- 1Institute for Drug and Alcohol Studies, Virginia Commonwealth University School of Medicine, Richmond, VA, United States
- 2Department of Psychiatry, Virginia Commonwealth University School of Medicine, Richmond, VA, United States
- 3Department of Radiology, Virginia Commonwealth University School of Medicine, Richmond, VA, United States
- 4Virginia Commonwealth University School of Medicine, Richmond, VA, United States
- 5Department of Pharmacology and Toxicology, Virginia Commonwealth University School of Medicine, Richmond, VA, United States
- 6Department of Neurology, Virginia Commonwealth University School of Medicine, Richmond, VA, United States
Dynamic causal modeling (DCM) is a method for analyzing functional magnetic resonance imaging (fMRI) and other functional neuroimaging data that provides information about directionality of connectivity between brain regions. A review of the neuropsychiatric fMRI DCM literature suggests that there may be a historical trend to under-report self-connectivity (within brain regions) compared to between brain region connectivity findings. These findings are an integral part of the neurologic model represented by DCM and serve an important neurobiological function in regulating excitatory and inhibitory activity between regions. We reviewed the literature on the topic as well as the past 13 years of available neuropsychiatric DCM literature to find an increasing (but still, perhaps, and inadequate) trend in reporting these results. The focus of this review is fMRI as the majority of published DCM studies utilized fMRI and the interpretation of the self-connectivity findings may vary across imaging methodologies. About 25% of articles published between 2007 and 2019 made any mention of self-connectivity findings. We recommend increased attention toward the inclusion and interpretation of self-connectivity findings in DCM analyses in the neuropsychiatric literature, particularly in forthcoming effective connectivity studies of substance use disorders.
Introduction
Dynamic causal modeling (DCM) is one means of computing and modeling neuronal effective (directional) connectivity between regions in the brain and likely the most commonly applied method to measure effective connectivity (Friston et al., 2019). DCM can also be used to measure self-connectivity which is also described in the literature as intrinsic connectivity or endogenous connectivity, technically described as intra-regional connections within any given volume of interest Friston et al. (2003). Despite increases in the relative rate of reporting these results in recent years, self-connectivity findings are much less commonly reported than extrinsic brain connectivity findings in the neuropsychiatric DCM literature.
Zeidman et al. (2019) stated that these findings are reported as the diagonal in the matrices of endogenous and modulatory effects defined in any given DCM – that is, every DCM will include these results. Self-connections provide only inhibitory (negative) influence on each region included in the model. Self-connections regulate the excitatory and inhibitory extrinsic (between-region) connections that may be estimated by the model and ultimately preclude the possibility of a runaway positive feedback loop in the neurologic model estimated by DCM. Extrinsic connections may contribute to the inhibitory regulation of any given region but, ultimately, it is the inhibitory (negative) inputs from the self-connections that control the gain of the regional response to extrinsic connection. Self-connections neurobiologically control the excitatory-inhibitory balance – in this sense, they are physically, chemically, as well as neuro- and electro-physiologically necessary for describing a viable model. For example, as the magnitude of the self-connection parameter increases, the net effect of extrinsic inputs on that region decreases and vice versa. Focusing on self-connectivity (intrinsic connectivity) in functional magnetic resonance imaging (fMRI) analyses, Friston et al. (2003) describe this effect as “augmenting or attenuating” the decay of synaptic activity.
At this time, most of the pre-existing discussion on the topic of self-connectivity pertains primarily to fMRI analyses. However, there is some discussion on utilizing self-connectivity findings in other imaging modalities, as well, namely electroencephalography (EEG) and magnetoencephalography (MEG). Brown and Friston (2012) discuss the modeling of self-connectivity using EEG data to model the self-inhibition of regions by superficial pyramidal neurons. They make brief reference of utilizing MEG and PET data to model similar interactions. We acknowledge the importance of these other neuroimaging modalities in the neuropsychiatric literature. But, our aim in this mini-review is to take account of the rate of reporting these kinds of results in the fMRI literature on neuropsychiatric disorders to understand better the trends in this aspect of DCM analyses.
Methods
We conducted a literature review to identify references including dynamic causal analyses of fMRI data in the neuropsychiatric and neuro-behavioral literature. We further evaluated this subset of references for mention and consideration of self-connectivity findings, to include any mention, actual discussion in the text, and any kind of graphical representation of self-connectivity findings. We also conducted a more involved assessment of each reference recording more details on the number of participants, number of models, regions of interest (ROI), as well as number and type of connections reported to assess whether there may be any difference between the studies that reported self-connectivity findings and those that did not. We computed descriptive statistics of trends in reporting self-connectivity findings in the literature over the period of time considered. We also tabulated the number and type of imaging modalities utilized in this subset of the literature.
Eligibility Criteria
We considered studies with: (1) analysis of fMRI data findings; (2) use of DCM to analyze imaging data; (3) variable group diagnosed with a neuropsychiatric disorder; and (4) publication in a PubMed-indexed journal. According to the protocol, neuropsychiatric disorders considered in the search included: attention deficit-hyperactivity disorder, anorexia, autism spectrum disorder, bipolar disorder, cannabis use disorder, cocaine use disorder, dementia, depression, gaming/gambling disorder, intellectual disability, obsessive-compulsive disorder, opioid use disorder, Parkinson’s disease, post-traumatic stress disorder, seizure disorder, schizophrenia, and tobacco use disorder.
Information Search Strategy
The search was conducted by using search terms to identify articles that incorporated DCM analyses conducted on populations with neuropsychiatric disorders. To screen for pertinent articles, we used PubMed/MEDLINE for either “DCM” OR “DCM” AND one of the above neuropsychiatric disorders or pertaining acronym, to include the terms: “attention deficit-hyperactivity disorder,” “ADHD,” “anorexia,” “autism spectrum disorder,” “autism,” “bipolar disorder,” “cannabis use disorder,” “cocaine use disorder,” “dementia,” “depression,” “gambling disorder,” “intellectual disability,” “obsessive-compulsive disorder,” “opioid use disorder,” “Parkinson’s disease,” “post-traumatic stress disorder,” “PTSD,” “seizure disorder,” “schizophrenia,” OR “tobacco use disorder.” In essence, the goal was to identify articles including effective connectivity models pertaining only to a manageably broad interpretation of the neuropsychiatric literature. We also searched Google Scholar for additional references and included them regardless of PubMed indexing status to maximize the number of references captured for the review. The date of the last search was June 1st, 2020.
