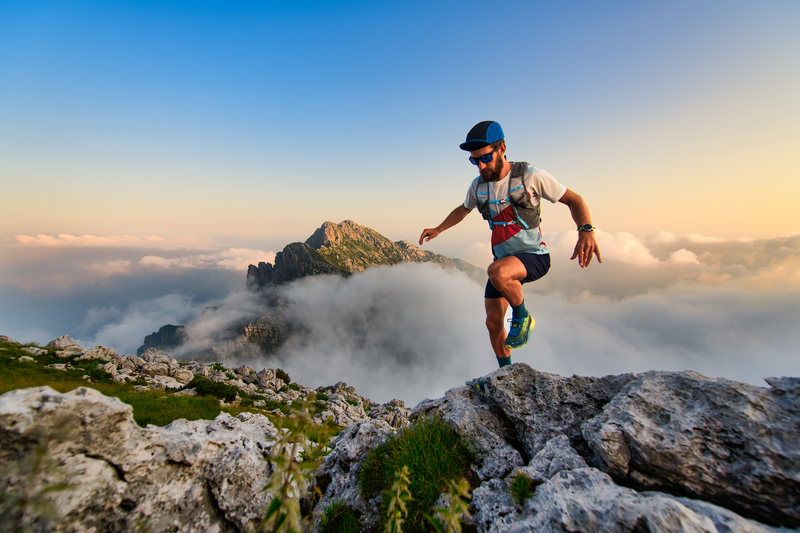
95% of researchers rate our articles as excellent or good
Learn more about the work of our research integrity team to safeguard the quality of each article we publish.
Find out more
MINI REVIEW article
Front. Neurosci. , 12 November 2021
Sec. Sleep and Circadian Rhythms
Volume 15 - 2021 | https://doi.org/10.3389/fnins.2021.755464
This article is part of the Research Topic Unraveling sleep and its disorders using novel analytical approaches View all 12 articles
The interactions of heart rate variability and respiratory rate and tidal volume fluctuations provide key information about normal and abnormal sleep. A set of metrics can be computed by analysis of coupling and coherence of these signals, cardiopulmonary coupling (CPC). There are several forms of CPC, which may provide information about normal sleep physiology, and pathological sleep states ranging from insomnia to sleep apnea and hypertension. As CPC may be computed from reduced or limited signals such as the electrocardiogram or photoplethysmogram (PPG) vs. full polysomnography, wide application including in wearable and non-contact devices is possible. When computed from PPG, which may be acquired from oximetry alone, an automated apnea hypopnea index derived from CPC-oximetry can be calculated. Sleep profiling using CPC demonstrates the impact of stable and unstable sleep on insomnia (exaggerated variability), hypertension (unstable sleep as risk factor), improved glucose handling (associated with stable sleep), drug effects (benzodiazepines increase sleep stability), sleep apnea phenotypes (obstructive vs. central sleep apnea), sleep fragmentations due to psychiatric disorders (increased unstable sleep in depression).
The prevalence of sleep disorders has been increasing over the last two decades (Acquavella et al., 2020). Disorders like insomnia and sleep apnea have a prevalence of as much as 20% in the general population (Franklin and Lindberg, 2015; Acquavella et al., 2020). There is a need for nimble sleep state estimation, diagnostics, and tracking. One approach seeing increasing utilization both in formal medical and consumer wearable devices is through analysis of heart rate and respiration. There is a strong correlation between changes in heart rate variability and sleep during health and disease (Tobaldini et al., 2013). High frequency (HF) components mainly present parasympathetic activity, while low frequency (LF) components is partly a quantitative marker of sympathetic modulation. LF and the LF/HF ratio are high in Wake and decrease in NREM sleep, peaking once more during REM sleep, while HF follows the opposite trend. Deep NREM sleep (N3) typically has the greatest HF power. Sleep disruptive influences such as sleep apnea (Qin et al., 2021; Ucak et al., 2021), insomnia (Spiegelhalder et al., 2011; Dodds et al., 2017; Cosgrave et al., 2021), and depression (Hyunbin et al., 2017; Gao et al., 2019; Eddie et al., 2020) are associated with an increase in the LF components.
Different techniques have been used to assess for such changes one of which is analysis of cardiopulmonary coupling and coherence (CPC) patterns. In this technique a single lead electrocardiogram (ECG) or photo plethysmogram (PPG) is used to extract heart rate variability and ECG or PPG signal derived respiration (EDR/PDR) (Thomas et al., 2005; Hilmisson et al., 2020). Contrary to the stage/grade approach to conventional sleep characterization, CPC analysis based on coupling and coherence provides a novel and complementary view of sleep, that of bistability, which are particularly well defined during NREM sleep. Thus, distinct patterns of CPC are observed: high frequency coupling (HFC) which is associated with stable NREM sleep and low frequency coupling (LFC) which is associated with unstable and often fragmented NREM sleep (Thomas et al., 2005). A third CPC pattern named very low frequency coupling occur in both REM sleep and wake, which may be differentiated by analysis of signal quality and motion artifact (Al Ashry et al., 2021). High and low frequency coupling are mutually exclusive and shift logically with disease states and treatments. For example, there is an increase LFC in patients with insomnia and this can be tracked in the ambulatory setting (Thomas et al., 2017a,b). There is increased LFC during sleep in unmedicated patients with major depression and improvement with therapy of major depression (Yang et al., 2011). Integrating CPC with oximetry allows generating a true FDA approved apnea-hypopnea index (AHI), which shows good correlation with conventional polysomnogram-derived AHI (Hilmisson et al., 2020; Al Ashry et al., 2021).
The overall goal of this article is to review physiological basis, techniques, and applications of CPC spectrograms in sleep in health and disease. There are three aims for this review, to show that—(a) CPC shows a fundamental characteristic of NREM sleep—bimodality, across a number of physiologies; (b) CPC has several uses in sleep apnea care—diagnosis, phenotype, tracking outcomes; (c) CPC can diagnose and track non-apneic sleep fragmentation and medication effects, and should be used in the appropriate clinical context.
