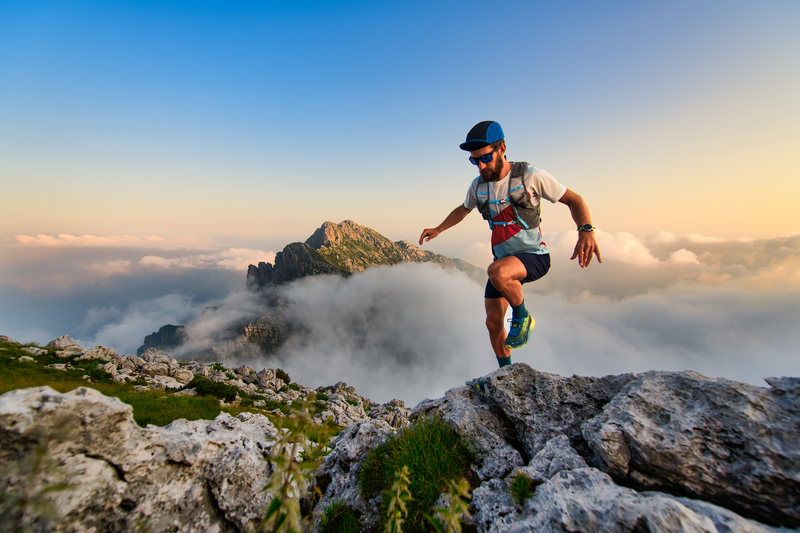
95% of researchers rate our articles as excellent or good
Learn more about the work of our research integrity team to safeguard the quality of each article we publish.
Find out more
ORIGINAL RESEARCH article
Front. Neurosci. , 07 June 2021
Sec. Neurogenomics
Volume 15 - 2021 | https://doi.org/10.3389/fnins.2021.661663
This article is part of the Research Topic Bioinformatic tools for the detection and identification of mechanisms in immune cells of neuroimmunological diseases View all 9 articles
Intracerebral hemorrhage (ICH) is a dangerous neurological disease. The mechanism of ferroptosis in ICH remains unclear. Using bioinformatics analysis, we aimed to identify the key molecules involved in ferroptosis and provide treatment targets for ICH to further explore the mechanism of ferroptosis in ICH. GSE24265 was downloaded from the Gene Expression Omnibus (GEO) dataset and intersected with ferroptosis genes. A total of 45 differentially expressed genes (DEGs) were selected, most of which were involved in the TNF signaling pathway and oxidative stress response. Key modules constructed by the protein–protein interaction (PPI) network analysis and screening of genes related to the TNF signaling pathway led to the confirmation of the following genes of interest: MAPK1, MAPK8, TNFAIP3, ATF4, and SLC2A1. Moreover, MAPK1 was one of the key genes related to TNF signaling and oxidative stress, and it may play an important role in ferroptosis after cerebral hemorrhage. The MAPK1-related molecules included hsa-miR-15b-5P, hsa-miR-93-5P, miR-20b-5p, SNHG16, XIST, AC084219.4, RP11-379K17.11, CTC-444N24.11, GS1-358P8.4, CTB-89H12.4, RP4-773N10.5, and FGD5-AS1. We also generated a hemorrhage rat model, which was used to conduct exercise intervention in ICH rats, and qRT-PCR was used to assess the expression levels of our genes of interest. The mRNA levels after cerebral hemorrhage showed that MAPK1, ATF4, SLC2A1, and TNFAIP3 were upregulated, whereas MAPK8 was downregulated. Treadmill training increased the expression of anti-inflammatory molecules TNFAIP3 and SLC2A1 and reduced the expression of MAPK1, ATF4, and MAPK8, indicating that treadmill training may be utilized as antioxidant therapy to decrease neuronal ferroptosis. The results of this study indicated that the MAPK1-related mRNA–miRNA–lncRNA interaction chain could be potentially employed as a biomarker of the inception and progression of ferroptosis after cerebral hemorrhage.
Intracerebral hemorrhage (ICH) is a disease associated with high mortality, disability, and dysfunction (Qureshi et al., 2001). The pathological accumulation of blood in the brain parenchyma occurs due to a sudden rupture of a blood vessel. Brain damage after ICH develops due to the growth of hematoma, which leads to the destruction of brain tissue and mechanical deformation (Xi et al., 2006). In addition, brain damage can occur due to mitochondrial dysfunction, microglia activation, disorders of neurotransmitters, and release of inflammatory mediators (Kim-Han et al., 2006). A number of factors are triggered after ICH, resulting in hematoma and deep brain cell death. The loss of nerve cells is a major cause of mortality in ICH patients. Programmed cell death plays an important role in cerebral hemorrhage (Bobinger et al., 2018). Ferroptosis, a programmed cell death-like process, has been identified after ICH, but the mechanism of ferroptosis in cerebral hemorrhage remains unclear.
Ferroptosis was first described by Stockwell in 2012 as a type of cell death that differs from apoptosis, and its distinguishing feature is iron-dependent accretion of lipid peroxidation by reactive oxygen species (ROS) (Dixon et al., 2012). Recent studies have revealed that this process is related to the cell death mechanisms involved in ICH (Bao et al., 2020). It has been found that the expression levels of some key molecules change after ferroptosis. In particular, some studies have revealed that the level of phospho-mitogen-activated protein kinase 1 (p-MAPK1) significantly increases after ICH, and the MAPK/ERK pathway is involved in ferroptosis regulation of ICH (Chen S. et al., 2019; Su et al., 2019). With respect to the downstream mediators of ferroptosis, a previous study found that cyclooxygenase 2 (COX-2), which is encoded by the PTGS-2 gene, significantly increased in mice after ICH, and it was inhibited by fe-1 (Chen B. et al., 2019). Activating transcription factor 4 (ATF4) is activated in the ferroptosis-induced transcriptional response in neurons, and the neurons of the ATF4 deletion germline are resistant to homocysteine-induced ferroptosis (Karuppagounder et al., 2016; Chen et al., 2017). However, many ferroptosis genes have not yet been found, so further studies of ferroptosis-related genes are needed.
