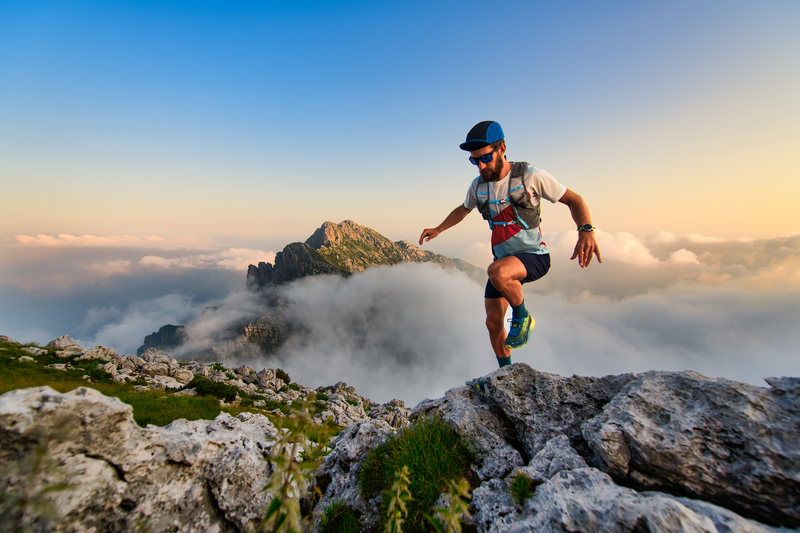
94% of researchers rate our articles as excellent or good
Learn more about the work of our research integrity team to safeguard the quality of each article we publish.
Find out more
REVIEW article
Front. Neurosci. , 03 February 2021
Sec. Neurodegeneration
Volume 15 - 2021 | https://doi.org/10.3389/fnins.2021.614251
This article is part of the Research Topic Multi-omics, Epigenomics and Computational Analysis of Neurodegenerative Disorders View all 11 articles
Parkinson’s disease (PD) is a multifactorial disorder characterized by progressively debilitating dopaminergic neurodegeneration in the substantia nigra and the striatum, along with various metabolic dysfunctions and molecular abnormalities. Metabolomics is an emerging study and has been demonstrated to play important roles in describing complex human diseases by integrating endogenous and exogenous sources of alterations. Recently, an increasing amount of research has shown that metabolomics profiling holds great promise in providing unique insights into molecular pathogenesis and could be helpful in identifying candidate biomarkers for clinical detection and therapies of PD. In this review, we briefly summarize recent findings and analyze the application of molecular metabolomics in familial and sporadic PD from genetic mutations, mitochondrial dysfunction, and dysbacteriosis. We also review metabolic biomarkers to assess the functional stage and improve therapeutic strategies to postpone or hinder the disease progression.
As the second most common chronic neurodegenerative disorder after Alzheimer’s disease, Parkinson’s disease (PD) is a multisystemic disease with multiple mechanisms and neurochemical features, affecting around >2% of all persons above 65 years of age and >4% of all persons over the age of 80 (GBD 2015 Neurological Disorders Collaborator Group, 2017; Santos Garcia et al., 2019; Xu et al., 2019). E Ray Dorsey et al. make an important point about the global burden of PD, with the number of affected individuals having risen from 2.5 million in 1990 to 6.1 million in 2016, with projections that by 2050 the number of PD patients will be at 12 million (GBD 2016 Parkinson’s Disease Collaborators, 2018). From an etiological perspective, the two hallmarks and indicators of a definite diagnosis of PD are the deterioration of dopaminergic neurons and the accumulation of intracytoplasmic protein α-Synuclein (α-Syn), called Lewy bodies, in the substantia nigra region of the brain (Spillantini et al., 1997; Spillantini and Goedert, 2018). They are mainly relevant to various neuropathological insults, such as genetic mutants (Kim and Alcalay, 2017), oxidative stress (Puspita et al., 2017), apoptosis, neuroinflammation (De Virgilio et al., 2016; Rocha et al., 2018), mitochondrial dysfunction (Bose and Beal, 2016), disrupting intercellular communication (Hou et al., 2019), endocrine disorders (De Pablo-Fernández et al., 2017), and inhibition of aberrant protein degradation pathways (Tofaris et al., 2001; Spencer et al., 2014; Sugeno et al., 2014). The α-Syn is linked to PD pathology, which possesses prion-like behavior and presents in various throughout the nervous systems before neuronal death and classical symptoms (Grassi et al., 2018; Ma et al., 2019). However, as a multifactorial disease, PD is also influenced by dietary factors (Tufi et al., 2014; Fitzgerald et al., 2017; Lehmann et al., 2017; Zhao et al., 2019), microorganisms (Keshavarzian et al., 2015; Scheperjans et al., 2015), and different environmental elements, such as metal (Kim et al., 2018), neurotoxins (Bove and Perier, 2012), light exposure (Willis et al., 2018), and infection. The disease has complex etiopathogenesis that has still not been fully elucidated. Though four categories of biomarkers have been recommended to confer accurate diagnosis and assess the condition of patients, including clinical symptoms, genetic mutation, pathological, and neuroimaging changes (Delenclos et al., 2016), markers for an early diagnosis and effective treatments of PD are still lacking. Clinically, there is a high rate of misdiagnosis of the disease and clinical accuracy of PD diagnosis is only 76–84%. Therefore, a better understanding of the etiology and pathogenesis, as well as molecular events associated with clinical symptoms, will be significant for early diagnosis and therapeutic strategies.
Metabolomics is an emerging and effective approach used in the identification and discovery of metabolic biomarkers; it relies on the assessment of various biological samples and provides a series of metabolic signatures involving molecular processes that elucidate pathological changes of diseases. The technology links various metabolic molecular mechanisms to neuronal activity alterations, protein changes or genetic mutations, mitochondrial dysfunction, or dysbacteriosis. As an advanced technique of omics, metabolomics can integrate endogenous and exogenous cellular metabolic activities, holding great promise in its ability to probe biochemical details about the pathological status, progression, and treatment of many chronic metabolic diseases, such as cancer, neurodegenerative disease, and kidney disease (Kalim and Rhee, 2017; Willis et al., 2018). Interestingly, an increasing number of scholars devoted to PD research have indicated that metabolomics can be considered as a powerful tool to define biochemical information, detect metabolomic status, and speculate on underlying mechanisms in the disease (Koeth et al., 2013; Pannkuk et al., 2015). Metabolomics’ high-sensitivity and high-throughput properties might support detailed information of the end-product abnormalities arising from interactions between genes, chemicals, protein structure, and various environmental factors. In this respect, metabolomics could be more applicable than other “omics” techniques, including genomics, pharmacogenomics, and transcriptomics, in the qualitative and quantitative analysis of metabolites from cell or biologic specimens to effectively reflect subtle changes of metabolites (Stoessel et al., 2018). Therefore, the introduction of metabolomics in PD research would provide a new solution for seeking underlying metabolic biomarkers for the predication and treatment of the disease.
