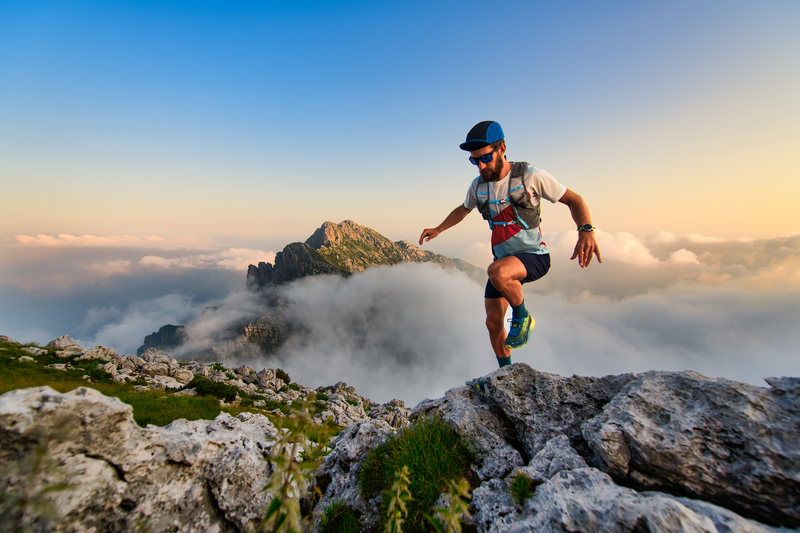
94% of researchers rate our articles as excellent or good
Learn more about the work of our research integrity team to safeguard the quality of each article we publish.
Find out more
ORIGINAL RESEARCH article
Front. Neurosci. , 03 September 2021
Sec. Neurogenomics
Volume 15 - 2021 | https://doi.org/10.3389/fnins.2021.680762
This article is part of the Research Topic Shared Genetic Risk Factors among Psychiatric Diseases and other Medical Diseases and Traits View all 8 articles
Impaired phonological processing is a leading symptom of multifactorial language and learning disorders suggesting a common biological basis. Here we evaluated studies of dyslexia, dyscalculia, specific language impairment (SLI), and the logopenic variant of primary progressive aphasia (lvPPA) seeking for shared risk genes in Broca’s and Wernicke’s regions, being key for phonological processing within the complex language network. The identified “phonology-related genes” from literature were functionally characterized using Atlas-based expression mapping (JuGEx) and gene set enrichment. Out of 643 publications from the last decade until now, we extracted 21 candidate genes of which 13 overlapped with dyslexia and SLI, six with dyslexia and dyscalculia, and two with dyslexia, dyscalculia, and SLI. No overlap was observed between the childhood disorders and the late-onset lvPPA often showing symptoms of learning disorders earlier in life. Multiple genes were enriched in Gene Ontology terms of the topics learning (CNTNAP2, CYFIP1, DCDC2, DNAAF4, FOXP2) and neuronal development (CCDC136, CNTNAP2, CYFIP1, DCDC2, KIAA0319, RBFOX2, ROBO1). Twelve genes showed above-average expression across both regions indicating moderate-to-high gene activity in the investigated cortical part of the language network. Of these, three genes were differentially expressed suggesting potential regional specializations: ATP2C2 was upregulated in Broca’s region, while DNAAF4 and FOXP2 were upregulated in Wernicke’s region. ATP2C2 encodes a magnesium-dependent calcium transporter which fits with reports about disturbed calcium and magnesium levels for dyslexia and other communication disorders. DNAAF4 (formerly known as DYX1C1) is involved in neuronal migration supporting the hypothesis of disturbed migration in dyslexia. FOXP2 is a transcription factor that regulates a number of genes involved in development of speech and language. Overall, our interdisciplinary and multi-tiered approach provided evidence that genetic and transcriptional variation of ATP2C2, DNAAF4, and FOXP2 may play a role in physiological and pathological aspects of phonological processing.
Developmental language and learning disorders severely impair children’s abilities in speaking, reading, writing, calculating, and combinations thereof (American Psychiatric Association, 2013). One cognitive domain commonly involved in the majority of these disorders is phonological processing which refers to the analysis and synthesis of the sound structure of spoken and written language (phonological awareness), phonological representations and the rapid access (rapid automatized naming) or memorizing (phonological working memory) thereof. The different phonological domains are highly inter-correlated and part of the shared construct of phonological processing.
At the behavioral level, patients with dyslexia, dyscalculia, or specific language impairment (SLI) show deficits in key domains of phonological processing such as phonological awareness, phonological working memory, and/or speeded access to phonological codes during rapid automatized naming, as summarized in Supplementary Table 1. Dyslexia is primarily associated with impaired phonological awareness (Steinbrink et al., 2008; Pennington and Bishop, 2009; Hasselhorn et al., 2010; Child et al., 2019). This, like rapid automatized naming, may be considered as a predictive factor for early-onset dyslexia (Thompson et al., 2015). In addition, dyslexia patients perform poorly on phonological working memory (De Carvalho et al., 2014; Child et al., 2019) and show deficits in phonological representations leading to difficulties in phoneme awareness and phonological coding (Pennington and Bishop, 2009). In dyscalculia, connections between phonological processing and arithmetic abilities can be observed (De Smedt and Boets, 2010; Raghubar et al., 2010; De Smedt, 2018). The phonological working memory was identified to serve as a short-time buffer for mathematical operations based on the triple code model which codes numbers as numerals, number words, and abstract numerosities (Dehaene, 1992; Dehaene et al., 2003; Träff and Passolunghi, 2015; Pollack and Ashby, 2018). Patients with SLI, especially with a speech sound disorder, exhibit an inadequate phonological realization and use of particular phonemes in spontaneous speech due to impaired phonological awareness abilities and a lower quality of phonological representations (Lewis et al., 2011; Claessen and Leitão, 2012; American Psychiatric Association, 2013). Some studies also showed lower scores in SLI in phonological working memory increasing the language difficulties (Torrens and Yagüe, 2016).
In addition to impaired phonological processing with effects on language, reading, and mathematical abilities early in life, phonological deficits may have an onset late in life, e.g., in patients with primary progressive aphasia, a progressive neurodegenerative disease (Magnin et al., 2016). The logopenic variant of primary progressive aphasia (lvPPA) was included in this research for this reason and due to high comorbidity with developmental learning disorders meaning that lvPPA patients have a higher probability for these disorders in childhood (Rogalski et al., 2008). Like children with SLI, adults with lvPPA exhibit phonological paraphasias, i.e., errors in the phonological realization of a word, in spontaneous speech as well as in naming (Gorno-Tempini et al., 2011). One central mechanism for these lvPPA symptoms is a deficit of phonological working memory. In addition, alterations in phonological representations may be observable (Gorno-Tempini et al., 2008; Henry et al., 2016).
Phonological processing is related to the language areas of the fronto-temporal network, in particular Broca’s region (opercular and triangular parts of the left inferior frontal gyrus) and Wernicke’s region (left inferior parietal lobule and posterior superior temporal gyrus; Müller and Knight, 2006; Rogalsky and Hickok, 2011; Hartwigsen, 2015, 2016; Binder, 2017; Klaus and Hartwigsen, 2019; Yi et al., 2019; Hartwigsen et al., 2020). Broca’s and Wernicke’s regions represent central nodes of the phonological processing network, which are integrated into phonological processes in phonological production as well as in phonological perception (Heim et al., 2003; Indefrey and Levelt, 2004; Friederici, 2011; Indefrey, 2011; Hagoort and Indefrey, 2014). While Broca’s region is associated with phonological word fluency, phonological decisions, and the phonological loop (Heim et al., 2008, 2009a,b; Aboitiz et al., 2010; Liakakis et al., 2011; Katzev et al., 2013; Wagner et al., 2014; Klaus and Hartwigsen, 2019), Wernicke’s region is involved in phonological speech perception and auditory word-form recognition (Buchsbaum et al., 2001; DeWitt and Rauschecker, 2013). Both regions are connected by the language-relevant pathways, arcuate fasciculus and superior longitudinal fasciculus (Catani et al., 2005; Friederici, 2009, 2011, 2012; Friederici and Gierhan, 2013). Both fasciculi are associated with the transport of order information as a part of the phonological loop (Papagno et al., 2017) and the arcuate fasciculus is also involved in automatic repetition (Catani et al., 2005; Berthier et al., 2012; Papagno et al., 2017). Furthermore, a high functional connectivity between Broca’s and Wernicke’s regions based on the arcuate fasciculus leads to a higher linguistic performance, e.g., in phonological word learning (López-Barroso et al., 2013).
Functional resonance imaging and diffusion tensor imaging studies suggested that Broca’s and Wernicke’s regions are affected in patients with SLI, dyslexia, dyscalculia, and also the lvPPA (McCrone, 2002; Sonty et al., 2007; Heim et al., 2010; Verhoeven et al., 2012; Dinkel et al., 2013). In children with SLI, an abnormal connection between both regions was reported in contrast to age-matched controls, i.e., a significantly reduced mean fractional anisotropy was shown in the superior longitudinal fasciculus (Verhoeven et al., 2012). Dyslexia patients showed lower activations in Broca’s region during phonological decision tasks as well as in Wernicke’s region during auditory discrimination tasks (Heim et al., 2010). In dyscalculia patients, both regions are the common regions for the interaction of phonological processing and mathematical abilities (Pollack and Ashby, 2018). Additionally, reduced connectivity between Wernicke’s region and the intraparietal sulcus was found in dyscalculia patients (McCrone, 2002). Moreover, Broca’s region was excessively activated in dyscalculia patients during a calculation task (Dinkel et al., 2013). In lvPPA patients, Sonty et al. (2007) reported a lower effective connectivity (dysfunctional network interaction) between Broca’s and Wernicke’s regions.
Twin studies suggest that reading and language skills are heritable, with influences from both genetic factors and environmental factors (Hensler et al., 2010; Wadsworth et al., 2010; Tosto et al., 2017; Rice et al., 2020). Similar to developmental disorders, primary progressive aphasia also has a genetic component (Henry and Gorno-Tempini, 2010; Kim et al., 2016). Yet, there are no systematic approaches published that relate genes to the domains of phonological processing, but rather only to isolated phonological features within language and/or learning disorders or the physiological language. Furthermore, there is no study about a specific expression analysis of language-related genes in Broca’s and Wernicke’s regions of the adult human brain. However, two studies investigated the developing brain using data-driven, whole-transcriptome approaches to identify profiles of regionally co- or differentially expressed genes. Johnson et al. (2009) screened different brain regions in human fetuses including the perisylvian cortex (ventrolateral prefrontal, motor-somatosensory, parietal association, temporal auditory), where FOXP2 showed a modest upregulation. Lambert et al. (2011) directly screened precursor structures of Broca’s and Wernicke’s regions in the fetal brain and found several FOXP2 regulated genes among the differentially expressed genes. An overview of the other recent studies on Broca’s and Wernicke’s regions in humans and model organisms is provided by Supplementary Table 2.
Given that the named language and/or learning disorders in childhood and late adulthood have commonalities in (a) phonological processing deficits as one cognitive signature, (b) repercussions in the neural processing circuits for phonology, and (c) genetic predispositions, we wanted to investigate whether there is a specific genetic contribution to general phonological processing principles for dyslexia, dyscalculia, SLI, and lvPPA. For this purpose, we developed a workflow to integrate clinical, neuroanatomical, and genetic knowledge about phonological processing abilities in these disorders (Figure 1). First, we performed a screening of the literature for potentially candidate genes for phonological processing (“phonology-related genes”) shared between the disorders. We then characterized these genes by enrichment analysis of gene functions and expression analysis in the two key regions involved in phonological processing (Broca’s and Wernicke’s regions) using a new computational tool (JuGEx, Bludau et al., 2018) for the joint statistical analysis of microstructural variability (Julich-Brain Atlas; Amunts et al., 2020) and transcriptional variability (Hawrylycz et al., 2012, 2015; Allen Human Brain Atlas, 2013) in adult human brains.
The following criteria were used to screen the literature and had to be fulfilled: (a) Publication date between 01/01/2010 and 23/05/2021, (b) investigation of at least one of the disorders dyslexia, dyscalculia, SLI, and lvPPA, (c) report of at least one human gene as significant original/replicated result (p < 0.05), (d) report of at least one gene as a risk factor for a disorder or trait/phenotype related with the disorder, (e) sample containing more than one individual (no single-case study), (f) sample of European origin to reduce a potential influence of population stratification on our results, (g) study either a linkage analysis, a genome-wide association study (GWAS), or a candidate gene association study.
