- 1Department of Medical Imaging, The First Affiliated Hospital of Xi’an Jiaotong University, Xi’an, China
- 2Department of Neurology, The First Affiliated Hospital of Xi’an Jiaotong University, Xi’an, China
Introduction: The brainstem is an important component in the pathology of amyotrophic lateral sclerosis (ALS). Although neuroimaging studies have shown multiple structural changes in ALS patients, few studies have investigated structural alterations in the brainstem. Herein, we compared the brainstem structure between patients with ALS and healthy controls.
Methods: A total of 33 patients with ALS and 33 healthy controls were recruited in this study. T1-weighted and diffusion tensor imaging (DTI) were acquired on a 3 Tesla magnetic resonance imaging (3T MRI) scanner. Volumetric and vertex-wised approaches were implemented to assess the differences in the brainstem’s morphological features between the two groups. An atlas-based region of interest (ROI) analysis was performed to compare the white matter integrity of the brainstem between the two groups. Additionally, a correlation analysis was used to evaluate the relationship between ALS clinical characteristics and structural features.
Results: Volumetric analyses showed no significant difference in the subregion volume of the brainstem between ALS patients and healthy controls. In the shape analyses, ALS patients had a local abnormal surface contraction in the ventral medulla oblongata and ventral pons. Compared with healthy controls, ALS patients showed significantly lower fractional anisotropy (FA) in the left corticospinal tract (CST) and bilateral frontopontine tracts (FPT) at the brainstem level, and higher radial diffusivity (RD) in bilateral CST and left FPT at the brainstem level by ROI analysis in DTI. Correlation analysis showed that disease severity was positively associated with FA in left CST and left FPT.
Conclusion: These findings suggest that the brainstem in ALS suffers atrophy, and degenerative processes in the brainstem may reflect disease severity in ALS. These findings may be helpful for further understanding of potential neural mechanisms in ALS.
Introduction
Amyotrophic lateral sclerosis (ALS) is a fatal neurodegenerative disease. It predominantly affects the upper and the lower motor neurons in the cerebral cortex, brainstem, and spinal cord, leading to progressive limb strength loss, dysarthria, drooling, tongue wasting, and dysphagia (Brown and Al-Chalabi, 2017; van Es et al., 2017). More recently, it appears that ALS pathology involves more than the motor system and pathological TAR DNA-binding protein 43 (TDP-43) inclusions have been confirmed to be in four stages in the whole brain, inclusive of extra-motor cortical and subcortical structures and the brainstem (Braak et al., 2013; Brettschneider et al., 2013; Qiao et al., 2020).
Advanced magnetic resonance imaging (MRI) methods are robust imaging techniques that enable the evaluation of neurological systems degeneration in ALS in vivo (Chio et al., 2014). Neuroimaging studies have revealed that anatomical and functional changes not only involve precentral gyrus (Walhout et al., 2015; Alshikho et al., 2018; Grapperon et al., 2019; Contarino et al., 2020) and corticospinal tract (CST) (Senda et al., 2017; Gorges et al., 2018; Ishaque et al., 2018; Broad et al., 2019; Baek et al., 2020) but also spread to the frontal cortex (Consonni et al., 2018, 2019), thalamus (Schönecker et al., 2018; Tu et al., 2018), and basal ganglia (Bede et al., 2013; Machts et al., 2015). On the other hand, quantitative volumetric studies in ALS have detected both gray and white atrophy results in global spinal cord atrophy in ALS (Rasoanandrianina et al., 2017; Paquin et al., 2018). Furthermore, CST and anterior horns degeneration and alterations in ALS are associated with functional impairment (Cohen-Adad et al., 2013). The brainstem pathology is considered as the “first stage” in the suggested four-stage pathological staging system based on pathological TDP-43 burden patterns (Brettschneider et al., 2013). However, few neuroimaging studies have addressed morphological changes in the brainstem and its subregions in ALS patients.
Corticospinal disruption is generally studied in ALS (Chio et al., 2014), while alterations in other sensorimotor pathways are less well understood. This could be because several experiments have studied morphological changes in the cerebrum, in which most of the ascending and descending brain pathways overlap. Besides, the sensorimotor pathways are separated in the brainstem (Vanderah and Gould, 2015; Gray, 2016). The brainstem is a fundamental structure that communicates motor and sensory information between the cerebral cortex and the spinal cord. Previous researches using diffusion tensor imaging (DTI) have confirmed the extensive degeneration of the brain in ALS patients. However, most studies only established the most significant alterations in CST in the ALS brainstem areas, with limited research investigating extra significant white matter tract alterations in the brainstem regions in the ALS group.
Therefore, the current study aimed to reveal the patterns of focal gray matter atrophy and white matter damage in brainstem regions of ALS patients. Both volumetric and vertex-wise approaches were performed for the brainstem to compare ALS patients and healthy controls. Then the region of interest (ROI) analysis was used to compare diffusion metrics in ALS patients with the white matter in healthy controls. Additionally, correlation analysis was used to evaluate the relationship between ALS clinical features and volume or diffusion metrics.
Materials and Methods
Participants
Thirty-three patients were recruited. Twenty-two patients with definite ALS and 11 patients with probable ALS were clinically diagnosed based on the revised El Escorial criteria. None of the ALS patients in this study had a family history of ALS. With respect to ALS phenotypes classification, as formerly identified by Chiò et al. (2011), 29 patients displayed classic phenotype and four exhibited bulbar phenotype. To evaluate disease severity, the Revised ALS Functional Rating Scale (ALSFRS-R) (Brooks et al., 2000) was used. The duration of the disease was calculated from the onset of symptoms to the acquisition of MR imaging in months. To modify the degree of disability for disease duration, the rate of disease progression was calculated using the following formula: (48-ALSFRS-R score)/(disease duration). None of the ALS patients had a history of cerebrovascular events, intracranial pathology, or other neurological diseases. None of the ALS patients had clinical signs of frontotemporal dementia. Mini-Mental Status Examination (MMSE) was used to assess general cognitive functions. Thirty-three right-handed healthy controls matched for age and gender were recruited. There was no history of mental and neurological problems in healthy controls.
Written informed consent was obtained from all participants. Ethical approval for all procedures was obtained from the Ethics Committee of the First Affiliated Hospital of Xi’an Jiaotong University in advance.
MRI Acquisition
Magnetic resonance imaging data were acquired on a 3 Tesla GE scanners (General Electric Healthcare, Milwaukee, WI, United States) using an eight-channel parallel head coil. The high-resolution T1-weighted MRI images of the brain were obtained using a 3D T1 fast spoiled gradient-echo sequence with the following parameters: TE = 4.8 ms; TR = 10.8 ms; field of view (FOV) = 256 mm × 256 mm; matrix = 256 × 256; voxel size = 1 × 1 × 1 mm. The conventional T2 weighted imaging, fluid-attenuated inversion recovery (FLAIR) sequences were obtained to rule out cerebral infarction, tumors, and other incidental findings. Whole-brain DTI images were performed using an echo-planar imaging sequence with the following parameters: TR = 14 s, TE = 90.7 ms, FOV = 256 mm × 256 mm, matrix = 128 × 128, slice thickness = 2.5 mm, 35 isotropic directions, b-value = 1000 s/mm1. In addition, one scan without diffusion weighting (b = 0 s/mm2) was acquired. Resting-state functional MRI (reported elsewhere) was also obtained. The total acquisition time was approximately 30 min for each subject. During scanning, a tight but comfortable sponge pad inside the head coil was used to restrict head motion.
Image Analysis
FreeSurfer software version 6.0 (Massachusetts General Hospital, Boston, MA, United States2) was used for the preprocessing of T1-weighted images (Fischl et al., 1999). The main recon stream (“recon-all”) in FreeSurfer is used for volumetric segmentation, specifically including motion correction, skull-stripping, non-parametric non-uniform intensity normalization, Talairach transformation (affine transform from the original volume to the MNI305 atlas), volumetric registration, and topology correction (Fischl et al., 2001; Segonne et al., 2007; Reuter et al., 2010). Automated segmentation and volume computations of the whole brainstem and four brainstem substructures [pons, midbrain, medulla, and superior cerebellar peduncle (SCP)] were completed using the brainstem substructures toolbox implemented in FreeSurfer software. Segmentation was conducted using a robust and accurate Bayesian algorithm relying on a probabilistic atlas of the brainstem and neighboring anatomical structures (Iglesias et al., 2015). Each subject’s T1 imaging outputs in all processes were carefully inspected for errors by two trained independent researchers to ensure the quality of brainstem segmentation.