At present, there have been no bioinformatics-based studies on the mechanism of ferroptosis genes after ICH. Therefore, we used data mining and data analysis techniques to screen differentially expressed genes (DEGs) in perihematomal tissue (PH) and contralateral normal tissue of ICH patients. These DEGs were then intersected with the ferroptosis dataset to obtain ferroptosis DEGs. Moreover, to identify crucial biomarkers and establish the pathogenesis of ICH at the molecular level, we investigated key miRNAs and lncRNAs that may play principal roles in ICH. We also constructed a rat ICH model and an intervention model using treadmill training to test the above-mentioned hypothesis. Our results will help to understand ferroptosis after ICH and provide new thoughts for clinical diagnosis and treatment of ICH.
Adult male Sprague–Dawley rats (2 months old) were purchased from Qingdao Peng Yue Animal Husbandry Co., Ltd., and kept under a 12-h light/dark cycle in the same colony room, with controlled temperature and humidity. The animals were randomly assigned into the following three groups: (1) sham (n = 7): rats that underwent surgery without ICH; (2) ICH (n = 7): the ICH group, animals that received autologous blood injection; and (3) ICH+ early exercise (n = 7): rats that received additional treadmill training after ICH. The animal study was reviewed and approved by the Ethics Committee of the Affiliated Hospital of Qingdao.
As in previous studies, cerebral hemorrhage was induced in the right caudate nucleus (Wu et al., 2016). The rats were anesthetized with isoflurane and fixed in a stereotaxic apparatus. A hole was perforated 4.0 mm laterally to the median line of the brain and 0.1 mm anterior to the bregma, and 1.5 μl saline mixed with 0.38U of bacterial collagenase (type IV; Sigma-Aldrich, St. Louis, MO, United States) was injected via a 5.0-mm needle below the plane of the cranial bones.
The exercise program used throughout the study was slightly adjusted compared with the method used in previous studies (Tamakoshi et al., 2018). In short, 3 days before the operation, the rats were acclimated to the treadmill training environment for 20 min at 1–2 m/min. We ensured that the rats were able to carry out normal exercise training in the next exercise protocol and excluded the rats that did not participate in the training. On postoperative day 2, the ICH group did not carry out treadmill training and lived in the above-mentioned conventional feeding environment for the next 2 weeks. In contrast, the ICH+ early exercise group was subjected to the training for 14 days. The training time was set to 30 min/day, with the training speed set at 6 M/min on the first day and 10 M/min thereafter.
The clinical information of patients with cerebral hemorrhage was obtained from Gene Expression Omnibus (GEO)1. We downloaded the dataset GSE24265 stored by Rosell from the GEO (Rosell et al., 2011). This dataset includes microarray data of perihematoma tissues and contralateral corresponding parts (white matter and gray matter) of four patients with ICH (Liu et al., 2019). This dataset was used for further analysis and mining. In this experiment, the microarray data of 11 samples, including perihematomal tissue (PH) and the corresponding contralateral white and gray matter, were obtained from public databases, so the consent of patients and ethics committee approval were unnecessary.
GEO2R, an online analysis tool, was used to perform the analysis of differential expression (Barrett et al., 2013). The expression profiles of PH and normal tissue were compared to identify the DEGs. T test was employed to determine p-values and adjusted p-values in the differential gene expression (DGE) analysis.
Genes retained from diverse tissues were selected using the following criteria: a | log2 (fold-change)| > 1 and adjusted P-value < 0.05. We also obtained a dataset that included 265 genes from the Ferroptosis Database (FerrDb; zhounan.org) and intersected it with GSE24265 to identify ferroptosis DEGs. The online tool Venny2.1 was employed to generate a Venn diagram of DEGs, and a heat map of DEGs was drawn using Heml Software.
Functional enrichment analysis of DEGs was performed using DAVID 6.8, Metascape, and WebGestalt. These different enrichment analysis tools have different algorithms, which can play the role of mutual verification. Gene set enrichment analysis (GSEA) is a method to sort genes according to the degree of differential expression of two samples (Subramanian et al., 2005). It is a method to analyze the whole genome expression profile chip data and compare genes with predefined gene sets (Debrabant, 2017). We uploaded the ferroptosis DEGs to the GSEA of WebGestalt for further study. The GSEA of WebGestalt first filtered the gene sets according to the number of genes contained, with a minimum number of seven genes and a maximum number of 2,000 genes per gene set by default. Kyoto Encyclopedia of Genes and Genomes (KEGG) analysis was obtained from the GSEA of WebGestalt. A common enrichment tool, David v6.8, was also used for enrichment analysis, focusing on the KEGG pathway analysis and KEGG enrichment analysis to find the pathways where the target gene is involved and analyze the significance of each pathway. We uploaded the obtained ferroptosis DEGs in Metascape, an online tool for gene function annotation analysis. Annotation of biological processes was performed by Metascape, using the genes that were shared between GSE24265 and the ferroptosis dataset. Moreover, the biological pathways of miRNAs were analyzed in an enrichment analysis tool, Funrich. The difference was statistically significant at P < 0.05.
To predict protein–protein interactions (PPIs), STRING, an online database which can retrieve the interaction between a group of proteins, was utilized in the PPI network analysis (Szklarczyk et al., 2017). Cytoscape network visualization was obtained with interaction scores > 0.4. The nodes represented genes, and the edges represented the links between the genes. In addition, the PPI network was built and visualized by Cytoscape v3.6.0 software.
Molecular complex detection (MCODE) was used for clustering analysis of gene networks to identify key PPI network modules (Bader and Hogue, 2003). The function of MCODE is to select the key sub-networks, i.e., modules. A PPI module refers to a PPI module where one module points to one function. In a module, different genes have different module scores, and key genes can be selected according to the scores. In order to identify the key modules, P < 0.05 was considered to show a significant difference.
In addition to the abovementioned analytical tools, we also used miRWalk 2.0 to predict targeted pivotal miRNAs and build the gene–miRNA interaction networks that are related to cluster 1 (Dweep et al., 2011). We intersected the predicted results of the MiRTarBase and miRWalk database to ensure the accuracy of the results. We screened miRNAs that target more than two genes.
lncRNAs, which are upstream molecules of miRNAs, were identified using StarBase v2.0 (Dweep et al., 2011). We selected the cross-linked diagram showing the interaction of the calculated results of every miRNA to confirm relevant lncRNAs.