Criterion (b) was not met if a disorder was named as an attendant symptom of another disorder, e.g., dyslexia as an attendant symptom of tuberous sclerosis. In contrast, articles primarily dealing with specific language and/or learning disorders with additional comorbidities were included, e.g., dyslexia with comorbid attention-deficit hyperactivity disorder. Our criteria referred to the terminology defined by the two most recent Diagnostic and Statistical Manuals of the American Psychiatric Association [DSM-IV (American Psychiatric Association, 1994); DSM-5 (American Psychiatric Association, 2013)] and exact synonyms. Studies investigating the normal variability of a trait in a population sample, e.g., reading skills in a cohort of healthy children, were only included if a direct relation to a corresponding pathology was reported, e.g., in terms of the analysis of risk genes for that pathology.
We performed eleven single searches fully covering the potential combinations of language and/or learning disorders (Supplementary Table 3) using PubMed Advanced Search1 with our search string, composed of clinical categories and genetic terms as described in Supplementary Table 4. Each search consisted of two analytical steps: First, a computational text mining of the abstracts and titles from all identified articles using PubTator2 (Wei et al., 2013). Second, a manual text mining of the full text was performed. Articles were selected for inclusion if they fulfilled all criteria a) to g). Clinical categories found in these articles were summarized in Supplementary Table 5. Candidate genes were extracted from research articles, while review articles were excluded from evaluation results because they did not represent original research results. Symbols and names of genes were checked and edited, if necessary, in order to match definitions by the Human Genome Nomenclature Committee3. To identify phonology-related genes, we looked for genes that were reported for more than one of the disorders using a Venn diagram tool4 : A phonology-related gene had to be involved in at least two of the four disorders (overlaps).
Genes were annotated by gene ontology (GO; Gene Ontology Consortium, 2021) using ToppFun, a tool of the ToppGene Suite5 (Chen et al., 2009). ToppFun was used to find out which GO terms and identifiers (as of 12/03/2021) from the categories “Biological Process,” “Molecular Function,” or “Cellular Component” were over-represented (enriched) in the list of phonology-related genes from the Venn diagram intersections. The following analytical parameters were chosen: hypergeometric probability mass function as p-value method, Bonferroni corrected q-value < 0.05, gene limits 1 ≤ n ≤ 2000, 2 as minimum feature count in the test set. To interpret the relationships of the identified GO terms, we used ancestor charts from QuickGo6. These charts are conceptual figures to represent a tree of hierarchically linked GO terms (parental and child terms). Under the same parameters, an enrichment analysis with different pathway annotations was performed with ToppFun which did not yield biologically meaningful significant results possibly due to the small number of candidate genes and/or limited functional annotations for these genes.
Gene expression differences between pairs of brain regions, technically referred to as volumes of interest (VOIs), were analyzed using the workflow and graphical user interfaces (GUIs) of JuGEx (Bludau et al., 2018). The tool is based on scripts coded in MATLAB (version R2018b, 64 bit; The MathWorks) which are freely accessible (available online7). JuGEx integrates regional RNA data from the Allen Human Brain Atlas from three right-handed, two left-handed, and one ambidextrous donors (Hawrylycz et al., 2012) with the three-dimensional cytoarchitectonic probability maps from the Julich-Brain Atlas (Amunts et al., 2020) which are based on observer-independent mapping of cytoarchitectonic areas in ten postmortem brains. Allen Human Brain Atlas and Julich-Brain data are based on brain tissue from deceased adult donors without a psychiatric or neurological diagnosis; the brains did not overlap between both atlas systems (Bludau et al., 2018). The expression data derived from anisotropically distributed tissue samples of Allen Human Brain Atlas and cytoarchitectonic maps from Julich-Brain were registered to the reference space of the Montreal Neuroscience Institute 152 (MNI152) reference brain (Evans et al., 2012). To enable a comparison of gene expression between VOIs in different brains, JuGEx uses relative expression values that were normalized across all regions and six brains from Allen Human Brain Atlas using z-scores (Hawrylycz et al., 2015).
A gene was investigated using the “all-probes mode” which calculates a winsorized mean based on the microarray probes for each gene (Bludau et al., 2018). We considered a p-value smaller than 0.05 as a significant difference between the z-scores of tissue samples in the compared VOIs. The nominal p-values from the permuted n-way analysis of variance (ANOVA) were corrected for multiple comparisons by applying a family-wise error (FWE) correction with the number of analyzed genes (n = 21, pFWE < 0.05). The permuted n-way ANOVA was repeated 10,000 times with randomly shuffled labels of the analyzed VOIs under the assumption of label exchangeability. The regional specificity of a gene was defined as significantly higher expression (z-score) in that area (first VOI) compared to another area (second VOI).
Main analysis: In order to define “Broca’s region” anatomically, the maps of areas 44 and 45, both cytoarchitectonically described by Amunts et al. (1999, 2004), were merged using JuGEx. We decided to merge both areas considering the spatial resolution of gene expression data in Allen Human Brain Atlas with rather large intervals between sections in relation to the size of the areas. The Te3 map of the Julich-Brain Atlas was used as a proxy for “Wernicke’s region,” a cytoarchitectonically defined area located in the lateral bulge of the superior temporal gyrus (Morosan et al., 2005). Te3 overlaps in parts with Brodmann’s area 22 as defined by Brodmann (1909). Since the left hemisphere is dominant for language in most right- and left-handers (Branch et al., 1964; Mazoyer et al., 2014), we investigated the activity (expression) of potentially functionally relevant candidate genes in the left hemispheres.
Regional specification analysis: To characterize the regional specificity in the frontal lobe, the Julich-Brain maps of premotor areas 6d1, 6d2, and 6d3 were combined and compared with Broca’s region. The same was done in the temporal lobe by merging the maps Fg1, Fg2, Fg3, and Fg4 of the fusiform gyrus and by comparing them with Wernicke’s region. We considered support for regional specificity if the candidate gene was not significantly upregulated in the non-language areas.
Control analysis: To further evaluate the main analysis, another comparison between Broca’s and Wernicke’s regions was performed with two additional gene sets. The first set was chosen from the Allen Human Brain Atlas genes and comprised 25 genes, hereafter called “random genes” (Supplementary Table 6A). The second set was intentionally taken from the Allen Human Brain Atlas genes and included 14 genes from GWAS of eye, hair, and skin coloration, hereafter called “color genes” (Supplementary Table 6B). Both random and color genes were assembled and considered as biological negative controls as described by Bludau et al. (2018).
The following numbers of tissue samples were identified through the aforementioned VOIs in the six brains from Allen Human Brain Atlas using a Julich-Brain map threshold of 20% (this means that only tissue samples were included with a probability of 20% or higher belonging to the corresponding VOIs): left areas 44 and 45 (ntissue samples = 25), left area Te3 (ntissue samples = 17), left areas 6d1, 6d2, and 6d3 (ntissue samples = 30), left areas Fg1, Fg2, Fg3, and Fg4 (ntissue samples = 57). Before statistical analyses, the spatial assignment of tissue samples to maps was manually checked using the “3D visualization” GUI of JuGEx.
The full data of the used maps are provided by the Julich-Brain Atlas via the Human Brain Project (available online8): Areas 44 (doi: 10.25493/N13Y-Y3F), 45 (doi: 10.25493/K06P-R2S), Te3 (doi: 10.25493/ZZQH-932), 6d1 (doi: 10.25493/KSY8-H3F), 6d2 (doi: 10.25493/WJQ5-HWC), 6d3 (doi: 10.25493/D41S-AG7), Fg1 (doi: 10.25493/RTDM-GK4), Fg2 (doi: 10.25493/N7ZP-17X), Fg3 (doi: 10.25493/F87E-JRA), and Fg4 (doi: 10.25493/MTWF-X4V).
The literature evaluation process comprised two main steps, a computational text mining of titles, abstracts, and key words, followed by a manual text mining of the whole text body and supplement, to find original research articles and genes (Figure 2). The computational text mining of dyslexia, dyscalculia, SLI, and lvPPA literature from the last 11 years using search strings of clinical categories and genetic terms (Supplementary Table 4) revealed 643 journal articles. Of these, 98 articles were found more than once, and additional 36 articles were excluded since they were reviews (Supplementary Table 7A). Thus, 509 unique research articles provided the basis for further manual text mining which led to the exclusion of another 438 articles that were filtered out with PubTator or did not meet the above mentioned inclusion criteria at whole-text screening. Eventually, 71 research articles remained reporting 77 potential candidate genes (Supplementary Tables 7B,C). For the final selection, we put the information of Supplementary Table 7C forward to a Venn diagram to seek for overlapping candidate genes from the different disorders (Figure 3).
Figure 3. Venn diagram used to determine phonology-related genes from candidate genes overlapping between language and/or learning disorders. The three black frames indicate overlaps of genes associated with at least two disorders. DysL, dyslexia; DysC, dyscalculia; SLI, specific language impairment; lvPPA, logopenic variant of primary progressive aphasia.
For dyslexia/SLI, we found ATP2C2 (Newbury et al., 2011; Müller et al., 2017; Martinelli et al., 2021), CCDC136 (Gialluisi et al., 2014; Adams et al., 2017), CMAHP (Eicher et al., 2014), CMIP (Newbury et al., 2011; Scerri et al., 2011; Luciano et al., 2018), CNTNAP2 (Newbury et al., 2011; Peter et al., 2011; Eicher et al., 2013; Luciano et al., 2018), COL4A2 (Eicher et al., 2013), FLNC (Gialluisi et al., 2014; Adams et al., 2017), FOXP2 (Peter et al., 2011; Wilcke et al., 2012; Eicher et al., 2013; Mozzi et al., 2017; Sánchez-Morán et al., 2018; Doust et al., 2020), KIAA0319 (Couto et al., 2010; Czamara et al., 2011; Elbert et al., 2011; König et al., 2011; Newbury et al., 2011; Scerri et al., 2011; Cope et al., 2012; Zou et al., 2012; Eicher et al., 2013, 2014; Powers et al., 2013, 2016; Mascheretti et al., 2015; Adams et al., 2017; Carrion-Castillo et al., 2017; Neef et al., 2017; Centanni et al., 2018; Luciano et al., 2018; Sánchez-Morán et al., 2018), NOP9 (Pettigrew et al., 2016), RBFOX2 (Gialluisi et al., 2014), RIPOR2 (Eicher et al., 2014), and ZNF385D (Eicher et al., 2013). For dyslexia/dyscalculia, we observed CYFIP1 (Ulfarsson et al., 2017), DNAAF4 (Bates et al., 2010; Marino et al., 2011; Paracchini et al., 2011; Mascheretti et al., 2013, 2015; Müller et al., 2017; Luciano et al., 2018), MYO18B (Ludwig et al., 2013), NIPA1 (Ulfarsson et al., 2017), NIPA2 (Ulfarsson et al., 2017), and TUBGCP5 (Ulfarsson et al., 2017). For dyslexia/dyscalculia/SLI, we found DCDC2 (Lind et al., 2010; Czamara et al., 2011; König et al., 2011; Marino et al., 2011, 2012, 2014; Newbury et al., 2011; Scerri et al., 2011; Cope et al., 2012; Eicher et al., 2013, 2014, 2015; Powers et al., 2013, 2016; Cicchini et al., 2015; Gori et al., 2015; Matsson et al., 2015; Adams et al., 2017; Neef et al., 2017; Luciano et al., 2018; Riva et al., 2019) and ROBO1 (Bates et al., 2011; Mascheretti et al., 2014; Tran et al., 2014; Luciano et al., 2018). From the final selection of phonology-related candidate genes (Supplementary Table 7D), the most significant genetic markers of each gene reported by the original study were extracted and summarized in Supplementary Table 7E.
All candidate genes were analyzed for statistical over-representation in GO using ToppFun and the original ancestor charts from GO (Methods section “Functional Enrichment Analysis”). Eleven GO terms showed significant enrichments of phonology-related gene sets that withstood a correction for multiple comparisons (Table 1). Total results of this analysis including terms that did not withstand correction are described in Supplementary Table 8. The most significant result of the enrichment analysis was the “Biological Process” term “response to auditory stimulus” from the topic learning, where we found an enrichment of CNTNAP2, FOXP2, and KIAA0319 out of 28 genes from this term (GO:0010996). In hierarchical relationships to this were the child terms “vocal learning” and “response to auditory behavior,” where the genes CNTNAP2 and FOXP2 were enriched. Within the top eleven results were three additional GO terms which support learning. They form a group of terms (cluster), where the neighboring cluster starts at the parent term “cognition” (GO:0050890; enriched genes CNTNAP2, CYFIP1, DCDC2, DNAAF4, FOXP2), went over “observational learning” (GO:0098597) and “imitative learning” (GO:0098596) and finally ended this GO term path in “vocal learning.”