Entrainment between heart rate and respiration in humans has been described since the early twentieth century (Galletly and Larsen, 1998). It has been suggested that such synchrony between heart rate and respiration improves pulmonary gas exchange and computational models have shown that healthy cardiopulmonary coupling minimizes the heart workload while maintaining adequate ventilation (Yasuma and Hayano, 2004; Ben-Tal et al., 2012). Such strong cardiopulmonary coupling is seen at its best during deep sleep, sedation, and anesthesia (Dick et al., 2014). There is a critical influence of the autonomic nervous system on cardiopulmonary coupling (Bartsch et al., 2012). Non-rapid eye movement (NREM) sleep is associated with decreased sympathetic activity, a decrease in heart rate, and a decrease of average blood pressure and blood pressure variability in comparison to the wake state (Somers et al., 1993). Respiratory sinus arrythmia is a phenomenon in which the heart rate variability is synchronized beat-to-beat with respiration and is most pronounced during deep NREM sleep (Zemaityte et al., 1984; Yasuma and Hayano, 2004). In contrast, during rapid eye movement (REM) sleep there is dominance of sympathetic control and a burst frequency of sympathetic activity that is actually higher than during wakefulness leading to increases in blood pressure variability and heart rates similar to what is seen during wakefulness (Somers et al., 1993). Using spectral analysis, a frequency of 0.1 Hz and above has been associated with parasympathetic activity dominance and frequencies below 0.1 Hz have been associated with dominance of sympathetic activity (Appel et al., 1989; Cui et al., 2020).
Cardiopulmonary sleep spectrograms were first obtained from a single lead ECG (Thomas et al., 2005, 2007). ECG-derived respiration (EDR) is obtained either by using R-S wave amplitudes or variations in QRS complexes area (Zheng et al., 2016). Several studies have looked at improving the accuracy of deducing EDR from single lead ECG and reducing noise but is beyond the scope of this paper (Thayer et al., 1996; Leanderson et al., 2003; Liu et al., 2012; Zheng et al., 2016). In parallel to extracting the EDR, ectopic beats are identified and removed and normal sinus—normal sinus (NN) intervals are extracted and outliers are filtered (Thomas et al., 2005). After extracting the N-N interval series on ECG and its associated EDR, the signals are then resampled using cubic splines at 2 Hz. The Fast Fourier Transform is applied to 3 overlapping 512 sample sub-windows within the 1,024 coherence window. The 1,024 coherence window is then advanced by 256 samples (2.1 min) and the calculation repeated until the entire N-N interval/EDR series is analyzed. Thus, the cross-spectral power and coherence of these two signals are calculated over a 1,024 sample (8.5 min) window.
For each 1,024 window the product of the coherence and cross-spectral power is used to calculate the ratio of coherent cross power in the low frequency (0.01–0.1 Hz.) band to that in the high frequency (0.1–0.4 Hz.) band. The logarithm of the high to low frequency cardiopulmonary coupling ratio [log (HFC/LFC)] is then computed to yield a continuously varying measure of cardiopulmonary coupling (Al Ashry et al., 2021). While originally the ECG signal was used as input, any signal or signal set which encodes respiration and heart rate variability may be used to compute the CPC sleep spectrogram. Figure 1 shows the steps in computing CPC.
Figure 1. Schematic for CPC analysis. ECG, Electrocardiogram; PPG, Photoplethysmogram; EDR, ECG-derived respiration; PDR, Photoplethysmogram derived respiration R-S and QRS are ECG waveforms; N-N intervals, Normal sinus to normal sinus intervals; Hz, frequency; HFC, high frequency coupling; LFC, low frequency coupling.
The conventional characterization of sleep stages dictates a “graded” approach to NREM sleep, from lightest (N1) to deepest (N3). The difference between N2 and N3 are relatively arbitrary, dependent on the proportion of high amplitude slow waves (20% threshold) for a given epoch. However, there is a great variability of depth of NREM sleep, as can be readily objectively demonstrated by techniques such as the Odds Ratio Product (Younes et al., 2015). A unique and key feature of CPC sleep states is poor correlation of HFC and LFC with conventional NREM sleep stages. Thus, in health, the majority if N2 is also HFC, N3 is usually HFC but at times LFC, while N1 is always LFC. There is a moderate correlation with a well-described stability dimension of NREM sleep, Cyclic Alternating Pattern (CAP) (Thomas et al., 2005). CAP is a distinct pattern that can be seen on electroencephalography (EEG) during unstable NREM sleep. High frequency coupling dominates when CAP is sparse or absent, while LFC is reliably associated with CAP. Conventional NREM stage N3 is usually HFC, but so is the majority of healthy N2, where non-CAP periods also dominate. Thus, CAP and CPC capture significantly overlapping domains of NREM sleep stability while both measures correlate only partially with conventional measures of sleep depth.
Slow-wave power in the sleep EEG has highly characteristic spatial and temporal evolution patterns across a night. Power in the 1–4 Hz frequencies dominates the first half of the night, but the ebb and flow of slow-wave power continues throughout the night. High frequency coupling strongly covaries with slow-wave power across the whole night, while low frequency power in heart rate variability is inversely related to EEG delta power (Brandenberger et al., 2001; Ako et al., 2003; Thomas et al., 2014). One interesting finding when aligning HFC with delta power is that there is a consistent lag of delta power after HFC where HFC usually precedes an increase in delta power by an average of 6 min, suggesting that subcortical/brain-stem mechanisms may lead large-scale cortical synchrony during sleep (Thomas et al., 2014).
A key dimension of health is a reduction of blood pressure during sleep (blood pressure “dipping”). Sleep blood pressure is known to dip during stage N3, and rise during REM sleep, yet the majority of sleep is N2, and dipping profiles occupy the entire sleep period, not just N3-enriched zones. This discrepancy has been solved by correlating blood pressure during sleep with stable sleep as determined by CPC (high frequency coupling). Blood pressure dipping occurs only during HFC periods (Wood et al., 2020). In a randomized trial targeting sleep apnea treatment in patients with cardiovascular risk factors, it was shown that those who were treated with CPAP had more HFC during sleep, which was in turn associated with improvement in blood pressure dipping and mean arterial pressures during sleep (Magnusdottir and Hilmisson, 2018).
