to identify abnormalities in the insular subregions network and related brain regions. Ultimately, the Pearson correlation analysis was carried out between the dysfunction results and the neuropsychological tests. Compared with the healthy control group, the OSA patients exhibited disturbed FC from the dAI to areas relevant to cognition, such as the bilateral cerebellum posterior lobe, superior frontal gyrus, right middle frontal gyrus and middle temporal gyrus; decreased FC from the vAI to areas linked with emotion, such as the bilateral fusiform gyrus, superior parietal lobule, precuneus and cerebellum posterior lobe; and abnormal FC from the PI to the brain regions involved in sensorimotor such as the bilateral precentral gyrus, right superior/middle temporal gyrus and left superior frontal gyrus. The linear regression result showed that the apnea-hypopnea index was positively correlated with the increased FC between the right PI and the right precuneus (after Bonferroni correlation, P < 0.001) In conclusion, the abnormal FC between insular subregions and other brain regions were related to cognitive, emotional and sensorimotor networks in OSA patients. These results may provide a new imaging perspective for further understanding of OSA-related cognitive and affective disorders.
Obstructive sleep apnea (OSA) is a sleep-related breathing disorder characterized by recurrent complete or partial pharyngeal collapse during sleep (Jordan et al., 2014). These episodes bring about intermittent nocturnal apnea and hypoxia, hypercapnia, and sleep fragmentation, negatively impacting cognitive, neuropsychological, sensorimotor, and affective functioning (Vanek et al., 2020; Beaudin et al., 2021; Lee et al., 2021). Evidence has displayed that the occurrence of OSA is related to abnormal structure and function in the central nervous system, especially impairments in the insular cortex (Li et al., 2014; Rosenzweig et al., 2014). However, why is the insula stressed in the progression of OSA?
The human insular cortex, which accounts for less than 2% of the total cortical surface area, is extensively connected with the frontal, temporal, cerebellar and limbic regions (Ghaziri et al., 2017) and is implicated in a large number of widely different functions, ranging from cognition and speech production to the processing of emotions (Nieuwenhuys, 2012), all of which, coincidentally, are important characteristics in OSA. An increasing number of neuroimaging studies have indicated that prominent activation of the insular cortex is involved in sleep-disordered breathing (von Leupoldt and Dahme, 2005; Davis et al., 2008; Schon et al., 2008), which occurs in OSA due to airway collapse. Specifically, activation of the insular cortex increases the control of the habenula on the raphe nucleus, reducing the release of 5-hydroxytryptamine, decreasing muscle tension of the genioglossus and blocking the airway (Wang et al., 2014; Li et al., 2015). A meta-analysis highlighted the importance of the insular cortex in somatosensory, neurocognitive, perceptual, and affective deficits in OSA subjects (Tahmasian et al., 2016). Forasmuch, insular cortex, a multimodal integration region, plays a crucial role in the development of OSA, which is in line with the perspective of Cui et al. (2012). Damage to the insula may be related to cognitive, neuropsychological, sensorimotor and emotional impairments.
Interestingly, some research in animals and humans has demonstrated that the insular cortex is not homogeneous and that it can be functionally subdivided into meaningful subregions with different connectivity patterns (Kurth et al., 2010; Deen et al., 2011). The dorsal anterior insula (dAI) broadly maps onto cognitive tasks involving attention, memory, speech, and executive control. The ventral anterior insula (vAI) is associated with emotion, chemosensation, and autonomic function, and the posterior insula (PI) is linked primarily to pain, sensorimotor function and language, according to previous studies (Chang et al., 2013; Uddin et al., 2014). Therefore, anomalies in different insular subregions may result in impaired local functional magnetic resonance imaging (fMRI) responses to evoked cognitive function, emotions, sensorimotor function and dyspnea. In fact, abnormalities in the insula have been revealed in studies of brain functional activity in OSA subjects. For example, Peng et al. (2014) found that higher regional homogeneity (ReHo) occurred in the insula of male patients with severe OSA. In addition, altered limbic-striatal-cortical functional connectivity was reported in studies of altered hippocampal resting-state functional networks in OSA (Song et al., 2018; Kang et al., 2020; Zhou et al., 2020). Although ReHo and resting-state functional connectivity (FC) are both based on resting-state fMRI, ReHo, a method for analyzing the coherence and similarities of intraregional spontaneous low-frequency (0.01–0.08 Hz) signal fluctuations was used to detect unpredicted hemodynamic responses (Zang et al., 2004). Resting-state FC, focusing on the statistical correlation between signals in the whole brain or regional brain areas (Fox and Raichle, 2007), has shown substantial clinical research potential for exploring synchronous brain activity, which is also the highlight of the current research. Region-of-interest (ROI) studies based on resting-state FC have been considered sensitive and reliable (Hampson et al., 2002), and these techniques have been widely applied in various objective analyses of brain functional activity, varying from sleep disorders to neuropsychiatric diseases (Lei et al., 2019; Guo et al., 2020). Thus, resting-state FC studies based on ROI may be incredibly useful in evaluating insular FC integrity in patients with OSA. Nevertheless, the insula is rarely regarded as an ROI in studies of resting-state FC in OSA; A small number of studies that have focused on the insular cortex (Zhang et al., 2015; Park et al., 2016a), regarded it as a whole. The characteristics of rs-FC between different subregions of the insula and all other brain regions and the neural mechanisms underlying OSA remain unclear.
Consequently, based on the above reasons, we hypothesized that FC integrity in insular subregions would be impaired in OSA subjects in brain regions that regulate affective, cognitive and sensorimotor function. To test this hypothesis, we first investigated the rs-FC patterns of insular subregions with other brain regions using spherical seed ROI-based resting-state FC analysis in newly diagnosed untreated OSA participants and healthy controls (HCs) and then explored the differences in functional connectivity of insular subregions with other brain regions across the whole brain between the two groups. Subsequently, we evaluated possible correlations between aberrant rs-FC changes in insular subregions and OSA severity as well as neuropsychological performance to analyze the potential neuroimaging process of cognitive deficits in OSA patients.
Ninety-two newly diagnosed untreated male patients with OSA and eighty-nine male HCs matched in age and education were enrolled in this study. All patients were enrolled from the Sleep Monitoring Room at the Respiratory Department of the First Affiliated Hospital of Nanchang University. HCs were recruited through local advertisements. Patients were jointly diagnosed by 3 experienced respiratory doctors based on the criteria from the Clinical Practice Guideline for Diagnostic Testing for Adult Obstructive Sleep Apnea proposed by the American Academy of Sleep Medicine (AASM) in 2017 (Kapur et al., 2017). Patients with moderate to severe OSA, indicated by an apnea-hypopnea index (AHI) ≥15, were selected for this study. All participants were males, right-handed, native Chinese speakers, aged from 22 to 60 years. The exclusion criteria for all subjects were as follows: (1) sleep disorders other than OSA; (2) history of cardiovascular diseases, respiratory diseases, diabetes, hypothyroidism or central nervous system disorders that included neurodegenerative disease, epilepsy, depression, tumor, brain trauma or infarction; (3) abuse of alcohol or illicit drugs or current intake of psychoactive medications; (4) MRI contraindications, such as claustrophobia, metallic implants, or devices in the body; and (5) suboptimal imaging data quality. The HCs did not have a current or lifetime history of sleep-related disorders or a history of psychotic, mood, or sleep-related disorders in first-degree relatives. Finally, 83 patients with OSA and 84 HCs were included. This study was approved by the Medical Research Ethics Committee of The First Affiliated Hospital of Nanchang University, and each participant provided written informed consent.