Data Management
Literature search results were recorded from a Google Chrome internet browser using the Zotero reference manager application plug-in. Specific pertinent details for each reference were extracted and maintained in a Google Spreadsheet document. One author conducted the search and maintained the database of results. All authors discussed and reviewed the eligibility criteria and confirmed the viability of the references. Any duplicates from PubMed and Google Scholar were excluded upon review of the whole spreadsheet. There were no discrepancies or ambiguous inclusions in the reference list. All references identified were written in English.
Data Extraction
All authors discussed and agreed upon the data to be extracted from the literature search. One researcher conducted the review and extraction of the pertinent data from the references obtained from literature search. Among the information collected were the following: neuropsychiatric disorder, report of self-connectivity results (binary), inclusion of discussion of self-connectivity results (binary), depiction of graphical representation of self-connectivity results (binary), number of subjects, number of controls, number of models reported, number of ROI, names of ROIs, number of extrinsic connections, number of self-connections, version of SPM used, version of DCM module used, type of model (deterministic, stochastic), and other information (authors, year of publication, and title). Report of, discussion of, and depiction of graphical representation of self-connectivity results were recorded using a binary variable (0 = not present, 1 = present). Numbers of subjects, controls, models reported, ROIs, extrinsic connections, and self-connections were all recorded as continuous variables. Neuropsychiatric disorders, names of ROIs, type of model were recorded with the appropriate designations.
Data Analysis
We conducted basic descriptive statistics on the number of references including self-connectivity findings as well as the percent of self-connectivity findings overall as well as within each neuropsychiatric disorder included in the search. We also conducted unpaired t-tests of the continuous variables collected in an attempt to assess any potential differences between studies reporting self-connectivity findings and those without. Statistical tests were not conducted for number of self-connections given that the group not reporting these findings would have a count of zero by default.
Results
The literature search revealed 81 references published during the years 2007 to 2019, spanning most of the neuropsychiatric conditions included in the aforementioned list. The distribution of neuropsychiatric disorders represented in the literature references are summarized in Table 1.
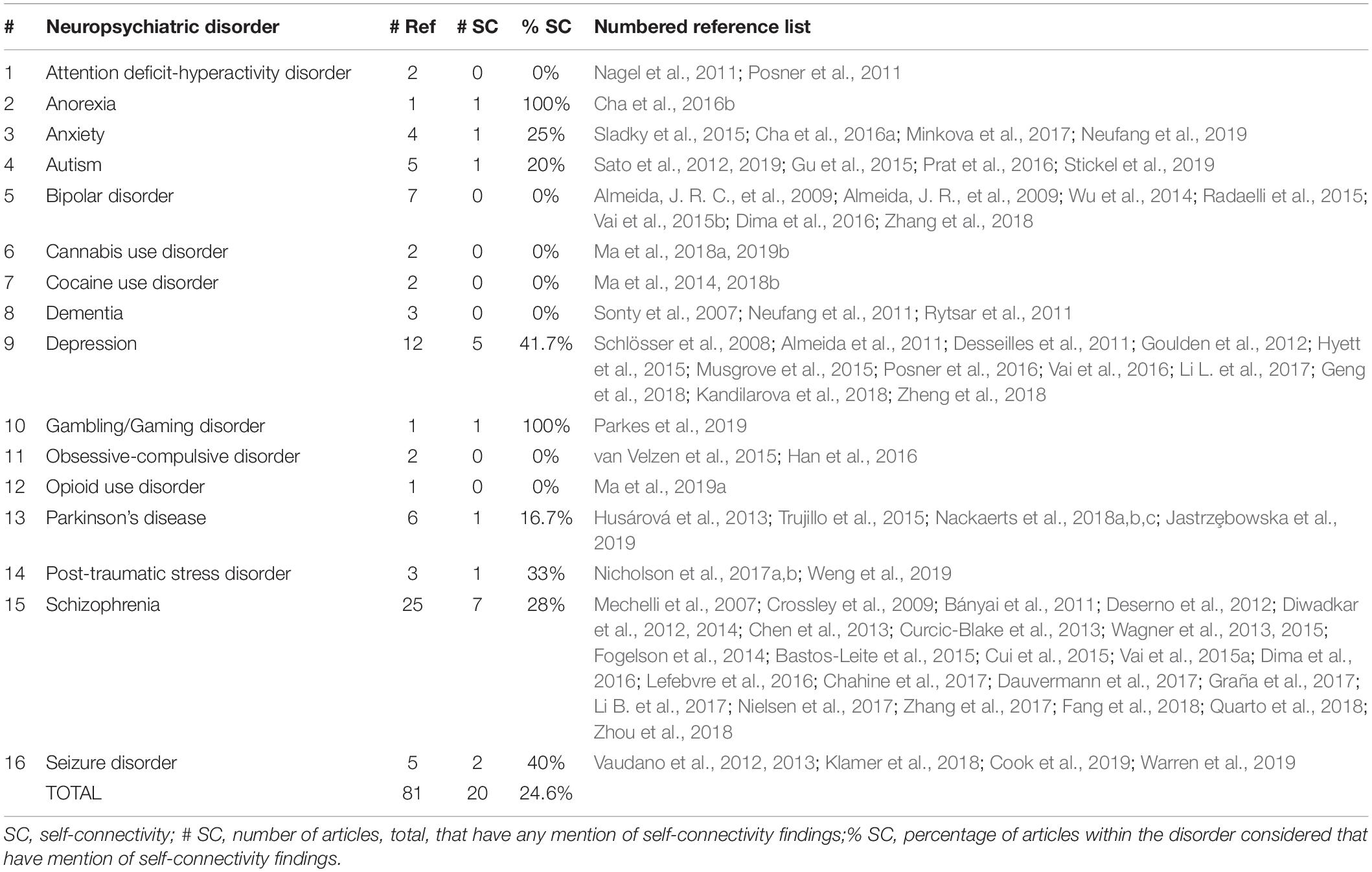
Table 1. Summary of neuropsychiatric disorders represented in the literature review by numerical count and percentage of total number of references.