On postoperative day 15 (sham: n = 7; ICH: n = 7; ICH + early exercise: n = 7), PH was extracted, and qRT-PCR was performed. Briefly, total RNA was collected from the PH of rats using TRIzol. RNA purity was tested using Quantus Fluorometer. First, total RNA was used for reverse transcription reaction. Then, the pre-amplified cDNA samples were mixed with One-Step SYBR PrimeScript PLUS RTPCR Kit. Finally, the reaction was conducted on AriaMx HRM. GAPDH was used as a positive control, and the comparative Ct method was used to calculate each sample (Table 1).
GraphPad Prism 7.0 software was used to draw graphics and perform statistical analysis. All of the data are shown as mean ± standard deviation (SD). One-way analysis of variance (ANOVA) was used for statistical analysis of sham, ICH, and ICH + early exercise groups, followed by Tukey’s multiple-comparisons post hoc tests. A t-test was employed to determine p-values and adjusted p-values in the DGE analysis, where p-values were adjusted by FDR. The difference was statistically significant at the level of P < 0.05.
GSE24265, the microarray expression profiling dataset, was downloaded from the GEO database, and DEGs were obtained by comparing PH and the corresponding gray and contralateral white matter. We also gained the dataset including 265 genes from the Ferroptosis Database (FerrDb) and intersected them with GSE24265 to identify ferroptosis DEGs. We found altogether 40 upregulated and five downregulated genes (Table 2). The heat map and Venn diagram of the DEGs are shown in Figure 1. The DEGS were further classified as ferroptosis driver, ferroptosis suppressor, and ferroptosis marker via the FerrDb online tool (Table 3).
Figure 1. (A) There were 1,327 differentially expressed genes (DEGs) in perihematomal tissue and normal tissues. The first 50 differentially expressed genes were shown in the heat map, with red representing significantly up-regulated genes and blue representing significantly down-regulated genes in the samples. (B) Venn diagram of ferroptosis differentially expressed genes. We intersected ferroptosis dataset with GSE24265 to identify ferroptosis DEGs.
Table 3. The ferroptosis differentially expressed genes were divided into ferroptosis driver, suppressor, and marker.
Pathway enrichment analyses of DEGs were performed using the online tools Metascape, WebGestalt, and DAVID. First, we uploaded the related information of DEGs in the PH samples and normal samples to the WebGestalt software. The result of the enrichment gene dataset analysis indicated that the genes significantly enriched were involved in the TNF signaling pathway, MAPK signaling pathway, fluid shear stress, atherosclerosis, and Kaposi’s sarcoma-associated herpesvirus infection (Figure 2). DAVID was utilized to analyze the biological pathways and processes of 45 DEGs in ICH samples. KEGG functional analysis revealed that the TNF signaling pathway, HIF-1 signaling pathway, MAPK signaling pathway, and NOD-like receptor signaling pathway were significantly activated in the gene sets (Figure 3). Second, 45 DEGs were then uploaded to Metascape, and it was shown that the biological processes were remarkably enriched in response to oxidative stress, multicellular organismal homeostasis RNA polymerase II promoter in response to stress, and cellular response to stress. The biological process was significantly activated in response to stress. The biological pathway was significantly activated in the TNF signaling pathway, fluid shear stress, atherosclerosis, and Kaposi’s sarcoma-associated herpesvirus infection (Figure 4). Notably, the TNF signaling pathway was the major biological pathway involved and was identified by Metascape, WebGestalt, and DAVID.
Figure 2. The result of the enrichment gene dataset analysis indicated that the genes significantly enriched were involved in the TNF signaling pathway, MAPK signaling pathway, fluid shear stress, atherosclerosis, and Kaposi’s sarcoma-associated herpesvirus infection. Gene set enrichment analysis of WebGestalt first filtered the gene set according to the number of genes contained in the gene set, with a minimum number of seven genes and a maximum number of 2,000 genes by default.
Figure 3. Top eight biological pathways were selected and shown according to enrichment score. The TNF signaling pathway was significantly enriched.
Figure 4. (A) Network of enriched terms. (B) The Metascape drew a bar chart of 20 biological pathways based on the P-value and the percentage of genes, among which biological pathways with P-value < 0.01 are statistically significant. The results showed that the biological processes that were significantly enriched were in response to oxidative stress.
We obtained the PPI network containing 40 nodes and 330 edges. Five of the 45 genes were not related to other molecules and did not form a molecular network. The network was set to the default cutoff (interaction score > 0.4) in the STRING online database. Genes were represented by nodes, and the interactions between the genes were indicated by edges. The upregulated genes were marked in blue, while the downregulated genes were labeled in red. MCODE, the application from Cytoscape, was used for clustering analysis of gene networks to draw the key modules (Table 4). Two key modules with one downregulated gene (MAPK8) and 17 upregulated genes were established (Figure 5). These 18 genes were the key genes screened by MCODE. Genes of interest were selected from the genes identified by key module analyses involved in the TNF pathways with the highest MCODE scores, including MAPK1, MAPK8, TNFAIP3, ATF4, and SLC2A1. Furthermore, the functional analysis for cluster 1 using Metascape showed that these 18 genes were mainly involved in multicellular organismal homeostasis, TNF signaling pathway, and inorganic substance (Figure 6).
Table 4. Molecular complex detection was used to process the data downloaded from the STRING to further mining gene clusters.
Figure 5. (A) Cytoscape network visualization of the 40 nodes and 330 edges that were obtained with interaction scores > 0.4 according to the STRING online database. The nodes represent genes and the edges represent links between genes. Red represents downregulated genes, and blue represents upregulated genes. Two key modules were identified by MCODE, which was used to identify network gene clustering. (B) Cluster 2. (C) Cluster 1.
Figure 6. Functional enrichment analysis of cluster 1. The TNF signaling pathway was significantly enriched.