Moreover, the total intracranial volume (TIV) was calculated for each participant with SIENAX (Smith et al., 2002) in the FMRIB Software Library (FSL version 6.0.32) (Jenkinson et al., 2012), which was used as a covariate for subsequent volumetric comparisons.
While volumetric analysis can only offer information about the total size of the brainstem, vertex-wise subcortical shape analysis can identify changes in the shape of the brainstem and offer more information about regional abnormalities in the brainstem. Vertex-wise shape analysis was achieved through the algorithm FIRST, (Patenaude et al., 2011), a model-based segmentation and registration module implemented in FSL software. This method is based on a Bayesian framework model; the multivariate Gaussian shape and appearance of subcortical structures are constructed from a large set of manually labeled images (336 brains) provided by the Center for Morphometric Analysis, Massachusetts General Hospital, Boston. During registration, raw 3D T1 images are transformed to MNI152 template by standard 12 degrees of freedom and accurately registered to a Montreal Neurological Institute (MNI) 152 brainstem mask to exclude voxels outside the brainstem region. Then brainstem was automatically segmented based on a Bayesian framework. Afterward, the surface mesh output of the brainstem, which was used for surface-based vertex analyses, was generated.
Diffusion tensor imaging data processing and analysis were performed using software tools from FSL. Eddy current induced distortions and head motion in the diffusion-weighted images was corrected using EDDY tools provided in the FSL, (Andersson and Sotiropoulos, 2016). Then the quality of the dataset was assessed using QUAD and SQUAD (automated EDDY quality control framework in FSL), (Bastiani et al., 2019). The quality control criteria were set as average absolute volume to volume head motion of <3 mm or total outliers <5% by referencing the previous literature, (Zheng et al., 2021). An example of a quality control report is added in Supplementary Material. Skull stripping was performed for each participant using FSL’s Brain Extraction Tool (BET), (Smith, 2002). Afterward, by fitting a tensor model to the raw diffusion data, quantitative DTI parameters of fractional anisotropy (FA), axial diffusivity (AD), mean diffusivity (MD), and radial diffusivity (RD) images were calculated.
All subjects’ FA, MD, AD, and RD images were then registered to the standard MNI152 space using a non-linear registration algorithm (FSL’s FLIRT and FNIRT) (Smith et al., 2004; Greve and Fischl, 2009), which uses a b-spline representation of the registration warp field (Rueckert et al., 1999).
An atlas-based ROI analysis was performed to compare diffusion metrics of brainstem fiber pathways in ALS patients with healthy controls. Recently, a novel probabilistic atlas of 23 brainstem pathways using the Human Connectome Project (HCP) data was developed and publicly distributed (Tang et al., 2018). The atlas was in the MNI152 space, which can be downloaded on NITRIC3. ROIs were defined by anatomic marks obtained from the 23 brainstem pathways atlas and six ROIs of motor tracts were identified, which were respectively bilateral CST, bilateral frontopontine tracts (FPT), and bilateral parieto-occipito-temporo-pontine tracts (POTPT) (Figure 1). The average values within each region were calculated.
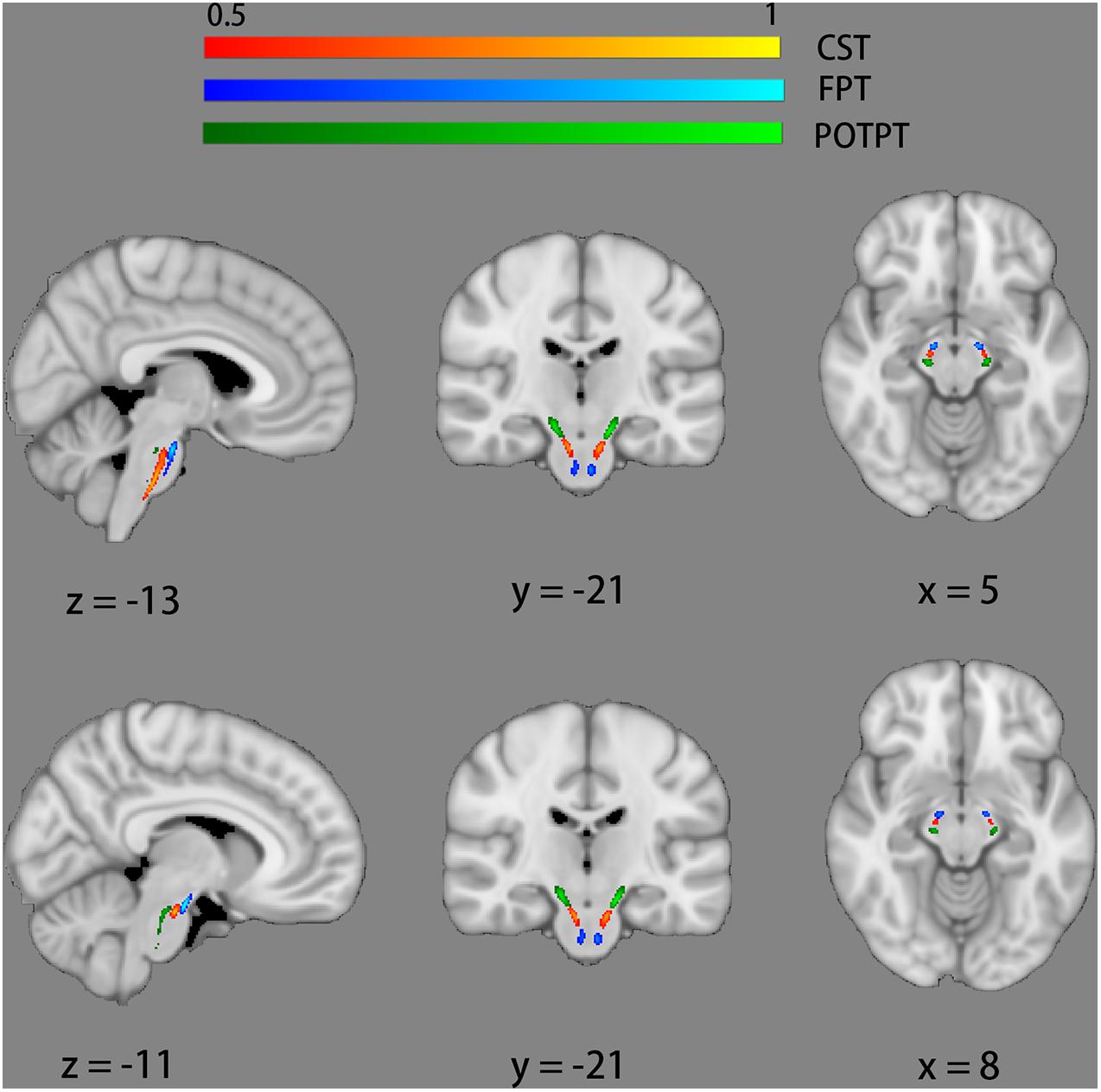
Figure 1. Six regions of interest of motor tracts defined by probabilistic atlases of 23 brainstem bundles. The bilateral corticospinal tracts (CST) are marked red-yellow. The bilateral fronto-pontine tracts (FPT) are displayed in blue. The bilateral parieto-occipito-temporo-pontine tracts (POTPT) are represented as green.
Statistics Analysis
Normal distribution assumption was examined by Kolmogorov–Smirnov tests. Group differences in the clinical data were evaluated using a t-test for continuous variables and Chi-square tests for discrete variables. All non-voxel-wise statistical analyses were carried out with Statistical Package for the Social Sciences (SPSS) V.20 (IBM SPSS, IBM Corp., NY, United States).
An analysis of covariance (ANCOVA) was used to test for volume differences of the whole brainstem and its subregions between groups, adjusting for the effect of age and TIV to avoid spurious results.