The second and third most significant GO terms were “dendrite development” (GO:0016358), enriched by CYFIP1, DCDC2, KIAA0319, RBFOX2, and ROBO1, and “cellular component morphogenesis” (GO:0032989), enriched by CCDC136, CNTNAP2, CYFIP1, DCDC2, KIAA0319, RBFOX2, and ROBO1, both pointing to neuronal development as the second big topic of the enrichment analysis. This finding was complemented by the two terms from the GO category “Cellular Component” “neuron projection membrane” (GO:0032589) and “axolemma” (GO:0030673) and the “Biological Process” category term “cell morphogenesis involved in neuron differentiation” (GO:0048667). The only GO term from the “Molecular Function” category among our significant results was “magnesium ion transmembrane transporter activity” (GO:0015095).
In the main analysis, eighteen candidate genes showed similar expression levels in Broca’s and Wernicke’s regions (p > 0.05; Table 2). To allow an exploratory classification of these candidates in above-average and below-average expression, we calculated a mean value over all genes using their z-scores from Broca’s and Wernicke’s regions (x̄ = 0.125) and found twelve genes expressed above-average (overall mean > 0.125): ATP2C2, CMAHP, CMIP, CNTNAP2, CYFIP1, DCDC2, DNAAF4, FOXP2, NIPA2, RBFOX2, RIPOR2, and TUBGCP5, while DCDC2 showed the highest expression (overall mean = 0.573; Table 2). Three genes were differentially expressed between both regions: ATP2C2 showed a higher expression level in Broca’s region as compared to Wernicke’s region (p = 0.0291). DNAAF4 (p = 0.0001) and FOXP2 (p = 0.0006) showed higher expression levels in Wernicke’s region, both were stable against correction for multiple comparisons (pFWE < 0.05). The fine-mapped expression patterns of the three differentially expressed genes are shown in Figure 4.
Figure 4. 3D visualization of the main expression analysis using JuGEx in lateral views (top panels) and dorsal views (bottom panels) displaying the three significantly differentially expressed genes: DNAAF4 and FOXP2 show higher expression (FWE-corrected) in Wernicke’s region and ATP2C2 in Broca’s region. Investigated tissue samples are represented by spheres in the red areas (Broca’s region) and crosses in the blue areas (Wernicke’s region). RNA expression levels are indicated by z-scores showing ranges between maximal (green) and minimal values (pink).
Two types of follow-up analyses were performed. The regional specificity analysis in the frontal lobe found significantly higher expression levels in Broca’s region for CNTNAP2 (p = 0.001) and DCDC2 (p = 0.002), both pFWE < 0.05, compared with the language unspecific premotor area (areas 6d1-6d3; Supplementary Table 9A); in the temporal lobe, Wernicke’s region vs. fusiform gyrus (areas Fg1-Fg4) yielded no significant differential expression (p > 0.05; Supplementary Table 9B). The control analysis tested random and color genes, each, in Broca’s versus Wernicke’s regions. Neither of the two analyses demonstrated a differential expression stable against correction (both pFWE > 0.05; Supplementary Tables 9C,D).
Prior studies have been looking for links between risk genes and phonological aspects in individual disorders. Here we went beyond this point and addressed a more fundamental question, i.e., to identify cross-disease candidate phonological genes whose activity (expression level) we wanted to characterize in key regions of the language network using brain atlas-guided expression mapping. To achieve this, our approach comprised three steps. A systematic literature review that identified 21 phonology-related genes for developmental language and/or learning disorders. An expression analysis found that 12 of these genes were active in Broca’s and Wernicke’s regions, with two genes being more active in Wernicke and one gene being more active in Broca suggesting functional specialization. An enrichment analysis that found out that nine of these genes may contribute to processes of learning and neuronal development.
Our literature evaluation was designed on the assumption that phonological processing may have a common biological basis in the selected disorders, i.e., the “gene overlap hypothesis.” At the behavioral level, for example, impairments in working memory (Attout and Majerus, 2015; Gray et al., 2019; Johnson et al., 2020) and in learning ability (Rogalski et al., 2008; Bishop, 2009; Landerl et al., 2009) are evident in dyslexia, dyscalculia, SLI, and lvPPA, in addition to phonological impairment. These aspects are closely linked to phonology. However, since mainly linguistic variables of these disorders were investigated in the underlying studies, there is evidence that all genes can be assigned as phonology-related, although it cannot be assumed that this would be exclusively the case.
Because we based our candidate gene search on findings in cohorts of European ancestry, we had to ignore some potentially interesting candidates from the recent literature. One example was a GWAS of rapid automatized naming and rapid alternating stimulus conducted in Hispanic American and African American cohorts (Troung et al., 2019). Both parameters are considered as predictors for reading disability and may be relevant for investigating phonological processing. However, we could not consider the genes RPL7P34 and RNLS from this study. The reason is that genomic and transcriptomic variability (in normal and diseased individuals) may be influenced by population stratification, especially common variation is sensible to this, e.g., SNPs mapping to genes or influencing expression. To avoid this bias, we wanted to keep the data sources (study cohorts) as homogenous as possible.
The gene extractions from the consecutive computational and manual text mining were put forward to the overlap analysis (Figure 3 and Supplementary Table 7C) which revealed that the number of common phonology-related genes was 13 for dyslexia/SLI, six for dyslexia/dyscalculia, and two for dyslexia/dyscalculia/SLI, resulting in 21 genes in total (Supplementary Table 7D). Hence, three disorders contributed to this result, wherein dyslexia was connected to each phonology-related gene. The fourth language disorder, lvPPA, did not share any of its four genes (APOE, APP, GRN, MAPT) with the other disorders. LvPPA is a rare disease in the population and only a small number of putative risk genes are known yet (Gorno-Tempini et al., 2004, 2008). This is an important result and does not contradict our initial hypothesis. We consider it rather informative that the genuine developmental disorders with phonological core aspects cluster together and are somewhat distinct from a neurodegenerative disorder with acute phonological but developmentally more general learning deficits (Gorno-Tempini et al., 2008). We have examined lvPPA with the other disorders because there are two arguments from a clinical point of view: First, lvPPA shows clear phonological symptoms, which is why it belongs to the logopedic disorders. Second, Rogalski et al. (2008), for example, showed that patients with PPA are often affected by learning disorders earlier in life before the late-onset of PPA. The chance of a gene overlap with the more polygenic developmental disorders was therefore smaller. Overall, our evaluation supports the view of different molecular processes underlying lvPPA compared to the investigated developmental disorders.
Besides the fact that phonological processing is affected in developmental language and/or learning disorders, phonology is the motor for various language learning processes including writing (Treiman, 2017). To explore if phonology-related genes expressed in Broca’s and Wernicke’s regions acting together in the same Biological Processes, Molecular Functions, and Cellular Components, we performed an enrichment analysis using annotations of gene functions (GO terms). The overall results highlight relationships that can be summarized under the topics learning and neuronal development. Our findings may strengthen the links between phonology and behavioral and/or clinical aspects of the three investigated disorders.
Regarding learning, the most significant GO term was “response to auditory stimulus” referring to a process that results in a change in state or activity of a cell or an organism, e.g., gene expression, as a result of an auditory stimulus pointing to the aspect of hearing. In general, auditory processing forms the basis for phonological processing. In case of decreasing auditory performance caused by acquired hearing impairment, studies report a negative influence on phonological representations, for instance, a high working memory capacity was a compensating factor for negative impact of auditory deprivation on phonological processing abilities (Classon et al., 2013). Similar results were found for dyslexia and SLI. In fact, both disorders showed a substantial overlap concerning auditory processing and phonological skills (Fraser et al., 2010). For dyslexia, a link between hearing and reading abilities is known, i.e., changes in brain networks involved in phonological processing lead to deficits in matching speech sounds to their corresponding visual representations (Wallace, 2009).
Another finding of the topic learning provides a link between the term “vocal learning,” the transcription factor gene FOXP2, and one of its prominent transcriptional targets, the cell adhesion and receptor gene CNTNAP2, both contributing to all GO terms of the topic learning. Since the genes occur in five of eight outcomes, it can be concluded that both genes exert some dominance on result generation. A reason could be that there is plenty of experimental data on both in the literature and databases compared to other genes of our investigation. As FOXP2 is important in human language development and its disorders, its avian homolog FoxP2 is thought to play a crucial role in vocal learning and possible deficits in song-learning birds (Jarvis, 2019). CNTNAP2 was associated with neurodevelopmental disorders including language deficits (Vernes et al., 2008) and variation in language development in the general population (Whitehouse et al., 2011). Recent results suggested that CNTNAP2 is involved in a developmental cascade of effects in infants where it controls rapid auditory processing leading to early expressive language (Riva et al., 2018).
When comparing the two above-mentioned terms “response to auditory stimulus” and “vocal learning,” one gene (KIAA0319) only occurred in the term “response to auditory stimulus” and hence was probably the gene that distinguishes both findings. This observation may be interesting since a knock down of Kiaa0319 expression in a rat model altered cortical responses and sound processing in the auditory cortex which was taken as an analogy for phoneme processing in humans (Centanni et al., 2014). KIAA0319 encodes a cell adhesion molecule involved in development of the cerebral cortex. In fact, the gene mediates adhesion between migrating neurons and radial glial fibers as well as it regulates the morphology of dendrites (Peschansky et al., 2010). On the question of KIAA0319 being functionally related to FOXP2, there is little literature. In a fMRI study by Pinel et al. (2012) in a reading task in healthy subjects, they found evidence that common genetic variations (SNPs) in both genes may play an important role in language development and in normally developed language networks, but in different cerebral pathways: two FOXP2 SNPs were associated with variations of activation in the left frontal cortex, while one KIAA0319 SNP was associated with asymmetry in functional activation of the superior temporal sulcus.
The GO term “dendrite development” was the second most significant enrichment result and addresses the progression of dendrites, from its formation to a mature structure. Five of our candidate genes contributed to this term (CYFIP1, DCDC2, KIAA0319, RBFOX2, ROBO1). Dendritic extensions propagate electrochemical signals received from other neural cells to the cell body of the neuron. These issues are important constituents of learning and behavior, including synaptic plasticity, and therefore build a strong content-related connection to our first topic (learning). CYFIP1 coordinates the translation of messenger RNA at dendrites (Crespi, 2017). High expression of CYFIP1 due to gene duplication has been associated with autism (Oguro-Ando et al., 2015). Conversely, low expression by gene deletion was associated with risk of schizophrenia and impaired cognition among otherwise-neurotypical individuals (Stefansson et al., 2008, 2014; Tam et al., 2010). The deletion also affects cognitive, structural, and functional correlates of dyslexia as well as dyscalculia and mediates the strongest risk to the combined disorders (Ulfarsson et al., 2017). A GWAS found association between reading and language skills and SNP rs5995177 in RBFOX2 (Gialluisi et al., 2014). It encodes an alternative splicing regulator (RNA binding fox-1 homolog 2) involved in brain development (Gehman et al., 2012). A follow-up candidate gene study found a generalized effect of the SNP on cortical thickness in reading- and language-related brain regions of healthy adults (Gialluisi et al., 2017). The rodent homolog of ROBO1 (Robo1) encodes the roundabout guidance receptor 1 which is known for regulating the midline crossing of commissural nerve tracts, a process essential for mammalian brain formation. In family pedigrees from Finland, a rare ROBO1 haplotype was identified which dominantly co-segregates with the dyslexia diagnosis (Hannula-Jouppi et al., 2005). Individuals who carried this ROBO1 variant showed dose-dependent impaired interaural interaction suggesting that crossing of the auditory pathways requires an adequate ROBO1 expression during development (Lamminmäki et al., 2012). DCDC2 and KIAA0319 provide experimental evidence for a dual role in both topics. In neuronal development, DCDC2 (doublecortin domain-containing protein 2) is involved in regulating neuronal migration, especially for the cerebral cortex (Galaburda et al., 2006). In learning, DCDC2 was associated with phonological awareness and auditory phonological representations (Goswami, 2011; Eicher et al., 2015). KIAA0319 (neuronal migration) mediates axon guidance as well as dendrite formation. In this respect, DCDC2 and KIAA0319 are among the most studied genes in language and/or learning disorders and future studies of phonological processing that aggregate variation of all five genes seem to be worthwhile.