We screened 13 genes in cluster 1 and performed gene–miRNA analysis by using miRWalk 2.0 software. The cross-linked miRNAs were selected by miRWalk and miRTarBase databases to ensure the accuracy and reliability of our results. The following criteria were used in filtering the results: P < 0.05, seed sequence lengths > 7, and 3′UTR as the target gene-binding regions (Figure 7). The miRNAs with higher amounts of cross-linked genes (≥2) are shown in Table 5. A total of 300 miRNA expression genes were uploaded to Funrich, and the results of the enrichment analysis indicated that the molecular function was significantly enriched in transcription factor activity, protein serine kinase activity, and receptor binding. The biological pathways enriched included the TRAIL signaling pathway, glypican pathway, and proteoglycan syndecan-mediated signaling events (Figure 8).
Figure 7. Interaction network between genes of cluster 1 and its targeted miRNAs. Genes were colored in blue, miRNAs were colored in orange, and the cross-linked genes were colored in green.
Figure 8. (A) The molecular function of cluster 1-related miRNAs was significantly enriched in the transcription factor activity, protein serine kinase activity, and receptor binding. (B) Biological pathways were enriched in the TRAIL signaling pathway, Glypican pathway, and proteoglycan syndecan-mediated signaling events.
Nine miRNAs had higher amounts of cross-linked genes (≥2). Only hsamiR-15b-5P, hsa-miR-93-5P, and miR-20b-5p had the upstream molecule lncRNA and had higher reliability, all targeting MAPK1. The corresponding lncRNAs of hsa-miR-15b-5P, hsa-miR-93-5P, and miR-20b-5p were predicted with StarBase 2.0. The highest reliability (very high stringency, >5) was selected as the criterion. After cross-linking, nine lncRNAs targeting three key miRNAs were revealed, namely, SNHG16, XIST, AC084219.4, RP11-379K17.11, CTC-444N24.11, GS1-358P8.4, CTB-89H12.4, RP4-773N10.5, and FGD5-AS1.
Five genes were verified. They had high reliability and were mostly related to TNF signaling and response to stress. SLC2A1, TNFAIP3, ATF4, MAPK1, and MAPK8 were identified by MCODE as the genes with high MCODE score in the two clusters. Then, the filtered biomarkers, including MAPK1, MAPK8, TNFAIP3, ATF4, and SLC2A1, were verified in ICH samples using qRT-PCR. The results indicated that the expression levels of MAPK1, ATF4, SLC2A1, and TNFAIP3 were visibly higher and that the expression of MAPK8 was lower in ICH rats than in the sham controls. After the exercise training, MAPK1, MAPK8, and ATF4 showed low expression levels compared with the ICH group. In contrast, TNFAIP3 and SLC2A1 were upregulated by exercise training (Figure 9).
Figure 9. qRT-PCR results show that the expression levels of MAPK1 (P-value = 0.011), SLC2A1 (P-value = 0.0002), TNFAIP3 (P-value < 0.0001), and ATF4 (P-value = 0.0022) were obviously higher, and MAPK8 (P-value = 0.0003) was lower in intracerebral hemorrhage rats than that of healthy controls. After treadmill training, SLC2A1 (P-value = 0.0087) and TNFAIP3 (P-value < 0.0028) were obviously upregulated, and ATF4 (P-value = 0.0468), MAPK8 (P-value = 0.0017), and MAPK1 (P-value = 0.0408) were downregulated by comparing the treatment group and the model groups.
The present study identified the key genes involved in ferroptosis and further explored the mechanisms of ferroptosis in ICH. Our study obtained 45 DEGs, including six downregulated genes and 39 upregulated genes, from the intersection of DEGs of the datasets GSE24265 and FerrDb. Then, pathway enrichment analyses of DEGs were performed using the online tools Metascape, GSEA, and DAVID, and the results indicated that these genes are mainly involved in stress responses and the TNF signaling pathway. The changes in ferroptosis gene expression after ICH followed by treadmill training were identified, suggesting that exercise may influence ferroptosis in the treatment of ICH and proving the reliability of the bioinformatics analysis. Moreover, the regulatory network of MAPK1 molecules can affect the TNF signaling pathway after ICH. The study also identified several genes that had not yet been mentioned in the area of ICH and ferroptosis. This study may provide effective reference for the pathological mechanism of ICH from the perspective of bioinformatics analysis.
Ferroptosis is characterized by the intracellular collection of lipid ROS, which are closely related and eventually result in the oxidation of lipids, thereby leading to cell membrane injury and cell death. Ferroptosis is associated with oxidative stress produced by the undue collection of ROS during aerobic metabolism (Dixon et al., 2012). After oxidative stress, some signaling pathways are activated, such as the MAPK pathway. According to previous studies, the MAPK pathway and the NF-κB pathway may induce the production of free radicals after ICH, which can induce ferroptosis and apoptosis; in that context, antioxidant therapy can decrease the ferroptosis of neurons as well as apoptosis after ICH (Haddad and Land, 2002; Speer et al., 2013). A previous study confirmed that the MAPK pathway is activated following iron accumulation, and the suppression of MAPK activation improves functional results and reduces neuronal cell death (Yu and Richardson, 2011). Therefore, together with the induction of ferroptosis, the MAPK signaling pathway and the NF-κB pathway might be activated to promote the generation of ROS, aggravating cell injury and resulting in a vicious circle.
The TNF signaling pathway plays a significant role in inflammation and oxidative stress (Fischer and Maier, 2015). In the binding of TNF to TNFR1, the signaling complex I adjusts the NF-κB pathway via the activation of the IKK complex, causing the translocation of NF-κB to the nucleus, which changes the expression of cell adhesion molecules, proinflammatory cytokines, and ROS-generating enzymes (Walczak, 2011). In contrast, TNFR2 does not possess a death domain and thus indirectly leads to cell death. Signaling pathways activated by TNFR2 include the NF-κB and JNK pathways, canonical and non-canonical (Cabal-Hierro et al., 2014). TNFα/TNFR2 signaling can regulate the enzyme TNFAIP3/A20 to reduce IL-17A expression in conventional T cells, thereby exhibiting an anti-inflammatory effect (Urbano et al., 2019). Therefore, the TNF signaling pathway is also a double-edged sword and has potential research value. A previous experiment in mice showed that TNF-α treatment notably raised the level of glutamate, resulting in stress in astrocytes during neuroinflammation (Wang et al., 2017). Neuroinflammation through glutamate toxicity is considered an important target in the pathophysiology of ICH-induced nervous system injury (Shah et al., 2016). Glutamate toxicity closely involves ferroptosis. Therefore, the TNF signaling pathway may play an important role in ferroptosis following hemorrhage stroke.