Meanwhile, ANCOVA was performed to test for changes in DTI parameters within the brainstem fiber pathways between groups with age as a covariate. The analysis was carried out with average values of FA, MD, RD, and AD, respectively. After Bonferroni correction, P < 0.008 (0.05/6) was considered statistically significant. In line with recent recommendations (Chen et al., 2017), partial eta squared was calculated to estimate effect sizes.
For statistical analysis of brainstem shape data, general linear models consisting of age as a covariate were used for permutation-based non-parametric statistics. The non-parametric permutation approach (5000 permutations) was accomplished by the randomize tool commanded in FSL, (Winkler et al., 2014). Results with P < 0.05 were taken into consideration significantly after threshold-free cluster enhancement (TFCE) and family-wise error corrected (FWE) correction for multiple comparisons (Smith and Nichols, 2009).
Due to skewed distributions, the Spearman correlation test was used to analyze the relationship between different variables. Correlation significant at P < 0.008 (0.05/6) was considered statistically significant after Bonferroni correction for multiple comparisons.
Results
Demographic and Clinical Characteristics
The demographic and clinical data of enrolled individuals are summarized in Table 1. There were no significant differences in age (P = 0.852) or gender (P = 0.622) between patient groups and the healthy controls. There were no significant differences in MMSE (P = 0.07).
Comparisons of the Volume and Shape of Brainstem Structures Between ALS Patients and Healthy Controls
There were no significant differences in the volumes of the whole brainstem and three brainstem subregions between ALS patients and the healthy control group (Table 2).
Comparisons of the vertex-wise shape of brainstem structures between ALS patients and healthy controls are shown in Figure 2. Automated brainstem vertex-wise analysis revealed that the ventral medulla oblongata and a small part of the ventral pons had significant group differences in the ALS group than in the healthy controls group, following TFCE and FWE correction (P < 0.05).
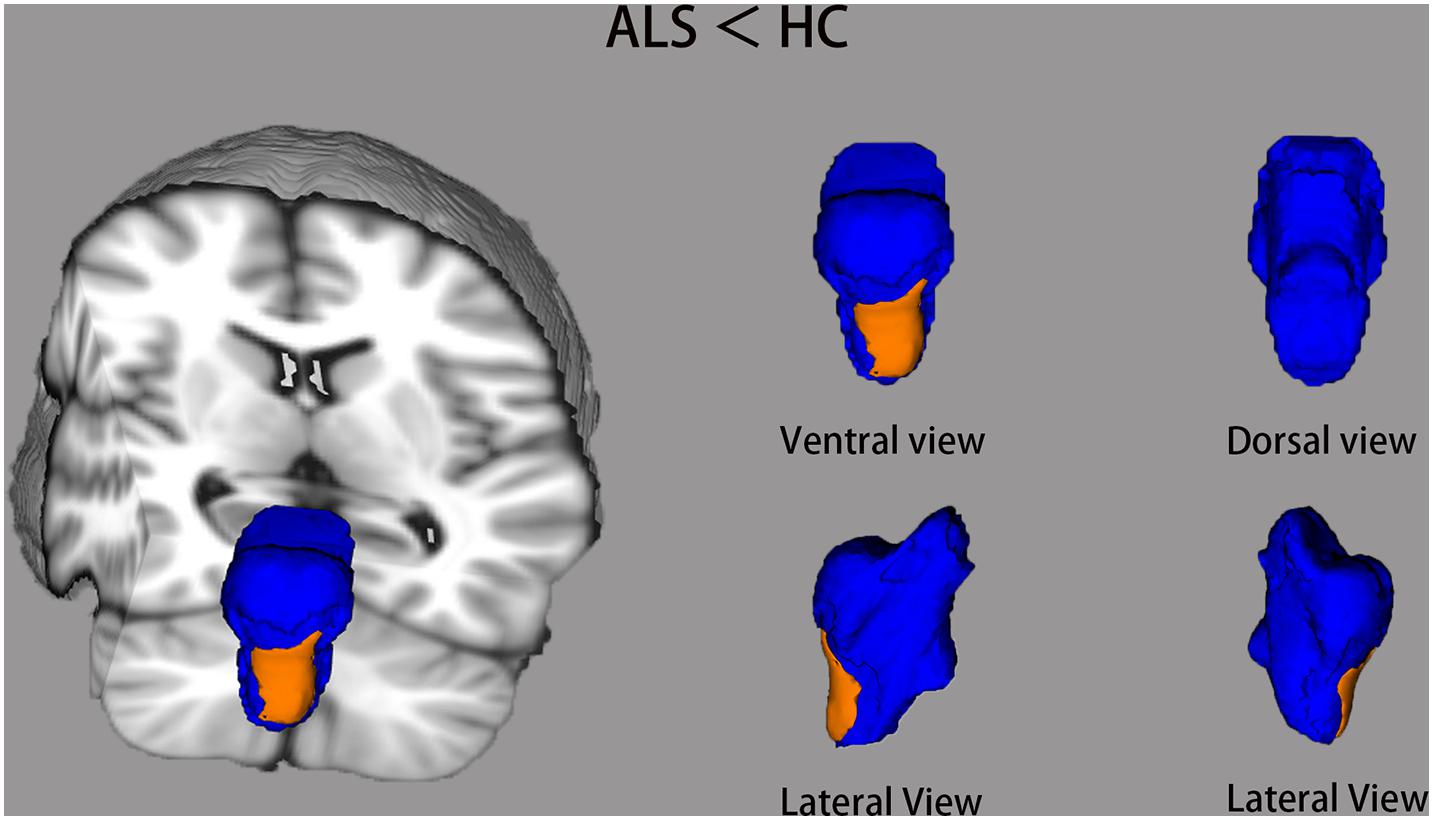
Figure 2. The brainstem shape differences between ALS groups and healthy controls (HC) using vertex-wise surface analyses. The blue color indicates the 3D brainstem mesh. The regions in orange represent ALS-related local shape deformations (ALS < HC). The results are corrected for multiple testing with the threshold-free cluster enhancement (TFCE) and family-wise error (FWE) method (P < 0.05).
Comparison of DTI Findings With Atlas-Based ROI Analysis
Figure 3 and Table 3 show comparisons of diffusion metrics between ALS patients and healthy controls for six motor tracts of brainstem pathways. In the ROI analysis, ALS patients had significantly decreased FA values in the left CST but increased RD values in the bilateral CST at the brainstem level (P < 0.008). In addition, ALS patients had significantly decreased FA values in the bilateral FPT but increased RD values in the left FPT (P < 0.008).