Expression differences of a gene in two language network regions are not direct evidence for a functional involvement of this gene in phonological processing. However, this arises from combining expression mapping and gene function associated with phonology determined in previous studies. The same is true for associations with disorders, because the expression data from the Allen Human Brain Atlas and mapping data from the Julich-Brain Atlas were collected in brains of people who had no history for neurological or psychiatric disease. Since the Allen expression data do not provide additional genotype data, we cannot directly examine the relationship between genome (genotype), transcriptome (RNA), and phenotype (disease or imaging trait like gray matter volume).
Regarding the main analysis, we found evidence for a significant differential expression of three phonology-related genes. In fact, expression of ATP2C2 was upregulated in Broca’s region, while expression of DNAAF4 and FOXP2 was upregulated in Wernicke’s region. The regional specification analyses for the frontal and the temporal lobes showed that almost all phonology-related genes, including our top findings, were not differentially expressed between Broca’s region and premotor region (6d1–6d3) in the frontal lobe, and between Wernicke’s region and fusiform gyrus (Fg1-Fg4) in the temporal lobe (Supplementary Tables 9A,B). The only follow-up analysis showing a significant and FWE correction-stable expression difference, was the frontal lobe comparison, revealing a higher expression of CNTNAP2 and DCDC2 in Broca suggesting their activity in this part of the language network. Because none of our candidate genes was significantly upregulated after correction in the non-language areas, we hypothesize that ATP2C2, DNAAF4, and FOXP2 were specifically expressed in cortical regions being language and/or phonology relevant. To further evaluate the biological specificity of these results, we performed two additional control analyses. Neither the genes of a random selection from the whole transcriptome nor the genes of human body coloration achieved a correction stable result. Both negative results, together with knowledge about the disorders, brain regions, and gene functions, supported the specificity of regional expressions of ATP2C2, DNAAF4, and FOXP2.
The literature analysis suggested an overlap between dyslexia and SLI for ATP2C2 (Newbury et al., 2011; Müller et al., 2017; Martinelli et al., 2021). In the enrichment analysis, it did not show up among our top findings but provided nominal significance in a gene set with ROBO1 for a non-brain related biological process (Supplementary Table 8). Different studies provided evidence that ATP2C2 is involved in phonological processing, for instance, regarding a modulation of phonological working memory in language impairment (Newbury et al., 2009; Smith et al., 2015). ATP2C2 encodes a magnesium-dependent calcium transporter. Regarding a translation of our ATP2C2 finding to a clinical level, it seems to be important that imbalances of magnesium and calcium ions in blood serum were reported in patients with dyslexia and other communication disorders (Kurup and Kurup, 2003).
For DNAAF4, the literature analysis provided a link between dyscalculia and dyslexia. In the enrichment analysis, the gene was part of the GO term “cognition” highlighting the topic learning. DNAAF4, formerly known as DYX1C1 (dyslexia susceptibility 1 candidate 1), was described as axonemal dynein assembly factor required for ciliary motility (Tarkar et al., 2013). More relevant for our study was a previous finding of a protein-protein interaction with estrogen receptors possibly linking its well-known role in neuronal migration during cortical development to an involvement of hormonal pathways in the development of dyslexia (Wang et al., 2006; Massinen et al., 2009). In this line, DNAAF4 was found to be associated with phonological memory in dyslexia (Lim et al., 2014) as well as with phonological working memory in healthy participants (Marino et al., 2007). Of note, knockdown of Dnaaf4 in rat embryos demonstrated effects on auditory processing, visual attention as well as cortical and thalamic anatomy (Szalkowski et al., 2013). In humans, two SNPs, rs17819126 in DNAAF4 and rs8053211 in ATP2C2, represented the only correction stable results in an association screen of ten candidate genes tagged by 23 independent SNPs for event-related potential mismatch response, an indicator for auditory discrimination capabilities in dyslexia patients (Müller et al., 2017). Müller et al. (2017) provided evidence that genetic variation in ATP2C2 and DNAAF4 may be a putative modulator of mismatch response in dyslexia. This aspect is highly interesting since auditory discrimination is an essential prerequisite for phonological processing and should be investigated in a future study, especially in context with disease-dependent changes of structural and functional connectivity between Broca’s and Wernicke’s regions.
FOXP2 is considered to be one of the most important genes for speech development and disorder. Its relevance was hypothesized when rare variation (a missense mutation) was discovered in members of a British family with frequent problems in articulating, formulating, and understanding of speech (Lai et al., 2001). Subsequently, common variation (SNPs) was investigated at this gene locus and associations between SNPs and dyslexia as well as other language and/or learning disorders were identified (Mascheretti et al., 2017). FOXP2 expression is relevant during brain development, when the transcription factor controls the transcriptional activity of different target genes, e.g., CNTNAP2 (Castro Martínez et al., 2019). Our literature evaluation provided a link between FOXP2 and dyslexia and SLI (Eicher et al., 2013; Doust et al., 2020). In our enrichment analysis, the gene contributed to the best findings of the topic learning. The significantly higher expression of FOXP2 in Wernicke’s region may fit to a finding of a study by Wilcke et al. (2012). The authors report that the dyslexia-associated SNP rs12533005 in FOXP2 was associated with grapheme-phoneme correspondence abilities (linking letters to speech sounds) in written language in an inferior parietal area near Wernicke’s region involved in phonological processing (Wilcke et al., 2012). We speculate whether the higher activity of FOXP2 in Wernicke’s region could support the concept of regional specificity. Currently, FOXP2 is mainly known as a gene linked to childhood apraxia of speech and speech motor planning deficits (Morgan et al., 2016). The possible link between FOXP2 and phonology could be a new aspect to investigate further in the future.
The search criteria (“clinical categories”) of the literature evaluation were rather broad to cover the terminological variability between published studies of the four different disorders as much as possible. Consequently, we had to make the compromise of obtaining results at the genomic level that contained broader findings in addition to the desired disorders, e.g., genes for arithmetical weakness in case of dyscalculia. Basically, we only extracted markers and genes from a study that were self-reported as best findings by the study authors. We specified p < 0.05 for criterion (c) as a general threshold for significance; for GWAS, we tried to stick to genome-wide significant findings (p < 5 × 10–8) if reported. Thus, we always stayed within the logic of the respective study and did not make an arbitrary decision for or against a selection. This is the reason why we did not consider negative findings in our study. Many phonology-related genes have been selected from traditional candidate gene studies rather than large studies such as GWAS. The main reason is that we studied four disorders for which the literature is very diverse. Some disorder phenotypes already have GWAS, while others do not yet. Therefore, we did not prioritize study types in the design (GWAS, candidate gene study, linkage study). This may be a limitation, but in practice it was not feasible otherwise. Therefore, our gene selection may be interpreted with caution. To minimize the risk of unrecognized studies and genes, we performed a supporting search in the GWAS Catalog database for common genetic variants and their mapped genes across the four disorders during the period of the primary literature search (2010–2021). Despite thorough mining, we could not prevent the selection of gene markers with weak replication evidence in independent studies, e.g., RBFOX2. Again, we urge caution in interpreting the results. Ultimately, only meta-analyses will help to separate the robust associations from the false positives.
The assumption of the enrichment analysis was that the phonology-related genes we have yet to find will be consistent with what is already known about phonological processing and/or its genetic basis which may not always be true. The enrichment analysis can only be as accurate as the underlying annotations (GO categories) since not all genes, especially in humans, have phenotype annotations and there are many genes whose functions are not defined yet. As a result of these limitations, enrichments may arise in GO terms where certain genes show noticeable dominance that may bias interpretation, e.g., CNTNAP2 and FOXP2 in the terms of the topic “learning.” This is a caveat to keep in mind when interpreting results.
In the expression analysis, we focused on Broca’s and Wernicke’s regions. This does not mean that we ignore the broader network including the subcortical regions from which we did not include basal ganglia and striatum because these structures are associated with speech motor processes rather than phonology (Eickhoff et al., 2009). A similar reason applies for the cerebellum which is mainly responsible for speech motor control (Houde and Nagarajan, 2011). Because of the left hemispheric dominance for language for left- and right-handed people (Branch et al., 1964; Mazoyer et al., 2014), and the fact that there is more data for the left hemisphere in the Allen Human Brain Atlas expression data, only data from the left hemisphere were analyzed. Although the exclusive use of left hemispheric data is based on a strong biological assumption, this should still be considered as a possible limitation and taken into account when interpreting the data. Areas 44 and 45 were combined into one single VOI, considering the spatial constraints of availability of gene expression data, which did not allow searching in a more detailed way. At the same time, differences between both areas at the level of cyto- and receptorarchitecture (Amunts et al., 2010) as well as connectivity and function (Anwander et al., 2007; Friederici and Gierhan, 2013) have been described in the past, and the functional specialization of both areas undergoes changes during ontogeny until it is finally differentiated (Amunts et al., 2003; Skeide and Friederici, 2016). Therefore, it can be hypothesized that both areas may also differentially contribute to the findings of the present study. In addition, it cannot be excluded that the detected expression differences between Broca’s and Wernicke’s regions were influenced, to a certain degree, by differences in their normal cell type proportions of the bulk tissue used by Allen Brain. Further studies will be necessary to more precisely elucidate the role of the different cortical areas underlying Broca’s and Wernicke’s regions. The strength of the dataset used is the dense spatial coverage of the tissue samples whose gene expressions were analyzed. Together with the probability-based location information contained in the Julich-Brain maps, a meaningful differential gene expression analysis can be calculated. However, the relatively small number of six donors is also a statistical limitation that would suggest further molecular genetic analyses.
In the present study, we gathered previously published evidence for genetic factors underlying phonological processing symptoms of the language and learning disorders dyslexia, dyscalculia, and SLI and performed expression fine-mapping in two key regions of the language network. While most previous studies sought to link candidate genes and phonological aspects of a single disorder, we focused on a more general aim, to understand whether a symptom, i.e., phonological processing, may have a common genetic regulation in different disorders. Overall, the identified regional expression of ATP2C2, DNAAF4, and FOXP2 together with markers from the literature provide evidence of a putative role not only in impaired phonological processing but also for the healthy subjects. In this regard, our study may be a use-case for larger studies on the genetics of phonological processing in the future. To investigate new hypotheses for such gene-phenotype correlates in vivo, one option would be to test for association between DNA variation (SNPs, copy-number variants) at candidate gene loci and effects on phonological processing in cortical areas like Broca’s and Wernicke’s regions, and also in subcortical areas of the language network using large samples of the three disorders and beyond at different ages.
Data from Allen Human Brain Atlas are available at http://human.brain-map.org/ and data from Julich-Brain are available at https://www.jubrain.fz-juelich.de. A stand-alone version of JuGEx is available at http://www.fz-juelich.de/inm/inm-1/jugex. JuGEx is also part of the Atlas of the Human Brain Project, available at https://www.humanbrainproject.eu/en/explore-the-brain/atlases/.
The study computationally analyzed data from the Allen Human Brain Atlas and Julich-Brain Atlas. For Allen Brain: Brain tissues were collected after obtaining informed consent from the decedent’s next-of-kin. Institutional Review Board review and approval were obtained for the collection of tissue and non-identifying case information at the tissue banks and repositories that provided tissue for this project (http://help.brain-map.org/display/humanbrain/Documentation). For Julich-Brain: The brains were obtained through the body donor program of the Department of Anatomy at the University of Düsseldorf in accordance with the rules of the local ethics committee (#4863). The patients/participants’ next of kind provided their written informed consent to participate in this study.
NU, DIH, and TWM conducted analyses. NU, SH, KA, and TWM analyzed and interpreted the results. SB supported the JuGEx analyses. PP provided digital versions of the cytoarchitectonic probability maps. SH, SC, KA, and TWM supervised all aspects of the study. NU wrote a first draft of the manuscript. All authors critically revised the manuscript and approved its publication.
This research was supported by the European Union’s Horizon 2020 Research and Innovation Program [Specific Grant Agreement No. 785907 (Human Brain Project SGA2) and Specific Grant Agreement No. 945539 (Human Brain Project SGA3) to SC and KA] as well as by the Swiss National Science Foundation (SNSF, grant 156791 to SC).
The authors declare that the research was conducted in the absence of any commercial or financial relationships that could be construed as a potential conflict of interest.
All claims expressed in this article are solely those of the authors and do not necessarily represent those of their affiliated organizations, or those of the publisher, the editors and the reviewers. Any product that may be evaluated in this article, or claim that may be made by its manufacturer, is not guaranteed or endorsed by the publisher.
The Supplementary Material for this article can be found online at: https://www.frontiersin.org/articles/10.3389/fnins.2021.680762/full#supplementary-material
Supplementary Table 1 | Overview of phonological deficits in the four investigated disorders.