A broad range of neuropsychiatric and neurobehavioral disorders are covered in the DCM literature reviewed including mood, psychotic, addiction, and even eating disorders; some disorders are better represented with more numerous references than others. Schizophrenia, for example, was by far the most-studied among neuropsychiatric disorders considered using DCM of neuroimaging data with nearly a third of the references (26 out of 81, 32%). Depression was the second most prevalent with 12 (14.8%), followed by bipolar disorder (7, 8.6%) and Parkinson’s disease (6, 7.4%). The other disorders listed in the analysis include only a few to several references each and appear to be relatively less fully examined from an effective connectivity standpoint.
Of the total 81 references, 73 (90%) were found to have a graphical representation of a DCM model. Within the total 81 references, 21 references (25.9%) were found to have any kind of mention of self-connectivity findings – 19 (23.5%) of which included actual discussion of such findings and 14 (17.3%) had a graphical representation of self-connectivity findings.
Graphical depiction of the reference count by year as well as percentage mention of self-connectivity are included below in Figure 1. Note that the incidence is expressed as a percentage of references with mention, discussion, or a graphical representation for the particular year as a fraction of the total 81 references. For example, four references with mention of self-connectivity findings in 2019 would represent 4.9% of the total references reviewed reporting self-connectivity findings during that year alone.
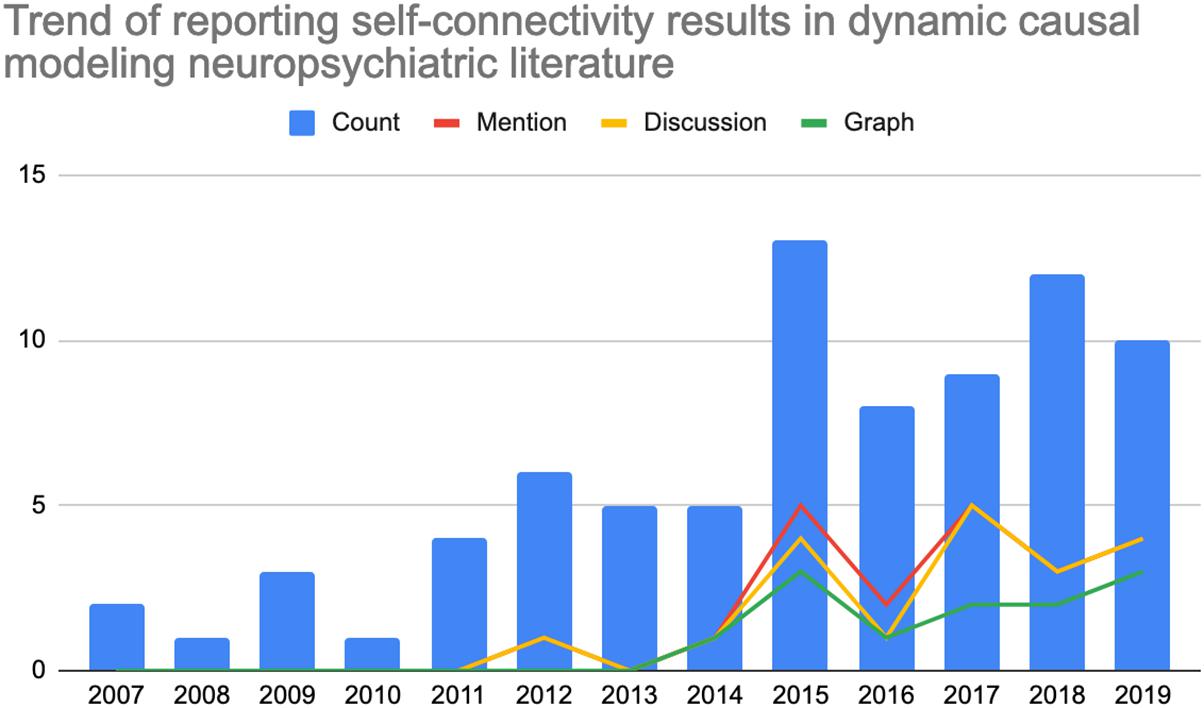
Figure 1. Count of references (left axis) by year within the literature review which (0, blue) total number of neuropsychiatric references reporting dynamic causal modeling findings per year; (1, red) count of references making any mention of self-connectivity results; (2, yellow) count of references including self-connectivity with the discussion; or (3, green) count of references demonstrating self-connectivity findings in graphical form.
There were no statistically significant differences between the studies reporting self-connectivity findings and those that did not for the other variables considered (e.g., number of subjects, number of ROI, number of models reported, etc.). There was, however, a general trend for later SPM versions for studies reporting self-connectivity findings.
Discussion
The rate of reporting self-connectivity results in the DCM literature pertaining to neuropsychiatric disorders does not appear to have kept up with the relatively rapid adoption of DCM as an analytic technique in this area. Despite rapid adoption of DCM as an analytic technique in the neuropsychiatric literature – up from two publications in 2007 to 10 in 2019 – only 21 (25.9%) of the articles during this 13 year period have included any mention of self-connectivity findings. Under-reporting appears to have occurred in the context of what appears to be sufficient representation of the phenomenon of self-connectivity in the technical DCM literature. Because all DCM analyses include self-connectivity findings, some kind of statement should be included that summarizes these results, even non-significant ones. Regardless of statistical significance, these findings attribute important characteristics to the neurologic system being modeled using DCM.
Though the better-studied disorders feature self-connectivity reporting approaching 30–40% (e.g., depression, schizophrenia), none of them exceed even this modest threshold consistently. Perhaps most notable in this regard is bipolar disorder with 7 references identified articles reporting DCM results, zero of which reported self-connectivity findings. There are a few disorders with higher rates of reporting self-connectivity results (e.g., anorexia, gaming/gambling disorder), but these are also typically less well-examined in the DCM literature and amount to a few references in total. Some of the disparity in quantity of publications per disorder is likely due to the relative prevalence of certain disorders, perceived public health need and thus historical availability of funding. Relative to the other types of disorders and considering prevalence, substance use disorder appear to be particularly under-represented among DCM results – not to mention also with zero reports of self-connectivity findings. In all likelihood, the representation of substance use disorders in this list will increase most notably in the future given renewed recent interest, awareness of prevalence and increasing public health concern, as well as associated increase in research funding available.