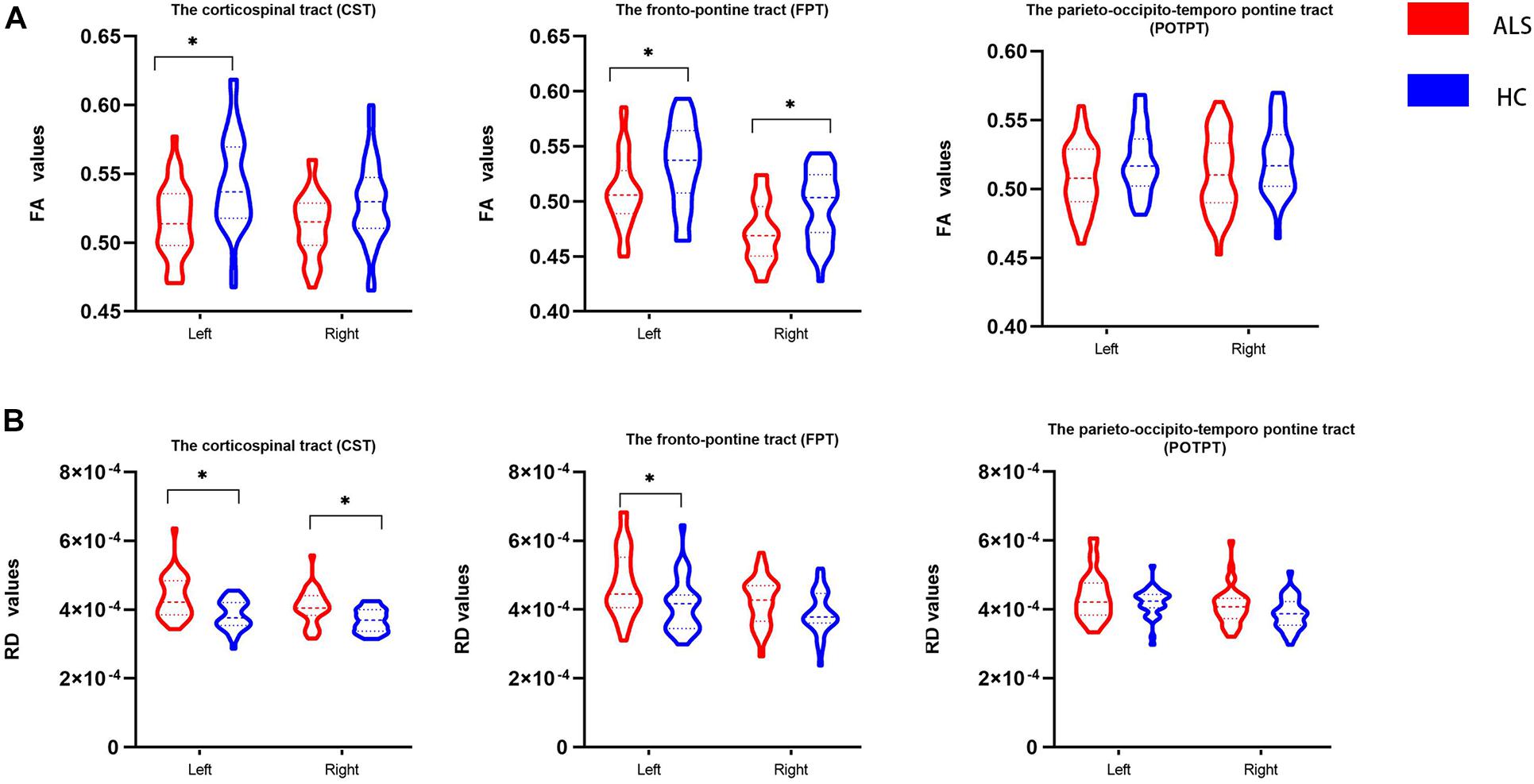
Figure 3. The violin plot depicts the diffusion measures from the region of interest analysis of ALS patients and healthy control groups. The blue color represents healthy controls (HC), and the red represents ALS patients. Inner violin plot shows the quartile, median. Asterisk indicates significance after Bonferroni correction. (A) Comparison of FA values between groups. (B) Comparison of RD values between groups.
Correlations Between Imaging Findings and Clinical Characteristics
As shown in Figure 4, ALSFRS-R showed significant positive correlations with FA values of the left CST (r = 0.468, P = 0.006). Similarly, the bulbar sub-score showed positive correlations with FA values in the left CST (r = 0.590, P < 0.001). Moreover, the upper sub-score showed significant positive correlations with left CST (r = 0.550, P < 0.001) and the left FPT (r = 0.475, P = 0.005). There were no other significant associations between imaging findings (volumetric metrics and vertex data) and clinical data.
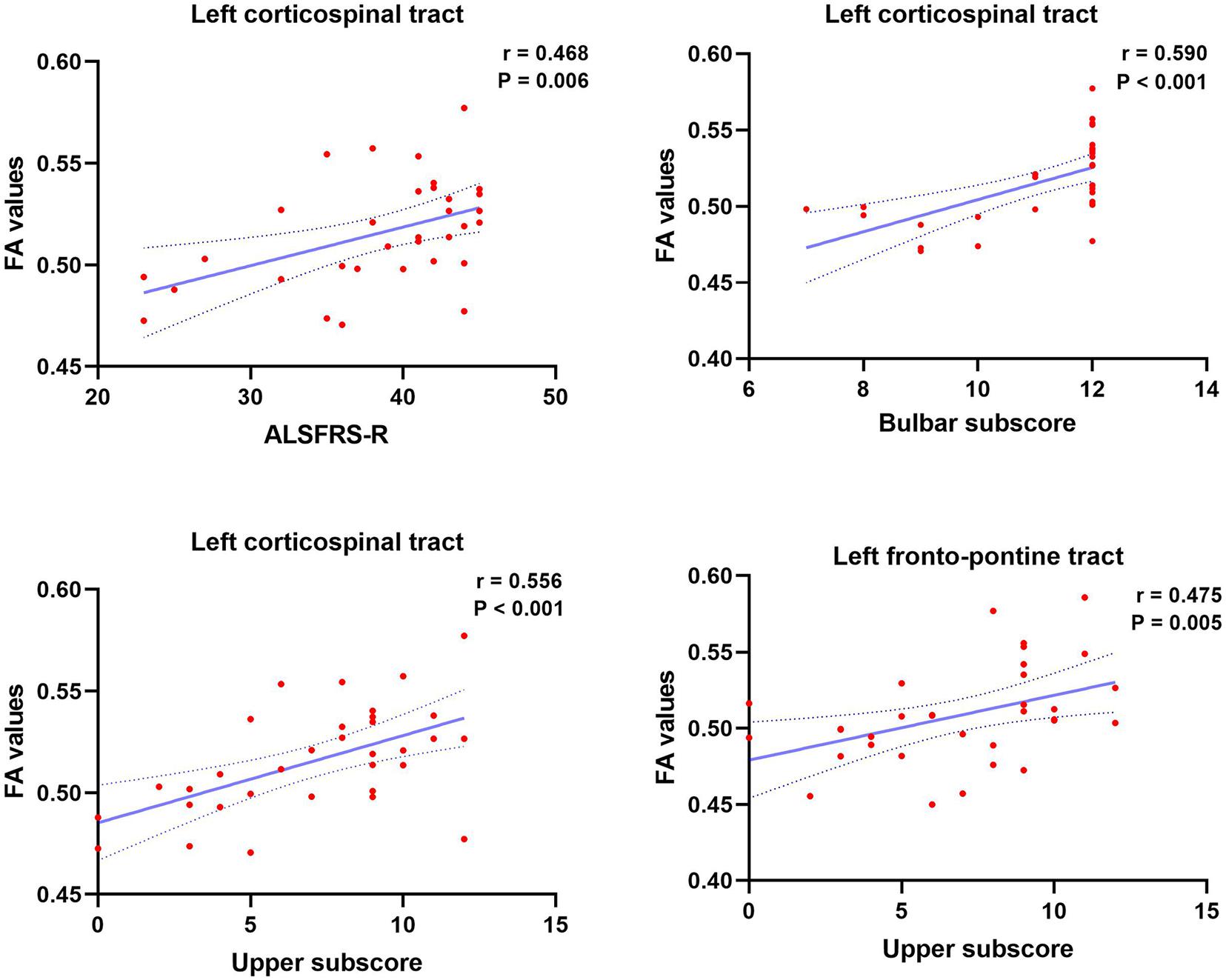
Figure 4. Significant clinical correlates of ALSFRS-R, ALSFRS-R bulbar subscore, and upper limb subscore, with FA values of brainstem pathways in patients with ALS. Dotted lines represent the 95% confidence region of the linear model fit. P < 0.008 (0.05/6) was considered statistically significant after Bonferroni correction. ALSFRS-R, Revised Amyotrophic Lateral Sclerosis Functional Rating Scale.
Discussion
This study demonstrated the patterns of structural degeneration of the brainstem in ALS using the volumetric and surface-based approach, in addition to selective fiber integrity investigation. The results revealed that ALS patients encounter specific brainstem volume loss and white matter degeneration. The volume loss in the brainstem is mainly characterized by the local shape deformation in the medulla oblongata and pons. Selective degeneration in the CST and FPT related to clinical disease deterioration.