Considering clinical and experimental findings in pathological mechanisms, we know that multiple mechanisms may contribute to PD pathogenesis. Specially, most studies about the metabolomics of PD mainly focus on gene alterations, energy homeostasis, and redox reactions resulting from mitochondrial dysfunction. Meanwhile, declined antioxidation systems and mitochondrial disorders are also important causes of neuron inflammation and senescence associated with neuropathology (Boland et al., 2018). Updated preclinical evidence indicated that the bidirectional communication between the gut community patterns and the nervous system of the brain, hereto dysbacteriosis, was identified and plays an important role in both the metabolism and pathology of patients with PD. So, in-depth research on the metabolomics regarding potential metabolic indicators and pathways of PD should focus on its effects on pathogenesis and the pathological process.
In this review article, we provide a concise overview on technical methods and related operative procedures in the field of metabolomics. We review recent research on the relationship between metabolomics and neuropathological changes of PD in terms of genetic mutation, mitochondrial dysfunctions, and dysbacteriosis, and also summarize the molecular mechanisms and metabolites underlying pathological signs as promising biomarkers of pathogenesis in both sporadic and familial PD.
The metabolome is the entire collection of a wide range of small molecules that participate in body metabolic responses, such as saccharides, amino acids, nucleotides, lipids, and acylcarnitines. Metabolomics, as an analytical technique to investigate disorders in the metabolome of an organism, possess substantial sensitivity, selectivity, and identification capabilities of analyzing diverse varieties of molecular species in biofluids, ranging from ionic compounds in cell lysates to various organic compounds/composition in plasma, cerebrospinal fluid (CSF), urine, and tissue (Figure 1). Compared with traditional targeted approaches, the new untargeted metabolomics have great potential to identify some novel biomarkers and help in indicating the metabolite levels of body fluids, seeking different disease biomarkers to provide useful information about metabolic pathways, metabolites, and pathological mechanisms.
Figure 1. Metabolomics analysis methods. Molecular metabolites were extracted from different samples, ranging from ionic compounds in cell lysates to various organic compositions in plasma, cerebrospinal fluid (CSF), urine, and tissue. Samples chromatography and mass spectrometry were prepared and administrated for analysis and identification.
In the past decade, many analytical technologies have been introduced and applied in various metabolomic research fields and thus have furthered the understanding of neurodegenerative diseases on the basis of relevant metabolites as biomarkers. In general, the methodologies used for metabolic identification mainly include proton nuclear magnetic resonance (NMR), magnetic resonance spectroscopy (MRS), liquid chromatography mass spectrometry (LCMS), gas chromatography mass spectrometry (GCMS), flourier transform infrared spectrum (FTIR), and high-performance liquid chromatography (HPLC). The first two metrics utilize the magnetic properties of molecular atomic nuclei in metabolic samples to obtain detailed chemical, structural, and quantities information of metabolites in little samples. By contrast, chromatography mass spectrometry (CMS), which combines the efficient separation capability of chromatography with the high detectability of mass spectrometry, has high analytical precision and superior reproducibility and versatility (Kiraly et al., 2016).
With the development of technologies and current research, there are different groups of metabolic biomarkers used in susceptivity, diagnosis, pharmacodynamic response, and prognostic assessment of diseases (Correia et al., 2017). Emerging evidence has revealed that related metabolomics would be a potential tool for screening and monitoring molecular mechanisms and chemical phenotypes and seeking metabolic signatures as diagnostic and prognostic biomarkers of familial and idiopathic PD. For instance, Bogdanov et al. reported the differences of metabolomic profiling of plasma from idiopathic PD and LRRK2 patients with the G2019S mutation, implicating that the familial PD has unique metabolomic profiles associated with the purine pathway and oxidative processes (Bogdanov et al., 2008; Johansen et al., 2009; Bolner et al., 2011). Similarly, metabolic profiles of blood in idiopathic PD are also different from healthy groups, such as alpha-synuclein, tau protein, urate, and a series of amino acid metabolism (Bolner et al., 2011; Luan et al., 2015; Saiki et al., 2017; Chang et al., 2018; Goldman et al., 2018). These disturbances in the metabolic pathways are related to mitochondrial dysfunctions and the concomitant changes in energy homeostasis and redox reaction, which are thought to be the final common pathways of most endogenous and exogenous factors that are involved in the etiology of PD (Bhinderwala et al., 2019). Recent studies have revealed that there are metabolic differences between treated and drug-naïve PD patients (Bogdanov et al., 2008; Troisi et al., 2019), as well as patients with and without dementia or depression (Hatano et al., 2016; Dong et al., 2018). In addition, biofluids metabolome has potential to distinguish the phenotype of PD. For example, James Roede et al. (2013), used mass spectrometry-based metabolic profiling and showed that polyamine dopamine metabolism was significantly altered in the rapid motor progression of PD compared to both healthy subjects and slow progression PD subjects, which potentially effects of neurodegeneration on neuroinflammation or dopamine metabolism. The metabolomics of animal models demonstrated disturbed metabolic pathways in acylcarnitines, glycerophospholipid, and 4-hydroxypoline in serum, indicating the metabolism influence on the onset and progression of α-Syn pathology (Graham et al., 2018).
Genomics is the upstream regulator of metabolomics and participates in the modulation of differential metabolite concentrations. Since 1977, studies have provided initial insights into molecular genetics and identified the key contributors that give rise to the occurrence and progression of familial PD cases (Polymeropoulos et al., 1997; Kruger et al., 1998; Braak et al., 2003; Zarranz et al., 2004). Until now, 27 PD-associated genes regions have been identified, affecting 20% of all PD patients (Klein and Westenberger, 2012; Correia et al., 2017; Arkinson and Walden, 2018). There are six genes contributing to the clinically classical form of PD, including three autosomal dominant (SNCA, LRRK2, and VPS35) and three autosomal recessively (PINK1, PARK2, and DJ-1). Additionally, some singular gene mutations are associated with an increased risk of developing PD, including autosomal dominant (PARK3, GIGYF2, HTRA2, EIF4G1, RAB39B, TMEM230, CHCHD2, RIC3, and GBA) and autosomal recessive (ATP13A2, PLA2GB, FBXO7, DNAJC13, SYNJ1, and VPS13C). Previous studies revealed that three types of metabolic defects mainly play important roles in the progression of PD: a-Syn protein aggregation, mitochondrial dysfunction, and related oxidative damage. From the structural and functional perspectives, these cellular dysfunctions are associated with different sites and types of alterations in these genes (Figure 2). Even though most familial monogenic forms of PD are identified, metabolic research mainly focuses on the minority of PD related-genes mutations, including SNCA, LRRK2, PARK2, and GBA. Therefore, a thorough understanding of these gene-related metabolomics will provide available biomarkers for diagnosing and tracking familial PD.
Figure 2. Genetic metabolomics in PD. The complexity of the pathology of PD stemming from the overlap of multiple gene mutants and complex environmental factors. Both locally and systemically, these hazards contribute to a series of responses associated with mitochondrial dysfunction, neuroinflammation, and the failure of clearance mechanisms.