Perhaps most noteworthy is the total absence of reported self-connectivity findings between the years of 2007–2011. Among subsequent years, only a minority of references included mention (25.9%). Even fewer articles include actual discussion (23.4%) or a graphical representation of self-connectivity findings (17.3%). So, even when results are being reported in the literature, they may not be receiving full consideration among other interregional results that have historically received greater focus. Overall, the historical trend points to a somewhat recently emergent tendency of reporting these results in some form, beginning in 2012 and continuing more later in the 2010s. Even in 2015 – the year with the highest incidence of self-connectivity findings being reported – only 5 of the 13 references reviewed (38.4%) for that year included mention of self-connectivity findings. This number was lower in 2016 (2 of 8, 25%) but the overall trend appears to demonstrate a non-linear but increasing adoption for the time being, perhaps due to ongoing efforts of experts in the field in providing additional guidance on interpreting and reporting such findings as well as increasing awareness over time.
The lack of statistically significant differences in the above study characteristics (e.g., number of subjects, ROI, number of extrinsic connections, etc.) may not be altogether surprising given that the DCM methodology may be otherwise consistently applied across the time period specified. Expectations for appropriate powering of neuroimaging studies have increased with time but such expectations are likely sustained at a high level through the specified interval. A tendency to report full models (perhaps with the historically apparent exception of self-connectivity findings) would indicate a similar number of ROIs and extrinsic connections. The later versions of SPM cited for studies reporting self-connectivity findings is consistent with the relative recency of consistent reporting of these results.
We acknowledge that other neuroimaging modalities (EEG and MEG) may have more robust inclusion and discussion of self-connectivity findings. Indeed, our literature search revealed a number of such references which were ultimately excluded in favor of focusing on fMRI findings for this mini-review. Perhaps this topic could be expanded further in a subsequent technical review or interval update which would include discussion of the factors which may result in differences in reporting self-connectivity findings across different imaging modalities.
Conclusion
Despite a sufficient technical literature on the topic, there appears to be a tendency in the neuropsychiatric DCM literature to under-report self-connectivity findings. A number of possible explanations may arise for this observation including confusion about interpreting this kind of result in the context of perhaps “more interesting” inter-regional connections; only more recently emerging guidance on interpretation of self-connectivity findings; as well as a historically greater interest in “mapping out circuits” across the brain versus parsing out the intra-regional and inter-regional components in those circuits. Our review of the literature and consideration of our own self-connectivity findings suggest that self-connections represent an integral but under-reported part of DCM analysis. We encourage neuropsychiatric researchers – and particularly substance use disorder researchers – to reconsider the role of self-connectivity findings in future analyses.
Author Contributions
AS, LM, and JS developed the literature review criteria. AS conducted the literature review and analysis. AS, LM, JS, KW, and FM wrote the manuscript. All authors contributed to the article and approved the submitted version.
Funding
This work was supported by the National Institute on Drug Abuse (U54 DA038999 (NIDA) and UL1 TR002649 (NIDA)).
Conflict of Interest
The authors declare that the research was conducted in the absence of any commercial or financial relationships that could be construed as a potential conflict of interest.
Publisher’s Note
All claims expressed in this article are solely those of the authors and do not necessarily represent those of their affiliated organizations, or those of the publisher, the editors and the reviewers. Any product that may be evaluated in this article, or claim that may be made by its manufacturer, is not guaranteed or endorsed by the publisher.
References
Almeida, J. R., Kronhaus, D. M., Sibille, E. L., Langenecker, S. A., Versace, A., and Labarbara, E. J. (2011). Abnormal left-sided orbitomedial prefrontal cortical-amygdala connectivity during happy and fear face processing: a potential neural mechanism of female MDD. Front. Psychiatry 2:69. doi: 10.3389/fpsyt.2011.00069
Almeida, J. R., Versace, A., Mechelli, A., Hassel, S., Quevedo, K., Kupfer, D. J., et al. (2009). Abnormal amygdala-prefrontal effective connectivity to happy faces differentiates bipolar from major depression. Biol. Psychiatry 66, 451–459. doi: 10.1016/j.biopsych.2009.03.024
Almeida, J. R. C., Mechelli, A., Hassel, S., Versace, A., Kupfer, D. J., and Phillips, M. L. (2009). Abnormally increased effective connectivity between parahippocampal gyrus and ventromedial prefrontal regions during emotion labeling in bipolar disorder. Psychiatry Res. 174, 195–201. doi: 10.1016/j.pscychresns.2009.04.015
Bányai, M., Diwadkar, V. A., and Erdi, P. (2011). Model-based dynamical analysis of functional disconnection in schizophrenia. NeuroImage 58, 870–877. doi: 10.1016/j.neuroimage.2011.06.046
Bastos-Leite, A. J., Ridgway, G. R., Silveira, C., Norton, A., Reis, S., and Friston, K. J. (2015). Dysconnectivity within the default mode in first-episode schizophrenia: a stochastic dynamic causal modeling study with functional magnetic resonance imaging. Schizophr. Bull. 41, 144–153. doi: 10.1093/schbul/sbu080