Our vertex-wised analyses identified shape deformations of the medulla oblongata in ALS patients compared with healthy controls. A fraction of involvement of posterior pons was also demonstrated in ALS patients. A previous study by Bede et al. (2019) proved significant alterations of the brainstem in ALS patients. Brainstem pathology refers to stage one of the lately proposed phosphorylated TDP-43 (pTDP-43) pathological staging scheme, which is characterized by the involvement in the brainstem motor nuclei of cranial V, VII, and X–XII of TDP-43 pathology (Braak et al., 2013; Brettschneider et al., 2013). Furthermore, the aforementioned cranial nerves are mainly distributed in the medulla oblongata and pons. Our volumetric analysis did not reveal the general volume reduction of the gray matter related to ALS patients in the three subregions of the brainstem. Metabolism has been shown to increase in bilateral midbrain and pons areas in ALS patients, suggesting the local activation of microglia and astrocytes in the brainstem of ALS patients (Haukedal and Freude, 2019; Volonté et al., 2019). Studies of ALS postmortem (Cardenas et al., 2017) and mouse model pathology (Chiarotto et al., 2019; Espejo-Porras et al., 2019) have shown astrogliosis and active microglia surrounding the neurodegeneration region. One possible reason for the negative result may be that the increased number of astrocytes in brain regions influenced by ALS occupies the place of the dead neurons, compensating for volume loss by shrinkage of neurons.
The relationship between the volume and the shape structure on the brainstem surface is yet to be understood (Rahayel et al., 2019). Volume analysis only provides information about the global size of the brainstem and neither presents any location information for volume changes nor offers any information about the shape (Patenaude et al., 2011). The present results showed that the estimated total brainstem volume might not capture structural alterations in ALS patients. The change in shape may precede volume changes of the brainstem. Therefore, a shape-based morphological analysis could be a useful tool for detecting early brainstem atrophy in ALS.
In our study, significantly reduced FA values in the left CST at the brainstem level and increased RD values in the bilateral CST at the brainstem level were observed. Other DTI studies have also investigated significant abnormalities at brainstem levels of the CST in ALS patients (Cardenas-Blanco et al., 2014; Floeter et al., 2014; Schuster et al., 2016; Baek et al., 2020). A previous multicenter imaging study detected a reduced FA value within CST at the brainstem level (Müller et al., 2016). Furthermore, a recent voxel-based meta-analysis revealed significant FA reduction in the right CST that stretched to the right cerebral peduncle (Zhang et al., 2018). Decreased FA and increased RD values commonly reflect some degree of impaired fiber integrity (Hutton et al., 2020). FA assesses the extent of anisotropy of diffusion processes (van Veluw et al., 2019). RD is a straight estimate that represents vertical directions to the tract, offering more indirect information on pathological changes in the axons or myelin compared to FA or MD (Fattah et al., 2020). Recent research has validated RD metrics as sensitive to characterize myelin (Geeraert et al., 2018). In addition, pathological changes of the white matter, including myelin damage and axonal degeneration, have been revealed in animal ALS models and postmortem studies of ALS patients (Kang et al., 2013; Wang et al., 2020). Therefore, the DTI results indicated a disruption and degeneration of CST in the brainstem region.
The present analysis showed a significant positive association between FA values of the left CST and total ALSFRS-R, which is consistent with former DTI studies (Bao et al., 2018; Kassubek et al., 2018). Lower performance in the bulbar function and the upper limb function was associated with lower FA values of the left CST in the brainstem level in ALS patients. A previous study has found an increase in functional brain network connectivity along with ALS disease progression (Sorrentino et al., 2018). In addition, a previous neuroimaging study showed that disease-related gray and white matter changes in ALS patients propagated from the motor to extra-motor areas as ALS progresses (Trojsi et al., 2015). The current findings may reinforce the notion that disease may propagate through axonal pathways in multiple regions of the brain. Following this evidence, we suggest that the CST at the brainstem level may be a reliable region for monitoring disease severity. Meanwhile, the results may provide further in vivo evidence for the proposed staging scheme of ALS-associated pathology (Braak et al., 2013; Brettschneider et al., 2013).
Patients with ALS had decreased FA values in bilateral FPT underlying the brainstem and increased RD values in the left FPT in the brainstem level. FPT is part of the cortico-ponto-cerebellar system, which is a white matter tract stretching from the cerebral cortex via pontine nuclei to the cerebellum (Jang et al., 2014; Palesi et al., 2017). This system plays an important role in movement regulation and modulates higher cognitive functions (Palesi et al., 2017; Wagner and Luo, 2020). Based on a multicenter DTI study, corticopontine tract (CPT) FA decreases have been related to stage 2 of a lately suggested pathological staging system (Kassubek et al., 2014; Müller and Kassubek, 2018). Moreover, a DTI study has shown that disruption of white matter integrity of the cortico-ponto-cerebellar system in ALS patients was associated with the pseudobulbar syndrome, (Floeter et al., 2014). Further, cortico-ponto-cerebellar system microstructural changes have been related to memory impairment in ALS patients, (Trojsi et al., 2020)., Mean FA of the CPT was reduced in ALS patients in a longitudinal multicenter study (Kalra et al., 2020). The CPT is associated with hand dominance-related alterations (Kim et al., 2019). In addition, previous researches have demonstrated that the integrity of the CPT in patients with chronic stroke affects the residual upper limb function (Schulz et al., 2015; Guder et al., 2020). A positive association was observed between the FA of the left FPT and the upper limb sub-score, which concurs with the association between the FA of the CPT and the finger-tapping-score in ALS patients (Kalra et al., 2020). Thus, the identified alterations may contribute to abnormal upper limb function of ALS patients.
However, some limitations should be acknowledged in our study. First, the spatial resolution of DTI in our study was relatively low, and there were still some residual distortions in the brainstem even after the application of correction procedures. These may reduce the interpretability of the results. Therefore, more high-resolution and accurate assessment methods, such as High Angular Resolution Diffusion-weighted Imaging or Diffusion Spectrum Imaging, need to be performed in future studies. Second, the sample size of our patients is relatively small. It is undoubtedly that the limited sample size of a single-center study is challenged by inherent disease heterogeneity in ALS. Therefore, multicenter cooperation is required to solve this problem. In addition, the limitation of this study lies in its cross-sectional nature. Thus, further studies should be performed to assess the changes of longitudinal volume and diffusion in the brainstem of ALS patients.
Conclusion
In summary, the present study provides evidence for brainstem involvement in ALS patients, which is characterized by local atrophy in the medulla oblongata and white matter degeneration in the CST and FPT in the brainstem region. Furthermore, FA reduction in the left CST and FPT may reflect the severity of the disease. These findings may be helpful for further understanding of potential neural mechanisms in ALS.
Data Availability Statement
The original contributions presented in the study are included in the article/Supplementary Material, further inquiries can be directed to the corresponding author/s.
Ethics Statement
The studies involving human participants were reviewed and approved by the Ethics Committee of The First Affiliated Hospital of Xi’an Jiaotong University. The patients/participants provided their written informed consent to participate in this study.
Author Contributions
MZ, HL, and QZ designed the experiments and wrote the manuscript. HL, QD, and QZ carried out the experiment. HL, JJ, and FH performed the acquisition of data. HL, JD, and QZ analyzed and interpreted the data. All authors read and approved the final manuscript.
Funding
This work was supported by the funds of The First Affiliated Hospital of Xi’an Jiaotong University (No. 2018QN-10).
Conflict of Interest
The authors declare that the research was conducted in the absence of any commercial or financial relationships that could be construed as a potential conflict of interest.
Acknowledgments
We would like to acknowledge all participants involved in this study.