In order to test and verify our hypothesis, enrichment analyses containing modular enrichment analysis and gene sets were performed by different bioinformatics analysis tools. DAVID and GSEA of WebGestalt revealed that the TNF signaling pathway was significantly enriched by KEGG pathway analysis, and there were seven genes correlating with this pathway, namely, IL6, MAPK8, TNFAIP3, MAPK1, PTGS2, CXCL2, and ATF4. The Metascape analysis also revealed that oxidative stress was markedly activated in the Gene Ontology process, and there were 15 genes associated with this pathway, namely, ATF4, CRYAB, DUSP1, GCH1, HBA1, HMOX1, HSPB1, IL6, NCF2, PRKAA2, MAPK1, MAPK8, PTGS2, TNFAIP3, and SESN2.
The genes of interest were selected from the genes identified by the key module analyses involved in the TNF pathway with the highest MCODE scores, including MAPK1, MAPK8, TNFAIP3, ATF4, and SLC2A1. MAPK1 is a serine/threonine kinase that plays important roles in several cellular processes. It has been shown that P-MAPK1 expression considerably increased and promoted neuroinflammation after ICH (Haddad and Land, 2002). The suppression of ferroptosis ameliorated hemoglobin-induced cell death by inhibiting MAPK/ERK1 (Codenotti et al., 2018). MAPK8, which is also known as C-JUN N-terminal kinase (JNK), is a member of the MAPK signaling pathway. A previous study on ICH suggested that the JNK pathway could be initiated, with upregulation of pJNK (Hibi et al., 1993; Pei et al., 2016). Other studies indicated that JNK is involved in ferroptotic cell death in mouse cortex and PC-12 cells (Qin et al., 2020). TNFAIP3, an important anti-inflammatory factor, can repress the expression of inflammatory NF-κB signaling and promote cell survival (Catrysse et al., 2014). A previous study suggested that TNFAIP3 could prevent ICH-caused damaging inflammation of the brain. However, TNFAIP3 overexpression increased oxygen free radical generation and enhanced ferroptosis induced by erastin (Meng et al., 2017; Xiao et al., 2019). In the nervous system, ATF4 is a key redox-regulated, pro-death transcriptional activator that propagates death responses to oxidative stress in stroke (Ameri and Harris, 2008). It has been shown that ATF4 increases the expression of transcriptional activator CHOP (DDIT3) and caspase-12, which promote apoptosis and reduce neuronal survival after brain hemorrhage (Wu et al., 2020). Upregulated ATF4 increases the expression of HSPA5 and activity of glutathione peroxidase 4 (GPX4), thus protecting glioma cells from ferroptosis (Chen Y. et al., 2019). Insufficiency of the SLC2A1 gene and the weakness of its translated product, glucose transporter-1 (Glut1) protein, affect glucose uptake, disrupt brain function, and cause neurodevelopmental disorders (Veys et al., 2020). A previous study demonstrated that lymphoid-specific helicase inhibited ferroptosis, partly through Glut1 (Jiang et al., 2017). These data suggest that the changes in MAPK1, MAPK8, TNFAIP3, ATF4, and SLC2A1 gene expression may be the basis for the increased incidence of ferroptosis after ICH.
miRNAs are endogenous non-coding RNA molecules that target the 3′UTR region of a gene and can regulate gene expression to degrade or inhibit the translation of the target gene (Sun et al., 2015). In our study, we identified nine miRNAs targeting at least two genes. However, among these nine miRNAs, only hsa-miR-15b-5p, hsa-miR-93-5p, and miR-20b-5p can forecast the relevant upstream lncRNAs. In previous studies, miR-20b-5p and miR-15b-5p in ICH were limited, while miR-15b-5p was upregulated in meningitis (de Queiroz et al., 2018). miR-93-5p is significantly downregulated in ICH (Cheng et al., 2020). Our results indicated that the mRNA–miRNA–lncRNA connection chain may exist in patients with ICH. Further experiments should be carried out to determine the specific molecular mechanism.
lncRNAs has also been affirmed as a new target for disease therapy and diagnosis (Zhang and Wang, 2019). The expression level of miRNA is also affected by its upstream molecules, lncRNAs (Militello et al., 2017). While H19, Igsf7, and Lilrb4 are upregulated, Irs4 and LOC103691048 are downregulated in ICH patients (Kim et al., 2019). Some studies indicated that dysregulated lncRNAs were involved in immune response, immune system process, the type I interferon signaling pathway, and defense response (Suarez et al., 2020). This study showed that hsa-miR-15b-5p, hsa-mir-93-5p, and miR-20b-5p were highly correlated with MAPK1; thus, SNHG16, XIST, AC084219.4, RP11-379K17.11, CTC-444N24.11GS1-358P8.4, CTB-89H12.4, RP4-773N10.5, and FGD5-AS1 may be potentially used as novel biomarkers.
In order to further verify our conclusions, we also performed in vivo experiments, generated the ICH model, and conducted exercise training for the treatment of cerebral hemorrhage. PCR analysis revealed that MAPK8 was downregulated in ICH, whereas ATF4, MAPK1, SLC2A1, and TNFAIP3 were upregulated, and these results are concordant with the findings of previous research. It has been revealed that early exercise training can ameliorate neuron cell injury after ICH (Macko et al., 2005). Our study revealed that exercise training may inhibit the MAPK signaling pathway and reduce the expression of MAPK1 and MAPK8. ATF4 was also inhibited as a ferroptosis driver gene. In contrast, the levels of TNFAIP3 and SLC2A1 increased after exercise training. It may be that TNFAIP3 is an antineuritic factor in ICH, and SLC2A1 is a beneficial energy transporter. Therefore, exercise training as an antioxidant therapy may ameliorate nerve injury after cerebral hemorrhage via the above-mentioned ferroptosis genes. Furthermore, the PCR results of our in vivo experiments preliminarily verified the rationality of our bioinformatics results.