Brown, H. R., and Friston, K. J. (2012). Dynamic causal modelling of precision and synaptic gain in visual perception—an EEG study. Neuroimage 63, 223–231. doi: 10.1016/j.neuroimage.2012.06.044
Cha, J., DeDora, D., Nedic, S., Ide, J., Greenberg, T., Hajcak, G., et al. (2016a). Clinically anxious individuals show disrupted feedback between inferior frontal gyrus and prefrontal-limbic control circuit. J. Neurosci. 36, 4708–4718. doi: 10.1523/JNEUROSCI.1092-15.2016
Cha, J., Ide, J. S., Bowman, F. D., Simpson, H. B., Posner, J., and Steinglass, J. E. (2016b). Abnormal reward circuitry in anorexia nervosa: a longitudinal, multimodal MRI study. Hum. Brain Mapp. 37, 3835–3846. doi: 10.1002/hbm.23279
Chahine, G., Richter, A., Wolter, S., Goya-Maldonado, R., and Gruber, O. (2017). Disruptions in the left frontoparietal network underlie resting state endophenotypic markers in schizophrenia. Hum. Brain Mapp. 38, 1741–1750. doi: 10.1002/hbm.23477
Chen, P.-J., Fan, L.-Y., Hwang, T.-J., Hwu, H.-G., Liu, C.-M., and Chou, T.-L. (2013). The deficits on a cortical-subcortical loop of meaning processing in schizophrenia. Neuroreport 24, 147–151. doi: 10.1097/WNR.0b013e32835df562
Cook, C. J., Hwang, G., Mathis, J., Nair, V. A., Conant, L. L., Allen, L., et al. (2019). Effective connectivity within the default mode network in left temporal lobe epilepsy: findings from the epilepsy connectome project. Brain Connect. 9, 174–183. doi: 10.1089/brain.2018.0600
Crossley, N. A., Mechelli, A., Fusar-Poli, P., Broome, M. R., Matthiasson, P., Johns, L. C., et al. (2009). Superior temporal lobe dysfunction and frontotemporal dysconnectivity in subjects at risk of psychosis and in first-episode psychosis. Hum. Brain Mapp. 30, 4129–4137. doi: 10.1002/hbm.20834
Cui, L.-B., Liu, J., Wang, L.-X., Li, C., Xi, Y.-B., Guo, F., et al. (2015). Anterior cingulate cortex-related connectivity in first-episode schizophrenia: a spectral dynamic causal modeling study with functional magnetic resonance imaging. Front. Hum. Neurosci. 9:589. doi: 10.3389/fnhum.2015.00589
Curcic-Blake, B., Liemburg, E., Vercammen, A., Swart, M., Knegtering, H., Bruggeman, R., et al. (2013). When Broca goes uninformed: reduced information flow to Broca’s area in schizophrenia patients with auditory hallucinations. Schizophr. Bull. 39, 1087–1095. doi: 10.1093/schbul/sbs107
Dauvermann, M. R., Moorhead, T. W., Watson, A. R., Duff, B., Romaniuk, L., Hall, J., et al. (2017). Verbal working memory and functional large-scale networks in schizophrenia. Psychiatry Res. Neuroimaging 270, 86–96. doi: 10.1016/j.pscychresns.2017.10.004
Deserno, L., Sterzer, P., Wüstenberg, T., Heinz, A., and Schlagenhauf, F. (2012). Reduced prefrontal-parietal effective connectivity and working memory deficits in schizophrenia. J. Neurosci. 32, 12–20. doi: 10.1523/JNEUROSCI.3405-11.2012
Desseilles, M., Schwartz, S., Dang-Vu, T. T., Sterpenich, V., Ansseau, M., Maquet, P., et al. (2011). Depression alters “top-down” visual attention: a dynamic causal modeling comparison between depressed and healthy subjects. NeuroImage 54, 1662–1668. doi: 10.1016/j.neuroimage.2010.08.061
Dima, D., Roberts, R. E., and Frangou, S. (2016). Connectomic markers of disease expression, genetic risk and resilience in bipolar disorder. Transl. Psychiatry 6:e706. doi: 10.1038/tp.2015.193
Diwadkar, V. A., Bakshi, N., Gupta, G., Pruitt, P., White, R., and Eickhoff, S. B. (2014). Dysfunction and dysconnection in cortical-striatal networks during sustained attention: genetic risk for schizophrenia or bipolar disorder and its impact on brain network function. Front. Psychiatry 5:50. doi: 10.3389/fpsyt.2014.00050
Diwadkar, V. A., Wadehra, S., Pruitt, P., Keshavan, M. S., Rajan, U., Zajac-Benitez, C., et al. (2012). Disordered corticolimbic interactions during affective processing in children and adolescents at risk for schizophrenia revealed by functional magnetic resonance imaging and dynamic causal modeling. Arch. Gen. Psychiatry 69, 231–242. doi: 10.1001/archgenpsychiatry.2011.1349
Fang, X., Wang, Y., Cheng, L., Zhang, Y., Zhou, Y., Wu, S., et al. (2018). Prefrontal dysconnectivity links to working memory deficit in first-episode schizophrenia. Brain Imaging Behav. 12, 335–344. doi: 10.1007/s11682-017-9692-0
Fogelson, N., Litvak, V., Peled, A., Fernandez-del-Olmo, M., and Friston, K. (2014). The functional anatomy of schizophrenia: a dynamic causal modeling study of predictive coding. Schizophr. Res. 158, 204–212. doi: 10.1016/j.schres.2014.06.011
Friston, K. J., Harrison, L., and Penny, W. (2003). Dynamic causal modelling. Neuroimage 19, 1273–1302. doi: 10.1016/s1053-8119(03)00202-7
Friston, K. J., Preller, K. H., Mathys, C., Cagnan, H., Heinzle, J., Razi, A., et al. (2019). Dynamic causal modelling revisited. Neuroimage 199, 730–744. doi: 10.1016/j.neuroimage.2017.02.045
Geng, X., Xu, J., Liu, B., and Shi, Y. (2018). Multivariate classification of major depressive disorder using the effective connectivity and functional connectivity. Front. Neurosci. 12:38. doi: 10.3389/fnins.2018.00038
Goulden, N., McKie, S., Thomas, E. J., Downey, D., Juhasz, G., Williams, S. R., et al. (2012). Reversed frontotemporal connectivity during emotional face processing in remitted depression. Biol. Psychiatry 72, 604–611. doi: 10.1016/j.biopsych.2012.04.031
Graña, M., Ozaeta, L., and Chyzhyk, D. (2017). Resting state effective connectivity allows auditory hallucination discrimination. Int. J. Neural Syst. 27:1750019. doi: 10.1142/S0129065717500198
Gu, X., Eilam-Stock, T., Zhou, T., Anagnostou, E., Kolevzon, A., Soorya, L., et al. (2015). Autonomic and brain responses associated with empathy deficits in autism spectrum disorder. Hum. Brain Mapp. 36, 3323–3338. doi: 10.1002/hbm.22840