Supplementary Material
The Supplementary Material for this article can be found online at: https://www.frontiersin.org/articles/10.3389/fnins.2021.675444/full#supplementary-material
Footnotes
- ^ http://www.fmrib.ox.ac.uk/fsl
- ^ http://surfer.nmr.mgh.harvard.edu
- ^ https://www.nitrc.org/projects/brainstem_atlas/
References
Alshikho, M. J., Zürcher, N. R., Loggia, M. L., Cernasov, P., Reynolds, B., Pijanowski, O., et al. (2018). Integrated magnetic resonance imaging and 11C-PBR28 positron emission tomographic imaging in amyotrophic lateral sclerosis. Ann. Neurol. 83, 1186–1197. doi: 10.1002/ana.25251
Andersson, J. L. R., and Sotiropoulos, S. N. (2016). An integrated approach to correction for off-resonance effects and subject movement in diffusion MR imaging. NeuroImage 125, 1063–1078. doi: 10.1016/j.neuroimage.2015.10.019
Baek, S., Park, J., Kim, Y. H., Seok, H. Y., Oh, K., Kim, H., et al. (2020). Usefulness of diffusion tensor imaging findings as biomarkers for amyotrophic lateral sclerosis. Sci. Rep. 10:5199. doi: 10.1038/s41598-020-62049-0
Bao, Y., Yang, L., Chen, Y., Zhang, B., Li, H., Tang, W., et al. (2018). Radial diffusivity as an imaging biomarker for early diagnosis of non-demented amyotrophic lateral sclerosis. Eur. Radiol. 28, 4940–4948. doi: 10.1007/s00330-018-5506-z
Bastiani, M., Cottaar, M., Fitzgibbon, S. P., Suri, S., Alfaro-Almagro, F., Sotiropoulos, S. N., et al. (2019). Automated quality control for within and between studies diffusion MRI data using a non-parametric framework for movement and distortion correction. Neuroimage 184, 801–812. doi: 10.1016/j.neuroimage.2018.09.073
Bede, P., Chipika, R. H., Finegan, E., Li Hi Shing, S., Doherty, M. A., Hengeveld, J. C., et al. (2019). Brainstem pathology in amyotrophic lateral sclerosis and primary lateral sclerosis: a longitudinal neuroimaging study. Neuroimage Clin. 24:102054. doi: 10.1016/j.nicl.2019.102054
Bede, P., Elamin, M., Byrne, S., McLaughlin, R. L., Kenna, K., Vajda, A., et al. (2013). Basal ganglia involvement in amyotrophic lateral sclerosis. Neurology 81, 2107–2115. doi: 10.1212/01.wnl.0000437313.80913.2c
Braak, H., Brettschneider, J., Ludolph, A. C., Lee, V. M., Trojanowski, J. Q., and Tredici, K. D. (2013). Amyotrophic lateral sclerosis—a model of corticofugal axonal spread. Nat. Rev. Neurol. 9, 708–714. doi: 10.1038/nrneurol.2013.221
Brettschneider, J., Del, T. K., Toledo, J. B., Robinson, J. L., Irwin, D. J., Grossman, M., et al. (2013). Stages of pTDP-43 pathology in amyotrophic lateral sclerosis. Ann. Neurol. 74, 20–38. doi: 10.1002/ana.23937
Broad, R. J., Gabel, M. C., Dowell, N. G., Schwartzman, D. J., Seth, A. K., Zhang, H., et al. (2019). Neurite orientation and dispersion density imaging (NODDI) detects cortical and corticospinal tract degeneration in ALS. J. Neurol. Neurosurg. Psychiatry 90, 404–411. doi: 10.1136/jnnp-2018-318830
Brooks, B. R., Miller, R. G., Swash, M., Munsat, T. L., and World, F. O. N. R. (2000). El Escorial revisited: revised criteria for the diagnosis of amyotrophic lateral sclerosis. Amyotroph. Lateral Scler. Other Motor Neuron Disord. 1, 293–299. doi: 10.1080/146608200300079536
Brown, R. H., and Al-Chalabi, A. (2017). Amyotrophic lateral sclerosis. N. Engl. J. Med. 377, 162–172. doi: 10.1056/NEJMra1603471
Cardenas, A. M., Sarlls, J. E., Kwan, J. Y., Bageac, D., Gala, Z. S., Danielian, L. E., et al. (2017). Pathology of callosal damage in ALS: an ex-vivo, 7 T diffusion tensor MRI study. Neuroimage Clin. 15, 200–208. doi: 10.1016/j.nicl.2017.04.024
Cardenas-Blanco, A., Machts, J., Acosta-Cabronero, J., Kaufmann, J., Abdulla, S., Kollewe, K., et al. (2014). Central white matter degeneration in bulbar- and limb-onset amyotrophic lateral sclerosis. J. Neurol. 261, 1961–1967. doi: 10.1007/s00415-014-7434-4
Chen, G., Taylor, P. A., and Cox, R. W. (2017). Is the statistic value all we should care about in neuroimaging? Neuroimage 147, 952–959. doi: 10.1016/j.neuroimage.2016.09.066
Chiarotto, G. B., Cartarozzi, L. P., Perez, M., Biscola, N. P., Spejo, A. B., Gubert, F., et al. (2019). Tempol improves neuroinflammation and delays motor dysfunction in a mouse model (SOD1(G93A)) of ALS. J. Neuroinflammation 16:218. doi: 10.1186/s12974-019-1598-x
Chiò, A., Calvo, A., Moglia, C., Mazzini, L., and Mora, G. (2011). Phenotypic heterogeneity of amyotrophic lateral sclerosis: a population based study. J. Neurol. Neurosurg. Psychiatry 82, 740–746. doi: 10.1136/jnnp.2010.235952
Chio, A., Pagani, M., Agosta, F., Calvo, A., Cistaro, A., and Filippi, M. (2014). Neuroimaging in amyotrophic lateral sclerosis: insights into structural and functional changes. Lancet Neurol. 13, 1228–1240. doi: 10.1016/S1474-4422(14)70167-X
Cohen-Adad, J., Zhao, W., Keil, B., Ratai, E. M., Triantafyllou, C., Lawson, R., et al. (2013). 7-T MRI of the spinal cord can detect lateral corticospinal tract abnormality in amyotrophic lateral sclerosis. Muscle Nerve 47, 760–762. doi: 10.1002/mus.23720
Consonni, M., Cappa, S. F., Dalla Bella, E., Contarino, V. E., and Lauria, G. (2019). Cortical correlates of behavioural change in amyotrophic lateral sclerosis. J. Neurol. Neurosurg. Psychiatry 90, 380–386. doi: 10.1136/jnnp-2018-318619
Consonni, M., Contarino, V. E., Catricalà, E., Dalla Bella, E., Pensato, V., Gellera, C., et al. (2018). Cortical markers of cognitive syndromes in amyotrophic lateral sclerosis. Neuroimage Clin. 19, 675–682. doi: 10.1016/j.nicl.2018.05.020
Contarino, V. E., Conte, G., Morelli, C., Trogu, F., Scola, E., Calloni, S. F., et al. (2020). Toward a marker of upper motor neuron impairment in amyotrophic lateral sclerosis: a fully automatic investigation of the magnetic susceptibility in the precentral cortex. Eur. J. Radiol. 124:108815. doi: 10.1016/j.ejrad.2020.108815
Espejo-Porras, F., Garcia-Toscano, L., Rodriguez-Cueto, C., Santos-Garcia, I., de Lago, E., and Fernandez-Ruiz, J. (2019). Targeting glial cannabinoid CB2 receptors to delay the progression of the pathological phenotype in TDP-43 (A315T) transgenic mice, a model of amyotrophic lateral sclerosis. Br. J. Pharmacol. 176, 1585–1600. doi: 10.1111/bph.14216
Fattah, M., Raman, M. M., Reiss, A. L., and Green, T. (2020). PTPN11 mutations in the Ras-MAPK signaling pathway affect human white matter microstructure. Cerebral Cortex 31, 1489–1499. doi: 10.1093/cercor/bhaa299
Fischl, B., Liu, A., and Dale, A. M. (2001). Automated manifold surgery: constructing geometrically accurate and topologically correct models of the human cerebral cortex. IEEE Trans. Med. Imaging 20, 70–80. doi: 10.1109/42.906426
Fischl, B., Sereno, M. I., and Dale, A. M. (1999). Cortical surface-based analysis. II: inflation, flattening, and a surface-based coordinate system. Neuroimage 9, 195–207. doi: 10.1006/nimg.1998.0396