The current study identified several genes that are closely associated with ferroptosis after cerebral hemorrhage, some of which had not yet been mentioned in the context of cerebral hemorrhage. These novel genes provide new concepts in ferroptosis after ICH. We found that the stress response pathway, especially the TNF signaling pathway, is extremely important in ferroptosis after cerebral hemorrhage. In addition, molecules significantly associated with TNF signaling, MAPK1 and its associated miRNA and lncRNAs, were found to be potential biomolecules in the ferroptosis pathway after cerebral hemorrhage. We also treated ICH rats by treadmill training to verify the results of our analysis and compared these with healthy controls. We showed that MAPK8 was downregulated, whereas ATF4, MAPK1, SLC2A1, and TNFAIP3 were upregulated in ICH rats. After the exercise intervention, the anti-inflammatory molecule TNFAIP3 and energy regulation factor SLC2A1 were upregulated, while MAPK8, MAPK1, and ATF4 were downregulated, which suggests that treadmill training may influence ferroptosis. The identification of these genes can provide new therapeutic targets for ferroptosis after cerebral hemorrhage, and the regulatory network of MAPK1 molecules can affect the TNF signaling pathway after cerebral hemorrhage.
Publicly available datasets were analyzed in this study. This data can be found here: FerrDb (http://www.zhounan.org/ferrdb/) and https://www.ncbi.nlm.nih.gov/geo/query/acc.cgi?acc=GSE24265.
The animal study was reviewed and approved by The Ethics Committee of Affiliated Hospital of Qingdao University.
QW and TL designed the experiments. YW, TL, XL, and YC performed the experiments. TL, XL, PM, and GZ wrote the manuscript and analyzed the data. All authors contributed to the article and approved the submitted version.
This study was supported by the Natural Science Foundation of Shandong Province, ZR2020MH282 and Clinical Medicine + X grant of The Affiliated Hospital of Qingdao University, China, QDFY+X2019005.
The authors declare that the research was conducted in the absence of any commercial or financial relationships that could be construed as a potential conflict of interest.
We thank Sophie Domingues, Institut de Recerca Hospital Vall d’Hebron, Barcelona, Spain, for the excellent work on the original dataset published in the journal PLoS One (https://journals.plos.org/plosone/article?id=10.1371/journal.pone.0016750).
The Supplementary Material for this article can be found online at: https://www.frontiersin.org/articles/10.3389/fnins.2021.661663/full#supplementary-material
Ameri, K., and Harris, A. L. (2008). Activating transcription factor 4. Int. J. Biochem. Cell Biol. 40, 14–21.
Bader, G. D., and Hogue, C. W. (2003). An automated method for finding molecular complexes in large protein interaction networks. BMC Bioinformatics 4:2.
Bao, W. D., Zhou, X. T., Zhou, L. T., Wang, F., Yin, X., Lu, Y., et al. (2020). Targeting miR-124/Ferroportin signaling ameliorated neuronal cell death through inhibiting apoptosis and ferroptosis in aged intracerebral hemorrhage murine model. Aging Cell 19:e13235.
Barrett, T., Wilhite, S. E., Ledoux, P., Evangelista, C., Kim, I. F., Tomashevsky, M., et al. (2013). NCBI GEO: archive for functional genomics data sets–update. Nucleic Acids Res. 41, D991–D995.
Bobinger, T., Burkardt, P., Huttner, H. B., and Manaenko, A. (2018). Programmed cell death after intracerebral hemorrhage. Curr. Neuropharmacol. 16, 1267–1281. doi: 10.2174/1570159x15666170602112851
Cabal-Hierro, L., Rodriguez, M., Artime, N., Iglesias, J., Ugarte, L., Prado, M. A., et al. (2014). TRAF-mediated modulation of NF-kB AND JNK activation by TNFR2. Cell Signal 26, 2658–2666. doi: 10.1016/j.cellsig.2014.08.011
Catrysse, L., Vereecke, L., Beyaert, R., and van Loo, G. (2014). A20 in inflammation and autoimmunity. Trends Immunol. 35, 22–31. doi: 10.1016/j.it.2013.10.005
Chen, B., Chen, Z., Liu, M., Gao, X., Cheng, Y., Wei, Y., et al. (2019). Inhibition of neuronal ferroptosis in the acute phase of intracerebral hemorrhage shows long-term cerebroprotective effects. Brain Res. Bull. 153, 122–132. doi: 10.1016/j.brainresbull.2019.08.013
Chen, D., Fan, Z., Rauh, M., Buchfelder, M., Eyupoglu, I. Y., Savaskan, N., et al. (2017). ATF4 promotes angiogenesis and neuronal cell death and confers ferroptosis in a xCT-dependent manner. Oncogene 36, 5593–5608. doi: 10.1038/onc.2017.146
Chen, S., Zuo, Y., Huang, L., Sherchan, P., Zhang, J., Yu, Z., et al. (2019). The MC4 receptor agonist RO27-3225 inhibits NLRP1-dependent neuronal pyroptosis via the ASK1/JNK/p38 MAPK pathway in a mouse model of intracerebral haemorrhage. Br. J. Pharmacol. 176, 1341–1356. doi: 10.1111/bph.14639
Chen, Y., Mi, Y., Zhang, X., Ma, Q., Song, Y., Zhang, L., et al. (2019). Dihydroartemisinin-induced unfolded protein response feedback attenuates ferroptosis via PERK/ATF4/HSPA5 pathway in glioma cells. J. Exp. Clin. Cancer Res. 38:402.
Cheng, X., Ander, B. P., Jickling, G. C., Zhan, X., Hull, H., Sharp, F. R., et al. (2020). MicroRNA and their target mRNAs change expression in whole blood of patients after intracerebral hemorrhage. J. Cereb. Blood Flow. Metab. 40, 775–786. doi: 10.1177/0271678x19839501
Codenotti, S., Poli, M., Asperti, M., Zizioli, D., Marampon, F., Fanzani, A., et al. (2018). Cell growth potential drives ferroptosis susceptibility in rhabdomyosarcoma and myoblast cell lines. J. Cancer Res. Clin. Oncol. 144, 1717–1730. doi: 10.1007/s00432-018-2699-0
de Queiroz, K. B., Dos Santos Fontes Pereira, T., Araujo, M. S. S., Gomez, R. S., and Coimbra, R. S. (2018). Resveratrol acts anti-inflammatory and neuroprotective in an infant rat model of pneumococcal meningitis by modulating the hippocampal miRNome. Mol. Neurobiol. 55, 8869–8884. doi: 10.1007/s12035-018-1037-5
Debrabant, B. (2017). The null hypothesis of GSEA, and a novel statistical model for competitive gene set analysis. Bioinformatics 33, 1271–1277.