To make an accurate diagnosis while excluding sleep breathing disorders other than OSA or potential sleep disturbances in HCs, all HCs and OSA subjects were required to undergo overnight polysomnography (PSG) using a Respironics LE-Series physiological monitoring system (Alice 5 LE, Respironics, Orlando, FL, United States). The day before PSG, all participants were asked to avoid hypnotics, alcoholic beverages and coffee. The PSG monitor assessed standard electroencephalogram (EEG) derivations from frontal, central, and occipital regions, F4/M1, C4/M1, and O2/M1, and back-up derivations, F3/M2, C3/M2, and O1/M2; electrooculograms (EOGs) obtained from the cornea and retina; chin electromyogram (EMG) obtained from three chin electrodes and one placed on the middle of the right anterior tibialis; electrocardiogram (ECG); body position; thoracic and abdominal respiratory movements; oral and nasal airflow; snoring; and oxygen saturation (SaO2). Total sleep time, sleep latency, sleep efficiency, sleep stages, arousal, and respiratory events were likewise recorded. PSG was conducted from 10 PM to 6 AM the next morning.
Obstructive apnea was described as a continuous reduction in airflow ≥90% for ≥10 s along with evident respiratory effort. Hypopnea was identified as a 30% or greater drop in airflow for ≥10 s accompanied by ≥4% oxygen desaturation or alternatively, a 50% or greater drop in airflow lasting ≥10 s accompanied by ≥3% oxygen desaturation or EEG arousal. The AHI was obtained from the aggregate numbers of apnea and hypopnea events per hour during sleep. According to the American Academy of Sleep Medicine Clinical Practice Guideline for Adult Obstructive Sleep Apnea (Berry et al., 2012, 2017), an AHI from 5 to 15 was diagnosed as mild; an AHI ≥ 15/h but <30/h was considered moderate, and an AHI ≥ 30/h was considered severe OSA.
The Epworth Sleepiness Scale (ESS) assess contains eight different categories and was used to evaluate self-reported answers to sleep questions regarding daytime sleepiness in OSA and control subjects. ESS total scores can range from 0 to 24 (each category scored from 0 to 3), and scores of 0, 6, 11, and 16 correspond to four different sleepiness levels (Johns, 1991). The Montreal Cognitive Assessment (MoCA), assessing executive functions, orientation, abstraction, attention, calculation, conceptual thinking, language, and memory, was used to assess cognitive function. A total MoCA score lower than 26 indicated cognitive impairment (Chen et al., 2016).
All images were captured on a 3.0 Tesla MRI system with an 8-channel phased-array head coil (Siemens, Munich, Germany). To reduce patient head movement and minimize scanner noise, we used foam pads and earplugs. First, conventional T1-weighted imaging (repetition time (TR) = 250 ms, echo time (TE) = 2.46 ms, thickness = 5 mm, gap = 1.5 mm, field-of-view (FOV) = 220 mm × 220 mm, slices = 19) and T2-weighted imaging (TR = 4,000 ms, TE = 113 ms, thickness = 5 mm, gap = 1.5 mm, FOV = 220 mm × 220 mm, slices = 19) were performed. rs-fMRI data were collected using a Gradient-Recalled Echo-planar Imaging (EPI) pulse sequence with the following parameters: TR = 2,000 ms, TE = 30 ms, flip angle = 90°, FOV = 230 mm × 230 mm, matrix = 64, thickness = 4 mm, gap = 1.2 mm. Each brain volume consisted of 30 axial sections, and each functional run comprised 240 volumes. During the rs-fMRI scans, all subjects were asked to close their eyes, stay as still as possible, not think about anything specific, and not fall asleep. Finally, high-resolution three-dimensional T1-weighted images were obtained by using a spoiled gradient-recalled echo sequence (TR = 1,900 ms, TE = 2.26 ms, thickness = 1.0 mm, gap = 0.5 mm, FOV = 250 mm × 250 mm, matrix = 256 × 256, flip angle = 9°, 176 sagittal slices). The images were read jointly by two senior radiologists to rule out obvious brain lesions.
MRIcro software1 was used to check the resting-state fMRI data and discard suboptimal ones. resting-state fMRI data were preprocessed with the Data Processing & Analysis for Brain Imaging toolbox DPABI2 (Yan et al., 2016), which was run on MATLAB 2018b (Mathworks, Natick, MA, United States). First, the first 10 time points were removed. Then the remaining 230 volumes were slice-time corrected and three-dimensional head-motion rectified. Three OSA patients and two HCs were excluded because the maximum displacement in any direction (x, y, or z) was more than 2.0 mm, or the angular rotation on any axis exceeded 2.0°, or the framewise displacement (FD) a relative displacement measure (Power et al., 2012) of any of the 230 volumes exceeded 2.5 standard deviations during the entire fMRI scanning process. Then, all the functional data were spatially normalized to the Montreal Neurological Institute (MNI) template using non-linear transformation procedures with SPM12 (Pirzada et al., 2020), resampled to 3 mm × 3 mm × 3 mm voxels, and smoothed with a 6-mm full-width at half-maximum (FWHM) filter. Finally, a temporal filter (0.01–0.08 Hz) was utilized to suppress the effects of low-frequency drift and high-frequency noise. To further reduce the influence of confounding factors, a multiple regression method was performed to regress interference signals, including the mean time series of all voxels from white matter, cerebrospinal fluid, global signals and Friston 24-parameters head motion (6 head motion parameters, 6 head motion parameters one time point before, and the 12 corresponding squared items) (Friston et al., 1996; Saad et al., 2012; Satterthwaite et al., 2013).
The whole insula was divided into three different functional subregions based on previous cluster analysis of the resting-state FC (Kurth et al., 2010; Deen et al., 2011; Uddin et al., 2014). According to the previous study (Li et al., 2019), the six subregions of the bilateral insula were defined as spherical seed ROIs with a radius of 6 mm and the following MNI coordinates: left vAI (−33, 13, −7), right vAI (32, 10, −6), left dAI (−38, 6, 2), right dAI (35, 7, 3), left PI (−38, −6, 5), and right PI (35, −11, 6).
The functional connectivity with different subregions ROIs was calculated by DPABI (see text footnote 2). First, the time series of each spherical seed region was extracted, that is, the average value of the fMRI time series of all voxels in the region. For each seed region, the Pearson correlation coefficients of the time series with that of all other voxels in the brain were calculated. The resulting correlation matrix is the whole-brain FC map of each subject. Finally, the Fisher r-to-z transform was utilized to transform the correlation graph into a z-value map to improve the normality of the correlation coefficients.
The demographic and clinical data of the two groups were compared by independent sample t-tests with Statistical Package for the Social Sciences version 24.0 (SPSS, Chicago, IL, United States); when p < 0.05, the differences are considered significant.
For the rs-FC between each ROI and the remaining whole brain voxels, one-sample t-tests were analyzed in two groups to identify spatial distribution, respectively. Then, analysis of covariance was applied to analyze intergroup differences, with body mass index (BMI) and age as covariates using DPABI (see text footnote 2) software in MATLAB 2018b (Mathworks, Natick, MA, United States). The results were corrected using AlphaSim3 implemented in DPABI Viewer part with p < 0.001 and were reported using REST V1.84 (Song et al., 2011). The resulting z-value maps were overlaid on the rendered views using BrainNet Viewer, and the anatomy of the surviving brain regions was reported using xjView software5.
To examine whether there is a relationship between the abnormal FC and clinical evaluation. First, we preserved the abnormal brain regions as masks. We then extract the functional connection signal z-value for each mask in every subject using REST V1.8 (see text footnote 4). Linear regression analysis was calculated between the averaged z-values of the abnormal brain regions and clinical variables, with BMI and age as covariates using SPSS version 24.0. P < 0.001 was considered to be statistically significant for each set of independent variables (10 clinical variables × 36 regions) after the Bonferroni correlation.