Han, H. J., Jung, W. H., Yun, J.-Y., Park, J. W., Cho, K. K., Hur, J.-W., et al. (2016). Disruption of effective connectivity from the dorsolateral prefrontal cortex to the orbitofrontal cortex by negative emotional distraction in obsessive-compulsive disorder. Psychol. Med. 46, 921–932. doi: 10.1017/S0033291715002391
Husárová, I., Mikl, M., Lungu, O. V., Mareček, R., Vaníček, J., and Bareš, M. (2013). Similar circuits but different connectivity patterns between the cerebellum, basal ganglia, and supplementary motor area in early Parkinson’s disease patients and controls during predictive motor timing. J. Neuroimaging 23, 452–462. doi: 10.1111/jon.12030
Hyett, M. P., Breakspear, M. J., Friston, K. J., Guo, C. C., and Parker, G. B. (2015). Disrupted effective connectivity of cortical systems supporting attention and interoception in melancholia. JAMA Psychiatry 72, 350–358. doi: 10.1001/jamapsychiatry.2014.2490
Jastrzȩbowska, M. A., Marquis, R., Melie-García, L., Lutti, A., Kherif, F., Herzog, M. H., et al. (2019). Dopaminergic modulation of motor network compensatory mechanisms in Parkinson’s disease. Hum. Brain Mapp. 40, 4397–4416. doi: 10.1002/hbm.24710
Kandilarova, S., Stoyanov, D., Kostianev, S., and Specht, K. (2018). Altered resting state effective connectivity of anterior insula in depression. Fron. Psychiatry 9:83. doi: 10.3389/fpsyt.2018.00083
Klamer, S., Ethofer, T., Torner, F., Sahib, A. K., Elshahabi, A., Marquetand, J., et al. (2018). Unravelling the brain networks driving spike-wave discharges in genetic generalized epilepsy-common patterns and individual differences. Epilepsia Open 3, 485–494. doi: 10.1002/epi4.12252
Lefebvre, S., Demeulemeester, M., Leroy, A., Delmaire, C., Lopes, R., Pins, D., et al. (2016). Network dynamics during the different stages of hallucinations in schizophrenia. Hum. Brain Mapp. 37, 2571–2586. doi: 10.1002/hbm.23197
Li, B., Cui, L.-B., Xi, Y.-B., Friston, K. J., Guo, F., Wang, H.-N., et al. (2017). Abnormal effective connectivity in the brain is involved in auditory verbal hallucinations in schizophrenia. Neurosci. Bull. 33, 281–291. doi: 10.1007/s12264-017-0101-x
Li, L., Li, B., Bai, Y., Liu, W., Wang, H., Leung, H.-C., et al. (2017). Abnormal resting state effective connectivity within the default mode network in major depressive disorder: a spectral dynamic causal modeling study. Brain Behav. 7:e00732. doi: 10.1002/brb3.732
Ma, L., Steinberg, J. L., Bjork, J. M., Keyser-Marcus, L., Vassileva, J., Zhu, M., et al. (2018a). Fronto-striatal effective connectivity of working memory in adults with cannabis use disorder. Psychiatry Res. 278, 21–34. doi: 10.1016/j.pscychresns.2018.05.010
Ma, L., Steinberg, J. L., Cunningham, K. A., Bjork, J. M., Lane, S. D., Schmitz, J. M., et al. (2018b). Altered anterior cingulate cortex to hippocampus effective connectivity in response to drug cues in men with cocaine use disorder. Psychiatry Res. Neuroimaging 271, 59–66. doi: 10.1016/j.pscychresns.2017.10.012
Ma, L., Steinberg, J. L., Bjork, J. M., Taylor, B. A., Arias, A. J., Terplan, M., et al. (2019a). Cingulo-hippocampal effective connectivity positively correlates with drug-cue attentional bias in opioid use disorder. Psychiatry Res. Neuroimaging 294:110977. doi: 10.1016/j.pscychresns.2019.08.005
Ma, L., Steinberg, J. L., Bjork, J. M., Wang, Q., Hettema, J. M., Abbate, A., et al. (2019b). Altered effective connectivity of central autonomic network in response to negative facial expression in adults with cannabis use disorder. Biol. Psychiatry. Cogn. Neurosci. Neuroimaging 5, 84–96. doi: 10.1016/j.bpsc.2019.05.013
Ma, L., Steinberg, J. L., Hasan, K. M., Narayana, P. A., Kramer, L. A., and Moeller, F. G. (2014). Stochastic dynamic causal modeling of working memory connections in cocaine dependence. Hum. Brain Mapp. 35, 760–778. doi: 10.1002/hbm.22212
Mechelli, A., Allen, P., Amaro, E., Fu, C. H. Y., Williams, S. C. R., Brammer, M. J., et al. (2007). Misattribution of speech and impaired connectivity in patients with auditory verbal hallucinations. Hum. Brain Mapp. 28, 1213–1222. doi: 10.1002/hbm.20341
Minkova, L., Sladky, R., Kranz, G. S., Woletz, M., Geissberger, N., Kraus, C., et al. (2017). Task-dependent modulation of amygdala connectivity in social anxiety disorder. Psychiatry Res. Neuroimaging 262, 39–46. doi: 10.1016/j.pscychresns.2016.12.016
Musgrove, D. R., Eberly, L. E., Klimes-Dougan, B., Basgoze, Z., Thomas, K. M., Mueller, B. A., et al. (2015). Impaired bottom-up effective connectivity between amygdala and subgenual anterior cingulate cortex in unmedicated adolescents with major depression: results from a dynamic causal modeling analysis. Brain Connect. 5, 608–619. doi: 10.1089/brain.2014.0312
Nackaerts, E., Michely, J., Heremans, E., Swinnen, S., Smits-Engelsman, B., Vandenberghe, W., et al. (2018a). Being on target: visual information during writing affects effective connectivity in Parkinson’s disease. Neuroscience 371, 484–494. doi: 10.1016/j.neuroscience.2017.12.027
Nackaerts, E., Michely, J., Heremans, E., Swinnen, S. P., Smits-Engelsman, B. C. M., Vandenberghe, W., et al. (2018b). Training for micrographia alters neural connectivity in Parkinson’s disease. Front. Neurosci. 12:3. doi: 10.3389/fnins.2018.00003
Nackaerts, E., Nieuwboer, A., Broeder, S., Swinnen, S., Vandenberghe, W., and Heremans, E. (2018c). Altered effective connectivity contributes to micrographia in patients with Parkinson’s disease and freezing of gait. J. Neurol. 265, 336–347. doi: 10.1007/s00415-017-8709-3
Nagel, B. J., Maia, T. V., Mechling, A., Oh, M., Wang, Z., and Peterson, B. S. (2011). Abnormal amygdalar activation and connectivity in adolescents with attention-deficit/hyperactivity disorder. J. Am. Acad. Child Adolesc. Psychiatry 50, 828–837.