There is normally a reduction of the heart rate (HR) during sleep, a HR-dip, which roughly follows the blood pressure dipping pattern. Heart rate during sleep is, however, far simpler to measure than blood pressure and provides a window into cardiovascular health. By aligning heart rate profiles with the CPC spectrogram (Figure 2), unique insights and cardiovascular risk profiles are potentially extractable, and can be tracked over time. Normally, HR dipping occurs during HFC periods.
Figure 2. Photoplethysmogram/oximetry-based CPC-heart rate analysis. The oximetry-based analysis provides a full CPC-sleep spectrogram, an apnea-hypopnea index by integrating CPC LFC and oxygen desaturation events, and a profile of heart rate across the night. In the upper segment of the figure, “dipping” of heart rate is noted along with abundant high frequency coupling/stable sleep. In the lower sample, there is less stable state, but the heart rate profile is distinctly abnormal, with an elevation even during stable state. Such relative tachycardia during stable NREM sleep may suggest obstructive hypoventilation. In both examples, oxygen desaturation itself is mild. Stable and unstable sleep (HFC and LFC, respectively) occur intermittently through the night. HFC, high frequency coupling; LFC, low frequency coupling; e-LFC, elevated low frequency coupling; HR, heart rate; CHVR, cyclic heart rate variation.
Cardiopulmonary coupling analysis established that sleep is bimodal than graded. That is, while conventional NREM sleep stages moves across the N1 to N3 grades, the CPC-spectrogram shows that NREM sleep has only two distinct and completely non-overlapping forms—stable and unstable (HFC and LFC, respectively), which intermittently switch across the entire night. While N3 dominates in the first half of the night, HFC occurs throughout (Figure 3). This bimodality or stability domain is especially clear when incorporating autonomic and respiratory variables with electrocortical activity, specifically, delta power and the < 1 Hz slow oscillation. Stable NREM is characterized by high probability of occurrence of the < 1 Hz slow oscillation, high delta power, non-CAP EEG, stable breathing, blood pressure dipping, strong sinus arrhythmia and vagal dominance, and high frequency CPC. Conversely, unstable NREM exhibits opposite features: a fragmented and discontinuous < 1 Hz slow oscillation, CAP patterns on the EEG, non-dipping of blood pressure, unstable respiration, cyclic variation in heart rate, and low frequency CPC (Wood et al., 2020).
Figure 3. The oximeter-extracted CPC spectrogram. The basic graphical representation of the CPC-spectrogram has high, low, and very low frequency coupling (HFC, LFC, and VLFC, respectively) components. Actigraphy is integrated, and VLFC without movement is considered REM sleep, whereas VLFC with movement is Wake. Cyclic variation of heart rate is also displayed, as well as e-LFC as a measure of sleep fragmentation. The oximeter signal itself provides standard oximetry metrics, such as an oxygen desaturation index. As shows, periods of HFC and LFC alternate throughout the night. LFC, low frequency coupling; VLFC, very low frequency coupling; HFC, high frequency coupling; CHVR, cyclic heart rate variation.
Sleep apnea reliably induces strongly coupled low-frequency oscillations in heart rate and respiration. This results in strong ECG or PPG amplitude fluctuations, besides cyclic variation in heart rate, enabling computing an AHI. This computation requires knowing the number of oxygen desaturation events, the amount of time in coupled low-frequency oscillations, the mean frequency of these computed oscillations, and the total sleep period.
The first step in using CPC for sleep apnea detection involves a second-levels analysis of the LFC zone, where a spectral band designated as elevated-LFC (e-LFC) was found which correlated highly with scored apneas and hypopneas. Within e-LFC, two further patterns were discernable, one with a wide dispersion of coupling spectra and another with a narrow band of coupling spectra (broad and narrow-band e-LFC, or e-LFCBB and e-LFCNB). However, other causes of sleep fragmentation may also cause similar patterns, especially e-LFCBB, a limitation which may be minimized by integrating oxygen saturation fluctuations into the computation. In two recent large studies combining CPC AHI with oximetry desaturation index events in one index have improved the accuracy of derived AHI in comparison to PSG AHI (Hilmisson et al., 2020; Al Ashry et al., 2021). This derived AHI was approved to be equivalent to PSG AHI in adults and children in 2019 by the FDA (K182618).
These LFCNB and LFCBB indices have been used in several studies in the adult and pediatric populations for automated detection of sleep apnea (Table 1, section A). There are several advantages for using CPC through wearable devices, especially the current embodiment of a ring-form oximeter, in the sleep apnea population. These include: (1) cost-effective screening of high risk adult and pediatric populations; (2) minimizing patient (wearing) and system (scoring) burdens; (3) detection of expressed high loop gain (central apnea and periodic breathing), which can be a risk stratification approach, as such patients are at risk for treatment-emergent central sleep apnea, reduced adherence to therapy, and persistent respiratory instability during apnea therapy.
Table 1. Studies that used cardiopulmonary coupling to diagnose and follow treatment response in various sleep disorders.
Successful sleep apnea treatment is expected to increase HFC relative to LFC, including following oral appliance therapy and upper airway surgery (Schramm and Thomas, 2012; Lee et al., 2014, 2016; Choi et al., 2015). A similar pattern is noted in pediatric patients with OSA after adenotonsillectomy (Lee et al., 2012; Chen and He, 2019). The same results are seen with CPAP treatment of OSA (Harrington et al., 2013; Cho and Kim, 2017). Successful treatment of OSA with CPAP is associated with improvement in HFC/LFC ratio (Roche et al., 1999; Shiina et al., 2010). Gilman et al. (2008) randomized patients with heart failure (ejection fraction less than 45%) who had moderate to severe OSA to CPAP vs. usual care. After 1 month the CPAP treated group showed an increase in HFC compared to the control group (Gilman et al., 2008). Harrington et al. (2013) looked at CPAP titration studies and defined successful CPAP titration and optimum CPAP pressures as AHI less ≤ 5 / h of sleep for 30 min during supine REM; higher HFC was found in successful CPAP PSGs and higher LFC in unsuccessful titrations (Harrington et al., 2013).