The demographic and clinical data from each group are summarized in Table 1. There were no significant differences in age, education years, total sleep time, stage 2 sleep or mean FD between the OSA patients and HCs (P > 0.05). Compared with the HC group, the OSA group had significantly higher BMI, AHI, stage 1 sleep, SaO2 <90%, AI and ESS scores and significantly lower sleep efficiency, stage 3 + 4 sleep, rapid eye movement REM sleep, nadir SaO2 and MoCA scores.
The OSA patients and HCs showed similar resting-state functional connectivity patterns for the different insular functional subregions, as shown in Figures 1A,B, 2A,B, 3A,B.
Figure 1. The spatial distribution of the FC maps of the bilateral dAI in the two groups and between-group comparisons of the rs-FC of the bilateral dAI. The FC patterns of the bilateral dAI in the HC and OSA groups are shown in (A,B), respectively. Color bars indicate z-values; warmer colors indicate higher z-values relative to the whole-brain average, while colder colors indicate lower z-values. (C) Denotes the altered FC based on the left dAI seed in OSAs relative to HCs. (D) Denotes the altered FC based on the right dAI seed in OSAs relative to HCs (voxel-level P < 0.001, AlphaSim corrected). Color bars indicate t-scores; warm colors indicate areas where the FC value in OSAs is greater than that in HCs, while cold colors indicate the opposite. HCs, healthy controls; OSAs, patients with obstructive sleep apnea; dAI, dorsal anterior insula; FC, functional connectivity; SFG, superior frontal gyrus; MFG, middle frontal gyrus; MTG, middle temporal gyrus; PreCG, precentral gyrus; CPL, cerebellum posterior lobe; CAL, cerebellum anterior lobe; L, left; R, right; A, anterior; P, posterior.
Figure 2. The spatial distribution of the FC maps of the bilateral vAI in the two groups and between-group comparisons of the rs-FC of the bilateral vAI. The FC patterns of the bilateral vAI in the HC and OSA groups are shown in (A,B), respectively. Color bars indicate z-values; warmer colors indicate higher z-values relative to the whole-brain average, while colder colors indicate lower z-values. (C) Denotes the altered FC based on the left vAI seed in OSAs relative to HCs. (D) Denotes the altered FC based on the right vAI seed in OSAs relative to HCs (voxel-level P < 0.001, AlphaSim corrected). Color bars indicate t-scores; warm colors indicate areas where the FC value in OSAs is greater than that in HCs, while cold colors indicate the opposite. HCs, healthy controls; OSAs, obstructive sleep apnea patients; vAI, ventral anterior insula; FC, functional connectivity; STG, superior temporal gyrus; ITG, inferior temporal gyrus; LN, lentiform nucleus; Put, putamen; PreCG, precentral gyrus; PostCG, postcentral gyrus; CPL, cerebellum posterior lobe; Fus, fusiform gyrus; SPL, superior parietal lobule; Pre, precuneus; L, left; R, right; A, anterior; P, posterior.
Figure 3. The spatial distribution of the FC maps of the bilateral PI in the two groups and between-group comparisons of the rs-FC of the bilateral PI. The FC patterns of the bilateral PI in the HC and OSA groups are shown in (A,B), respectively. Color bars indicate z-values; warmer colors indicate higher z-values relative to the whole-brain average, while colder colors indicate lower z-values. (C) Denotes the altered FC based on the left PI seed in OSAs relative to HCs. (D) Denotes the altered FC based on the right PI seed in OSAs relative to HCs (voxel-level P < 0.001, AlphaSim corrected). Color bars indicate t-scores; warm colors indicate areas where the FC value in OSAs is greater than that in HCs, while cold colors indicate the opposite. HCs, healthy controls; OSAs, obstructive sleep apnea patients; PI, posterior insula; FC, functional connectivity; SFG, superior frontal gyrus; MeFG, medial frontal gyrus; STG, superior temporal gyrus; MTG, middle temporal gyrus; PreCG, precentral gyrus; Pre, precuneus; CPL, cerebellum posterior lobe; L, left; R, right; A, anterior; P, posterior.
The results of intergroup rs-FC comparisons at the voxel-based whole-brain level based on bilateral dAI spherical seed points are displayed in Figure 1 and Table 2. When taking the left dAI as the seed, the patients with OSA showed significantly lower rs-FC with the bilateral cerebellum posterior lobe (CPL) and left cerebellum anterior lobe but significantly higher rs-FC with the bilateral superior frontal gyrus (SFG), right middle frontal gyrus (MFG), and left precentral gyrus (PreCG) than the HCs (Figure 1C). When taking the right dAI as the seed, the patients with OSA showed significantly lower rs-FC with the left CPL but significantly higher rs-FC with the right middle temporal gyrus (MTG) and left PreCG than the HCs (Figure 1D).
Table 2. Brain areas showing functional connectivity differences with insular subdivisions between OSA patients and HCs.
The results of intergroup rs-FC comparisons at the voxel-based whole-brain level based on bilateral vAI seed points are shown in Figure 2 and Table 2. When taking the left vAI as the seed, the patients with OSA had significantly lower rs-FC with the bilateral CPL, fusiform gyrus, superior parietal lobule, and precuneus but significantly higher rs-FC with the bilateral superior temporal gyrus (STG), right lentiform nucleus and putamen, and left PreCG and postcentral gyrus than the HCs (Figure 2C). When taking the right vAI as the seed, the patients with OSA showed significantly lower rs-FC with the right CPL but significantly higher rs-FC with the right inferior temporal gyrus, left lentiform nucleus, putamen and precuneus than the HCs (Figure 2D).
The results of intergroup rs-FC comparisons at the voxel-based whole-brain level based on bilateral PI seed points are displayed in Figure 3 and Table 2. When taking the left PI as the seed, the patients with OSA showed significantly lower rs-FC with the bilateral CPL but significantly higher rs-FC with the right STG and MFG and left SFG than the HCs (Figure 3C). When taking the right PI as the seed, the patients with OSA showed significantly lower rs-FC with the bilateral CPL but significantly higher rs-FC with the bilateral PreCG and right MTG, STG, and precuneus than the HCs (Figure 3D).
To explore symptom-related insular functional connectivity, linear correlation analysis between rs-FC and clinical variables was performed. Table 3 exhibited statistically significant results with P < 0.05 before multiple comparison correction. However, only the AHI was positively correlated with the increased FC between the right PI and the right precuneus after Bonferroni correlation with P < 0.001 among the OSA patients. There was no significant correlation between FC values and the clinical and neuropsychologic variables in the control group.
Table 3. Significant associations between insular functional connectivity and clinical characteristic in OSA patients.
To the best of our knowledge, this study is the first to explore the FC of insular subregions using spherical seed functional connectivity analysis in OSA patients. Our results showed that abnormal functional connections of the vAI, dAI, and PI were distributed across different brain regions separately involving emotion, cognition (executive function, attention, and memory) and sensorimotor function. Structural or functional damage has also been shown in these brain regions in previous studies on OSA, and the abnormal functional connections in these areas were related to sleep and neuropsychological variables, which is consistent with our hypothesis. Additionally, we found that there were differential FC patterns between the two sides of the insula, which was similar to previous laterality results observed in this part of the brain (Van Essen et al., 2012), which may be due to the distinct order of bilateral insular lobe development: the surface area of the left lobe is larger than that of the right lobe, and the right lobe stops growing earlier than the left lobe (Cauda et al., 2011). In addition, resting-state brain activity may be affected by obesity, age and sex, the main risk factors for OSA, as shown in previous studies (Black et al., 2014; Li et al., 2016; Kumar et al., 2021). In our study, we also found that BMI in the OSA patients was significantly higher than that in HCs. To avoid the influence of the above three factors on the rs-FC results between OSA and HC patients, we recruited only adult male OSA patients and used BMI and age as covariates.