Dixon, S. J., Lemberg, K. M., Lamprecht, M. R., Skouta, R., Zaitsev, E. M., Gleason, C. E., et al. (2012). Ferroptosis: an iron-dependent form of nonapoptotic cell death. Cell 149, 1060–1072. doi: 10.1016/j.cell.2012.03.042
Dweep, H., Sticht, C., Pandey, P., and Gretz, N. (2011). miRWalk–database: prediction of possible miRNA binding sites by “walking” the genes of three genomes. J. Biomed. Inform. 44, 839–847. doi: 10.1016/j.jbi.2011.05.002
Fischer, R., and Maier, O. (2015). Interrelation of oxidative stress and inflammation in neurodegenerative disease: role of TNF. Oxid. Med. Cell Longev. 2015:610813.
Haddad, J. J., and Land, S. C. (2002). Redox/ROS regulation of lipopolysaccharide-induced mitogen-activated protein kinase (MAPK) activation and MAPK-mediated TNF-alpha biosynthesis. Br. J. Pharmacol. 135, 520–536. doi: 10.1038/sj.bjp.0704467
Hibi, M., Lin, A., Smeal, T., Minden, A., and Karin, M. (1993). Identification of an oncoprotein- and UV-responsive protein kinase that binds and potentiates the c-Jun activation domain. Genes Dev. 7, 2135–2148. doi: 10.1101/gad.7.11.2135
Jiang, Y., Mao, C., Yang, R., Yan, B., Shi, Y., Liu, X., et al. (2017). EGLN1/c-myc induced lymphoid-specific helicase inhibits ferroptosis through lipid metabolic gene expression changes. Theranostics 7, 3293–3305. doi: 10.7150/thno.19988
Karuppagounder, S. S., Alim, I., Khim, S. J., Bourassa, M. W., Sleiman, S. F., John, R., et al. (2016). Therapeutic targeting of oxygen-sensing prolyl hydroxylases abrogates ATF4-dependent neuronal death and improves outcomes after brain hemorrhage in several rodent models. Sci. Transl. Med. 8:328ra29. doi: 10.1126/scitranslmed.aac6008
Kim, J. M., Moon, J., Yu, J. S., Park, D. K., Lee, S. T., and Jung, K. H. (2019). Altered long noncoding RNA profile after intracerebral hemorrhage. Ann. Clin. Transl. Neurol. 6, 2014–2025. doi: 10.1002/acn3.50894
Kim-Han, J. S., Kopp, S. J., Dugan, L. L., and Diringer, M. N. (2006). Perihematomal mitochondrial dysfunction after intracerebral hemorrhage. Stroke 37, 2457–2462. doi: 10.1161/01.str.0000240674.99945.4e
Liu, Z., Zhang, R., Chen, X., Yao, P., Yan, T., Liu, W., et al. (2019). Identification of hub genes and small-molecule compounds related to intracerebral hemorrhage with bioinformatics analysis. PeerJ 7:e7782.
Macko, R. F., Ivey, F. M., Forrester, L. W., Hanley, D., Sorkin, J. D., Katzel, L. I., et al. (2005). Treadmill exercise rehabilitation improves ambulatory function and cardiovascular fitness in patients with chronic stroke: a randomized, controlled trial. Stroke 36, 2206–2211. doi: 10.1161/01.str.0000181076.91805.89
Meng, Z., Zhao, T., Zhou, K., Zhong, Q., Wang, Y., Xiong, X., et al. (2017). A20 ameliorates intracerebral hemorrhage-induced inflammatory injury by regulating TRAF6 polyubiquitination. J. Immunol. 198, 820–831. doi: 10.4049/jimmunol.1600334
Militello, G., Weirick, T., John, D., Doring, C., Dimmeler, S., and Uchida, S. (2017). Screening and validation of lncRNAs and circRNAs as miRNA sponges. Brief Bioinform. 18, 780–788.
Pei, H., Jiang, T., Liu, G., Li, Z., Luo, K., and An, J. (2016). The effect of minimally invasive hematoma aspiration on the JNK signal transduction pathway after experimental intracerebral hemorrhage in rats. Int. J. Mol. Sci. 17:710. doi: 10.3390/ijms17050710
Qin, X., Tang, Q., Jiang, X., Zhang, J., Wang, B., Liu, X., et al. (2020). Zinc Oxide Nanoparticles Induce Ferroptotic Neuronal Cell Death in vitro and in vivo. Int. J. Nanomed. 15, 5299–5315. doi: 10.2147/ijn.s250367
Qureshi, A. I., Tuhrim, S., Broderick, J. P., Batjer, H. H., Hondo, H., Hanley, D. F., et al. (2001). Spontaneous intracerebral hemorrhage. N. Engl. J. Med. 344, 1450–1460.
Rosell, A., Vilalta, A., Garcia-Berrocoso, T., Fernandez-Cadenas, I., Domingues-Montanari, S., Cuadrado, E., et al. (2011). Brain perihematoma genomic profile following spontaneous human intracerebral hemorrhage. PLoS One 6:e16750. doi: 10.1371/journal.pone.0016750
Shah, S. A., Amin, F. U., Khan, M., Abid, M. N., Rehman, S. U., Kim, T. H., et al. (2016). Anthocyanins abrogate glutamate-induced AMPK activation, oxidative stress, neuroinflammation, and neurodegeneration in postnatal rat brain. J. Neuroinflam. 13:286.