Endotypes are the mechanisms which drive pathology, while phenotypes are the expression of these endotypic effects. Multiple driver endotypes are now recognized as important in the pathogenesis of obstructive sleep apnea, including high loop gain, low arousal threshold, airway collapsibility, impaired negative pressure response, and sleep fragmentation resulting in amplified wake-sleep transitional instability (Dutta et al., 2021; Finnsson et al., 2021). Thus, what is considered “obstructive sleep apnea” can be caused by one or more of the above driving mechanisms, which can be classified into anatomical and non-anatomical. High loop gain, reflecting respiratory control instability and an imbalance between input (oxygen and carbon dioxide levels) and output (neural drive to respiratory muscles and upper airway) of the respiratory system, is perhaps the most important non-anatomical endotype. When loop gain is more than 1, self-sustained oscillations are inevitable. The importance of high loop gain is that treatment failure risk is high and options such as oxygen (Edwards et al., 2016; Sands et al., 2018) and acetazolamide (Edwards et al., 2012) can be beneficial. Though mathematical methods can accurately quantify endotypes, analysis of the expressed phenotypes can also accurately identify sleep apnea with high loop gain.
When high loop gain is manifested, the polysomnographic patterns include classic central sleep apnea, periodic breathing, complex apnea with codominant loop gain and airway pathology, treatment-emergent central sleep apnea, and NREM-dominant obstructive sleep apnea. A common theme across all these conditions is self-similar (metronomic timing, identical morphology of consecutive events) of respiratory abnormality (Oppersma et al., 2021), which induce e-LFCNB, which is a marker of this expressed high loop gain (Figure 4; Thomas et al., 2007). In a study of 671 subjects with sleep apnea which compared CPC indices to conventional PSG scoring (Thomas et al., 2007), e-LFCNB was associated with respiratory instability during CPAP titration. Since e-LFCNB is a marker of expressed high loop gain and “central” sleep apnea, Ramar et al. (2013) evaluated if could be used as a marker of adaptive servo-ventilation titration success in 106 patients with complex sleep apnea. Overall ASV titration success as defined as AHI < 10/h on ASV was found in 81% of patients and no correlation was found between percentage of LFCNB and ASV titration success (Ramar et al., 2013). One limitation of this study was the use of opiates, which causes ataxic breathing, and is unlikely to cause the exact self-similarity needed to induce e-LFCNB. Table 1 (section B) summarizes the studies that used CPC in phenotyping and following response of treatment of sleep apnea.
Figure 4. Sleep apnea phenotyping. 3-Dimensional graphical view of the CPC- spectrogram in a patient with severe sleep apnea and no stable (HFC) sleep. Color code: orange = VLFC, blue = e-LFCBB (broadband coupling), and red = e-LFCNB (narrowband coupling). The offset view (right) shows the narrow dispersion of coupling frequencies induced by periodic breathing toward the end of the recording period, while earlier in the night the e-LFC spectra are “broadly dispersed,” consistent with predominantly obstructive sleep apnea. On the figure to the right the time axis is cut off and the figure is off set to show the narrow band best.
CPC has been used to study other sleep disorders beyond OSA. Patients with insomnia have been shown to exhibit increased LFC even in the absence of sleep disordered breathing (Thomas et al., 2018). It appears that LFCBB is the main LFC pattern seen in pure insomnia so the coexistence of LFCNB should raise the suspicion for coexisting sleep apnea (Hilmisson et al., 2019b). Schramm et al. (2013) studied CPC in a group of primary insomnia and compared to a group of good sleepers. They found increased LFC and a lower HFC/LFC ratio among the insomnia group (Schramm et al., 2013). Zhang et al. (2021) studied insomnia patients with cognitive impairment and found decreased HFC indicating predominance of unstable sleep compared to insomnia patients with normal cognition. However, Jarrin et al. (2016) found that improvements in some sleep parameters in insomnia patients subjected to 6 weeks of cognitive behavior therapy was associated with decreased HFC. One of the limitations of this study was absence of control group. A systematic review of cardiovascular autonomic activity in insomnia patients showed that increased LFC/HFC ratio is a consistent finding in those patients (Nano et al., 2017). Similar findings of increased LFC/HFC ratio were also seen in CPC studies of populations with conditions that would predispose them to secondary insomnia/short sleep durations including: sleep deprivation, fibromyalgia, and periodic limb movement disorder (Sforza et al., 2005; Thomas et al., 2010; Chien et al., 2013; Lin et al., 2013).
Since insomnia is common in patients with uncontrolled psychiatric disorders (Sateia and Nowell, 2004); CPC could be used in studying and tracking treatment response in such patients. In comparison to controls, patients with untreated major depression have reduced HFC and increased LFC (Yang et al., 2011). Sun et al. (2019) studied 41 patients with depression and showed that the increase in HFC following 2 weeks of antidepressant medications treatment was associated with improvement in psychiatric questionnaire scores and suggested that this can be used to predict early response to treatment in such patients. Table 1 (section C) summarizes the studies that used CPC in sleep disorders other than sleep apnea.
The CPC sleep spectrogram provides a novel window into sleep physiology and key information about sleep during health and disease. Because such data can be obtained from simple/reduced and even contactless signal acquisition methods, it allows studying sleep in greater numbers, and with greater ease, in a wider range of conditions, with nearly limitless repeatability, than typically possible with traditional polysomnograms or current home sleep apnea testing devices.
HA and RT wrote the manuscript. All authors contributed to study design and the literature search, and revised the manuscript.
RC1HL099749-01 (National Heart Lung Blood Institute, United States) to RT.
RT has the following disclosures: (1) Patent for a device to regulate CO2 in the positive airway pressure circuit, for treatment of central/complex apnea. (2) Patent and license for an ECG-based method to phenotype sleep quality and sleep apnea (to MyCardio, LLC, through Beth Israel Deaconess Medical Center). (3) Patent, past consultant—DeVilbiss-Drive, CPAP auto-titrating algorithm. (4) GLG Councils and Guidepoint Global– general sleep medicine consulting.
The remaining authors declare that the research was conducted in the absence of any commercial or financial relationships that could be construed as a potential conflict of interest.
All claims expressed in this article are solely those of the authors and do not necessarily represent those of their affiliated organizations, or those of the publisher, the editors and the reviewers. Any product that may be evaluated in this article, or claim that may be made by its manufacturer, is not guaranteed or endorsed by the publisher.