In the current study, we found that compared with HCs, OSA patients showed abnormal FC between the dAI and certain cognitive regulatory brain regions, including the CPL, SFG, MFG, and MTG. The dAI is considered an influential functional center of the human brain and is often described as a “cognitive” area (Uddin et al., 2014). Growing evidence suggests that respiratory and sleep disorders in OSA patients lead to cognitive impairment, encompassing language, attention, executive control and so on (Beaudin et al., 2021). Newly emerging evidence has demonstrated that damage to the cerebellar cortex, including among abnormally connected brain regions, leads to cognitive impairment (Ramnani, 2012). The CPL is associated with cognitive processing in OSA (Shi et al., 2017).
Based on the results of Li et al. (2013) and Shen et al. (2020), the SFG, a connecting node between the central executive network and default mode network (DMN), is linked to the cognitive control network and the cognitive executive network. The MFG, a location where the ventral and dorsal attention networks converge, is involved in language fluency, object naming and directed attention and is part of the cognitive executive network (Japee et al., 2015; Viñas-Guasch and Wu, 2017). The MTG has been proven to be an important structure in the memory consolidation process and a crucial node in the language network, as it participates in semantic control and social cognition (Acheson and Hagoort, 2013; Chen Y. et al., 2020). As one piece of evidence, we showed exaggerated connection weights from the left dAI to the bilateral SFG, right MFG and left PreCG and from the right dAI to the right MTG and left PreCG. Zhang et al. (2013) discovered impaired FC of the PreCG in OSA patients and confirmed structural and functional disruptions in the anterior DMN containing the SFG, and speculated these damages may be a probable candidate mechanism for cognitive and emotional disorders in patients with OSA. Furthermore, the increase in FC in the temporal gyrus of OSA patients has been associated with neurocognitive impairment, as affirmed by the study of Zhou et al. (2020). The brain areas with abnormal FC in our study overlapped with those previously reported to show structural or functional aberrations in OSA, such as the cerebellum, frontal lobe and temporal lobe, which are distributed in the cognitive control circuit along with the dAI and are responsible for memory, attention, language and executive control (Park et al., 2016a; Caporale et al., 2021). The exaggerated FC may bring about synaptic plasticity, which could alter the functional compensatory response in the early stages of cognitive impairments.
In addition, there was some degree of correlation between the MoCA score and FC of the left dAI to the right SFG (t = −2.051, P = 0.043), the left dAI to the left SFG (t = −2.344, P = 0.022), however, which only present before the Bonferroni correction. One possible explanation is that the OSA patients were in the early stage of neurological abnormalities, with mild cognitive symptoms and a small decrease in MoCA score, similar to the findings of several previous studies (Park et al., 2016a; Yu et al., 2019).
Patients with OSA often show emotional abnormalities, such as anxiety or depression (Kim et al., 2019). The vAI participates in the experience of negative emotions and shows abnormal activation in the studies of affect and emotion (Gogolla, 2017). In addition, a neuroimaging study revealed that the vAI is connected to the area representing the sensory input associated with emotional expression (Touroutoglou et al., 2012). Additionally, the connectivity of the vAI with other brain regions is altered in anxiety disorders (Grupe and Nitschke, 2013). We found altered FC between the vAI and regulatory networks of emotion including the CPL, superior parietal lobule, precuneus, superior/inferior temporal gyrus, fusiform gyrus, precentral and postcentral gyrus. The CPL is linked to the expression of cognitive sentiment, and emotional motor responses (Strata, 2015), with increased activation in the state of anxiety or depression (Pico-Perez et al., 2017), and its dysfunction has been observed in OSA (Yu et al., 2019). Bae et al. (2019) found that emotional perception test performance was associated with brain connectivity from the fusiform gyrus to the insula. Brain regions, incorporating the parietal lobe, frontal lobe, precuneus, temporal lobe and insula are activated in patients with depressive disorders or OSA patients with depression and anxiety symptoms (Pico-Perez et al., 2017; Song et al., 2018). Significantly, compared with those without emotional symptoms, OSA subjects with emotional symptoms have shown exaggerated damage in specific brain regions, including the insula, parietal lobe and temporal lobe (Cross et al., 2008; Kumar et al., 2009), which contain the emotion-related abnormal brain regions revealed in our results.
Reduced activation may reflect a defective response and dysfunction between the vAI and emotionally related areas of the bilateral CPL, superior parietal lobule, precuneus, and fusiform gyrus of OSA subjects. While, the exaggerated FC may bring about synaptic plasticity, which could alter the functional compensatory response in the early stages of mood disorders in OSA patients. It seems the left vAI plays a more important role than the right.
In this study, disrupted FC was exhibited between the PI and PreCG, STG, MTG, SFG, medial frontal gyrus, and precuneus. Caused by upper respiratory tract muscle abnormalities in OSA, hypoxemia further disrupts sensorimotor function (Eckert et al., 2011). Previous studies have found structural and functional connectivity disorders in sensorimotor areas in patients with OSA. The PI is functionally connected with the motor, somatosensory and temporal cortices, which are associated with skeletal movement, body orientation, environmental monitoring and response selection, as demonstrated by FC research involving the insula (Cauda et al., 2011). In our study, significantly increased connection weights were observed between the bilateral PreCG, right MTG, STG and precuneus and the right PI, and increased connection weights were observed between the right STG, medial frontal gyrus, and left SFG and the left PI. The bilateral PreCG, namely, the premotor cortex, is part of one of the classic sensorimotor subregions in charge of complex motor task signal processing (Cooke and Graziano, 2004; Gao et al., 2020). Zhang et al. (2013) identified deficits in the sensorimotor-related brain networks associated with the PreCG and medial prefrontal cortex in patients with OSA as predictors of impaired motor function. Although the other abnormally connected brain regions are not in sensorimotor areas, they are important for different aspects of sensorimotor control. Neuroimaging data have shown that the temporal gyrus acts as a hub for action-related information and processing and participates in language control, linking semantic knowledge about meaningful action to sensorimotor activity (Rinat et al., 2020) and contributing to the kinesthetic perception of joint motion and higher-order sensory integration (Goossens et al., 2018). Abnormal blood-oxygen-level-dependent signals appear in the medial frontal gyrus and precuneus during hand and foot movement (Nakata et al., 2019). In addition, the correlation results indicated that the connection between the right precuneus and right PI was positively correlated with the AHI. Interestingly, an authoritative study demonstrated that the insular lobe acts as an intermediary in the sensorimotor-insular-frontal lobe loop, promoting the interaction between the primary sensorimotor cortex and higher regions (Namkung et al., 2017). Disruption of the FC in the sensorimotor area-posterior insula in OSA may signify abnormal sensorimotor activity, deserving our attention.
Surprisingly, our results revealed that all insular subregions had impaired connections with the cerebellum. Several neuroimaging results have also supported the idea that the cerebellum is the most structurally and functionally affected area in OSA subjects (Macey et al., 2008; Kumar et al., 2012; Park et al., 2016b). The cerebellum is regarded as having a considerable border role in sensory and perceptual processing (Baumann et al., 2015) that is not only germane to motor function (Goossens et al., 2018) but also related to cognitive processing and emotional control (Moreno-Rius, 2018). Lobule VI is part of the neural circuit of fear and anxiety (Lange et al., 2015). In addition, the cerebellum is involved in maintaining sleep, a lack of which may disrupt cerebellar function (Shi et al., 2017). Whether cerebellar abnormalities can truly explain the clinical symptoms of OSA from the perspective of neuroimaging requires further exploration, and could possibly be the focus of our next study. A recent study about cerebellar functional changes in OSA enhanced our confidence (Park et al., 2021).