Supplementary Table 2 | Overview of studies on Broca’s and Wernicke’s regions in humans and model organisms.
Supplementary Table 3 | Literature evaluation—The eleven single searches using PubMed Advanced Search.
Supplementary Table 4 | Literature evaluation—PubMed Advanced Search combining clinical categories and genetic terms for the identification of phonology-related genes.
Supplementary Table 5 | Literature evaluation—Clinical categories found in research articles.
Supplementary Table 6A | Gene expression analysis—Random genes used as negative control.
Supplementary Table 6B | Gene expression analysis—Color genes used as negative control.
Supplementary Table 7A | Literature evaluation—The 36 excluded review articles.
Supplementary Table 7B | Literature evaluation—The 71 research articles providing the 77 candidate genes.
Supplementary Table 7C | Literature evaluation—The 77 candidate genes from the remaining 71 research articles.
Supplementary Table 7D | Literature evaluation—Basic annotation of the 21 phonology-related genes.
Supplementary Table 7E | Literature evaluation—The genetic items extracted from the 21 phonology-related genes.
Supplementary Table 8 | Total results of the functional enrichment analysis of phonology-related genes.
Supplementary Table 9A | Follow-up gene expression analysis in the frontal lobe—Phonology-related genes—Broca’s region vs. premotor areas.
Supplementary Table 9B | Follow-up gene expression analysis in the temporal lobe—Phonology-related genes—Wernicke’s region vs. fusiform gyrus.
Supplementary Table 9C | Follow-up gene expression analysis—Random genes—Broca’s region vs. Wernicke’s region.
Supplementary Table 9D | Follow-up gene expression analysis—Color genes—Broca’s region vs. Wernicke’s region.
Aboitiz, F., Aboitiz, S., and García, R. R. (2010). The phonological loop: a key innovation in human evolution. Curr. Anthropol. 51, S55–S65. doi: 10.1086/650525
Adams, A. K., Smith, S. D., Truong, D. T., Willcutt, E. G., Olson, R. K., DeFries, J. C., et al. (2017). Enrichment of putatively damaging rare variants in the DYX2 locus and the reading-related genes CCDC136 and FLNC. Hum. Genet. 136, 1395–1405. doi: 10.1007/s00439-017-1838-z
Allen Human Brain Atlas (2013). Case Qualification and Donor Profiles. Technical White Paper. v7. 2013. Seattle, WA: Allen Human Brain Atlas.
American Psychiatric Association (1994). Diagnostic and Statistical Manual of Mental Disorders: (DSM-IV), 4th Edn. Washington, DC: American Psychiatric Publishing.
American Psychiatric Association (2013). Diagnostic and Statistical Manual of Mental Disorders: (DSM-5), 5th Edn. Washington, DC: American Psychiatric Publishing.
Amunts, K., Lenzen, M., Friederici, A. D., Schleicher, A., Morosan, P., Palomero-Gallagher, N., et al. (2010). Broca’s region: novel organizational principles and multiple receptor mapping. PLoS Biol. 8:e1000489. doi: 10.1371/journal.pbio.1000489
Amunts, K., Mohlberg, H., Bludau, S., and Zilles, K. (2020). Julich-brain: a 3D probabilistic atlas of human brain’s cytoarchitecture. Science 369, 988–992. doi: 10.1126/science.abb4588
Amunts, K., Schleicher, A., and Zilles, K. (2004). Outstanding language competence and cytoarchitecture in Broca’s speech region. Brain Lang. 89, 346–353. doi: 10.1016/S0093-934X(03)00360-2
Amunts, K., Schleicher, A., Bürgel, U., Mohlberg, H., Uylings, H. B. M., Zilles, K., et al. (1999). Broca’s region revisited: cytoarchitecture and intersubject variability. J. Comp. Neurol. 412, 319–341. doi: 10.1002/(SICI)1096-9861(19990920)412:2<319::AID-CNE10<3.0.CO;2-7
Amunts, K., Schleicher, A., Ditterich, A., and Zilles, K. (2003). Broca’s region: cytoarchitectonic asymmetry and developmental changes. J. Comp. Neurol. 465, 72–89. doi: 10.1002/cne.10829
Anwander, A., Tittgemeyer, M., von Cramon, D. Y., Friederici, A. D., and Knösche, T. R. (2007). Connectivity-based parcellation of Broca’s area. Cereb. Cortex 17, 816–825. doi: 10.1093/cercor/bhk034
Attout, L., and Majerus, S. (2015). Working memory deficits in developmental dyscalculia: the importance of serial order. Child Neuropsychol. 21, 432–450. doi: 10.1080/09297049.2014.922170
Bates, T. C., Lind, P. A., Luciano, M., Montgomery, G. W., Martin, N. G., and Wright, M. J. (2010). Dyslexia and DYX1C1: deficits in reading and spelling associated with a missense mutation. Mol. Psychiatry 15, 1190–1196. doi: 10.1038/mp.2009.120
Bates, T. C., Luciano, M., Medland, S. E., Montgomery, G. W., Wright, M. J., and Martin, N. G. (2011). Genetic variance in a component of the language acquisition device: ROBO1 polymorphisms associated with phonological buffer deficits. Behav. Genet. 41, 50–57. doi: 10.1007/s10519-010-9402-9
Berthier, M. L., Lambon Ralph, M. A., Pujol, J., and Green, C. (2012). Arcuate fasciculus variability and repetition: the left sometimes can be right. Cortex 48, 133–143. doi: 10.1016/j.cortex.2011.06.014
Binder, J. R. (2017). Current controversies on Wernicke’s area and its role in language. Curr. Neurol. Neurosci. 17:58. doi: 10.1007/s11910-017-0764-8
Bishop, D. V. M. (2009). Specific language impairment as a language learning disability. Child Lang. Teach. Ther. 25, 163–165. doi: 10.1177/0265659009105889
Bludau, S., Mühleisen, T. W., Eickhoff, S. B., Hawrylycz, M. J., Cichon, S., and Amunts, K. (2018). Integration of transcriptomic and cytoarchitectonic data implicates a role for MAOA and TAC1 in the limbic-cortical network. Brain Struct. Funct. 223, 2335–2342. doi: 10.1007/s00429-018-1620-6
Branch, C., Milner, B., and Rasmussen, T. (1964). Intracarotid sodium Amytal for the lateralization of cerebral speech dominance: observations in 123 patients. Neurosurgery 21, 399–405. doi: 10.3171/jns.1964.21.5.0399
Buchsbaum, B. R., Hickok, G., and Humphries, C. (2001). Role of left posterior superior temporal gyrus in phonological processing for speech perception and production. Cogn. Sci. 25, 663–678. doi: 10.1207/s15516709cog2505_2
Carrion-Castillo, A., Maassen, B., Franke, B., Heister, A., Naber, M., Van Der Leij, A., et al. (2017). Association analysis of dyslexia candidate genes in a Dutch longitudinal sample. Eur. J. Hum. Genet. 25, 452–460. doi: 10.1038/ejhg.2016.194
Castro Martínez, X. H., Moltó Ruiz, M. D., Morales Marin, M. E., Flores Lázaro, J. C., González Fernández, J., Gutiérrez Najera, N. A., et al. (2019). FOXP2 and language alterations in psychiatric pathology. Salud Mental 42, 297–308. doi: 10.17711/sm.0185-3325.2019.039
Catani, M., Jones, D. K., and Ffytche, D. H. (2005). Perisylvian language networks of the human brain. Ann. Neurol. 57, 8–16. doi: 10.1002/ana.20319
Centanni, T. M., Booker, A. B., Sloan, A. M., Chen, F., Maher, B. J., Carraway, R. S., et al. (2014). Knockdown of the dyslexia-associated gene Kiaa0319 impairs temporal responses to speech stimuli in rat primary auditory cortex. Cereb. Cortex 24, 1753–1766. doi: 10.1093/cercor/bht028
Centanni, T. M., Pantazis, D., Truong, D. T., Gruen, J. R., Gabrieli, J. D. E., and Hogan, T. P. (2018). Increased variability of stimulus-driven cortical responses is associated with genetic variability in children with and without dyslexia. Dev. Cogn. Neurosci. 34, 7–17. doi: 10.1016/j.dcn.2018.05.008
Chen, J., Bardes, E. E., Aronow, B. J., and Jegga, A. G. (2009). ToppGene suite for gene list enrichment analysis and candidate gene prioritization. Nucleic Acids Res. 37, W305–W311. doi: 10.1093/nar/gkp427
Child, A. E., Cirino, P. T., Fletcher, J. M., Willcutt, E. G., and Fuchs, L. S. (2019). A cognitive dimensional approach to understanding shared and unique contributions to reading, math, and attention skills. J. Learn. Disabil. 52, 15–30. doi: 10.1177/0022219418775115
Cicchini, G. M., Marino, C., Mascheretti, S., Perani, D., and Morrone, M. C. (2015). Strong motion deficits in dyslexia associated with DCDC2 gene alteration. J.Neurosci. 35, 8059–8064. doi: 10.1523/JNEUROSCI.5077-14.2015
Claessen, M., and Leitão, S. (2012). Phonological representations in children with SLI. Child Lang. Teach. Ther. 28, 211–223. doi: 10.1177/0265659012436851
Classon, E., Rudner, M., and Rönnberg, J. (2013). Working memory compensates for hearing related phonological processing deficit. J. Commun. Disord. 46, 17–29. doi: 10.1016/j.jcomdis.2012.10.001
Cope, N., Eicher, J. D., Meng, H., Gibson, C. J., Hager, K., Lacadie, C., et al. (2012). Variants in the DYX2 locus are associated with altered brain activation in reading-related brain regions in subjects with reading disability. NeuroImage 63, 148–156. doi: 10.1016/j.neuroimage.2012.06.037
Couto, J. M., Livne-Bar, I., Huang, K., Xu, Z., Cate-Carter, T., Feng, Y., et al. (2010). Association of reading disabilities with regions marked by acetylated H3 histones in KIAA0319. Am. J. Med. Genet. 153, 447–462. doi: 10.1002/ajmg.b
Crespi, B. J. (2017). “Autism as a disorder of high intelligence,” in Autism Spectrum Disorders (ASD) – Searching for the Biological Basis for Behavioral Symptoms and New Therapeutic Targets, eds B. Gesundheit, J. Rosenzweig, and Y. Shoenfeld (Lausanne: Frontiers Media), 68–94. doi: 10.3389/978-2-88945-112-8
Czamara, D., Bruder, J., Becker, J., Bartling, J., Hoffmann, P., Ludwig, K. U., et al. (2011). Association of a rare variant with mismatch negativity in a region between KIAA0319 and DCDC2 in dyslexia. Behav. Genet. 41, 110–119. doi: 10.1007/s10519-010-9413-6
De Carvalho, C. A. F., Kida, A. D. S. B., Capellini, S. A., and de Avila, C. R. B. (2014). Phonological working memory and reading in students with dyslexia. Front. Psychol. 5:746. doi: 10.3389/fpsyg.2014.00746
De Smedt, B. (2018). “Language and arithmetic: the potential role of phonological processing,” in Heterogeneity of Function in Numerical Cognition, eds A. Henik and W. Fias (San Diego, CA: Academic Press), 51–74. doi: 10.1016/C2016-0-00729-5
De Smedt, B., and Boets, B. (2010). Phonological processing and arithmetic fact retrieval: evidence from developmental dyslexia. Neuropsychologia 48, 3973–3981. doi: 10.1016/j.neuropsychologia.2010.10.018
Dehaene, S. (1992). Varieties of numerical abilities. Cognition 44, 1–42. doi: 10.1016/0010-0277(92)90049-n
Dehaene, S., Piazza, M., Pinel, P., and Cohen, L. (2003). Three parietal circuits for number processing. Cogn. Neuropsychol. 20, 487–506. doi: 10.1080/02643290244000239
DeWitt, I., and Rauschecker, J. P. (2013). Wernicke’s area revisited: parallel streams and word processing. Brain Lang. 127, 181–191. doi: 10.1016/j.bandl.2013.09.014
Dinkel, P. J., Willmes, K., Krinzinger, H., Konrad, K., and Koten, J. W. (2013). Diagnosing developmental dyscalculia on the basis of reliable single case FMRI methods: promises and limitations. PLoS One 8:e83722. doi: 10.1371/journal.pone.0083722