Acquavella, J., Mehra, R., Bron, M., Suomi, J. M.-H., and Hess, G. P. (2020). Prevalence of narcolepsy and other sleep disorders and frequency of diagnostic tests from 2013-2016 in insured patients actively seeking care. J. Clin. Sleep Med. JCSM 16, 1255–1263. doi: 10.5664/jcsm.8482
Ako, M., Kawara, T., Uchida, S., Miyazaki, S., Nishihara, K., Mukai, J., et al. (2003). Correlation between electroencephalography and heart rate variability during sleep. Psychiatry Clin. Neurosci. 57, 59–65. doi: 10.1046/j.1440-1819.2003.01080.x
Al Ashry, H. S., Hilmisson, H., Ni, Y., and Thomas, R. J. (2021). Automated Apnea-Hypopnea Index from Oximetry and Spectral Analysis of Cardiopulmonary Coupling. Ann. Am. Thoracic Soc. 18, 876–883. doi: 10.1513/AnnalsATS.202005-510OC
Al-Abed, M. A., Manry, M., Burk, J. R., Lucas, E. A., and Behbehani, K. (2009). Sleep disordered breathing detection using heart rate variability and R-peak envelope spectrogram. Annu. Int. Conf. IEEE Eng. Med. Biol. Soc. 2009, 7106–7109. doi: 10.1109/IEMBS.2009.5332897
Appel, M. L., Berger, R. D., Saul, J. P., Smith, J. M., and Cohen, R. J. (1989). Beat to beat variability in cardiovascular variables: Noise or music? J. Am. Coll. Cardiol. 14, 1139–1148. doi: 10.1016/0735-1097(89)90408-7
Bartsch, R. P., Schumann, A. Y., Kantelhardt, J. W., Penzel, T., and Ivanov, P. C. (2012). Phase transitions in physiologic coupling. Proc. Natl. Acad. Sci. U S A. 109, 10181–10186. doi: 10.1073/pnas.1204568109
Ben-Tal, A., Shamailov, S. S., and Paton, J. F. R. (2012). Evaluating the physiological significance of respiratory sinus arrhythmia: Looking beyond ventilation-perfusion efficiency. J. Physiol. 590, 1989–2008. doi: 10.1113/jphysiol.2011.222422
Brandenberger, G., Ehrhart, J., Piquard, F., and Simon, C. (2001). Inverse coupling between ultradian oscillations in delta wave activity and heart rate variability during sleep. Clin. Neurophysiol. 112, 992–996. doi: 10.1016/s1388-2457(01)00507-7
Chang, K.-M. (2009). Portable obstructive sleep apnea screening system using overnight ECG and a PDA-based wireless transmission system. Telemed. J. E Health 15, 353–361. doi: 10.1089/tmj.2008.0142
Chen, J., and He, S. (2019). Drug-induced sleep endoscopy-directed adenotonsillectomy in pediatric obstructive sleep apnea with small tonsils. PLoS One 14:e0212317. doi: 10.1371/journal.pone.0212317
Chien, P.-L., Su, H.-F., Hsieh, P.-C., Siao, R.-Y., Ling, P.-Y., and Jou, H.-J. (2013). Sleep Quality among Female Hospital Staff Nurses. Sleep Disord. 2013:283490. doi: 10.1155/2013/283490
Cho, J. H., and Kim, H. J. (2017). The effect of continuous positive airway pressure on cardiopulmonary coupling. Sleep Breathing Schlaf Atmung 21, 341–345. doi: 10.1007/s11325-016-1419-8
Choi, J. H., Thomas, R. J., Suh, S. Y., Park, I. H., Kim, T. H., Lee, S. H., et al. (2015). Sleep quality change after upper airway surgery in obstructive sleep apnea: Electrocardiogram-based cardiopulmonary coupling analysis. Laryngoscope 125, 1737–1742. doi: 10.1002/lary.25101
Cosgrave, J., Phillips, J., Haines, R., Foster, R. G., Steinsaltz, D., and Wulff, K. (2021). Revisiting nocturnal heart rate and heart rate variability in insomnia: A polysomnography-based comparison of young self-reported good and poor sleepers. J. Sleep Res. 30:e13278.
Cui, J., Huang, Z., Wu, J., and Jiang, H. (2020). Cardiopulmonary Resonance Function and Indices—A Quantitative Measurement for Respiratory Sinus Arrhythmia. Front. Physiol. 11:867. doi: 10.3389/fphys.2020.00867
Dick, T. E., Hsieh, Y.-H., Dhingra, R. R., Baekey, D. M., Galán, R. F., Wehrwein, E., et al. (2014). Cardiorespiratory coupling: Common rhythms in cardiac, sympathetic, and respiratory activities. Prog. Brain Res. 209, 191–205. doi: 10.1016/B978-0-444-63274-6.00010-2
Dodds, K. L., Miller, C. B., Kyle, S. D., Marshall, N. S., and Gordon, C. J. (2017). Heart rate variability in insomnia patients: A critical review of the literature. Sleep Med. Rev. 33, 88–100.
Dutta, R., Delaney, G., Toson, B., Jordan, A. S., White, D. P., Wellman, A., et al. (2021). A Novel Model to Estimate Key Obstructive Sleep Apnea Endotypes from Standard Polysomnography and Clinical Data and Their Contribution to Obstructive Sleep Apnea Severity. Ann. Am. Thorac. Soc. 18, 656–667.
Eddie, D., Bentley, K. H., Bernard, R., Yeung, A., Nyer, M., Pedrelli, P., et al. (2020). Major depressive disorder and insomnia: Exploring a hypothesis of a common neurological basis using waking and sleep-derived heart rate variability. J. Psychiatr. Res. 123, 89–94.
Edwards, B. A., Sands, S. A., Eckert, D. J., White, D. P., Butler, J. P., Owens, R. L., et al. (2012). Acetazolamide improves loop gain but not the other physiological traits causing obstructive sleep apnoea. J. Physiol. 590, 1199–1211.