The SNCA gene is the first gene to be implicated in PD. Of note, the gene encodes a-Syn protein and its pathogenic mutations were linked with the abnormal accumulation of the presynaptic protein. The initial link between the SNCA gene and PD was found by Polymeropoulos et al. (1997) when a missense mutation (A53T) of SNCA was implicated in patients with autosomal dominant Parkinsonism from a large Italian family. Shortly thereafter, accumulating evidence has shown the mutations of A30Pro (Kruger et al., 1998), E46K (Zarranz et al., 2004), H50Q, G51D, and A53E in the alpha-synuclein gene (Parajuli et al., 2020). All six-point mutants have been involved in a-Syn overexpression, accumulation, and aggregation, conferring the risk of the disease’s onset or causing familial PD (Singleton et al., 2013). In addition, the SNCA duplication or triplication events (PARK4 variant), as well as the promoter’s variation, were also involved in the formation of toxic oligomers, misfolded α-Syn, and nigrostriatal denervation, which are vital causes of the disease (Gatto et al., 2010; Koros et al., 2018).
Apart from protein-encoding, research has suggested the role of the SNCA gene in fatty acid synthesis, lipid metabolism (Golovko et al., 2007), mitochondrial membrane composition (Barcelo-Coblijn et al., 2007), and inflammatory responses in the brain (Castagnet et al., 2005; Golovko et al., 2009). Consistent with previous reports, a current study supports previous findings of the SNCA involvement in substance metabolism of the brain. It is worth noting that the authors identified a range of metabolic changes related to the gene through untargeted metabolomic profiling of the brain, such as glycogen depletion, impaired activity of succinate dehydrogenase, and the abnormality of taurine and glutamine (Musgrove et al., 2014). The metabolic alterations not only reflect impaired mitochondrial function in energy production, but also indicate the pathologies associated with other metabolic pathways. Similar to deteriorating metabolic abnormalities in the brain, the SNCA gene-related mutations could affect peripheral tissue metabolism in PD patients, which are useful in understanding the metabolic status of the brain and providing molecular signatures. Demonstrated in a cross-sectional study by Heather et al., the premotor A53T SNCA carriers have decreasing serotonin transporter densities and serotonergic pathologies compared with healthy controls (Wilson et al., 2019). In addition, the serotonergic abnormalities preceded dopaminergic neuron loss and clinical symptoms, suggesting the potential role of the serotonergic neurotransmitter system in screening and monitoring the progression of the disease (Qamhawi et al., 2015; Wilson et al., 2018). In a longitudinal study, the comparison between A53T transgenic mice and controls revealed that the A53T mutation could substantially increase guanosine levels as a positive regulation against neurodegeneration (Chen et al., 2015).
LRRK2 (Leucine-rich repeat kinase 2) is the most common gene related to PD, with a frequency of 10% in familiar cases (Paisan-Ruiz et al., 2008; Hernandez et al., 2016). Located in a region on chromosome 12, the gene consists of 51 exons which encode a 2,527 amino acid member of the ROCO protein family (Paisan-Ruiz et al., 2008), and relates to mitochondrial functions, cytoskeletal dynamics, and cellular processes (Guaitoli et al., 2016; Bae and Lee, 2019). Based on current research, eight pathogenic substitutions (p.Arg1441Cys/Gly/His, p.Asn1437His, p.Tyr1699Cys, p.Gly2019Ser, p.Ile2020Thr, and p.Ile2012Thr) and two susceptibility variants (p.Arg1628Pro and p.Gly2385Arg) in LRRK2 have been identified. The G2019S substitution is most frequent LRRK2-related mutation. These PD-associated LRRK2 mutations might increase intracellular ROS production and contribute to oxidative stress and the loss of dopaminergic neurons.
The correlation between the increase of oxidative stress markers and reduced antioxidant capacity and LRRK mutation was assessed in the current study (Loeffler et al., 2017). They measured oxidative stress and antioxidant markers in CSF from LRRK2-related PD patients, sporadic patients, and control subjects. Two direct indicators of oxidative stress, the 8-hydroxy-2’-deoxyguanosine (8-OHdG) and 8-isoprostane (8-ISO) concentrations, were increased in LRRK2 patients compared with healthy groups, while antioxidant capacity might decrease during the progression of the disease. Similar to the SNCA gene, the metabolomic profiles of low molecular weight substances in PD patients with LRRK2 mutations are also different from idiopathic PD and healthy controls (Johansen et al., 2009). In this study, the LRRK2 mutation patients showed significantly decreased hypoxanthine, Xanthine, and uric acid in plasma, suggesting the reduction of related antioxidant activities. In addition, several studies have provided evidence that blood levels of uric acid appeared to correlate negatively with the risk for developing PD (Annanmaki et al., 2007; Ascherio et al., 2009; Ou et al., 2017). These suggest that metabolites of the purine pathway play a potential role in elucidating pathogenesis and biomarkers of PD. Like uric acid, LRRK2 mutation was associated with impaired serine metabolism, showing decreased serine racemase expression and increased serine levels (Nickels et al., 2019). LRRK2 genes also took part in other metabolic responses, such as Akt signaling, glucose metabolism, or immunity, contributing to the identification of metabolism in LRRK-PD (Infante et al., 2015; Wile et al., 2017).
In autosomal recessively PD, PINK1, and PARK2 are associated with the neurodegenerative disorder, which encode the E3 ubiquitin ligase Parkin and the mitochondrial serine/threonine kinase PINK1 that play important roles in mitochondrial quality control and turnover (Arkinson and Walden, 2018). Under normal conditions, PINK1 can phosphorylate and recruit Parkin proteins from the cytoplasm to depolarized mitochondria, then meditate the ubiquitination of mitochondrial outer membrane proteins and activate mitophagy to degrade the ubiquitin mitochondrial proteins mitofusin 1 and 2 (Pickrell and Youle, 2015; Matheoud et al., 2016; Barodia et al., 2017). Similar to autosomal dominant genes, PINK1 and PARK2 mutations induce metabolomic changes in PD patients. Okuzumi et al. (2019) analyzed serum metabolomics from Parkin patients and age-matched controls, and revealed higher levels of oxidized lipids and fatty acid metabolites and lower levels of antioxidant markers in PARK2 patients, suggesting the relationship between the serum/plasma metabolomics and gene dysfunction. Additionally, as a way of ensuring mitochondrial quality control, the mutation effects the elimination of dysfunctional mitochondria that was associated with an increase of mitochondrial stress, manifesting a systemic oxidative stress markers for the pathomechanisms of Parkin-mutation patients (Ueno et al., 2020).