Doust, C., Gordon, S. D., Garden, N., Fisher, S. E., Martin, N. G., Bates, T. C., et al. (2020). The association of dyslexia and developmental speech and language disorder candidate genes with reading and language abilities in adults. Twin Res. Hum. Genet. 23, 23–32. doi: 10.1017/thg.2020.7
Eicher, J. D., Powers, N. R., Miller, L. L., Akshoomoff, N., Amaral, D. G., Bloss, C. S., et al. (2013). Genome-wide association study of shared components of reading disability and language impairment. Genes Brain Behav. 12, 792–801. doi: 10.1111/gbb.12085
Eicher, J. D., Powers, N. R., Miller, L. L., Mueller, K. L., Mascheretti, S., Marino, C., et al. (2014). Characterization of the DYX2 locus on chromosome 6p22 with reading disability, language impairment, and IQ. Hum. Genet. 133, 869–881. doi: 10.1007/s00439-014-1427-3
Eicher, J. D., Stein, C. M., Deng, F., Ciesla, A. A., Powers, N. R., Boada, R., et al. (2015). The DYX2 locus and neurochemical signaling genes contribute to speech sound disorder and related neurocognitive domains. Genes Brain Behav. 14, 377–385. doi: 10.1111/gbb.12214
Eickhoff, S. B., Heim, S., Zilles, K., and Amunts, K. (2009). A systems perspective on the effective connectivity of overt speech production. Philos. Trans. A Math. Phys. Eng. Sci. 367, 2399–2421. doi: 10.1098/rsta.2008.0287
Elbert, A., Lovett, M. W., Cate-Carter, T., Pitch, A., Kerr, E. N., and Barr, C. L. (2011). Genetic variation in the KIAA0319 5’ region as a possible contributor to dyslexia. Behav. Genet. 41, 77–89. doi: 10.1007/s10519-010-9434-1
Evans, A. C., Janke, A. L., Collins, D. L., and Baillet, S. (2012). Brain templates and atlases. NeuroImage 62, 911–922. doi: 10.1016/j.neuroimage.2012.01.024
Fraser, J., Goswami, U., and Conti-Ramsden, G. (2010). Dyslexia and specific language impairment: the role of phonology and auditory processing. Sci. Stud. Read. 14, 8–29. doi: 10.1080/10888430903242068
Friederici, A. D. (2009). Pathways to language: fibre tracts in the human brain. Trends Cogn. Sci. 13, 175–181. doi: 10.1016/j.tics.2009.01.001
Friederici, A. D. (2011). The brain basis of language processing: from structure to function. Physiol. Rev. 91, 1357–1392. doi: 10.1152/physrev.00006.2011
Friederici, A. D. (2012). Language development and the ontogeny of the dorsal pathway. Front. Evol. Neurosci. 4:3. doi: 10.3389/fnevo.2012.00003
Friederici, A. D., and Gierhan, S. M. E. (2013). The language network. Curr. Opin. Neurobiol. 23, 250–254. doi: 10.1016/j.conb.2012.10.002
Galaburda, A. M., LoTurco, J., Ramus, F., Fitch, R. H., and Rosen, G. D. (2006). From genes to behavior in developmental dyslexia. Nat. Neurosci. 9, 1213–1217. doi: 10.1038/nn1772
Gehman, L. T., Meera, P., Stoilov, P., Shiue, L., O’Brien, J. E., Meisler, M. H., et al. (2012). The splicing regulator Rbfox2 is required for both cerebellar development and mature motor function. Genes Dev. 26, 445–460. doi: 10.1101/gad.182477.111
Gene Ontology Consortium (2021). The gene ontology resource: enriching a gold mine. Nucleic Acids Res. 49, D325–D334. doi: 10.1093/nar/gkaa1113
Gialluisi, A., Guadalupe, T., Francks, C., and Fisher, S. E. (2017). Neuroimaging genetic analyses of novel candidate genes associated with reading and language. Brain Lang. 172, 9–15. doi: 10.1016/j.bandl.2016.07.002
Gialluisi, A., Newbury, D. F., Wilcutt, E. G., Olson, R. K., DeFries, J. C., Brandler, W. M., et al. (2014). Genome-wide screening for DNA variants associated with reading and language traits. Genes Brain Behav. 13, 686–701. doi: 10.1111/gbb.12158
Gori, S., Mascheretti, S., Giora, E., Ronconi, L., Ruffino, M., Quadrelli, E., et al. (2015). The DCDC2 intron 2 deletion impairs illusory motion perception unveiling the selective role of magnocellular-dorsal stream in reading (dis)ability. Cereb. Cortex 25, 1685–1695. doi: 10.1093/cercor/bhu234
Gorno-Tempini, M. L., Brambati, S. M., Ginex, V., Ogar, B. J., Dronkers, N. F., Marcone, A., et al. (2008). The logopenic/phonological variant of primary progressive aphasia. Neurology 71, 1227–1234. doi: 10.1212/01.wnl.0000320506.79811.da
Gorno-Tempini, M. L., Dronkers, N. F., Rankin, K. P., Ogar, J. M., Phengrasamy, L., Rosen, H. J., et al. (2004). Cognition and anatomy in three variants of primary progressive aphasia. Ann. Neurol. 55, 335–346. doi: 10.1002/ana.10825
Gorno-Tempini, M. L., Hillis, A. E., Weintraub, S., Kertesz, A., Mendez, M., Cappa, S. F., et al. (2011). Classification of primary progressive aphasia and its variants. Neurology 76, 1006–1014. doi: 10.1212/WNL.0b013e31821103e6
Goswami, U. (2011). A temporal sampling framework for developmental dyslexia. Trends Cogn Sci. 15, 3–10. doi: 10.1016/j.tics.2010.10.001
Gray, S., Fox, A. B., Green, S., Alt, M., Hogan, T. P., Petscher, Y., et al. (2019). Working memory profiles of children with dyslexia, developmental language disorder, or both. J. Speech Lang. Hear. Res. 62, 1839–1858. doi: 10.1044/2019_JSLHR-L-18-0148
Hagoort, P., and Indefrey, P. (2014). The neurobiology of language beyond single words. Annu. Rev. Neurosci. 37, 347–362. doi: 10.1146/annurev-neuro-071013-013847
Hannula-Jouppi, K., Kaminen-Ahola, N., Taipale, M., Eklund, R., Nopola-Hemmi, J., Kääriäinen, H., et al. (2005). The axon guidance receptor gene ROBO1 is a candidate gene for developmental dyslexia. PLoS Genet. 1:e50. doi: 10.1371/journal.pgen.0010050
Hartwigsen, G. (2015). The neurophysiology of language: insights from non-invasive brain stimulation in the healthy human brain. Brain Lang. 148, 81–94. doi: 10.1016/j.bandl.2014.10.007
Hartwigsen, G. (2016). Adaptive plasticity in the healthy language network: implications for language recovery after stroke. Neural Plast. 2016:9674790. doi: 10.1155/2016/9674790
Hartwigsen, G., Stockert, A., Charpentier, L., Wawrzyniak, M., Klingbeil, J., Wrede, K., et al. (2020). Short-term modulation of the lesioned language network. Elife 9:e54277. doi: 10.7554/eLife.54277
Hasselhorn, M., Schuchardt, K., and Mähler, C. (2010). Phonological working memory in children with specific learning disorders in reading and/or spelling: the effect of word length and lexicality on memory span. Z. Entwicklungspsychol. Padagog. Psychol. 42, 211–216. doi: 10.1026/0049-8637/a000024
Hawrylycz, M. J., Lein, E. S., Guillozet-Bongaarts, A. L., Shen, E. H., Ng, L., Miller, J. A., et al. (2012). An anatomically comprehensive atlas of the adult human brain transcriptome. Nature 489, 391–399. doi: 10.1038/nature11405
Hawrylycz, M., Miller, J. A., Menon, V., Feng, D., Dolbeare, T., Guillozet-Bongaarts, A. L., et al. (2015). Canonical genetic signatures of the adult human brain. Nat. Neurosci. 18, 1832–1844. doi: 10.1038/nn.4171
Heim, S., Eickhoff, S. B., and Amunts, K. (2008). Specialisation in Broca’s region for semantic, phonological, and syntactic fluency? NeuroImage 40, 1362–1368. doi: 10.1016/j.neuroimage.2008.01.009
Heim, S., Eickhoff, S. B., and Amunts, K. (2009a). Different roles of cytoarchitectonic BA 44 and BA 45 in phonological and semantic verbal fluency as revealed by dynamic causal modelling. NeuroImage 48, 616–624. doi: 10.1016/j.neuroimage.2009.06.044
Heim, S., Eickhoff, S. B., Ischebeck, A. K., Friederici, A. D., Stephan, K. E., and Amunts, K. (2009b). Effective connectivity of the left BA 44, BA 45, and inferior temporal gyrus during lexical and phonological decisions identified with DCM. Hum. Brain Map. 30, 392–402. doi: 10.1002/hbm.20512
Heim, S., Grande, M., Meffert, E., Eickhoff, S. B., Schreiber, H., Kukolja, J., et al. (2010). Cognitive levels of performance account for hemispheric lateralisation effects in dyslexic and normally reading children. NeuroImage 53, 1346–1358. doi: 10.1016/j.neuroimage.2010.07.009
Heim, S., Opitz, B., Müller, K., and Friederici, A. D. (2003). Phonological processing during language production: fMRI evidence for a shared production-comprehension network. Brain Res. Cogn. Brain Res. 16, 285–296. doi: 10.1016/s0926-6410(02)00284-7
Henry, M. L., and Gorno-Tempini, M.-L. (2010). The logopenic variant of primary progressive aphasia. Curr. Opin. Neurol. 23, 633–637. doi: 10.1097/WCO.0b013e32833fb93e
Henry, M. L., Wilson, S. M., Babiak, M. C., Mandelli, M. L., Beeson, P. M., Miller, Z. A., et al. (2016). Phonological processing in primary progressive aphasia. J. Cogn. Neurosci. 28, 210–222. doi: 10.1162/jocn_a_00901
Hensler, B. S., Schatschneider, C., Taylor, J., and Wagner, R. K. (2010). Behavioral genetic approach to the study of dyslexia. J. Dev. Behav. Pediatr. 31, 525–532. doi: 10.1097/DBP.0b013e3181ee4b70
Houde, J. F., and Nagarajan, S. S. (2011). Speech production as state feedback control. Front. Hum. Neurosci. 5:82. doi: 10.3389/fnhum.2011.00082
Indefrey, P. (2011). The spatial and temporal signatures of word production components: a critical update. Front. Psychol. 2:255. doi: 10.3389/fpsyg.2011.00255
Indefrey, P., and Levelt, W. J. M. (2004). The spatial and temporal signatures of word production components. Cognition 92, 101–144. doi: 10.1016/j.cognition.2002.06.001
Jarvis, E. D. (2019). Evolution of vocal learning and spoken language. Science 366, 50–54. doi: 10.1126/science.aax0287
Johnson, J. C. S., Jiang, J., Bond, R. L., Benhamou, E., Requena-Komuro, M. C., Russell, L. L., et al. (2020). Impaired phonemic discrimination in logopenic variant primary progressive aphasia. Ann. Clin. Transl. Neurol. 7, 1252–1257. doi: 10.1002/acn3.51101
Johnson, M. B., Kawasawa, Y. I, Mason, C. E., Krsnik, Ž, Coppola, G., Bogdanović, D., et al. (2009). Functional and evolutionary insights into human brain development through global transcriptome analysis. Neuron 62, 494–509. doi: 10.1016/j.neuron.2009.03.027
Katzev, M., Tüscher, O., Hennig, J., Weiller, C., and Kaller, C. P. (2013). Revisiting the functional specialization of left inferior frontal gyrus in phonological and semantic fluency: the crucial role of task demands and individual ability. J. Neurosci. 33, 7837–7845. doi: 10.1523/JNEUROSCI.3147-12.2013
Kim, G., Ahmadian, S. S., Peterson, M., Parton, Z., Memon, R., Weintraub, S., et al. (2016). Asymmetric pathology in primary progressive aphasia with progranulin mutations and TDP inclusions. Neurology 86, 627–636. doi: 10.1212/WNL.0000000000002375
Klaus, J., and Hartwigsen, G. (2019). Dissociating semantic and phonological contributions of the left inferior frontal gyrus to language production. Hum. Brain Map. 40, 3279–3287. doi: 10.1002/hbm.24597