Edwards, B. A., Sands, S. A., Owens, R. L., Eckert, D. J., Landry, S., White, D. P., et al. (2016). The Combination of Supplemental Oxygen and a Hypnotic Markedly Improves Obstructive Sleep Apnea in Patients with a Mild to Moderate Upper Airway Collapsibility. Sleep 39, 1973–1983.
Finnsson, E., Ólafsdóttir, G. H., Loftsdóttir, D. L., Jónsson, S. Æ, Helgadóttir, H., Ágústsson, J. S., et al. (2021). A scalable method of determining physiological endotypes of sleep apnea from a polysomnographic sleep study. Sleep 44:zsaa168.
Franklin, K. A., and Lindberg, E. (2015). Obstructive sleep apnea is a common disorder in the population-a review on the epidemiology of sleep apnea. J. Thoracic Dis. 7, 1311–1322. doi: 10.3978/j.issn.2072-1439.2015.06.11
Galletly, D. C., and Larsen, P. D. (1998). Relationship between cardioventilatory coupling and respiratory sinus arrhythmia. Br. J. Anaesthes. 80, 164–168. doi: 10.1093/bja/80.2.164
Gao, L., Li, P., Hu, C., To, T., Patxot, M., Falvey, B., et al. (2019). Nocturnal heart rate variability moderates the association between sleep-wake regularity and mood in young adults. Sleep 42:zsz034.
Gilman, M. P., Floras, J. S., Usui, K., Kaneko, Y., Leung, R. S. T., and Bradley, T. D. (2008). Continuous positive airway pressure increases heart rate variability in heart failure patients with obstructive sleep apnoea. Clin. Sci. 114, 243–249. doi: 10.1042/CS20070172
Guo, D., Peng, C.-K., Wu, H.-L., Mietus, J. E., Liu, Y., Sun, R.-S., et al. (2011). ECG-derived cardiopulmonary analysis of pediatric sleep-disordered breathing. Sleep Med. 12, 384–389. doi: 10.1016/j.sleep.2010.09.011
Harrington, J., Schramm, P. J., Davies, C. R., and Lee-Chiong, T. L. (2013). An electrocardiogram-based analysis evaluating sleep quality in patients with obstructive sleep apnea. Sleep Breathing Schlaf Atmung 17, 1071–1078. doi: 10.1007/s11325-013-0804-9
Hayano, J., Watanabe, E., Saito, Y., Sasaki, F., Fujimoto, K., Nomiyama, T., et al. (2011). Screening for obstructive sleep apnea by cyclic variation of heart rate. Circulat. Arrhythmia Electrophysiol. 4, 64–72. doi: 10.1161/CIRCEP.110.958009
Heneghan, C., de Chazal, P., Ryan, S., Chua, C.-P., Doherty, L., Boyle, P., et al. (2008). Electrocardiogram recording as a screening tool for sleep disordered breathing. J. Clin. Sleep Med. JCSM 4, 223–228.
Hilmisson, H., Berman, S., and Magnusdottir, S. (2020). Sleep apnea diagnosis in children using software-generated apnea-hypopnea index (AHI) derived from data recorded with a single photoplethysmogram sensor (PPG): Results from the Childhood Adenotonsillectomy Study (CHAT) based on cardiopulmonary coupling analysis. Sleep Breathing 24, 1739–1749. doi: 10.1007/s11325-020-02049-6
Hilmisson, H., Lange, N., and Duntley, S. P. (2019a). Sleep apnea detection: Accuracy of using automated ECG analysis compared to manually scored polysomnography (apnea hypopnea index). Sleep Breathing 23, 125–133. doi: 10.1007/s11325-018-1672-0
Hilmisson, H., Sveinsdottir, E., Lange, N., and Magnusdottir, S. (2019b). Insomnia symptoms in primary care: A prospective study focusing on prevalence of undiagnosed co-morbid sleep disordered breathing. Eur. J. Int. Med. 63, 19–26. doi: 10.1016/j.ejim.2019.01.011
Hyunbin, K., Heenam, Y., Dawoon, J., Choi, S., Choi, J., Lee, Y., et al. (2017). Heart rate variability in patients with major depressive disorder and healthy controls during non-REM sleep and REM sleep. Annu. Int. Conf. IEEE Eng. Med. Biol. Soc. 2017, 2312–2315.
Jarrin, D. C., Chen, I. Y., Ivers, H., Lamy, M., Vallières, A., and Morin, C. M. (2016). Nocturnal heart rate variability in patients treated with cognitive-behavioral therapy for insomnia. Health Psychol. 35, 638–641. doi: 10.1037/hea0000347
Leanderson, S., Laguna, P., and Sörnmo, L. (2003). Estimation of the respiratory frequency using spatial information in the VCG. Med. Engine. Phys. 25, 501–507. doi: 10.1016/s1350-4533(03)00017-1
Lee, S. H., Choi, J. H., Park, I. H., Lee, S. H., Kim, T. H., Lee, H. M., et al. (2012). Measuring sleep quality after adenotonsillectomy in pediatric sleep apnea. Laryngoscope 122, 2115–2121. doi: 10.1002/lary.23356
Lee, W. H., Ahn, J.-C., We, J., Rhee, C.-S., Lee, C. H., Yun, P.-Y., et al. (2014). Cardiopulmonary coupling analysis: Changes before and after treatment with a mandibular advancement device. Sleep Breathing 18, 891–896. doi: 10.1007/s11325-014-0961-5
Lee, W. H., Hong, S.-N., Kim, H. J., Rhee, C.-S., Lee, C. H., Yoon, I.-Y., et al. (2016). A Comparison of Different Success Definitions in Non-Continuous Positive Airway Pressure Treatment for Obstructive Sleep Apnea Using Cardiopulmonary Coupling. J. Clin. Sleep Med. JCSM 12, 35–41. doi: 10.5664/jcsm.5388
Lin, Y.-H., Ho, Y.-C., Lin, S.-H., Yeh, Y.-H., Liu, C.-Y., Kuo, T. B. J., et al. (2013). On-call duty effects on sleep-state physiological stability in male medical interns. PLoS One 8:e65072. doi: 10.1371/journal.pone.0065072
Liu, D., Yang, X., Wang, G., Ma, J., Liu, Y., Peng, C.-K., et al. (2012). HHT based cardiopulmonary coupling analysis for sleep apnea detection. Sleep Med. 13, 503–509. doi: 10.1016/j.sleep.2011.10.035
Lu, M., Fang, F., Sanderson, J. E., Ma, C., Wang, Q., Zhan, X., et al. (2019). Validation of a portable monitoring device for the diagnosis of obstructive sleep apnea: Electrocardiogram-based cardiopulmonary coupling. Sleep Breathing Schlaf Atmung 23, 1371–1378. doi: 10.1007/s11325-019-01922-3
Ma, Y., Sun, S., Zhang, M., Guo, D., Liu, A. R., Wei, Y., et al. (2020). Electrocardiogram-based sleep analysis for sleep apnea screening and diagnosis. Sleep Breathing 24, 231–240. doi: 10.1007/s11325-019-01874-8
Magnusdottir, S., and Hilmisson, H. (2018). Ambulatory screening tool for sleep apnea: Analyzing a single-lead electrocardiogram signal (ECG). Sleep Breathing 22, 421–429. doi: 10.1007/s11325-017-1566-6
Nano, M.-M., Fonseca, P., Vullings, R., and Aarts, R. M. (2017). Measures of cardiovascular autonomic activity in insomnia disorder: A systematic review. PLoS One 12:e0186716. doi: 10.1371/journal.pone.0186716
Oppersma, E., Ganglberger, W., Sun, H., Thomas, R. J., and Westover, M. B. (2021). Algorithm for automatic detection of self-similarity and prediction of residual central respiratory events during continuous positive airway pressure. Sleep 44:zsaa215.