The most common genetic risk factor for PD is the glucocerebrosidase (GBA) gene, which is located on chromosome 1q21 and contains 11 exons that encode the lysosomal enzyme glucocerebrosidase. In normal cells, the metabolism of glucocerebroside attributes to the efficacy of the glucocerebrosidase (GCase). Reports indicated that GCase not only increases the breakdown of glucocerebroside into glucose and ceramide, but also plays a role in α-Syn degradation (Sidransky and Lopez, 2012; Migdalska-Richards and Schapira, 2016). By contrast, previous studies have shown that the variants of p.E365K and p.T408M in the GBA gene are associated with PD (Liu et al., 2016; Mallett et al., 2016). The GBA mutations disturb the function of related lysosomal enzymes, which provoke a-Syn accumulation (Sidransky and Lopez, 2012), disrupt autophagy-lysosome and molecular homeostasis (Uemura et al., 2015), and impair the functional mitochondria by inhibiting mitophagy (Zampieri et al., 2017). For understanding metabolic consequences associated with the GBA gene alterations, recent research has detected the CSF of patients with glucocerebrosidase dysfunction, and observed impairments in mitochondrial function and the urea cycle that increased the abundance of several metabolites, such as 1,5-anhydro-D-glucitol, asparagine, ornithine, glutamine, and glycine (Greuel et al., 2020).
Most patients are diagnosed as sporadic idiopathic PD as opposed to familial patients, in which environmental hazards play an important role in the pathogenesis of neurodegeneration diseases. The pathogenesis of PD involves complex interactions among multifarious pathomechanisms that include oxidative stress, mitochondrial alterations, inflammatory response, and dysbacteriosis. These pathological changes usually accelerate the truncation (Kahle et al., 2001; Auluck et al., 2002; Liu et al., 2005) and multimerization of misfolding proteins through phospholipid binding, membrane compound altering, and change in the function of molecular chaperones (Tuttle et al., 2016; Gerez et al., 2019). The identification of aberrant biochemistry underlying neuronal degeneration could be an important step toward discovering mechanisms and accurate markers for the diagnosis and therapy of PD. Based on previous studies and updated evidence exploring the metabolomics profiling of biofluids in PD patients, most existing knowledge shows the alteration of different molecular species that mainly focus on genes alterations, energy homeostasis, and redox reaction resulting from mitochondrial dysfunction. Hence, we summarize the progress on metabolomics in idiopathic PD cases and focus on the metabolic biomarkers associated with mitochondrial dysfunction and dysbacteriosis.
As the dynamic powerhouse of a cell, the mitochondrion plays a major role in metabolic activity and generates over 90% of the ATP in a cell. Mitochondria contain their own genomes (mtDNA) and encode vital components associated with mitochondrial function. There is increasing evidence that the mitochondrial function extends well beyond the production of energy in carbohydrate, fatty acid, amino acid, and nucleotide metabolism, it aids in the stabilization of cytosolic calcium, and relates to metabolic pathways, such as the pyruvate oxidation, the Krebs cycle, and various immune responses (Luan et al., 2015; Di Maio et al., 2016). To date, diverse gene mutations and environmental factors have been identified as the cause of mitochondrial dysfunction; it likely to be a key contributor to PD pathogenesis by damaging the transport of mitochondrial proteins, inhibiting respiratory chain function, actuating the generation of reactive oxygen species (ROS), and increasing α-Syn aggregation. As shown in previous studies, the complex I function of the electron transport chain in mitochondrion is impaired because of exposure to environmental toxins such as paraquat, rotenone, and metals (Muthukumaran et al., 2014; Stauch et al., 2016; Thellung et al., 2019). Patients with sporadic PD not only present metabolic changes about abnormal mitochondrial activity in energy homeostasis and redox reaction (Krige et al., 1992; Haas et al., 1995; Penn et al., 1995), but have the presence of mitochondrial oxidative metabolism and insulin resistance (Marcovina et al., 2013; Gonzalez-Casacuberta et al., 2019; Djordjevic et al., 2020). As can be seen in Figure 3, these impaired mitochondrial protein import reduced mitochondrial dynamics, increase ROS, and create mitophagy abnormalities or bioenergetic defects that would deteriorate α-Syn protein misfolding and aggregation.
Figure 3. Mitochondrial metabolomics in PD patients. (A) Under normal status: 1. mitochondrial complex IV subunit 4 isoform (COX4I2) and proliferator-activated receptor gamma coactivator 1-α (PGC1α) facilitate mitochondrial biogenesis. Additionally, PINK1 and Parkin alleviates PARIS toxicity by phosphorylation and ubiquitination, respectively. 2. PINK1 acts on dynamin-related protein 1 (DRP1) to regulate mitochondrial fission and PKA (PINK1 inhibits protein kinase) inhibits the progress. As such, LRRK2 is also involved in mitochondrial dynamic by MFNs and OPA1 (two mitochondrial fusion proteins) as well as DRP1 (a mitochondrial fission protein). 3. PINK1, Parkin, and LRRK2 mediate mitochondrial transport. 4. PINK1/Parkin clears damaged mitochondria by mitophagy (B). Under gene mutant: 1. mitochondrial biogenesis is inhibited by upregulating PGC1α, which is vulnerable to ROS. 2. The imbalance of mitochondrial dynamics. 3. The mutation of PINK1, Parkin, or LRRK2 halt mitochondrial transportation via Miro, Milton, and motor protein Kinesin-1. In addition, altering LRRK2 expression can stabilize filamentous actin (F-actin) and promote tau neurotoxicity. 4. Hazards causes PINK1 to accumulate when Parkin is impaired, followed by failure in the mitophagy and production of ROS. 5. Deposition of GCB and misfolding a-Syn disrupt mitochondrial respiration, leading to the production of ROS and dysfunction of lysosomes.
These mitochondrial changes disturb a series of energy metabolism systems (pentose phosphate pathway, glycolysis, mitochondrial oxidative phosphorylation, glycolysis, acylcarnitines, and the tricarboxylic cycle) (Roede et al., 2013; Trupp et al., 2014; Willkommen et al., 2018), and are also involved in the upregulation or downregulation of amino acids, lipids, and antioxidant substances in PD (Bazinet and Laye, 2014; Lei et al., 2014; Tyurina et al., 2015). Like the correlation between mitochondrial function and gene alterations (Figure 3), comprehensive metabolic analysis of mitochondrial defects arising from environmental factors, such as oxidative stress and energy substance metabolism, might promote the discovery of some discern biomarkers for PD. For example, Younes-Mhenni et al. (2007) and Lewitt et al. (2013) found significantly higher activity of oxidized glutathione, superoxide dismutase (SOD), and catalase in PD patients compared with healthy people. Similarly, increasing 8-hydroxy-2-deoxyguanosine (8-OHdG), an oxidative product of damaged DNA, has also been detected in the blood and urine of PD patients (Roede et al., 2013). On the contrary, the high levels of antioxidants could lower the occurrence and slow the progression of the neurodegenerative disease (Ascherio et al., 2006). Except for metabolic alteration related to mitochondrial oxidation, recent research about metabolomic analysis of cell lysates showed that PD patients present with an increase of lactic acid and a depletion of pyruvic acid and aberrant choline metabolism in extracellular fluid (Amo et al., 2019). Reports have shown that acylcarnitine, as the essential amino acid for fatty acid transport into mitochondria for energy metabolism, was definite in upregulative stages and potentially effected the structure and function of substantia nigra (Mallah et al., 2019).