König, I. R., Schumacher, J., Hoffmann, P., Kleensang, A., Ludwig, K. U., Grimm, T., et al. (2011). Mapping for dyslexia and related cognitive trait loci provides strong evidence for further risk genes on chromosome 6p21. Am. J. Med. Genet. B Neuropsychiatr. Genet. 156, 36–43. doi: 10.1002/ajmg.b.31135
Kurup, R. K., and Kurup, P. A. (2003). Hypothalamic digoxin and hemispheric chemical dominance: relation to speech and language dysfunction. Int. J. Neurosci. 113, 797–814. doi: 10.1080/00207450390200936
Lai, C. S. L., Fisher, S. E., Hurst, J. A., Vargha-Khadem, F., and Monaco, A. P. (2001). A forkhead-domain gene is mutated in a severe speech and language disorder. Nature 413, 519–523. doi: 10.1038/35097076
Lambert, N., Lambot, M. A., Bilheu, A., Albert, V., Englert, Y., Libert, F., et al. (2011). Genes expressed in specific areas of the human fetal cerebral cortex display distinct patterns of evolution. PLoS One 6:e17753. doi: 10.1371/journal.pone.0017753
Lamminmäki, S., Massinen, S., Nopola-Hemmi, J., Kere, J., and Hari, R. (2012). Human ROBO1 regulates interaural interaction in auditory pathways. J. Neurosci. 32, 966–971. doi: 10.1523/JNEUROSCI.4007-11.2012
Landerl, K., Fussenegger, B., Moll, K., and Willburger, E. (2009). Dyslexia and dyscalculia: two learning disorders with different cognitive profiles. J. Exp. Child Psychol. 103, 309–324. doi: 10.1016/j.jecp.2009.03.006
Lewis, B. A., Avrich, A. A., Freebairn, L. A., Taylor, H. G., Iyengar, S. K., and Stein, C. M. (2011). Subtyping children with speech sound disorders by endophenotypes. Top. Lang. Disord. 31, 112–127. doi: 10.1097/TLD.0b013e318217b5dd
Liakakis, G., Nickel, J., and Seitz, R. J. (2011). Diversity of the inferior frontal gyrus – a meta-analysis of neuroimaging studies. Behav. Brain Res. 225, 341–347. doi: 10.1016/j.bbr.2011.06.022
Lim, C. K. P., Wong, A. M. B., Ho, C. S. H., and Waye, M. M. Y. (2014). A common haplotype of KIAA0319 contributes to the phonological awareness skill in Chinese children. Behav. Brain Funct. 10:23. doi: 10.1186/1744-9081-10-23
Lind, P. A., Luciano, M., Wright, M. J., Montgomery, G. W., Martin, N. G., and Bates, T. C. (2010). Dyslexia and DCDC2: normal variation in reading and spelling is associated with DCDC2 polymorphisms in an Australian population sample. Eur. J. Hum. Genet. 18, 668–673. doi: 10.1038/ejhg.2009.237
López-Barroso, D., Catani, M., Ripollés, P., Dell’Acqua, F., Rodríguez-Fornells, A., and de Diego-Balaguer, R. (2013). Word learning is mediated by the left arcuate fasciculus. Proc. Natl. Acad. Sci. U.S.A. 110, 13168–13173. doi: 10.1073/pnas.1301696110
Luciano, M., Gow, A. J., Pattie, A., Bates, T. C., and Deary, I. J. (2018). The influence of dyslexia candidate genes on reading skill in old age. Behav. Genet. 48, 351–360. doi: 10.1007/s10519-018-9913-3
Ludwig, K. U., Sämann, P., Alexander, M., Becker, J., Bruder, J., Moll, K., et al. (2013). A common variant in Myosin-18B contributes to mathematical abilities in children with dyslexia and intraparietal sulcus variability in adults. Transl. Psychiatry 3:e229. doi: 10.1038/tp.2012.148
Magnin, E., Démonet, J. F., Wallon, D., Dumurgier, J., Troussière, A. C., Jager, A., et al. (2016). Primary progressive aphasia in the network of French Alzheimer plan memory centers. J. Alzheimer’s Dis. 54, 1459–1471. doi: 10.3233/JAD-160536
Marino, C., Citterio, A., Giorda, R., Facoetti, A., Menozzi, G., Vanzin, L., et al. (2007). Association of short-term memory with a variant within DYX1C1 in developmental dyslexia. Genes Brain Behav. 6, 640–646. doi: 10.1111/j.1601-183X.2006.00291.x
Marino, C., Mascheretti, S., Riva, V., Cattaneo, F., Rigoletto, C., Rusconi, M., et al. (2011). Pleiotropic effects of DCDC2 and DYX1C1 genes on language and mathematics traits in nuclear families of developmental dyslexia. Behav. Genet. 41, 67–76. doi: 10.1007/s10519-010-9412-7
Marino, C., Meng, H., Mascheretti, S., Rusconi, M., Cope, N., Giorda, R., et al. (2012). DCDC2 genetic variants and susceptibility to developmental dyslexia. Psychiatr. Genet. 22, 25–30. doi: 10.1097/YPG.0b013e32834acdb2
Marino, C., Scifo, P., Della Rosa, P. A., Mascheretti, S., Facoetti, A., Lorusso, M. L., et al. (2014). The DCDC2/intron 2 deletion and white matter disorganization: focus on developmental dyslexia. Cortex 57, 227–243. doi: 10.1016/j.cortex.2014.04.016
Martinelli, A., Rice, M. L., Talcott, J. B., Diaz, R., Smith, S., Raza, M. H., et al. (2021). A rare missense variant in the ATP2C2 gene is associated with language impairment and related measures. Hum. Mol. Genet. 30, 1160–1171. doi: 10.1093/hmg/ddab111
Mascheretti, S., Bureau, A., Battaglia, M., Simone, D., Quadrelli, E., Croteau, J., et al. (2013). An assessment of gene-by-environment interactions in developmental dyslexia-related phenotypes. Genes Brain Behav. 12, 47–55. doi: 10.1111/gbb.12000
Mascheretti, S., Bureau, A., Trezzi, V., Giorda, R., and Marino, C. (2015). An assessment of gene-by-gene interactions as a tool to unfold missing heritability in dyslexia. Hum. Genet. 134, 749–760. doi: 10.1007/s00439-015-1555-4
Mascheretti, S., De Luca, A., Trezzi, V., Peruzzo, D., Nordio, A., Marino, C., et al. (2017). Neurogenetics of developmental dyslexia: from genes to behavior through brain neuroimaging and cognitive and sensorial mechanisms. Transl. Psychiatry 7:e987. doi: 10.1038/tp.2016.240
Mascheretti, S., Riva, V., Giorda, R., Beri, S., Lanzoni, L. F. E., Cellino, M. R., et al. (2014). KIAA0319 and ROBO1: evidence on association with reading and pleiotropic effects on language and mathematics abilities in developmental dyslexia. J. Hum. Genet. 59, 189–197. doi: 10.1038/jhg.2013.141
Massinen, S., Tammimies, K., Tapia-Páez, I., Matsson, H., Hokkanen, M. E., Söderberg, O., et al. (2009). Functional interaction of DYX1C1 with estrogen receptors suggests involvement of hormonal pathways in dyslexia. Hum. Mol. Genet. 18, 2802–2812. doi: 10.1093/hmg/ddp215
Matsson, H., Huss, M., Persson, H., Einarsdottir, E., Tiraboschi, E., Nopola-Hemmi, J., et al. (2015). Polymorphisms in DCDC2 and S100B associate with developmental dyslexia. J. Hum. Genet. 60, 399–401. doi: 10.1038/jhg.2015.37
Mazoyer, B., Zago, L., Jobard, D., Crivello, F., Joliot, M., Perchey, G., et al. (2014). Gaussian mixture modeling of hemispheric lateralization for language in a large sample of healthy individuals balanced for handedness. PLoS One 9:e101165. doi: 10.1371/journal.pone.0101165
Morgan, A., Fisher, S. E., Scheffer, I., and Hildebrand, M. (2016). “FOXP2-related speech and language disorders. 2016 Jun 23 [Updated 2017 Feb 2],” in GeneReviews® [Internet], eds M. P. Adam, H. H. Ardinger, R. A. Pagon, S. E. Wallace, L. J. H. Bean, G. Mirzaa, et al. (Seattle, WA: University of Washington), 1993–2021.
Morosan, P., Schleicher, A., Amunts, K., and Zilles, K. (2005). Multimodal architectonic mapping of human superior temporal gyrus. Anat. Embryol. 210, 401–406. doi: 10.1007/s00429-005-0029-1
Mozzi, A., Riva, V., Forni, D., Sironi, M., Marino, C., Molteni, M., et al. (2017). A common genetic variant in FOXP2 is associated with language-based learning (dis)abilities: evidence from two Italian independent samples. Am. J. Med. Genet. B Neuropsychiatr. Genet. 174, 578–586. doi: 10.1002/ajmg.b.32546
Müller, B., Schaadt, G., Boltze, J., Emmrich, F., Skeide, M. A., Neef, N. E., et al. (2017). ATP2C2 and DYX1C1 are putative modulators of dyslexia-related MMR. Brain Behav. 7:e00851. doi: 10.1002/brb3.851
Müller, N. G., and Knight, R. T. (2006). The functional neuroanatomy of working memory: contributions of human brain lesion studies. Neuroscience 139, 51–58. doi: 10.1016/j.neuroscience.2005.09.018
Neef, N. E., Müller, B., Liebig, J., Schaadt, G., Grigutsch, M., Gunter, T. C., et al. (2017). Dyslexia risk gene relates to representation of sound in the auditory brainstem. Dev. Cogn. Neurosci. 24, 63–71. doi: 10.1016/j.dcn.2017.01.008
Newbury, D. F., Paracchini, S., Scerri, T. S., Winchester, L., Addis, L., Richardson, A. J., et al. (2011). Investigation of dyslexia and SLI risk variants in reading- and language-impaired subjects. Behav. Genet. 41, 90–104. doi: 10.1007/s10519-010-9424-3
Newbury, D. F., Winchester, L., Addis, L., Paracchini, S., Buckingham, L. L., Clark, A., et al. (2009). CMIP and ATP2C2 modulate phonological short-term memory in language impairment. Am. J. Hum. Genet. 85, 264–272. doi: 10.1016/j.ajhg.2009.07.004
Oguro-Ando, A., Rosensweig, C., Herman, E., Nishimura, Y., Werling, D., Bill, B. R., et al. (2015). Increased CYFIP1 dosage alters cellular and dendritic morphology and dysregulates mTOR. Mol. Psychiatry 20, 1069–1078. doi: 10.1038/mp.2014.124
Papagno, C., Comi, A., Riva, M., Bizzi, A., Vernice, M., Casarotti, A., et al. (2017). Mapping the brain network of the phonological loop. Hum. Brain Mapp. 38, 3011–3024. doi: 10.1002/hbm.23569
Paracchini, S., Ang, Q. W., Stanley, F. J., Monaco, A. P., Pennell, C. E., and Whitehouse, A. J. O. (2011). Analysis of dyslexia candidate genes in the Raine cohort representing the general Australian population. Genes Brain Behav. 10, 158–165. doi: 10.1111/j.1601-183X.2010.00651.x
Pennington, B. F., and Bishop, D. V. M. (2009). Relations among speech, language, and reading disorders. Annu. Rev. Psychol. 60, 283–306. doi: 10.1146/annurev.psych.60.110707.163548
Peschansky, V. J., Burbridge, T. J., Volz, A. J., Fiondella, C., Wissner-Gross, Z., Galaburda, A. M., et al. (2010). The effect of variation in expression of the candidate dyslexia susceptibility gene homolog Kiaa0319 on neuronal migration and dendritic morphology in the rat. Cereb. Cortex 20, 884–897. doi: 10.1093/cercor/bhp154
Peter, B., Raskind, W. H., Matsushita, M., Lisowski, M., Vu, T., Berninger, V. W., et al. (2011). Replication of CNTNAP2 association with nonword repetition and support for FOXP2 association with timed reading and motor activities in a dyslexia family sample. J. Neurodev. Disord. 3, 39–49. doi: 10.1007/s11689-010-9065-0
Pettigrew, K. A., Frinton, E., Nudel, R., Chan, M. T. M., Thompson, P., Hayiou-Thomas, M. E., et al. (2016). Further evidence for a parent-of-origin effect at the NOP9 locus on language-related phenotypes. J. Neurodev. Dis. 8:24. doi: 10.1186/s11689-016-9157-6
Pinel, P., Fauchereau, F., Moreno, A., Barbot, A., Lathrop, M., Zelenika, M., et al. (2012). Genetic variants of FOXP2 and KIAA0319/TTRAP/THEM2 locus are associated with altered brain activation in distinct language-related regions. J. Neurosci. 32, 817–825. doi: 10.1523/JNEUROSCI.5996-10.2012
Pollack, C., and Ashby, N. C. (2018). Where arithmetic and phonology meet: the meta-analytic convergence of arithmetic and phonological processing in the brain. Dev. Cogn. Neurosci. 30, 251–264. doi: 10.1016/j.dcn.2017.05.003