Floeter, M. K., Katipally, R., Kim, M. P., Schanz, O., Stephen, M., Danielian, L., et al. (2014). Impaired corticopontocerebellar tracts underlie pseudobulbar affect in motor neuron disorders. Neurology 83, 620–627. doi: 10.1212/WNL.0000000000000693
Geeraert, B. L., Lebel, R. M., Mah, A. C., Deoni, S. C., Alsop, D. C., Varma, G., et al. (2018). A comparison of inhomogeneous magnetization transfer, myelin volume fraction, and diffusion tensor imaging measures in healthy children. Neuroimage 182, 343–350. doi: 10.1016/j.neuroimage.2017.09.019
Gorges, M., Del Tredici, K., Dreyhaupt, J., Braak, H., Ludolph, A. C., Müller, H., et al. (2018). Corticoefferent pathology distribution in amyotrophic lateral sclerosis: in vivo evidence from a meta-analysis of diffusion tensor imaging data. Sci. Rep. 8:15389. doi: 10.1038/s41598-018-33830-z
Grapperon, A., Ridley, B., Verschueren, A., Maarouf, A., Confort-Gouny, S., Fortanier, E., et al. (2019). Quantitative brain sodium MRI depicts corticospinal impairment in amyotrophic lateral sclerosis. Radiology 292, 422–428. doi: 10.1148/radiol.2019182276
Greve, D. N., and Fischl, B. (2009). Accurate and robust brain image alignment using boundary-based registration. Neuroimage 48, 63–72. doi: 10.1016/j.neuroimage.2009.06.060
Guder, S., Frey, B. M., Backhaus, W., Braass, H., Timmermann, J. E., Gerloff, C., et al. (2020). The influence of cortico-cerebellar structural connectivity on cortical excitability in chronic stroke. Cerebral Cortex 30, 1330–1344. doi: 10.1093/cercor/bhz169
Haukedal, H., and Freude, K. (2019). Implications of microglia in amyotrophic lateral sclerosis and frontotemporal dementia. J. Mol. Biol. 431, 1818–1829. doi: 10.1016/j.jmb.2019.02.004
Hutton, J. S., Dudley, J., Horowitz-Kraus, T., DeWitt, T., and Holland, S. K. (2020). Associations between screen-based media use and brain white matter integrity in preschool-aged children. JAMA Pediatr. 174:e193869. doi: 10.1001/jamapediatrics.2019.3869
Iglesias, J. E., Van Leemput, K., Bhatt, P., Casillas, C., Dutt, S., Schuff, N., et al. (2015). Bayesian segmentation of brainstem structures in MRI. Neuroimage 113, 184–195. doi: 10.1016/j.neuroimage.2015.02.065
Ishaque, A., Mah, D., Seres, P., Luk, C., Johnston, W., Chenji, S., et al. (2018). Corticospinal tract degeneration in ALS unmasked in T1-weighted images using texture analysis. Hum. Brain Mapp. 40, 1174–1183. doi: 10.1002/hbm.24437
Jang, S. H., Chang, P., Kim, Y. K., and Seo, J. P. (2014). Anatomical location of the frontopontine fibers in the internal capsule in the human brain. Neuroreport 25, 117–121. doi: 10.1097/WNR.0000000000000076
Jenkinson, M., Beckmann, C. F., Behrens, T. E. J., Woolrich, M. W., and Smith, S. M. (2012). FSL. Neuroimage 62, 782–790. doi: 10.1016/j.neuroimage.2011.09.015
Kalra, S., Müller, H., Ishaque, A., Zinman, L., Korngut, L., Genge, A., et al. (2020). A prospective harmonized multicenter DTI study of cerebral white matter degeneration in ALS. Neurology 95, e943–e952. doi: 10.1212/WNL.0000000000010235
Kang, S. H., Li, Y., Fukaya, M., Lorenzini, I., Cleveland, D. W., Ostrow, L. W., et al. (2013). Degeneration and impaired regeneration of gray matter oligodendrocytes in amyotrophic lateral sclerosis. Nat. Neurosci. 16, 571–579. doi: 10.1038/nn.3357
Kassubek, J., Müller, H., Del Tredici, K., Lulé, D., Gorges, M., Braak, H., et al. (2018). Imaging the pathoanatomy of amyotrophic lateral sclerosis in vivo: targeting a propagation-based biological marker. J. Neurol. Neurosurg. Psychiatry 89, 374–381. doi: 10.1136/jnnp-2017-316365
Kassubek, J., Muller, H. P., Del Tredici, K., Brettschneider, J., Pinkhardt, E. H., Lule, D., et al. (2014). Diffusion tensor imaging analysis of sequential spreading of disease in amyotrophic lateral sclerosis confirms patterns of TDP-43 pathology. Brain 137, 1733–1740. doi: 10.1093/brain/awu090
Kim, Y., Im, S., Kim, S. H., and Park, G. Y. (2019). Laterality of cerebellar afferent and efferent pathways in a healthy right-handed population: a diffusion tensor imaging study. J. Neurosci. Res. 97, 582–596. doi: 10.1002/jnr.24378
Machts, J., Loewe, K., Kaufmann, J., Jakubiczka, S., Abdulla, S., Petri, S., et al. (2015). Basal ganglia pathology in ALS is associated with neuropsychological deficits. Neurology 85, 1301–1309. doi: 10.1212/WNL.0000000000002017
Müller, H., and Kassubek, J. (2018). MRI-based mapping of cerebral propagation in amyotrophic lateral sclerosis. Front. Neurosci. 12:655. doi: 10.3389/fnins.2018.00655
Müller, H., Turner, M. R., Grosskreutz, J., Abrahams, S., Bede, P., Govind, V., et al. (2016). A large-scale multicentre cerebral diffusion tensor imaging study in amyotrophic lateral sclerosis. J. Neurol. Neurosurg. Psychiatry 87, 570–579. doi: 10.1136/jnnp-2015-311952
Palesi, F., De Rinaldis, A., Castellazzi, G., Calamante, F., Muhlert, N., Chard, D., et al. (2017). Contralateral cortico-ponto-cerebellar pathways reconstruction in humans in vivo: implications for reciprocal cerebro-cerebellar structural connectivity in motor and non-motor areas. Sci. Rep. 7:12841. doi: 10.1038/s41598-017-13079-8
Paquin, M. Ê, El Mendili, M. M., Gros, C., Dupont, S. M., Cohen-Adad, J., and Pradat, P. F. (2018). Spinal cord gray matter atrophy in amyotrophic lateral sclerosis. Am. J. Neuroradiol. 39, 184–192. doi: 10.3174/ajnr.A5427
Patenaude, B., Smith, S. M., Kennedy, D. N., and Jenkinson, M. (2011). A Bayesian model of shape and appearance for subcortical brain segmentation. Neuroimage 56, 907–922. doi: 10.1016/j.neuroimage.2011.02.046
Qiao, J., Zhao, X., Wang, S., Li, A., Wang, Z., Cao, C., et al. (2020). Functional and structural brain alterations in encephalitis with LGI1 antibodies. Front. Neurosci. 14:304. doi: 10.3389/fnins.2020.00304
Rahayel, S., Bocti, C., Sévigny Dupont, P., Joannette, M., Lavallée, M. M., Nikelski, J., et al. (2019). Subcortical amyloid load is associated with shape and volume in cognitively normal individuals. Hum. Brain Mapp. 40, 3951–3965. doi: 10.1002/hbm.24680
Rasoanandrianina, H., Grapperon, A. M., Taso, M., Girard, O. M., Duhamel, G., Guye, M., et al. (2017). Region-specific impairment of the cervical spinal cord (SC) in amyotrophic lateral sclerosis: a preliminary study using SC templates and quantitative MRI (diffusion tensor imaging/inhomogeneous magnetization transfer). NMR Biomed. 30:e3801. doi: 10.1002/nbm.3801
Reuter, M., Rosas, H. D., and Fischl, B. (2010). Highly accurate inverse consistent registration: a robust approach. Neuroimage 53, 1181–1196. doi: 10.1016/j.neuroimage.2010.07.020
Rueckert, D., Sonoda, L. I., Hayes, C., Hill, D. L. G., Leach, M. O., and Hawkes, D. J. (1999). Nonrigid registration using free-form deformations: application to breast MR images. IEEE Trans. Med. Imaging 18, 712–721. doi: 10.1109/42.796284