Notably, some alterations of oxidative stress metabolites might be used to evaluate different subtypes and stages of the disease. Based on CSF and blood samples from patients with PD, Karsten et al. observed specific increases of mannose and fructose, as well as increased threonic acid and reduced dehydroascorbic acid in early-stage PD patients (Trezzi et al., 2017). These changes in oxidation products could reflect the activation of antioxidative stress responses as a resistance mechanism against neuronal injury, in contrast to which, the failure in antioxidant reserve could aggregate the neurodegeneration (Dunn et al., 2014). Significant increases were seen in pyroglutamate and 2-oxoisocaproate and decreases in 3-hydroxyisovalerate, tryptophan, and creatinine, which supported an increase of marks in oxidative responses in preclinical PD (Liu and Wang, 2014). Additionally, some metabolites have also been identified as indicators of the severity of Parkinson’s disease, including uric acid and taurine (Engelborghs et al., 2003).
As we all know, gastrointestinal microbes and host usually remain in a mutualistic relationship, in which the microbes keep its diversification and function via the gut to absorb nutrition. In turn, the parasitic microbiota parasitize in the digestive tract and produce a series of biochemical compounds to contribute physical and bioactive barriers or trigger protective immune responses to withstand the effect of exogenous factors (Reza et al., 2019; Parker et al., 2020). Accumulating evidence suggests that many diseases have specific microbiome profiles and potentially communicate mechanisms between the gastrointestinal and the nervous systems, so alterations in gut microbiota have been linked to neurodegeneration, including AD, PD, and Multiple Sclerosis (Sasmita, 2019).
Over the last two decades, neurologists have begun to explore in detail the relationship between the gastrointestinal tract, gut microbiota, and the central nerve systems (CNS). In the last several years, the gut and related microbiome have gained increasing attention because of its close relationship with the etiology of PD. Clinical evidence revealed that neuropathological changes in PD are accompanied by varying symptoms of gastrointestinal dysfunction (indigestion, constipation, bloating, and dysbacteriosis) before the onset of motor symptoms. Experimental evidence showed that bacterial abnormalities and intestinal pathology may play a role in PD symptoms (Fasano et al., 2013; Tan et al., 2015; Van Laar et al., 2019; Mertsalmi et al., 2020). In recent reports, the gut and relevant metabolic products have been given increasing attention because of their importance in the disease pathogenesis (Sampson et al., 2016; Kim et al., 2019), showing that PD patients usually show significant changes in microbiotal abundance and diversity, as well as distinctive profiles of microbiota composition and intestinal metabolites (Keshavarzian et al., 2015; Vascellari et al., 2020; Wallen et al., 2020; Table 1). Although these microbiota composition alterations are heterogeneous, both microbiota disorders and intestinal damage could act as triggering events that lead to dopaminergic loss and pathological a-Syn (Matheoud et al., 2019). Further, numerous experimental and clinical reports indicated that the a-Syn could gather and spread from the gastrointestine to the deep brain (Braak et al., 2003; Kim et al., 2019; Van Den Berge et al., 2019). Notably, the alteration in microbiota abundance was noted in different subtypes and stages of the disease. A study has demonstrated that the abundancy of some microbial compositions, such as Lactococcus, Faecalibacterium, and Leptotrichia, was increased in early-stages of PD, while Comamonas was common in patients with late-onset symptoms. The abundance of Bacteroidetes and Firmicutes were significantly increased in patients with motor-symptoms (Keshavarzian et al., 2015; Lin et al., 2018). Likewise, decreased Prevotellaceae abundance and increased Enterobacteriaceae may have a positive association with intestinal dysfunction in PD patients. Keeping this point in mind, we know that the dysbacteriosis and microbiota metabolomics have potential relevance to the existence of gastrointestinal a-Syn and pathology. The understanding of microbiota metabolomics is essential for exploring the pathogenesis of PD and seeking specific biomarkers that support a more accurate assessment, earlier diagnosis, and better monitoring of the disease progression.
To our knowledge, gut microbiota contributes to host metabolism in the regulation of organic energy metabolism (e.g., lipids, amino acids, and vitamins), as well as to the differentiation and function of immune cells (Cani, 2018). The specific microbial metabolites are disordered when gut microbes are out of balance in abundance and diversity (Table 2). Based on previous studies, the PD-related dysbacteriosis could induce changes in carbohydrate fermentation, protein, and lipid metabolism which could generate SCFA, p-cresol and phenylacetylglutamine, protocatechuic acid, secondary bile acids, and other metabolites (Wahlstrom et al., 2016; Murota et al., 2018; Cirstea et al., 2020). Specifically, the concentration of short chain fatty acids (SCFA) have largely implicated a significant correlation between gut microbiota and PD, and has been implicated as a driver of the onset and progression of PD (Qiao et al., 2020). The SCFA is a metabolic product that possesses anti-inflammatory and anti-microbial function qualities and protects from intestinal permeability, oxidative stress, and immune injury (Donohoe et al., 2011; Sanchez-Guajardo et al., 2015). Further, the SCFA contain a functional composition—Butyrate—that not only supplies the main source of energy for the gut epithelium, but also strengthens the gastrointestinal barrier function (Volf et al., 2016; Agusti et al., 2018). Therefore, the lower abundance of the microbes that produce SCFA could have negative effects for the intestinal barrier and immune function to induce gastrointestinal symptoms of PD, including constipation, intestinal inflammation, and intestinal barrier leakiness (Segain et al., 2000). From what has been discussed above, the metabolic changes of SCFA caused by gut microbial dysbiosis may be a biomarker for better evaluation of PD conditions.
Collectively, these findings may mark a new step on the path toward the metabolomics of PD. Paralleling with the availability of test samples and advances in identification technology, metabolomics has been considerably applied as a tool in PD research (Koeth et al., 2013; Pannkuk et al., 2015). However, due to the heterogeneity of humans in regards to genetic expression, dietary habit, environmental exposure, and physical behaviors, only a few specific biomarkers can currently be recommended in clinical practice. Hence, further works on the correlation between metabolomics and the neurodegenerative disease would be valuable. It is of great clinical significance to discover specific biological markers of PD, so as to early screen high-risk populations and facilitate timely diagnosis and reasonable therapeutics.
YZ drafted the manuscript. TT was responsible for the design and conception of the work. JL, XZ, and DS participated in the discussion about article writing and revision. All authors read and approved the final manuscript.
This study was supported by funds from the National Natural Science Foundation of China (Grant No: U1804171).
The authors declare that the research was conducted in the absence of any commercial or financial relationships that could be construed as a potential conflict of interest.