The right AI is the critical node of the salience network (Touroutoglou et al., 2016), and serves to switch between the central executive network and DMN (Sridharan et al., 2008). A study of functional separation of the right AI revealed that OSA selectively damaged the rs-FC between the AI and the DMN, which may be a substrate for cognitive impairment in OSA patients (Zhang et al., 2015). The DMN is composed of two spatially independent subnetworks: the anterior DMN and the posterior DMN. The anterior DMN contains the SFG and is mainly responsible for emotion self-reference and management; however, the posterior DMN contains the precuneus and primarily presides over cognitive processing and memory extraction (Coutinho et al., 2016). Previous studies concluded that depression and anxiety were associated with DMN alterations, which highlights the role of the DMN in emotional processing (Vicentini et al., 2017). Our results of FC between the left vAI and posterior DMN was decreased, while FC between the left dAI and anterior DMN showed increased suggested a discrete connectivity between the AI and anterior/posterior DMN, consistent with the disruption of the DMN in OSA patients (Chen et al., 2018).
Several limitations should be noted in our study. First, we recruited only adult men with OSA, given the difficulty of sample collection and convention of homogeneity. Women and children with OSA need to be included in future studies. Second, the evaluation of the emotional state was not included in this study, since only a small number of patients were carried out with emotional assessments. Fortunately, previous studies have reported emotional abnormalities in OSA patients. An emotion scale should be added to better verify our findings in future studies. Finally, external physiological recordings were not obtained during fMRI acquisition (e.g., respiratory belt and photoplethysmography). Several recent studies have indicated that fluctuations in physiological processes may lead to artificial FC (Bright et al., 2020; Chen J. E. et al., 2020; Xifra-Porxas et al., 2021). Physiological recordings could in principle help to address whether the differences in insular subregions connectivities are related to neuronal brain activity or physiological fluctuations and should be included in future studies.
In summary, the insular subregions showed extensive abnormal connectivities with other brain areas in OSA patients. These abnormal connectivities were manifested in disrupted connections from the vAI to emotional regions, from the dAI to cognitive regions, from the PI to sensorimotor and language regions, and from the AI to DMN regions, where there exist in partial functional overlap rather than complete independence. This disorganized FC associated with neuropsychological disorders, may be responsible for the evoked sensorimotor, affective, and ventilatory challenges and cognitive deficits in OSA.
The datasets presented in this article are not readily available because these data are related to the follow-up longitudinal study of our research group, and the datasets are still being replenished. Requests to access the datasets should be directed to LK, ZG9ja29uZzY2NkAxNjMuY29t.
The studies involving human participants were reviewed and approved by Ethics Committee of The First Affiliated Hospital of Nanchang University. The patients/participants provided their written informed consent to participate in this study.
DP guided and designed the MRI experiment. HL analyzed the resting-state fMRI data. LK and YS analyzed and discussed the ideas of the manuscript. LK organized the results and wrote the manuscript. LK, XL, PL, KL, WX, and YZ collected the resting fMRI data and applied for ethics approval. HL and DP reviewed and revised the manuscript. All authors contributed to the article and approved the submitted version.
This study was supported by the National Natural Science Foundation of China (Grant Nos. 81860307 and 81560285), Natural Science Foundation Project of Jiangxi Province, China (Grant Nos. 20202BABL216036, 20181ACB20023, and 20171BAB205070), Education Department Project of Jiangxi Province, China (Grant Nos. 700544006 and GJJ190133), and Department of Health Project of Jiangxi Province, China (Grant No. 20181039).
The authors declare that the research was conducted in the absence of any commercial or financial relationships that could be construed as a potential conflict of interest.
All claims expressed in this article are solely those of the authors and do not necessarily represent those of their affiliated organizations, or those of the publisher, the editors and the reviewers. Any product that may be evaluated in this article, or claim that may be made by its manufacturer, is not guaranteed or endorsed by the publisher.
We are obliged to Huizhen Xin and Honghui Yu, Department of Radiology, The First Affiliated Hospital of Nanchang University, for their assistance with patient recruitment. We also thank Wenfeng Duan, Department of Radiology, The First Affiliated Hospital of Nanchang University, for his assistance with data collection.
Acheson, D. J., and Hagoort, P. (2013). Stimulating the brain’s language network: syntactic ambiguity resolution after TMS to the inferior frontal gyrus and middle temporal gyrus. J. Cogn. Neurosci. 25, 1664–1677. doi: 10.1162/jocn_a_00430
Bae, S., Kang, K. D., Kim, S. W., Shin, Y. J., Nam, J. J., and Han, D. H. (2019). Investigation of an emotion perception test using functional magnetic resonance imaging. Comput. Methods Programs Biomed. 179:104994. doi: 10.1016/j.cmpb.2019.104994
Baumann, O., Borra, R. J., Bower, J. M., Cullen, K. E., Habas, C., Ivry, R. B., et al. (2015). Consensus paper: the role of the cerebellum in perceptual processes. Cerebellum 14, 197–220. doi: 10.1007/s12311-014-0627-7
Beaudin, A. E., Raneri, J. K., Ayas, N. T., Skomro, R. P., Smith, E. E., and Hanly, P. J. (2021). Contribution of hypercapnia to cognitive impairment in severe sleep-disordered breathing. J. Clin. Sleep Med. doi: 10.5664/jcsm.9558
Berry, R. B., Brooks, R., Gamaldo, C., Harding, S. M., Lloyd, R. M., Quan, S. F., et al. (2017). AASM scoring manual updates for 2017 (Version 2.4). J. Clin. Sleep Med. 13, 665–666. doi: 10.5664/jcsm.6576
Berry, R. B., Budhiraja, R., Gottlieb, D. J., Gozal, D., Iber, C., Kapur, V. K., et al. (2012). Rules for scoring respiratory events in sleep: update of the 2007 AASM manual for the scoring of sleep and associated events. Deliberations of the sleep apnea definitions Task Force of the American Academy of Sleep Medicine. J. Clin. Sleep Med. 8, 597–619. doi: 10.5664/jcsm.2172
Black, W. R., Lepping, R. J., Bruce, A. S., Powell, J. N., Bruce, J. M., Martin, L. E., et al. (2014). Tonic hyper-connectivity of reward neurocircuitry in obese children. Obesity 22, 1590–1593. doi: 10.1002/oby.20741
Bright, M. G., Whittaker, J. R., Driver, I. D., and Murphy, K. (2020). Vascular physiology drives functional brain networks. Neuroimage 217:116907. doi: 10.1016/j.neuroimage.2020.116907
Caporale, M., Palmeri, R., Corallo, F., Muscara, N., Romeo, L., Bramanti, A., et al. (2021). Cognitive impairment in obstructive sleep apnea syndrome: a descriptive review. Sleep Breath. 25, 29–40. doi: 10.1007/s11325-020-02084-3
Cauda, F., D’Agata, F., Sacco, K., Duca, S., Geminiani, G., and Vercelli, A. (2011). Functional connectivity of the insula in the resting brain. Neuroimage 55, 8–23. doi: 10.1016/j.neuroimage.2010.11.049
Chang, L. J., Yarkoni, T., Khaw, M. W., and Sanfey, A. G. (2013). Decoding the role of the insula in human cognition: functional parcellation and large-scale reverse inference. Cereb. Cortex 23, 739–749. doi: 10.1093/cercor/bhs065
Chen, J. E., Lewis, L. D., Chang, C., Tian, Q., Fultz, N. E., Ohringer, N. A., et al. (2020). Resting-state “physiological networks”. Neuroimage 213:116707. doi: 10.1016/j.neuroimage.2020.116707
Chen, K. L., Xu, Y., Chu, A. Q., Ding, D., Liang, X. N., Nasreddine, Z. S., et al. (2016). Validation of the Chinese version of montreal cognitive assessment basic for screening mild cognitive impairment. J. Am. Geriatr. Soc. 64, e285–e290. doi: 10.1111/jgs.14530
Chen, L. T., Fan, X. L., Li, H. J., Ye, C. L., Yu, H. H., Xin, H. Z., et al. (2018). Aberrant brain functional connectome in patients with obstructive sleep apnea. Neuropsychiatr. Dis. Treat. 14, 1059–1070. doi: 10.2147/NDT.S161085
Chen, Y., Wang, Y., Zhao, Q., Wang, Y., Lu, Y., and Zhou, C. (2020). Watching video of discrete maneuvers yields better action memory and greater activation in the middle temporal gyrus in half-pipe snowboarding athletes. Neurosci. Lett. 739:135336. doi: 10.1016/j.neulet.2020.135336
Cooke, D. F., and Graziano, M. S. A. (2004). Sensorimotor integration in the precentral gyrus: polysensory neurons and defensive movements. J. Neurophysiol. 91, 1648–1660. doi: 10.1152/jn.00955.2003
Coutinho, J. F., Fernandesl, S. V., Soares, J. M., Maia, L., Goncalves, O. F., and Sampaio, A. (2016). Default mode network dissociation in depressive and anxiety states. Brain Imaging Behav. 10, 147–157. doi: 10.1007/s11682-015-9375-7
Cross, R. L., Kumar, R., Macey, P. M., Doering, L. V., Alger, J. R., Yan-Go, F. L., et al. (2008). Neural alterations and depressive symptoms in obstructive sleep apnea patients. Sleep 31, 1103–1109.