Neufang, S., Akhrif, A., Riedl, V., Förstl, H., Kurz, A., Zimmer, C., et al. (2011). Disconnection of frontal and parietal areas contributes to impaired attention in very early Alzheimer’s disease. J. Alzheimers Dis. 25, 309–321. doi: 10.3233/JAD-2011-102154
Neufang, S., Geiger, M. J., Homola, G. A., Mahr, M., Schiele, M. A., Gehrmann, A., et al. (2019). Cognitive-behavioral therapy effects on alerting network activity and effective connectivity in panic disorder. Eur. Arch. Psychiatry Clin. Neurosci. 269, 587–598. doi: 10.1007/s00406-018-0945-8
Nicholson, A. A., Friston, K. J., Zeidman, P., Harricharan, S., McKinnon, M. C., Densmore, M., et al. (2017a). Dynamic causal modeling in PTSD and its dissociative subtype: bottom-up versus top-down processing within fear and emotion regulation circuitry. Hum. Brain Mapp. 38, 5551–5561. doi: 10.1002/hbm.23748
Nicholson, A. A., Rabellino, D., Densmore, M., Frewen, P. A., Paret, C., Kluetsch, R., et al. (2017b). The neurobiology of emotion regulation in posttraumatic stress disorder: amygdala downregulation via real-time fMRI neurofeedback. Hum. Brain Mapp. 38, 541–560. doi: 10.1002/hbm.23402
Nielsen, J. D., Madsen, K. H., Wang, Z., Liu, Z., Friston, K. J., and Zhou, Y. (2017). Working memory modulation of frontoparietal network connectivity in first-episode schizophrenia. Cereb. Cortex 27, 3832–3841. doi: 10.1093/cercor/bhx050
Parkes, L., Tiego, J., Aquino, K., Braganza, L., Chamberlain, S. R., Fontenelle, L. F., et al. (2019). Transdiagnostic variations in impulsivity and compulsivity in obsessive-compulsive disorder and gambling disorder correlate with effective connectivity in cortical-striatal-thalamic-cortical circuits. NeuroImage 202, 116070. doi: 10.1016/j.neuroimage.2019.116070
Posner, J., Cha, J., Roy, A. K., Peterson, B. S., Bansal, R., Gustafsson, H. C., et al. (2016). Alterations in amygdala-prefrontal circuits in infants exposed to prenatal maternal depression. Transl. Psychiatry 6:e935. doi: 10.1038/tp.2016.146
Posner, J., Nagel, B. J., Maia, T. V., Mechling, A., Oh, M., Wang, Z., et al. (2011). Abnormal amygdalar activation and connectivity in adolescents with attention-deficit/hyperactivity disorder. J. Am. Acad. Child Adolesc. Psychiatry 50, 828–837. doi: 10.1016/j.jaac.2011.05.010
Prat, C. S., Stocco, A., Neuhaus, E., and Kleinhans, N. M. (2016). Basal ganglia impairments in autism spectrum disorder are related to abnormal signal gating to prefrontal cortex. Neuropsychologia 91, 268–281. doi: 10.1016/j.neuropsychologia.2016.08.007
Quarto, T., Paparella, I., De Tullio, D., Viscanti, G., Fazio, L., Taurisano, P., et al. (2018). Familial risk and a genome-wide supported DRD2 variant for schizophrenia predict lateral prefrontal-amygdala effective connectivity during emotion processing. Schizophr. Bull. 44, 834–843. doi: 10.1093/schbul/sbx128
Radaelli, D., Sferrazza Papa, G., Vai, B., Poletti, S., Smeraldi, E., Colombo, C., et al. (2015). Fronto-limbic disconnection in bipolar disorder. Eur. Psychiatry 30, 82–88. doi: 10.1016/j.eurpsy.2014.04.001
Rytsar, R., Fornari, E., Frackowiak, R. S., Ghika, J. A., and Knyazeva, M. G. (2011). Inhibition in early Alzheimer’s disease: an fMRI-based study of effective connectivity. NeuroImage 57, 1131–1139. doi: 10.1016/j.neuroimage.2011.05.029
Sato, W., Kochiyama, T., Uono, S., Yoshimura, S., Kubota, Y., Sawada, R., et al. (2019). Atypical amygdala-neocortex interaction during dynamic facial expression processing in autism spectrum disorder. Front. Hum. Neurosci. 13:351. doi: 10.3389/fnhum.2019.00351
Sato, W., Toichi, M., Uono, S., and Kochiyama, T. (2012). Impaired social brain network for processing dynamic facial expressions in autism spectrum disorders. BMC Neurosci. 13:99. doi: 10.1186/1471-2202-13-99
Schlösser, R. G. M., Wagner, G., Koch, K., Dahnke, R., Reichenbach, J. R., and Sauer, H. (2008). Fronto-cingulate effective connectivity in major depression: a study with fMRI and dynamic causal modeling. NeuroImage 43, 645–655. doi: 10.1016/j.neuroimage.2008.08.002
Sladky, R., Höflich, A., Küblböck, M., Kraus, C., Baldinger, P., Moser, E., et al. (2015). Disrupted effective connectivity between the amygdala and orbitofrontal cortex in social anxiety disorder during emotion discrimination revealed by dynamic causal modeling for FMRI. Cerebral Cortex 25, 895–903. doi: 10.1093/cercor/bht279
Sonty, S. P., Mesulam, M.-M., Weintraub, S., Johnson, N. A., Parrish, T. B., and Gitelman, D. R. (2007). Altered effective connectivity within the language network in primary progressive aphasia. J. Neurosci. 27, 1334–1345. doi: 10.1523/JNEUROSCI.4127-06.2007
Stickel, S., Weismann, P., Kellermann, T., Regenbogen, C., Habel, U., Freiherr, J., et al. (2019). Audio-visual and olfactory-visual integration in healthy participants and subjects with autism spectrum disorder. Hum. Brain Mapp. 40, 4470–4486. doi: 10.1002/hbm.24715
Trujillo, J. P., Gerrits, N. J. H. M., Veltman, D. J., Berendse, H. W., van der Werf, Y. D., and van den Heuvel, O. A. (2015). Reduced neural connectivity but increased task-related activity during working memory in de novo Parkinson patients. Hum. Brain Mapp. 36, 1554–1566. doi: 10.1002/hbm.22723