Agusti, A., Garcia-Pardo, M. P., Lopez-Almela, I., Campillo, I., Maes, M., Romani-Perez, M., et al. (2018). Interplay between the gut-brain axis, obesity and cognitive function. Front. Neurosci. 12:155. doi: 10.3389/fnins.2018.00155
Amo, T., Oji, Y., Saiki, S., and Hattori, N. J. B. (2019). Metabolomic analysis revealed mitochondrial dysfunction and aberrant choline metabolism in MPP-exposed SH-SY5Y cells. Biochem. Biophys. Res. Commun. 519, 540–546. doi: 10.1016/j.bbrc.2019.09.031
Annanmaki, T., Muuronen, A., and Murros, K. (2007). Low plasma uric acid level in Parkinson’s disease. Mov. Disord. 22, 1133–1137. doi: 10.1002/mds.21502
Ascherio, A., LeWitt, P., Watts, A., Kieburtz, K., Rudolph, A., Schwid, S., et al. (2006). “CSF as well as serum urate are predictors of Parkinson’s disease progression,” in Proceedings of the 10th International Conference of Parkinson’s Disease and Movement Disorders, Kyoto.
Ascherio, A., LeWitt, P. A., Xu, K., Eberly, S., Watts, A., Matson, W. R., et al. (2009). Urate as a predictor of the rate of clinical decline in Parkinson disease. Arch. Neurol. 66, 1460–1468. doi: 10.1001/archneurol.2009.247
Auluck, P. K., Chan, H. Y., Trojanowski, J. Q., Lee, V. M., and Bonini, N. M. (2002). Chaperone suppression of alpha-synuclein toxicity in a Drosophila model for Parkinson’s disease. Science 295, 865–868. doi: 10.1126/science.1067389
Bae, E. J., and Lee, S. J. (2019). The LRRK2-RAB axis in regulation of vesicle trafficking and alpha-synuclein propagation. Biochim. Biophys. Acta Mol. Basis Dis. 1866:165632. doi: 10.1016/j.bbadis.2019.165632
Barcelo-Coblijn, G., Golovko, M. Y., Weinhofer, I., Berger, J., and Murphy, E. J. (2007). Brain neutral lipids mass is increased in alpha-synuclein gene-ablated mice. J. Neurochem. 101, 132–141. doi: 10.1111/j.1471-4159.2006.04348.x
Barodia, S. K., Creed, R. B., and Goldberg, M. S. (2017). Parkin and PINK1 functions in oxidative stress and neurodegeneration. Brain Res. Bull. 133, 51–59. doi: 10.1016/j.brainresbull.2016.12.004
Bazinet, R. P., and Laye, S. (2014). Polyunsaturated fatty acids and their metabolites in brain function and disease. Nat. Rev. Neurosci. 15, 771–785. doi: 10.1038/nrn3820
Bhinderwala, F., Lei, S., Woods, J., Rose, J., Marshall, D. D., Riekeberg, E., et al. (2019). Metabolomics analyses from tissues in Parkinson’s disease. Methods Mol. Biol. 1996, 217–257. doi: 10.1007/978-1-4939-9488-5_19
Bogdanov, M., Matson, W. R., Wang, L., Matson, T., Saunders-Pullman, R., Bressman, S. S., et al. (2008). Metabolomic profiling to develop blood biomarkers for Parkinson’s disease. Brain 131, 389–396. doi: 10.1093/brain/awm304
Boland, B., Yu, W., Corti, O., Mollereau, B., Henriques, A., Bezard, E., et al. (2018). Promoting the clearance of neurotoxic proteins in neurodegenerative disorders of ageing. Nat. Rev. Drug Discov. 17, 660–688. doi: 10.1038/nrd.2018.109
Bolner, A., Pilleri, M., De Riva, V., and Nordera, G. P. (2011). Plasma and urinary HPLC-ED determination of the ratio of 8-OHdG/2-dG in Parkinson’s disease. Clin. Lab. 57, 859–866.
Bose, A., and Beal, M. F. (2016). Mitochondrial dysfunction in Parkinson’s disease. J. Neurochem. 139(Suppl. 1) 216–231. doi: 10.1111/jnc.13731
Bove, J., and Perier, C. (2012). Neurotoxin-based models of Parkinson’s disease. Neuroscience 211, 51–76. doi: 10.1016/j.neuroscience.2011.10.057
Braak, H., Del Tredici, K., Rub, U., de Vos, R. A., Jansen Steur, E. N., and Braak, E. (2003). Staging of brain pathology related to sporadic Parkinson’s disease. Neurobiol. Aging 24, 197–211. doi: 10.1016/s0197-4580(02)00065-9
Cani, P. D. (2018). Human gut microbiome: hopes, threats and promises. Gut 67, 1716–1725. doi: 10.1136/gutjnl-2018-316723
Castagnet, P. I., Golovko, M. Y., Barcelo-Coblijn, G. C., Nussbaum, R. L., and Murphy, E. J. (2005). Fatty acid incorporation is decreased in astrocytes cultured from alpha-synuclein gene-ablated mice. J. Neurochem. 94, 839–849. doi: 10.1111/j.1471-4159.2005.03247.x
Chang, K. H., Cheng, M. L., Tang, H. Y., Huang, C. Y., Wu, Y. R., and Chen, C. M. (2018). Alternations of metabolic profile and kynurenine metabolism in the plasma of Parkinson’s Disease. Mol. Neurobiol. 55, 6319–6328. doi: 10.1007/s12035-017-0845-3
Chen, X., Xie, C., Sun, L., and Cai, H. (2015). Longitudinal metabolomics profiling of Parkinson’s disease-related alpha-synuclein A53T transgenic mice. PLoS One 10:e0136612. doi: 10.1371/journal.pone.0136612
Cirstea, M., Yu, A., Golz, E., Sundvick, K., Kliger, D., Radisavljevic, N., et al. (2020). Microbiota composition and metabolism are associated with gut function in Parkinson’s disease. Mov. Disord. 35, 1208–1217. doi: 10.1002/mds.28052
Correia, C. N., Nalpas, N. C., McLoughlin, K. E., Browne, J. A., Gordon, S. V., MacHugh, D. E., et al. (2017). Circulating microRNAs as potential biomarkers of infectious disease. Front. Immunol. 8:118. doi: 10.3389/fimmu.2017.00118
De Pablo-Fernández, E., Breen, D. P., Bouloux, P., Barker, R., Foltynie, T., Warner, T., et al. (2017). Neuroendocrine abnormalities in Parkinson’s disease. J. Neurol. Neurosurg. Psychiatry 88, 176–185. doi: 10.1136/jnnp-2016-314601
De Virgilio, A., Greco, A., Fabbrini, G., Inghilleri, M., Rizzo, M. I., Gallo, A., et al. (2016). Parkinson’s disease: autoimmunity and neuroinflammation. Autoimmun. Rev. 15, 1005–1011. doi: 10.1016/j.autrev.2016.07.022
Delenclos, M., Jones, D. R., McLean, P. J., and Uitti, R. J. (2016). Biomarkers in Parkinson’s disease: advances and strategies. Parkinsonism Relat. Disord. 22(Suppl. 1) S106–S110. doi: 10.1016/j.parkreldis.2015.09.048