Schönecker, S., Neuhofer, C., Otto, M., Ludolph, A., Kassubek, J., Landwehrmeyer, B., et al. (2018). Atrophy in the thalamus but not cerebellum is specific for C9orf72 FTD and ALS patients : an atlas-based volumetric MRI study. Front. Aging Neurosci. 10:45. doi: 10.3389/fnagi.2018.00045
Schulz, R., Frey, B. M., Koch, P., Zimerman, M., Bönstrup, M., Feldheim, J., et al. (2015). Cortico-cerebellar structural connectivity is related to residual motor output in chronic stroke. Cerebral Cortex 27, 635–645. doi: 10.1093/cercor/bhv251
Schuster, C., Elamin, M., Hardiman, O., and Bede, P. (2016). The segmental diffusivity profile of amyotrophic lateral sclerosis associated white matter degeneration. Eur. J. Neurol. 23, 1361–1371. doi: 10.1111/ene.13038
Segonne, F., Pacheco, J., and Fischl, B. (2007). Geometrically accurate topology-correction of cortical surfaces using nonseparating loops. IEEE Trans. Med. Imaging 26, 518–529. doi: 10.1109/TMI.2006.887364
Senda, J., Atsuta, N., Watanabe, H., Bagarinao, E., Imai, K., Yokoi, D., et al. (2017). Structural MRI correlates of amyotrophic lateral sclerosis progression. J. Neurol. Neurosurg. Psychiatry 88, 901–907. doi: 10.1136/jnnp-2016-314337
Smith, S. M. (2002). Fast robust automated brain extraction. Hum. Brain Mapp. 17, 143–155. doi: 10.1002/hbm.10062
Smith, S. M., Jenkinson, M., Woolrich, M. W., Beckmann, C. F., Behrens, T. E. J., Johansen-Berg, H., et al. (2004). Advances in functional and structural MR image analysis and implementation as FSL. Neuroimage 23, S208–S219. doi: 10.1016/j.neuroimage.2004.07.051
Smith, S. M., and Nichols, T. E. (2009). Threshold-free cluster enhancement: addressing problems of smoothing, threshold dependence and localisation in cluster inference. Neuroimage 44, 83–98. doi: 10.1016/j.neuroimage.2008.03.061
Smith, S. M., Zhang, Y., Jenkinson, M., Chen, J., Matthews, P. M., Federico, A., et al. (2002). Accurate, robust, and automated longitudinal and cross-sectional brain change analysis. Neuroimage 17, 479–489. doi: 10.1006/nimg.2002.1040
Sorrentino, P., Rucco, R., Jacini, F., Trojsi, F., Lardone, A., Baselice, F., et al. (2018). Brain functional networks become more connected as amyotrophic lateral sclerosis progresses: a source level magnetoencephalographic study. Neuroimage Clin. 20, 564–571. doi: 10.1016/j.nicl.2018.08.001
Tang, Y., Sun, W., Toga, A. W., Ringman, J. M., and Shi, Y. (2018). A probabilistic atlas of human brainstem pathways based on connectome imaging data. Neuroimage 169, 227–239. doi: 10.1016/j.neuroimage.2017.12.042
Trojsi, F., Caiazzo, G., Corbo, D., Piccirillo, G., Cristillo, V., Femiano, C., et al. (2015). Microstructural changes across different clinical milestones of disease in amyotrophic lateral sclerosis. PLoS One 10:e119045. doi: 10.1371/journal.pone.0119045
Trojsi, F., Di Nardo, F., Caiazzo, G., Siciliano, M., D Alvano, G., Ferrantino, T., et al. (2020). Hippocampal connectivity in Amyotrophic Lateral Sclerosis (ALS): more than Papez circuit impairment. Brain Imaging Behav. doi: 10.1007/s11682-020-00408-1 [Epub ahead of print].
Tu, S., Menke, R. A. L., Talbot, K., Kiernan, M. C., and Turner, M. R. (2018). Regional thalamic MRI as a marker of widespread cortical pathology and progressive frontotemporal involvement in amyotrophic lateral sclerosis. J. Neurol. Neurosurg. Psychiatry 89, 1250–1258. doi: 10.1136/jnnp-2018-318625
van Es, M. A., Hardiman, O., Chio, A., Al-Chalabi, A., Pasterkamp, R. J., Veldink, J. H., et al. (2017). Amyotrophic lateral sclerosis. Lancet 390, 2084–2098. doi: 10.1016/S0140-6736(17)31287-4
van Veluw, S. J., Reijmer, Y. D., van der Kouwe, A. J., Charidimou, A., Riley, G. A., Leemans, A., et al. (2019). Histopathology of diffusion imaging abnormalities in cerebral amyloid angiopathy. Neurology 10–1212. doi: 10.1212/WNL.0000000000007005
Vanderah, T., and Gould, D. J. (2015). Nolte’s The Human Brain E-Book: An Introduction to its Functional Anatomy. Philadelphia: Elsevier Health Sciences.
Volonté, C., Amadio, S., Fabbrizio, P., and Apolloni, S. (2019). Functional microglia neurotransmitters in amyotrophic lateral sclerosis. Semin. Cell Dev. Biol. 94, 121–128. doi: 10.1016/j.semcdb.2019.04.014
Wagner, M. J., and Luo, L. (2020). Neocortex-cerebellum circuits for cognitive processing. Trends Neurosci. 43, 42–54. doi: 10.1016/j.tins.2019.11.002
Walhout, R., Westeneng, H. J., Verstraete, E., Hendrikse, J., Veldink, J. H., van den Heuvel, M. P., et al. (2015). Cortical thickness in ALS: towards a marker for upper motor neuron involvement. J. Neurol. Neurosurg. Psychiatry 86, 288–294. doi: 10.1136/jnnp-2013-306839
Wang, C., Foxley, S., Ansorge, O., Bangerter-Christensen, S., Chiew, M., Leonte, A., et al. (2020). Methods for quantitative susceptibility and R2∗ mapping in whole post-mortem brains at 7T applied to amyotrophic lateral sclerosis. Neuroimage 222:117216. doi: 10.1016/j.neuroimage.2020.117216
Winkler, A. M., Ridgway, G. R., Webster, M. A., Smith, S. M., and Nichols, T. E. (2014). Permutation inference for the general linear model. Neuroimage 92, 381–397. doi: 10.1016/j.neuroimage.2014.01.060
Zhang, F., Chen, G., He, M., Dai, J., Shang, H., Gong, Q., et al. (2018). Altered white matter microarchitecture in amyotrophic lateral sclerosis: a voxel-based meta-analysis of diffusion tensor imaging. Neuroimage Clin. 19, 122–129. doi: 10.1016/j.nicl.2018.04.005
Zheng, H., Bergamino, M., Ford, B. N., Kuplicki, R., Yeh, F., Bodurka, J., et al. (2021). Replicable association between human cytomegalovirus infection and reduced white matter fractional anisotropy in major depressive disorder. Neuropsychopharmacology (New York, N.Y.) 46, 928–938. doi: 10.1038/s41386-021-00971-1
Keywords: amyotrophic lateral sclerosis, magnetic resonance imaging, diffusion tensor imaging, shape analysis, brainstem
Citation: Li H, Zhang Q, Duan Q, Jin J, Hu F, Dang J and Zhang M (2021) Brainstem Involvement in Amyotrophic Lateral Sclerosis: A Combined Structural and Diffusion Tensor MRI Analysis. Front. Neurosci. 15:675444. doi: 10.3389/fnins.2021.675444
Received: 03 March 2021; Accepted: 12 April 2021;
Published: 02 June 2021.
Edited by:
Francesca Trojsi, University of Campania Luigi Vanvitelli, ItalyReviewed by:
Marcondes C. França Jr., State University of Campinas, BrazilAntonietta Canna, University of Salerno, Italy
Copyright © 2021 Li, Zhang, Duan, Jin, Hu, Dang and Zhang. This is an open-access article distributed under the terms of the Creative Commons Attribution License (CC BY). The use, distribution or reproduction in other forums is permitted, provided the original author(s) and the copyright owner(s) are credited and that the original publication in this journal is cited, in accordance with accepted academic practice. No use, distribution or reproduction is permitted which does not comply with these terms.
*Correspondence: Ming Zhang, zhangming01@xjtu.edu.cn