Cui, L., Wang, J. H., Wang, M., Huang, M., Wang, C. Y., Xia, H., et al. (2012). Injection of L-glutamate into the insular cortex produces sleep apnea and serotonin reduction in rats. Sleep Breath. 16, 845–853. doi: 10.1007/s11325-011-0586-x
Davis, K. A., Cantor, C., Maus, D., and Herman, S. T. (2008). A neurological cause of recurrent choking during sleep. J. Clin. Sleep Med. 4, 586–587. doi: 10.5664/jcsm.27354
Deen, B., Pitskel, N. B., and Pelphrey, K. A. (2011). Three systems of insular functional connectivity identified with cluster analysis. Cereb. Cortex 21, 1498–1506. doi: 10.1093/cercor/bhq186
Eckert, D. J., Lo, Y. L., Saboisky, J. P., Jordan, A. S., White, D. P., and Malhotra, A. (2011). Sensorimotor function of the upper-airway muscles and respiratory sensory processing in untreated obstructive sleep apnea. J. Appl. Physiol. 111, 1644–1653. doi: 10.1152/japplphysiol.00653.2011
Fox, M. D., and Raichle, M. E. (2007). Spontaneous fluctuations in brain activity observed with functional magnetic resonance imaging. Nat. Rev. Neurosci. 8, 700–711. doi: 10.1038/nrn2201
Friston, K. J., Williams, S., Howard, R., Frackowiak, R. S., and Turner, R. (1996). Movement-related effects in fMRI time-series. Magn. Reson. Med. 35, 346–355. doi: 10.1002/mrm.1910350312
Gao, F., Guo, Y., Chu, H., Yu, W., Chen, Z., Chen, L., et al. (2020). Lower-limb sensorimotor deprivation-related brain activation in patients with chronic complete spinal cord injury. Front. Neurol. 11:555733. doi: 10.3389/fneur.2020.555733
Ghaziri, J., Tucholka, A., Girard, G., Houde, J. C., Boucher, O., Gilbert, G., et al. (2017). The corticocortical structural connectivity of the human insula. Cereb. Cortex 27, 1216–1228. doi: 10.1093/cercor/bhv308
Goossens, N., Rummens, S., Janssens, L., Caeyenberghs, K., and Brumagne, S. (2018). Association between sensorimotor impairments and functional brain changes in patients with low back pain: a critical review. Am. J. Phys. Med. Rehabil. 97, 200–211. doi: 10.1097/PHM.0000000000000859
Grupe, D. W., and Nitschke, J. B. (2013). Uncertainty and anticipation in anxiety: an integrated neurobiological and psychological perspective. Nat. Rev. Neurosci. 14, 488–501. doi: 10.1038/nrn3524
Guo, M., Ren, Y., Yu, H., Yang, H., Cao, C., Li, Y., et al. (2020). Alterations in degree centrality and functional connectivity in Parkinson’s disease patients with freezing of gait: a resting-state functional magnetic resonance imaging study. Front. Neurosci. 14:582079. doi: 10.3389/fnins.2020.582079
Hampson, M., Peterson, B. S., Skudlarski, P., Gatenby, J. C., and Gore, J. C. (2002). Detection of functional connectivity using temporal correlations in MR images. Hum. Brain Mapp. 15, 247–262. doi: 10.1002/hbm.10022
Japee, S., Holiday, K., Satyshur, M. D., Mukai, I., and Ungerleider, L. G. (2015). A role of right middle frontal gyrus in reorienting of attention: a case study. Front. Syst. Neurosci. 9:23. doi: 10.3389/fnsys.2015.00023
Johns, M. W. (1991). A new method for measuring daytime sleepiness: the Epworth sleepiness scale. Sleep 14, 540–545. doi: 10.1093/sleep/14.6.540
Jordan, A. S., McSharry, D. G., and Malhotra, A. (2014). Adult obstructive sleep apnoea. Lancet 383, 736–747. doi: 10.1016/s0140-6736(13)60734-5
Kang, D., Qin, Z., Wang, W., Zheng, Y., Hu, H., Bao, Y., et al. (2020). Brain functional changes in Tibetan with obstructive sleep apnea hypopnea syndrome: a resting state fMRI study. Medicine 99:e18957. doi: 10.1097/MD.0000000000018957
Kapur, V. K., Auckley, D. H., Chowdhuri, S., Kuhlmann, D. C., Mehra, R., Ramar, K., et al. (2017). Clinical practice guideline for diagnostic testing for adult obstructive sleep apnea: an American Academy of Sleep Medicine clinical practice guideline. J. Clin. Sleep Med. 13, 479–504. doi: 10.5664/jcsm.6506
Kim, J. Y., Ko, I., and Kim, D. K. (2019). Association of obstructive sleep apnea with the risk of affective disorders. JAMA Otolaryngol. Head Neck Surg. 145, 1020–1026. doi: 10.1001/jamaoto.2019.2435
Kumar, R., Chavez, A. S., Macey, P. M., Woo, M. A., Yan-Go, F. L., and Harper, R. M. (2012). Altered global and regional brain mean diffusivity in patients with obstructive sleep apnea. J. Neurosci. Res. 90, 2043–2052. doi: 10.1002/jnr.23083
Kumar, R., Macey, P. M., Cross, R. L., Woo, M. A., Yan-Go, F. L., and Harper, R. M. (2009). Neural alterations associated with anxiety symptoms in obstructive sleep apnea syndrome. Depress Anxiety 26, 480–491. doi: 10.1002/da.20531
Kumar, S., Anton, A., and D’Ambrosio, C. M. (2021). Sex differences in obstructive sleep apnea. Clin. Chest Med. 42, 417–425. doi: 10.1016/j.ccm.2021.04.004
Kurth, F., Zilles, K., Fox, P. T., Laird, A. R., and Eickhoff, S. B. (2010). A link between the systems: functional differentiation and integration within the human insula revealed by meta-analysis. Brain Struct. Funct. 214, 519–534. doi: 10.1007/s00429-010-0255-z
Lange, I., Kasanova, Z., Goossens, L., Leibold, N., De Zeeuw, C. I., van Amelsvoort, T., et al. (2015). The anatomy of fear learning in the cerebellum: a systematic meta-analysis. Neurosci. Biobehav. Rev. 59, 83–91. doi: 10.1016/j.neubiorev.2015.09.019
Lee, S.-A., Kim, S. J., Lee, S. Y., and Kim, H. J. (2021). Periodic limb movements during sleep are associated with poor health-related quality of life in patients with obstructive sleep apnea. Sleep Breath. doi: 10.1007/s11325-021-02469-y
Lei, X., Zhong, M., Zhang, B., Yang, H., Peng, W., Liu, Q., et al. (2019). Structural and functional connectivity of the anterior cingulate cortex in patients with borderline personality disorder. Front. Neurosci. 13:971. doi: 10.3389/fnins.2019.00971