Di Maio, R., Barrett, P. J., Hoffman, E. K., Barrett, C. W., Zharikov, A., Borah, A., et al. (2016). Alpha-synuclein binds to TOM20 and inhibits mitochondrial protein import in Parkinson’s disease. Sci. Transl. Med. 8:342ra78. doi: 10.1126/scitranslmed.aaf3634
Djordjevic, J., Roy Chowdhury, S., Snow, W. M., Perez, C., Cadonic, C., Fernyhough, P., et al. (2020). Early onset of sex-dependent mitochondrial deficits in the cortex of 3xTg Alzheimer’s mice. Cells 9:1541. doi: 10.3390/cells9061541
Dong, M. X., Feng, X., Xu, X. M., Hu, L., Liu, Y., Jia, S. Y., et al. (2018). Integrated analysis reveals altered lipid and glucose metabolism and identifies NOTCH2 as a biomarker for Parkinson’s disease related depression. Front. Mol. Neurosci. 11:257. doi: 10.3389/fnmol.2018.00257
Donohoe, D., Garge, N., Zhang, X., Sun, W., O’Connell, T., Bunger, M., et al. (2011). The microbiome and butyrate regulate energy metabolism and autophagy in the mammalian colon. Cell Metab. 13, 517–526. doi: 10.1016/j.cmet.2011.02.018
Dunn, L., Allen, G., Mamais, A., Ling, H., Li, A., Duberley, K., et al. (2014). Dysregulation of glucose metabolism is an early event in sporadic Parkinson’s disease. Neurobiol. Aging 35, 1111–1115. doi: 10.1016/j.neurobiolaging.2013.11.001
Engelborghs, S., Marescau, B., and De Deyn, P. P. (2003). Amino acids and biogenic amines in cerebrospinal fluid of patients with Parkinson’s disease. Neurochem. Res. 28, 1145–1150. doi: 10.1023/a:1024255208563
Fasano, A., Bove, F., Gabrielli, M., Petracca, M., Zocco, M., Ragazzoni, E., et al. (2013). The role of small intestinal bacterial overgrowth in Parkinson’s disease. Mov. Disord. 28, 1241–1249. doi: 10.1002/mds.25522
Fitzgerald, J. C., Zimprich, A., Carvajal Berrio, D. A., Schindler, K. M., Maurer, B., Schulte, C., et al. (2017). Metformin reverses TRAP1 mutation-associated alterations in mitochondrial function in Parkinson’s disease. Brain 140, 2444–2459. doi: 10.1093/brain/awx202
Gatto, N. M., Rhodes, S. L., Manthripragada, A. D., Bronstein, J., Cockburn, M., Farrer, M., et al. (2010). Alpha-synuclein gene may interact with environmental factors in increasing risk of Parkinson’s disease. Neuroepidemiology 35, 191–195. doi: 10.1159/000315157
GBD 2015 Neurological Disorders Collaborator Group (2017). Global, regional, and national burden of neurological disorders during 1990-2015: a systematic analysis for the Global Burden of Disease Study 2015. Lancet Neurol. 16, 877–897. doi: 10.1016/S1474-4422(17)30299-5
GBD 2016 Parkinson’s Disease Collaborators (2018). Global, regional, and national burden of Parkinson’s disease, 1990-2016: a systematic analysis for the Global Burden of Disease Study 2016. Lancet Neurol. 17, 939–953. doi: 10.1016/S1474-4422(18)30295-3
Gerez, J. A., Prymaczok, N. C., Rockenstein, E., Herrmann, U. S., Schwarz, P., Adame, A., et al. (2019). A cullin-RING ubiquitin ligase targets exogenous alpha-synuclein and inhibits Lewy body-like pathology. Sci. Transl. Med. 11:eaau6722. doi: 10.1126/scitranslmed.aau6722
Goldman, J. G., Andrews, H., Amara, A., Naito, A., Alcalay, R. N., Shaw, L. M., et al. (2018). Cerebrospinal fluid, plasma, and saliva in the BioFIND study: relationships among biomarkers and Parkinson’s disease features. Mov. Disord. 33, 282–288. doi: 10.1002/mds.27232
Golovko, M. Y., Barceló-Coblijn, G., Castagnet, P. I., Austin, S., Combs, C. K., and Murphy, E. J. (2009). The role of α-synuclein in brain lipid metabolism: a downstream impact on brain inflammatory response. Mol. Cell. Biochem. 326, 55–66. doi: 10.1007/s11010-008-0008-y
Golovko, M. Y., Rosenberger, T. A., Feddersen, S., Faergeman, N. J., and Murphy, E. J. (2007). Alpha-synuclein gene ablation increases docosahexaenoic acid incorporation and turnover in brain phospholipids. J. Neurochem. 101, 201–211. doi: 10.1111/j.1471-4159.2006.04357.x
Gonzalez-Casacuberta, I., Juarez-Flores, D. L., Moren, C., and Garrabou, G. (2019). Bioenergetics and autophagic imbalance in patients-derived cell models of Parkinson disease supports systemic dysfunction in neurodegeneration. Front. Neurosci. 13:894. doi: 10.3389/fnins.2019.00894
Graham, S. F., Rey, N. L., Yilmaz, A., Kumar, P., Madaj, Z., Maddens, M., et al. (2018). Biochemical profiling of the brain and blood metabolome in a mouse model of prodromal Parkinson’s disease reveals distinct metabolic profiles. J. Proteome Res. 17, 2460–2469. doi: 10.1021/acs.jproteome.8b00224
Grassi, D., Howard, S., Zhou, M., Diaz-Perez, N., Urban, N. T., Guerrero-Given, D., et al. (2018). Identification of a highly neurotoxic alpha-synuclein species inducing mitochondrial damage and mitophagy in Parkinson’s disease. Proc. Natl. Acad. Sci. U.S.A. 115, E2634–E2643. doi: 10.1073/pnas.1713849115
Greuel, A., Trezzi, J., Glaab, E., Ruppert, M., Maier, F., Jäger, C., et al. (2020). GBA variants in Parkinson’s disease: clinical, metabolomic, and multimodal neuroimaging phenotypes. Mov. Disord. 35, 2201–2210. doi: 10.1002/mds.28225
Guaitoli, G., Raimondi, F., Gilsbach, B. K., Gomez-Llorente, Y., Deyaert, E., Renzi, F., et al. (2016). Structural model of the dimeric Parkinson’s protein LRRK2 reveals a compact architecture involving distant interdomain contacts. Proc. Natl. Acad. Sci. U.S.A. 113, E4357–E4366. doi: 10.1073/pnas.1523708113
Haas, R. H., Nasirian, F., Nakano, K., Ward, D., Pay, M., Hill, R., et al. (1995). Low platelet mitochondrial complex I and complex II/III activity in early untreated Parkinson’s disease. Ann. Neurol. 37, 714–722.