Qin, H., Steenbergen, N., Glos, M., Wessel, N., Kraemer, J. F., et al. (2021). The Different Facets of Heart Rate Variability in Obstructive Sleep Apnea. Front. Psychiatry 12:642333. doi: 10.3389/fpsyt.2021.642333
Ramar, K., Desrues, B., Ramar, P., and Morgenthaler, T. I. (2013). Analysis of cardiopulmonary coupling to assess adaptive servo-ventilation success in complex sleep apnea management. Sleep Breathing 17, 861–866. doi: 10.1007/s11325-012-0780-5
Roche, F., Court-Fortune, I., Pichot, V., Duverney, D., Costes, F., Emonot, A., et al. (1999). Reduced cardiac sympathetic autonomic tone after long-term nasal continuous positive airway pressure in obstructive sleep apnoea syndrome. Clin. Physiol. 19, 127–134. doi: 10.1046/j.1365-2281.1999.00163.x
Roche, F., Pichot, V., Sforza, E., Court-Fortune, I., Duverney, D., Costes, F., et al. (2003). Predicting sleep apnoea syndrome from heart period: A time-frequency wavelet analysis. Eur. Respirat. J. 22, 937–942. doi: 10.1183/09031936.03.00104902
Roche, F., Sforza, E., Duverney, D., Borderies, J.-R., Pichot, V., Bigaignon, O., et al. (2004). Heart rate increment: An electrocardiological approach for the early detection of obstructive sleep apnoea/hypopnoea syndrome. Clin. Sci. 107, 105–110. doi: 10.1042/CS20040036
Sands, S. A., Edwards, B. A., Terrill, P. I., Butler, J. P., Owens, R. L., Taranto-Montemurro, L., et al. (2018). Identifying obstructive sleep apnoea patients responsive to supplemental oxygen therapy. Eur. Respir. J. 52:1800674.
Sateia, M. J., and Nowell, P. D. (2004). Insomnia. Lancet 364, 1959–1973. doi: 10.1016/S0140-6736(04)17480-1
Schramm, P. J., and Thomas, R. J. (2012). Assessment of therapeutic options for mild obstructive sleep apnea using cardiopulmonary coupling measures. J. Clin. Sleep Med. JCSM 8, 315–320. doi: 10.5664/jcsm.1924
Schramm, P. J., Thomas, R., Feige, B., Spiegelhalder, K., and Riemann, D. (2013). Quantitative measurement of sleep quality using cardiopulmonary coupling analysis: A retrospective comparison of individuals with and without primary insomnia. Sleep Breathing 17, 713–721. doi: 10.1007/s11325-012-0747-6
Seo, M. Y., Yoo, J., Hwang, S. J., and Lee, S. H. (2020). Diagnosis of Obstructive Sleep Apnea in Adults Using the Cardiopulmonary Coupling-Derived Software-Generated Apnea-Hypopnea Index. Clin. Exp. Otorhinolaryngol. 2020:01984. doi: 10.21053/ceo.2020.01984
Sforza, E., Pichot, V., Barthelemy, J. C., Haba-Rubio, J., and Roche, F. (2005). Cardiovascular variability during periodic leg movements: A spectral analysis approach. Clin. Neurophysiol. 116, 1096–1104. doi: 10.1016/j.clinph.2004.12.018
Shiina, K., Tomiyama, H., Takata, Y., Yoshida, M., Kato, K., Saruhara, H., et al. (2010). Effects of CPAP therapy on the sympathovagal balance and arterial stiffness in obstructive sleep apnea. Respir. Med. 104, 911–916. doi: 10.1016/j.rmed.2010.01.010
Somers, V. K., Dyken, M. E., Mark, A. L., and Abboud, F. M. (1993). Sympathetic-nerve activity during sleep in normal subjects. New Engl. J. Med. 328, 303–307. doi: 10.1056/NEJM199302043280502
Spiegelhalder, K., Fuchs, L., Ladwig, J., Kyle, S. D., Nissen, C., Voderholzer, U., et al. (2011). Heart rate and heart rate variability in subjectively reported insomnia. J. Sleep Res. 20, 137–145.
Sun, Q.-M., Xing, L., Wang, C., and Liang, W. (2019). Cardiopulmonary coupling analysis predicts early treatment response in depressed patients: A pilot study. Psychiatry Res. 276, 6–11. doi: 10.1016/j.psychres.2019.04.002
Thayer, J. F., Peasley, C., and Muth, E. R. (1996). Estimation of respiratory frequency from autoregressive spectral analysis of heart period. Biomed. Sci. Instrument. 32, 93–99.