Li, H. J., Nie, X., Gong, H. H., Zhang, W., Nie, S., and Peng, D. C. (2016). Abnormal resting-state functional connectivity within the default mode network subregions in male patients with obstructive sleep apnea. Neuropsychiatr. Dis. Treat. 12, 203–212. doi: 10.2147/NDT.S97449
Li, J., Li, M. X., Liu, S. N., Wang, J. H., Huang, M., Wang, M., et al. (2014). Is brain damage really involved in the pathogenesis of obstructive sleep apnea? Neuroreport 25, 593–595. doi: 10.1097/WNR.0000000000000143
Li, M. X., Yan, C. Y., and Wang, S. (2015). New insights on the role of the insular cortex and habenula in OSA. Sleep Breath. 19, 1347–1353. doi: 10.1007/s11325-015-1168-0
Li, W., Qin, W., Liu, H., Fan, L., Wang, J., Jiang, T., et al. (2013). Subregions of the human superior frontal gyrus and their connections. Neuroimage 78, 46–58. doi: 10.1016/j.neuroimage.2013.04.011
Li, X.-B., Wang, L.-B., Xiong, Y.-B., Bo, Q.-J., He, F., Li, F., et al. (2019). Altered resting-state functional connectivity of the insula in individuals with clinical high-risk and patients with first-episode schizophrenia. Psychiatry Res. 282:112608. doi: 10.1016/j.psychres.2019.112608
Macey, P. M., Kumar, R., Woo, M. A., Valladares, E. M., Yan-Go, F. L., and Harper, R. M. (2008). Brain structural changes in obstructive sleep apnea. Sleep 31, 967–977.
Moreno-Rius, J. (2018). The cerebellum in fear and anxiety-related disorders. Prog. Neuropsychopharmacol. Biol. Psychiatry 85, 23–32. doi: 10.1016/j.pnpbp.2018.04.002
Nakata, H., Domoto, R., Mizuguchi, N., Sakamoto, K., and Kanosue, K. (2019). Negative BOLD responses during hand and foot movements: an fMRI study. PLoS One 14:e0215736. doi: 10.1371/journal.pone.0215736
Namkung, H., Kim, S. H., and Sawa, A. (2017). The insula: an underestimated brain area in clinical neuroscience, psychiatry, and neurology. Trends Neurosci. 40, 200–207. doi: 10.1016/j.tins.2017.02.002
Nieuwenhuys, R. (2012). The insular cortex: a review. Prog. Brain Res. 195, 123–163. doi: 10.1016/B978-0-444-53860-4.00007-6
Park, B., Palomares, J. A., Woo, M. A., Kang, D. W., Macey, P. M., Yan-Go, F. L., et al. (2016a). Aberrant insular functional network integrity in patients with obstructive sleep apnea. Sleep 39, 989–1000. doi: 10.5665/sleep.5738
Park, B., Palomares, J. A., Woo, M. A., Kang, D. W., Macey, P. M., Yan-Go, F. L., et al. (2016b). Disrupted functional brain network organization in patients with obstructive sleep apnea. Brain Behav. 6:e00441. doi: 10.1002/brb3.441
Park, H. R., Cha, J., Joo, E. Y., and Kim, H. (2021). Altered cerebrocerebellar functional connectivity in patients with obstructive sleep apnea and its association with cognitive function. Sleep zsab209. doi: 10.1093/sleep/zsab209
Peng, D. C., Dai, X. J., Gong, H. H., Li, H. J., Nie, X., and Zhang, W. (2014). Altered intrinsic regional brain activity in male patients with severe obstructive sleep apnea: a resting-state functional magnetic resonance imaging study. Neuropsychiatr. Dis. Treat. 10, 1819–1826. doi: 10.2147/NDT.S67805
Pico-Perez, M., Radua, J., Steward, T., Menchon, J. M., and Soriano-Mas, C. (2017). Emotion regulation in mood and anxiety disorders: a meta-analysis of fMRI cognitive reappraisal studies. Prog. Neuropsychopharmacol. Biol. Psychiatry 79, 96–104. doi: 10.1016/j.pnpbp.2017.06.001
Pirzada, S., Uddin, M. N., Figley, T. D., Kornelsen, J., Puig, J., Marrie, R. A., et al. (2020). Spatial normalization of multiple sclerosis brain MRI data depends on analysis method and software package. Magn. Reson. Imaging 68, 83–94. doi: 10.1016/j.mri.2020.01.016
Power, J. D., Barnes, K. A., Snyder, A. Z., Schlaggar, B. L., and Petersen, S. E. (2012). Spurious but systematic correlations in functional connectivity MRI networks arise from subject motion. Neuroimage 59, 2142–2154. doi: 10.1016/j.neuroimage.2011.10.018
Ramnani, N. (2012). Frontal lobe and posterior parietal contributions to the cortico-cerebellar system. Cerebellum 11, 366–383. doi: 10.1007/s12311-011-0272-3
Rinat, S., Izadi-Najafabadi, S., and Zwicker, J. G. (2020). Children with developmental coordination disorder show altered functional connectivity compared to peers. Neuroimage Clin. 27:102309. doi: 10.1016/j.nicl.2020.102309
Rosenzweig, I., Williams, S. C., and Morrell, M. J. (2014). The impact of sleep and hypoxia on the brain: potential mechanisms for the effects of obstructive sleep apnea. Curr. Opin. Pulm. Med. 20, 565–571. doi: 10.1097/MCP.0000000000000099
Saad, Z. S., Gotts, S. J., Murphy, K., Chen, G., Jo, H. J., Martin, A., et al. (2012). Trouble at rest: how correlation patterns and group differences become distorted after global signal regression. Brain Connect. 2, 25–32. doi: 10.1089/brain.2012.0080
Satterthwaite, T. D., Elliott, M. A., Gerraty, R. T., Ruparel, K., Loughead, J., Calkins, M. E., et al. (2013). An improved framework for confound regression and filtering for control of motion artifact in the preprocessing of resting-state functional connectivity data. Neuroimage 64, 240–256. doi: 10.1016/j.neuroimage.2012.08.052
Schon, D., Rosenkranz, M., Regelsberger, J., Dahme, B., Buchel, C., and von Leupoldt, A. (2008). Reduced perception of dyspnea and pain after right insular cortex lesions. Am. J. Respir. Crit. Care Med. 178, 1173–1179. doi: 10.1164/rccm.200805-731OC
Shen, Y. T., Yuan, Y. S., Wang, M., Zhi, Y., Wang, J. W., Wang, L. N., et al. (2020). Dysfunction in superior frontal gyrus associated with diphasic dyskinesia in Parkinson’s disease. NPJ Parkinsons Dis. 6:30. doi: 10.1038/s41531-020-00133-y
Shi, Y., Chen, L., Chen, T., Li, L., Dai, J., Lui, S., et al. (2017). A meta-analysis of voxel-based brain morphometry studies in obstructive sleep apnea. Sci. Rep. 7:10095. doi: 10.1038/s41598-017-09319-6
Song, X., Roy, B., Kang, D. W., Aysola, R. S., Macey, P. M., Woo, M. A., et al. (2018). Altered resting-state hippocampal and caudate functional networks in patients with obstructive sleep apnea. Brain Behav. 8:e00994. doi: 10.1002/brb3.994