Powers, N. R., Eicher, J. D., Butter, F., Kong, Y., Miller, L. L., Ring, S. M., et al. (2013). Alleles of a polymorphic ETV6 binding site in DCDC2 confer risk of reading and language impairment. Am. J. Hum. Genet. 93, 19–28. doi: 10.1016/j.ajhg.2013.05.008
Powers, N. R., Eicher, J. D., Miller, L. L., Kong, Y., Smith, S. D., Pennington, B. F., et al. (2016). The regulatory element READ1 epistatically influences reading and language, with both deleterious and protective alleles. J. Med. Genet. 53, 163–171. doi: 10.1136/jmedgenet-2015-103418
Raghubar, K. P., Barnes, M. A., and Hecht, S. A. (2010). Working memory and mathematics: a review of developmental, individual difference, and cognitive approaches. Learn. Individ. Differ. 20, 110–122. doi: 10.1016/j.lindif.2009.10.005
Rice, M. L., Taylor, C. L., Zubrick, S. R., Hoffman, L., and Earnest, K. K. (2020). Heritability of specific language impairment and nonspecific language impairment at ages 4 and 6 years across phenotypes of speech, language, and nonverbal cognition. J. Speech Lang. Hear. Res. 63, 793–813. doi: 10.1044/2019_JSLHR-19-00012
Riva, V., Cantiani, C., Benasich, A. A., Molteni, M., Piazza, C., Giorda, R., et al. (2018). From CNTNAP2 to early expressive language in infancy: the mediation role of rapid auditory processing. Cereb. Cortex 28, 1–9. doi: 10.1093/cercor/bhx115
Riva, V., Mozzi, A., Forni, D., Trezzi, V., Giorda, R., Riva, S., et al. (2019). The influence of DCDC2 risk genetic variants on reading: testing main and haplotypic effects. Neuropsychologia 130, 52–58. doi: 10.1016/j.neuropsychologia.2018.05.021
Rogalski, E., Johnson, N., Weintraub, S., and Mesulam, M. (2008). Increased frequency of learning disability in patients with primary progressive aphasia and their first-degree relatives. Arch. Neurol. 65, 244–248. doi: 10.1001/archneurol.2007.34
Rogalsky, C., and Hickok, G. (2011). The role of Broca‘s area in sentence comprehension. J. Cogn. Neurosci. 23, 1664–1680. doi: 10.1162/jocn.2010.21530
Sánchez-Morán, M., Hernández, J. A., Duñabeitia, J. A., Estévez, A., Bárcena, L., González-Lahera, A., et al. (2018). Genetic association study of dyslexia and ADHD candidate genes in a Spanish cohort: implications of comorbid samples. PLoS One 13:e0206431. doi: 10.1371/journal.pone.0206431
Scerri, T. S., Morris, A. P., Buckingham, L. L., Newbury, D. F., Miller, L. L., Monaco, A. P., et al. (2011). DCDC2, KIAA0319 and CMIP are associated with reading-related traits. Biol. Psychiatry 70, 237–245. doi: 10.1016/j.biopsych.2011.02.005
Skeide, M. A., and Friederici, A. D. (2016). The ontogeny of the cortical language network. Nat. Rev. Neurosci. 17, 323–332. doi: 10.1038/nrn.2016.23
Smith, A. W., Holden, K. R., Dwivedi, A., Dupont, B. R., and Lyons, M. J. (2015). Deletion of 16q24.1 supports a role for the ATP2C2 gene in specific language impairment. J. Child Neurol. 30, 517–521. doi: 10.1177/0883073814545113
Sonty, S. P., Mesulam, M. M., Weintraub, S., Johnson, N. A., Parrish, T. B., and Gitelman, D. R. (2007). Altered effective connectivity within the language network in primary progressive aphasia. J. Neurosci. 27, 1334–1345. doi: 10.1523/JNEUROSCI.4127-06.2007
Stefansson, H., Meyer-Lindenberg, A., Steinberg, S., Magnusdottir, B., Morgen, K., Arnarsdottir, S., et al. (2014). CNVs conferring risk of autism or schizophrenia affect cognition in controls. Nature 505, 361–366. doi: 10.1038/nature12818
Stefansson, H., Rujescu, D., Cichon, S., Pietiläinen, O. P. H., Ingason, A., Steinberg, S., et al. (2008). Large recurrent microdeletions associated with schizophrenia. Nature 455, 232–236. doi: 10.1038/nature07229
Steinbrink, C., Schwanda, S., and Vogt, K. (2008). Relations between cognitive skills and reading and spelling performance in first graders with poor spelling abilities. Nervenheilkunde 27, 644–651. doi: 10.1055/s-0038-1627124
Szalkowski, C. E., Booker, A. B., Truong, D. T., Threlkeld, S. W., Rosen, G. D., and Fitch, R. H. (2013). Knockdown of the candidate dyslexia susceptibility gene homolog dyx1c1 in rodents: effects on auditory processing, visual attention, and cortical and thalamic anatomy. Dev. Neurosci. 35, 50–68. doi: 10.1159/000348431
Tam, G. W. C., Van De Lagemaat, L. N., Redon, R., Strathdee, K. E., Croning, M. D. R., Malloy, M. P., et al. (2010). Confirmed rare copy number variants implicate novel genes in schizophrenia. Biochem. Soc. Trans. 38, 445–451. doi: 10.1042/BST0380445
Tarkar, A., Loges, N. T., Slagle, C. E., Francis, R., Dougherty, G. W., Tamayo, J. V., et al. (2013). DYX1C1 is required for axonemal dynein assembly and ciliary motility. Nat. Genet. 45, 995–1003. doi: 10.1038/ng.2707
Thompson, P. A., Hulme, C., Nash, H. M., Gooch, D., Hayiou-Thomas, E., and Snowling, M. J. (2015). Developmental dyslexia: predicting individual risk. J. Child Psychol. Psychiatry 56, 976–978. doi: 10.1111/jcpp.12412
Torrens, V., and Yagüe, E. (2016). The role of phonological working memory in children with SLI. Lang. Acquis. 25, 102–117. doi: 10.1080/10489223.2016.1187617
Tosto, M. G., Hayjiou-Thomas, M. E., Harlaar, N., Prom-Wormley, E., Dale, P. S., and Plomin, R. (2017). The genetic architecture of oral language, reading fluency, and reading comprehension: a twin study from 7 to 16 years. Dev. Psychol. 53, 1115–1129. doi: 10.1037/dev0000297
Träff, U., and Passolunghi, M. C. (2015). Mathematical skills in children with dyslexia. Learn. Individ. Differ. 40, 108–114. doi: 10.1016/j.lindif.2015.03.024
Tran, C., Wigg, K. G., Zhang, K., Cate-Carter, T. D., Kerr, E., Field, L. L., et al. (2014). Association of the ROBO1 gene with reading disabilities in a family-based analysis. Genes Brain Behav. 13, 430–438. doi: 10.1111/gbb.12126
Treiman, R. (2017). Learning to spell: phonology and beyond. Cogn. Neuropsychol. 34, 83–93. doi: 10.1080/02643294.2017.1337630
Troung, D. T., Adams, A. K., Paniagua, S., Frijters, J. C., Boada, R., Hill, D. E., et al. (2019). Multivariate genome-wide association study of rapid automatised naming and rapid alternating stimulus in Hispanic American and African-American youth. J. Med. Genet. 56, 557–566. doi: 10.1136/jmedgenet-2018-105874
Ulfarsson, M. O., Walters, G. B., Gustafsson, O., Steinberg, S., Silva, A., Doyle, O. M., et al. (2017). 15q11.2 CNV affects cognitive, structural and functional correlates of dyslexia and dyscalculia. Transl. Psychiatry 7:e1109. doi: 10.1038/tp.2017.77
Verhoeven, J. S., Rommel, N., Prodi, E., Leemans, A., Zink, I., Vandewalle, E., et al. (2012). Is there a common neuroanatomical substrate of language deficit between autism spectrum disorder and specific language impairment? Cereb. Cortex 22, 2263–2271. doi: 10.1093/cercor/bhr292
Vernes, S. C., Newbury, D. F., Abrahams, B. S., Winchester, L., Nicod, J., Groszer, M., et al. (2008). A functional genetic link between distinct developmental language disorders. N. Engl. J. Med. 359, 2337–2345. doi: 10.1056/NEJMoa0802828
Wadsworth, S. J., Olson, R. K., and Defries, J. C. (2010). Differential genetic etiology of reading difficulties as a function of IQ: an update. Behav. Genet. 40, 751–758. doi: 10.1007/s10519-010-9349-x
Wagner, S., Sebastian, A., Lieb, K., Tüscher, O., and Tadić, A. (2014). A coordinate-based ALE functional MRI meta-analysis of brain activation during verbal fluency tasks in healthy control subjects. BMC Neurosci. 15:19. doi: 10.1186/1471-2202-15-19
Wallace, M. T. (2009). Dyslexia: bridging the gap between hearing and reading. Curr. Biol. 19, R260–R262. doi: 10.1016/j.cub.2009.01.025
Wang, Y., Paramasivam, M., Thomas, A., Bai, J., Kaminen-Ahola, N., Kere, J., et al. (2006). DYX1C1 functions in neuronal migration in developing neocortex. Neuroscience 143, 515–522. doi: 10.1016/j.neuroscience.2006.08.022
Wei, C. H., Kao, H. Y., and Lu, Z. (2013). PubTator: a web-based text mining tool for assisting biocuration. Nucleic Acids Res. 41, W518–W522. doi: 10.1093/nar/gkt441
Whitehouse, A. J. O., Bishop, D. V. M., Ang, Q. W., Pennell, C. E., and Fisher, S. E. (2011). CNTNAP2 variants affect early language development in the general population. Genes Brain Behav. 10, 451–456. doi: 10.1111/j.1601-183X.2011.00684.x
Wilcke, A., Ligges, C., Burkhardt, J., Alexander, M., Wolf, C., Quente, E., et al. (2012). Imaging genetics of FOXP2 in dyslexia. Eur. J. Hum. Genet. 20, 224–229. doi: 10.1038/ejhg.2011.160
Yi, H. G., Leonard, M. K., and Chang, E. F. (2019). The encoding of speech sounds in the temporal gyrus. Neuron 102, 1096–1110. doi: 10.1016/j.neuron.2019.04.023
Keywords: development, phonology, gene expression, Broca’s area, Wernicke’s area
Citation: Unger N, Heim S, Hilger DI, Bludau S, Pieperhoff P, Cichon S, Amunts K and Mühleisen TW (2021) Identification of Phonology-Related Genes and Functional Characterization of Broca’s and Wernicke’s Regions in Language and Learning Disorders. Front. Neurosci. 15:680762. doi: 10.3389/fnins.2021.680762
Received: 15 March 2021; Accepted: 04 August 2021;
Published: 03 September 2021.
Edited by:
Silvia Pellegrini, University of Pisa, ItalyReviewed by:
Claudio V. Mello, Oregon Health and Science University, United StatesCopyright © 2021 Unger, Heim, Hilger, Bludau, Pieperhoff, Cichon, Amunts and Mühleisen. This is an open-access article distributed under the terms of the Creative Commons Attribution License (CC BY). The use, distribution or reproduction in other forums is permitted, provided the original author(s) and the copyright owner(s) are credited and that the original publication in this journal is cited, in accordance with accepted academic practice. No use, distribution or reproduction is permitted which does not comply with these terms.
*Correspondence: Thomas W. Mühleisen, dC5tdWVobGVpc2VuQGZ6LWp1ZWxpY2guZGU=
†ORCID: Nina Unger, orcid.org/0000-0001-5388-8814; Stefan Heim, orcid.org/0000-0002-5818-8355; Dominique I. Hilger, orcid.org/0000-0003-3360-5754; Sebastian Bludau, orcid.org/0000-0001-6173-9050; Peter Pieperhoff, orcid.org/0000-0001-6166-8926; Sven Cichon, orcid.org/0000-0002-9475-086X; Katrin Amunts, orcid.org/0000-0001-5828-0867; Thomas W. Mühleisen, orcid.org/0000-0002-6057-5952
Disclaimer: All claims expressed in this article are solely those of the authors and do not necessarily represent those of their affiliated organizations, or those of the publisher, the editors and the reviewers. Any product that may be evaluated in this article or claim that may be made by its manufacturer is not guaranteed or endorsed by the publisher.
Research integrity at Frontiers
Learn more about the work of our research integrity team to safeguard the quality of each article we publish.