Vai, B., Bulgarelli, C., Godlewska, B. R., Cowen, P. J., Benedetti, F., and Harmer, C. J. (2016). Fronto-limbic effective connectivity as possible predictor of antidepressant response to SSRI administration. Eur. Neuropsychopharmacol. 26, 2000–2010. doi: 10.1016/j.euroneuro.2016.09.640
Vai, B., Sferrazza Papa, G., Poletti, S., Radaelli, D., Donnici, E., Bollettini, I., et al. (2015a). Abnormal cortico-limbic connectivity during emotional processing correlates with symptom severity in schizophrenia. Eur. Psychiatry 30, 590–597. doi: 10.1016/j.eurpsy.2015.01.002
Vai, B., Poletti, S., Radaelli, D., Dallaspezia, S., Bulgarelli, C., Locatelli, C., et al. (2015b). Successful antidepressant chronotherapeutics enhance fronto-limbic neural responses and connectivity in bipolar depression. Psychiatry Res. 233, 243–253. doi: 10.1016/j.pscychresns.2015.07.015
van Velzen, L. S., de Wit, S. J., Ćurĉić-Blake, B., Cath, D. C., de Vries, F. E., Veltman, D. J., et al. (2015). Altered inhibition-related frontolimbic connectivity in obsessive-compulsive disorder. Hum. Brain Mapp. 36, 4064–4075. doi: 10.1002/hbm.22898
Vaudano, A. E., Avanzini, P., Tassi, L., Ruggieri, A., Cantalupo, G., Benuzzi, F., et al. (2013). Causality within the epileptic network: an EEG-fMRI study validated by intracranial EEG. Front. Neurol. 4:185. doi: 10.3389/fneur.2013.00185
Vaudano, A. E., Carmichael, D. W., Salek-Haddadi, A., Rampp, S., Stefan, H., Lemieux, L., et al. (2012). Networks involved in seizure initiation. A reading epilepsy case studied with EEG-fMRI and MEG. Neurology 79, 249–253. doi: 10.1212/WNL.0b013e31825fdf3a
Wagner, G., De la Cruz, F., Schachtzabel, C., Güllmar, D., Schultz, C. C., Schlösser, R. G., et al. (2015). Structural and functional dysconnectivity of the fronto-thalamic system in schizophrenia: a DCM-DTI study. Cortex 66, 35–45. doi: 10.1016/j.cortex.2015.02.004
Wagner, G., Koch, K., Schachtzabel, C., Schultz, C. C., Gaser, C., Reichenbach, J. R., et al. (2013). Structural basis of the fronto-thalamic dysconnectivity in schizophrenia: a combined DCM-VBM study. NeuroImage Clin. 3, 95–105. doi: 10.1016/j.nicl.2013.07.010
Warren, A. E. L., Harvey, A. S., Vogrin, S. J., Bailey, C., Davidson, A., Jackson, G. D., et al. (2019). The epileptic network of lennox-gastaut syndrome: cortically driven and reproducible across age. Neurology 93, e215–e226. doi: 10.1212/WNL.0000000000007775
Weng, Y., Qi, R., Zhang, L., Luo, Y., Ke, J., Xu, Q., et al. (2019). Disturbed effective connectivity patterns in an intrinsic triple network model are associated with posttraumatic stress disorder. Neurol. Sci. 40, 339–349. doi: 10.1007/s10072-018-3638-1
Wu, G., Wang, Y., Mwansisya, T. E., Pu, W., Zhang, H., Liu, C., et al. (2014). Effective connectivity of the posterior cingulate and medial prefrontal cortices relates to working memory impairment in schizophrenic and bipolar patients. Schizophr. Res. 158, 85–90. doi: 10.1016/j.schres.2014.06.033
Zeidman, P., Jafarian, A., Corbin, N., Seghier, M. L., Razi, A., Price, C. J., et al. (2019). A guide to group effective connectivity analysis, part 1: first level analysis with DCM for fMRI. NeuroImage 200, 174–190. doi: 10.1016/j.neuroimage.2019.06.031
Zhang, L., Li, B., Wang, H., Li, L., Liao, Q., Liu, Y., et al. (2017). Decreased middle temporal gyrus connectivity in the language network in schizophrenia patients with auditory verbal hallucinations. Neurosci. Lett. 653, 177–182. doi: 10.1016/j.neulet.2017.05.042
Zhang, L., Opmeer, E. M., van der Meer, L., Aleman, A., Ćurčić-Blake, B., and Ruhé, H. G. (2018). Altered frontal-amygdala effective connectivity during effortful emotion regulation in bipolar disorder. Bipolar Disord. 20, 349–358. doi: 10.1111/bdi.12611
Zheng, K.-Z., Wang, H.-N., Liu, J., Xi, Y.-B., Li, L., Zhang, X., et al. (2018). Incapacity to control emotion in major depression may arise from disrupted white matter integrity and OFC-amygdala inhibition. CNS Neurosci. Ther. 24, 1053–1062. doi: 10.1111/cns.12800
Keywords: dynamic causal modeling, intrinsic connectivity, extrinsic connectivity, effective connectivity, inhibitory interneuron, self-connectivity
Citation: Snyder AD, Ma L, Steinberg JL, Woisard K and Moeller FG (2021) Dynamic Causal Modeling Self-Connectivity Findings in the Functional Magnetic Resonance Imaging Neuropsychiatric Literature. Front. Neurosci. 15:636273. doi: 10.3389/fnins.2021.636273
Received: 01 December 2020; Accepted: 07 June 2021;
Published: 11 August 2021.
Edited by:
Plamen Ch. Ivanov, Boston University, United StatesReviewed by:
Frederik Van de Steen, Vrije University Brussel, BelgiumStavros I. Dimitriadis, Greek Association of Alzheimer’s Disease and Related Disorders, Greece
Copyright © 2021 Snyder, Ma, Steinberg, Woisard and Moeller. This is an open-access article distributed under the terms of the Creative Commons Attribution License (CC BY). The use, distribution or reproduction in other forums is permitted, provided the original author(s) and the copyright owner(s) are credited and that the original publication in this journal is cited, in accordance with accepted academic practice. No use, distribution or reproduction is permitted which does not comply with these terms.
*Correspondence: Andrew D. Snyder, YXNueWRlcjJAdmN1LmVkdQ==; Frederick G. Moeller, ZnJlZGVyaWNrLm1vZWxsZXJAdmN1aGVhbHRoLm9yZw==