Thomas, R. J., Mietus, J. E., Peng, C.-K., and Goldberger, A. L. (2005). An electrocardiogram-based technique to assess cardiopulmonary coupling during sleep. Sleep 28, 1151–1161. doi: 10.1093/sleep/28.9.1151
Thomas, R. J., Mietus, J. E., Peng, C.-K., Gilmartin, G., Daly, R. W., Goldberger, A. L., et al. (2007). Differentiating obstructive from central and complex sleep apnea using an automated electrocardiogram-based method. Sleep 30, 1756–1769. doi: 10.1093/sleep/30.12.1756
Thomas, R. J., Mietus, J. E., Peng, C.-K., Goldberger, A. L., Crofford, L. J., and Chervin, R. D. (2010). Impaired sleep quality in fibromyalgia: Detection and quantification with ECG-based cardiopulmonary coupling spectrograms. Sleep Med. 11, 497–498. doi: 10.1016/j.sleep.2009.09.003
Thomas, R. J., Mietus, J. E., Peng, C.-K., Guo, D., Gozal, D., Montgomery-Downs, H., et al. (2014). Relationship between delta power and the electrocardiogram-derived cardiopulmonary spectrogram: Possible implications for assessing the effectiveness of sleep. Sleep Med. 15, 125–131. doi: 10.1016/j.sleep.2013.10.002
Thomas, R. J., Shin, C., Bianchi, M. T., Kushida, C., and Yun, C.-H. (2017a). Distinct polysomnographic and ECG-spectrographic phenotypes embedded within obstructive sleep apnea. Sleep Sci. Pract. 1:11.
Thomas, R. J., Wood, C., and Bianchi, M. T. (2017b). Cardiopulmonary coupling spectrogram as an ambulatory clinical biomarker of sleep stability and quality in health, sleep apnea, and insomnia. Sleep 41:zsx196. doi: 10.1093/sleep/zsx196
Thomas, R. J., Wood, C., Bianchi, M. T., Kushida, C., and Yun, C. (2018). Cardiopulmonary coupling spectrogram as an ambulatory clinical biomarker of sleep stability and quality in health, sleep apnea, and insomnia. Sleep 41:zsx196. doi: 10.1093/sleep/zsx196
Tobaldini, E., Nobili, L., Strada, S., Casali, K. R., Braghiroli, A., and Montano, N. (2013). Heart rate variability in normal and pathological sleep. Front. Physiol. 4:294. doi: 10.3389/fphys.2013.00294
Ucak, S., Dissanayake, H. U., Sutherland, K., de Chazal, P., and Cistulli, P. A. (2021). Heart rate variability and obstructive sleep apnea: Current perspectives and novel technologies. J. Sleep Res. 30:e13274.
Vazir, A., Dayer, M., Hastings, P. C., McIntyre, H. F., Henein, M. Y., Poole-Wilson, P. A., et al. (2006). Can heart rate variation rule out sleep-disordered breathing in heart failure? Eur. Respir. J. 27, 571–577. doi: 10.1183/09031936.06.00130904
Wood, C., Bianchi, M. T., Yun, C. H., Shin, C., and Thomas, R. J. (2020). Multicomponent Analysis of Sleep Using Electrocortical, Respiratory, Autonomic and Hemodynamic Signals Reveals Distinct Features of Stable and Unstable NREM and REM Sleep. Front. Physiol. 11:592978. doi: 10.3389/fphys.2020.592978
Yang, A. C., Yang, C.-H., Hong, C.-J., Tsai, S.-J., Kuo, C.-H., Peng, C.-K., et al. (2011). Sleep state instabilities in major depressive disorder: detection and quantification with electrocardiogram-based cardiopulmonary coupling analysis. Psychophysiology 48, 285–291. doi: 10.1111/j.1469-8986.2010.01060.x
Yasuma, F., and Hayano, J.-I. (2004). Respiratory sinus arrhythmia: why does the heartbeat synchronize with respiratory rhythm? Chest 125, 683–690. doi: 10.1378/chest.125.2.683
Younes, M., Ostrowski, M., Soiferman, M., Younes, H., Younes, M., Raneri, J., et al. (2015). Odds ratio product of sleep EEG as a continuous measure of sleep state. Sleep 38, 641–654.
Zemaityte, D., Varoneckas, G., and Sokolov, E. (1984). Heart rhythm control during sleep. Psychophysiology 21, 279–289. doi: 10.1111/j.1469-8986.1984.tb02935.x
Zhang, X., Song, B., Liu, Y., Wan, Y., Zhou, K., and Xue, R. (2021). Cognitive deficit is correlated with sleep stability in insomnia: a cardiopulmonary coupling study. Brain Behav. e02068. doi: 10.1002/brb3.2068
Keywords: cardiopulmonary coupling (CPC), heart rate variability, sleep apnea, stable sleep, insomnia
Citation: Al Ashry HS, Ni Y and Thomas RJ (2021) Cardiopulmonary Sleep Spectrograms Open a Novel Window Into Sleep Biology—Implications for Health and Disease. Front. Neurosci. 15:755464. doi: 10.3389/fnins.2021.755464
Received: 08 August 2021; Accepted: 08 October 2021;
Published: 12 November 2021.
Edited by:
David Gozal, University of Missouri, United StatesReviewed by:
Danny Joel Eckert, Flinders University, AustraliaCopyright © 2021 Al Ashry, Ni and Thomas. This is an open-access article distributed under the terms of the Creative Commons Attribution License (CC BY). The use, distribution or reproduction in other forums is permitted, provided the original author(s) and the copyright owner(s) are credited and that the original publication in this journal is cited, in accordance with accepted academic practice. No use, distribution or reproduction is permitted which does not comply with these terms.
*Correspondence: Haitham S. Al Ashry, aGFsYXNocnlAZWxsaW90LWhzLm9yZw==
Disclaimer: All claims expressed in this article are solely those of the authors and do not necessarily represent those of their affiliated organizations, or those of the publisher, the editors and the reviewers. Any product that may be evaluated in this article or claim that may be made by its manufacturer is not guaranteed or endorsed by the publisher.
Research integrity at Frontiers
Learn more about the work of our research integrity team to safeguard the quality of each article we publish.