Speer, R. E., Karuppagounder, S. S., Basso, M., Sleiman, S. F., Kumar, A., Brand, D., et al. (2013). Hypoxia-inducible factor prolyl hydroxylases as targets for neuroprotection by “antioxidant” metal chelators: From ferroptosis to stroke. Free Radic. Biol. Med. 62, 26–36. doi: 10.1016/j.freeradbiomed.2013.01.026
Su, L., Jiang, X., Yang, C., Zhang, J., Chen, B., Li, Y., et al. (2019). Pannexin 1 mediates ferroptosis that contributes to renal ischemia/reperfusion injury. J. Biol. Chem. 294, 19395–19404. doi: 10.1074/jbc.ra119.010949
Suarez, B., Prats-Mari, L., Unfried, J. P., and Fortes, P. (2020). LncRNAs in the type I interferon antiviral response. Int. J. Mol. Sci. 21:6447. doi: 10.3390/ijms21176447
Subramanian, A., Tamayo, P., Mootha, V. K., Mukherjee, S., Ebert, B. L., Gillette, M. A., et al. (2005). Gene set enrichment analysis: a knowledge-based approach for interpreting genome-wide expression profiles. Proc. Natl. Acad. Sci. U.S.A. 102, 15545–15550. doi: 10.1073/pnas.0506580102
Sun, K. T., Chen, M. Y., Tu, M. G., Wang, I. K., Chang, S. S., and Li, C. Y. (2015). MicroRNA-20a regulates autophagy related protein-ATG16L1 in hypoxia-induced osteoclast differentiation. Bone 73, 145–153. doi: 10.1016/j.bone.2014.11.026
Szklarczyk, D., Morris, J. H., Cook, H., Kuhn, M., Wyder, S., Simonovic, M., et al. (2017). The STRING database in 2017: quality-controlled protein-protein association networks, made broadly accessible. Nucleic Acids Res. 45, D362–D368.
Tamakoshi, K., Ishida, K., Hayao, K., Takahashi, H., and Tamaki, H. (2018). Behavioral effect of short- and long-term exercise on motor functional recovery after intracerebral hemorrhage in rats. J. Stroke Cerebrovasc. Dis. 27, 3630–3635. doi: 10.1016/j.jstrokecerebrovasdis.2018.08.038
Urbano, P., He, X., van Heeswijk, B., Filho, O. P. S., Tijssen, H., Smeets, R. L., et al. (2019). TNFalpha-signaling modulates the kinase activity of human effector treg and regulates IL-17A expression. Front. Immunol. 10:3047.
Veys, K., Fan, Z., Ghobrial, M., Bouche, A., Garcia-Caballero, M., Vriens, K., et al. (2020). Role of the GLUT1 glucose transporter in postnatal CNS angiogenesis and blood-brain barrier integrity. Circ. Res. 127, 466–482.
Walczak, H. (2011). TNF and ubiquitin at the crossroads of gene activation, cell death, inflammation, and cancer. Immunol. Rev. 244, 9–28. doi: 10.1111/j.1600-065x.2011.01066.x
Wang, K., Ye, L., Lu, H., Chen, H., Zhang, Y., Huang, Y., et al. (2017). TNF-alpha promotes extracellular vesicle release in mouse astrocytes through glutaminase. J. Neuroinflam. 14:87.
Wu, J., Zhang, X., Yan, Y., Tang, Z., Sun, X., Huo, G., et al. (2016). The crucial role of cyclin-dependent kinase-5-ataxia-telangiectasia mutated axis in ICH-induced neuronal injury of rat model. Mol. Neurobiol. 53, 6301–6308. doi: 10.1007/s12035-015-9524-4
Wu, X., Luo, J., Liu, H., Cui, W., Guo, W., Zhao, L., et al. (2020). Recombinant adiponectin peptide promotes neuronal survival after intracerebral haemorrhage by suppressing mitochondrial and ATF4-CHOP apoptosis pathways in diabetic mice via Smad3 signalling inhibition. Cell Prolif. 53:e12759.
Xi, G., Keep, R. F., and Hoff, J. T. (2006). Mechanisms of brain injury after intracerebral haemorrhage. Lancet Neurol. 5, 53–63. doi: 10.1016/s1474-4422(05)70283-0
Xiao, F. J., Zhang, D., Wu, Y., Jia, Q. H., Zhang, L., Li, Y. X., et al. (2019). miRNA-17-92 protects endothelial cells from erastin-induced ferroptosis through targeting the A20-ACSL4 axis. Biochem. Biophys. Res. Commun. 515, 448–454. doi: 10.1016/j.bbrc.2019.05.147
Yu, Y., and Richardson, D. R. (2011). Cellular iron depletion stimulates the JNK and p38 MAPK signaling transduction pathways, dissociation of ASK1-thioredoxin, and activation of ASK1. J. Biol. Chem. 286, 15413–15427. doi: 10.1074/jbc.m111.225946
Keywords: intracerebral hemorhage, ferroptosis, TNF signaling pathway, MAPK1, bioinformactics
Citation: Liu T, Li X, Cui Y, Meng P, Zeng G, Wang Q and Wang Y (2021) Bioinformatics Analysis Identifies Potential Ferroptosis Key Genes in the Pathogenesis of Intracerebral Hemorrhage. Front. Neurosci. 15:661663. doi: 10.3389/fnins.2021.661663
Received: 31 January 2021; Accepted: 14 April 2021;
Published: 07 June 2021.
Edited by:
Sandeep Kumar Dhanda, St. Jude Children’s Research Hospital, United StatesReviewed by:
Feng Zhang, Third Hospital of Hebei Medical University, ChinaCopyright © 2021 Liu, Li, Cui, Meng, Zeng, Wang and Wang. This is an open-access article distributed under the terms of the Creative Commons Attribution License (CC BY). The use, distribution or reproduction in other forums is permitted, provided the original author(s) and the copyright owner(s) are credited and that the original publication in this journal is cited, in accordance with accepted academic practice. No use, distribution or reproduction is permitted which does not comply with these terms.
*Correspondence: Yuyang Wang, dG9ueXd5eUAxNjMuY29t; Qiang Wang, MTg2NjE4MDY3NzJAMTYzLmNvbQ==
Disclaimer: All claims expressed in this article are solely those of the authors and do not necessarily represent those of their affiliated organizations, or those of the publisher, the editors and the reviewers. Any product that may be evaluated in this article or claim that may be made by its manufacturer is not guaranteed or endorsed by the publisher.
Research integrity at Frontiers
Learn more about the work of our research integrity team to safeguard the quality of each article we publish.