Song, X. W., Dong, Z. Y., Long, X. Y., Li, S. F., Zuo, X. N., Zhu, C. Z., et al. (2011). REST: a toolkit for resting-state functional magnetic resonance imaging data processing. PLoS One 6:e25031. doi: 10.1371/journal.pone.0025031
Sridharan, D., Levitin, D. J., and Menon, V. (2008). A critical role for the right fronto-insular cortex in switching between central-executive and default-mode networks. Proc. Natl. Acad. Sci. U.S.A. 105, 12569–12574. doi: 10.1073/pnas.0800005105
Tahmasian, M., Rosenzweig, I., Eickhoff, S. B., Sepehry, A. A., Laird, A. R., Fox, P. T., et al. (2016). Structural and functional neural adaptations in obstructive sleep apnea: an activation likelihood estimation meta-analysis. Neurosci. Biobehav. Rev. 65, 142–156. doi: 10.1016/j.neubiorev.2016.03.026
Touroutoglou, A., Bliss-Moreau, E., Zhang, J., Mantini, D., Vanduffel, W., Dickerson, B. C., et al. (2016). A ventral salience network in the macaque brain. Neuroimage 132, 190–197. doi: 10.1016/j.neuroimage.2016.02.029
Touroutoglou, A., Hollenbeck, M., Dickerson, B. C., and Feldman Barrett, L. (2012). Dissociable large-scale networks anchored in the right anterior insula subserve affective experience and attention. Neuroimage 60, 1947–1958. doi: 10.1016/j.neuroimage.2012.02.012
Uddin, L. Q., Kinnison, J., Pessoa, L., and Anderson, M. L. (2014). Beyond the tripartite cognition-emotion-interoception model of the human insular cortex. J. Cogn. Neurosci. 26, 16–27. doi: 10.1162/jocn_a_00462
Van Essen, D. C., Glasser, M. F., Dierker, D. L., Harwell, J., and Coalson, T. (2012). Parcellations and hemispheric asymmetries of human cerebral cortex analyzed on surface-based atlases. Cereb. Cortex 22, 2241–2262. doi: 10.1093/cercor/bhr291
Vanek, J., Prasko, J., Genzor, S., Ociskova, M., Kantor, K., Holubova, M., et al. (2020). Obstructive sleep apnea, depression and cognitive impairment. Sleep Med. 72, 50–58. doi: 10.1016/j.sleep.2020.03.017
Vicentini, J. E., Weiler, M., Almeida, S. R. M., de Campos, B. M., Valler, L., and Li, L. M. (2017). Depression and anxiety symptoms are associated to disruption of default mode network in subacute ischemic stroke. Brain Imaging Behav. 11, 1571–1580. doi: 10.1007/s11682-016-9605-7
Viñas-Guasch, N., and Wu, Y. J. (2017). The role of the putamen in language: a meta-analytic connectivity modeling study. Brain Struct. Funct. 222, 3991–4004. doi: 10.1007/s00429-017-1450-y
von Leupoldt, A., and Dahme, B. (2005). Cortical substrates for the perception of dyspnea. Chest 128, 345–354. doi: 10.1378/chest.128.1.345
Wang, J., Wang, M., Wei, Z., Li, M., Huang, M., and Wang, S. (2014). The lateral habenular nucleus mediates signal transduction from the insular cortex in OSA rats. Sleep Breath. 18, 491–497. doi: 10.1007/s11325-013-0910-8
Xifra-Porxas, A., Kassinopoulos, M., and Mitsis, G. D. (2021). Physiological and motion signatures in static and time-varying functional connectivity and their subject identifiability. Elife 10:e62324. doi: 10.7554/eLife.62324
Yan, C. G., Wang, X. D., Zuo, X. N., and Zang, Y. F. (2016). DPABI: data processing & analysis for (Resting-State) brain imaging. Neuroinformatics 14, 339–351. doi: 10.1007/s12021-016-9299-4
Yu, H., Chen, L., Li, H., Xin, H., Zhang, J., Wei, Z., et al. (2019). Abnormal resting-state functional connectivity of amygdala subregions in patients with obstructive sleep apnea. Neuropsychiatr. Dis. Treat. 15, 977–987. doi: 10.2147/NDT.S191441
Zang, Y., Jiang, T., Lu, Y., He, Y., and Tian, L. (2004). Regional homogeneity approach to fMRI data analysis. Neuroimage 22, 394–400. doi: 10.1016/j.neuroimage.2003.12.030
Zhang, Q., Qin, W., He, X., Li, Q., Chen, B., Zhang, Y., et al. (2015). Functional disconnection of the right anterior insula in obstructive sleep apnea. Sleep Med. 16, 1062–1070. doi: 10.1016/j.sleep.2015.04.018
Zhang, Q., Wang, D., Qin, W., Li, Q., Chen, B., Zhang, Y., et al. (2013). Altered resting-state brain activity in obstructive sleep apnea. Sleep 36, 651B–659B. doi: 10.5665/sleep.2620
Keywords: insula, functional connectivity, brain network, cognitive impairment, magnetic resonance imaging
Citation: Kong L, Li H, Shu Y, Liu X, Li P, Li K, Xie W, Zeng Y and Peng D (2022) Aberrant Resting-State Functional Brain Connectivity of Insular Subregions in Obstructive Sleep Apnea. Front. Neurosci. 15:765775. doi: 10.3389/fnins.2021.765775
Received: 27 August 2021; Accepted: 13 December 2021;
Published: 20 January 2022.
Edited by:
Julio Fernandez-Mendoza, Penn State Health Milton S. Hershey Medical Center, United StatesReviewed by:
Peng Li, Harvard Medical School, United StatesCopyright © 2022 Kong, Li, Shu, Liu, Li, Li, Xie, Zeng and Peng. This is an open-access article distributed under the terms of the Creative Commons Attribution License (CC BY). The use, distribution or reproduction in other forums is permitted, provided the original author(s) and the copyright owner(s) are credited and that the original publication in this journal is cited, in accordance with accepted academic practice. No use, distribution or reproduction is permitted which does not comply with these terms.
*Correspondence: Dechang Peng, cGVuZ2RjZG9jdG9yQDE2My5jb20=
Disclaimer: All claims expressed in this article are solely those of the authors and do not necessarily represent those of their affiliated organizations, or those of the publisher, the editors and the reviewers. Any product that may be evaluated in this article or claim that may be made by its manufacturer is not guaranteed or endorsed by the publisher.
Research integrity at Frontiers
Learn more about the work of our research integrity team to safeguard the quality of each article we publish.