Hatano, T., Saiki, S., Okuzumi, A., Mohney, R. P., and Hattori, N. (2016). Identification of novel biomarkers for Parkinson’s disease by metabolomic technologies. J. Neurol. Neurosurg. Psychiatry 87, 295–301. doi: 10.1136/jnnp-2014-309676
Hernandez, D. G., Reed, X., and Singleton, A. B. (2016). Genetics in Parkinson disease: mendelian versus non-mendelian inheritance. J. Neurochem. 139(Suppl. 1) 59–74. doi: 10.1111/jnc.13593
Hou, Y., Dan, X., Babbar, M., Wei, Y., Hasselbalch, S. G., Croteau, D. L., et al. (2019). Ageing as a risk factor for neurodegenerative disease. Nat. Rev. Neurol. 15, 565–581. doi: 10.1038/s41582-019-0244-7
Infante, J., Prieto, C., Sierra, M., Sanchez-Juan, P., Gonzalez-Aramburu, I., Sanchez-Quintana, C., et al. (2015). Identification of candidate genes for Parkinson’s disease through blood transcriptome analysis in LRRK2-G2019S carriers, idiopathic cases, and controls. Neurobiol. Aging 36, 1105–1109. doi: 10.1016/j.neurobiolaging.2014.10.039
Johansen, K. K., Wang, L., Aasly, J. O., White, L. R., Matson, W. R., Henchcliffe, C., et al. (2009). Metabolomic profiling in LRRK2-related Parkinson’s disease. PLoS One 4:e7551. doi: 10.1371/journal.pone.0007551
Kahle, P. J., Neumann, M., Ozmen, L., Muller, V., Odoy, S., Okamoto, N., et al. (2001). Selective insolubility of alpha-synuclein in human Lewy body diseases is recapitulated in a transgenic mouse model. Am. J. Pathol. 159, 2215–2225. doi: 10.1016/s0002-9440(10)63072-6
Kalim, S., and Rhee, E. P. (2017). An overview of renal metabolomics. Kidney Int. 91, 61–69. doi: 10.1016/j.kint.2016.08.021
Keshavarzian, A., Green, S. J., Engen, P. A., Voigt, R. M., Naqib, A., Forsyth, C. B., et al. (2015). Colonic bacterial composition in Parkinson’s disease. Mov. Disord. 30, 1351–1360. doi: 10.1002/mds.26307
Kim, C. Y., and Alcalay, R. N. (2017). Genetic forms of Parkinson’s disease. Semin. Neurol. 37, 135–146. doi: 10.1055/s-0037-1601567
Kim, M. J., Oh, S. B., Kim, J., Kim, K., Ryu, H. S., Kim, M. S., et al. (2018). Association of metals with the risk and clinical characteristics of Parkinson’s disease. Parkinsonism Relat. Disord. 55, 117–121. doi: 10.1016/j.parkreldis.2018.05.022
Kim, S., Kwon, S.-H., Kam, T.-I., Panicker, N., Karuppagounder, S. S., Lee, S., et al. (2019). Transneuronal propagation of pathologic α-synuclein from the gut to the brain models Parkinson’s disease. Neuron 103, 627–641.e7.
Kiraly, M., Dalmadine Kiss, B., Vekey, K., Antal, I., and Ludanyi, K. (2016). [Mass spectrometry: past and present]. Acta Pharm. Hung. 86, 3–11.
Klein, C., and Westenberger, A. (2012). Genetics of Parkinson’s disease. Cold Spring Harb. Perspect Med. 2:a008888. doi: 10.1101/cshperspect.a008888
Koeth, R. A., Wang, Z., Levison, B. S., Buffa, J. A., Org, E., Sheehy, B. T., et al. (2013). Intestinal microbiota metabolism of L-carnitine, a nutrient in red meat, promotes atherosclerosis. Nat. Med. 19, 576–585. doi: 10.1038/nm.3145
Koros, C., Simitsi, A., Prentakis, A., Beratis, I., Papadimitriou, D., Kontaxopoulou, D., et al. (2018). 123I-FP-CIT SPECT [(123) I-2beta-carbomethoxy-3beta-(4-iodophenyl)-N-(3-fluoropropyl) nortropane single photon emission computed tomography] imaging in a p.A53T alpha-synuclein Parkinson’s disease cohort versus Parkinson’s disease. Mov. Disord. 33, 1734–1739. doi: 10.1002/mds.27451
Krige, D., Carroll, M. T., Cooper, J. M., Marsden, C. D., and Schapira, A. H. (1992). Platelet mitochondria function in Parkinson’s disease. Ann. Neurol. 32, 782–788.
Kruger, R., Kuhn, W., Muller, T., Woitalla, D., Graeber, M., Kosel, S., et al. (1998). Ala30Pro mutation in the gene encoding alpha-synuclein in Parkinson’s disease. Nat. Genet. 18, 106–108. doi: 10.1038/ng0298-106
Lehmann, S., Loh, S. H., and Martins, L. M. (2017). Enhancing NAD(+) salvage metabolism is neuroprotective in a PINK1 model of Parkinson’s disease. Biol. Open 6, 141–147. doi: 10.1242/bio.022186
Lei, S., Zavala-Flores, L., Garcia-Garcia, A., Nandakumar, R., Huang, Y., Madayiputhiya, N., et al. (2014). Alterations in energy/redox metabolism induced by mitochondrial and environmental toxins: a specific role for glucose-6-phosphate-dehydrogenase and the pentose phosphate pathway in paraquat toxicity. ACS Chem. Biol. 9, 2032–2048. doi: 10.1021/cb400894a
Lewitt, P., Li, J., Lu, M., Beach, T., Adler, C., Guo, L., et al. (2013). 3-hydroxykynurenine and other Parkinson’s disease biomarkers discovered by metabolomic analysis. Mov. Disord. 28, 1653–1660. doi: 10.1002/mds.25555
Lin, A., Zheng, W., He, Y., Tang, W., Wei, X., He, R., et al. (2018). Gut microbiota in patients with Parkinson’s disease in southern China. Parkinsonism Relat. Disord. 53, 82–88. doi: 10.1016/j.parkreldis.2018.05.007
Liu, C. W., Giasson, B. I., Lewis, K. A., Lee, V. M., Demartino, G. N., and Thomas, P. J. (2005). A precipitating role for truncated alpha-synuclein and the proteasome in alpha-synuclein aggregation: implications for pathogenesis of Parkinson disease. J. Biol. Chem. 280, 22670–22678. doi: 10.1074/jbc.M501508200
Liu, G., Boot, B., Locascio, J. J. I, Jansen, E., Winder-Rhodes, S., Eberly, S., et al. (2016). Specifically neuropathic Gaucher’s mutations accelerate cognitive decline in Parkinson’s. Ann. Neurol. 80, 674–685. doi: 10.1002/ana.24781
Liu, J., and Wang, L. N. (2014). Mitochondrial enhancement for neurodegenerative movement disorders: a systematic review of trials involving creatine, coenzyme Q10, idebenone and mitoquinone. CNS Drugs 28, 63–68. doi: 10.1007/s40263-013-0124-4
Loeffler, D. A., Klaver, A. C., Coffey, M. P., Aasly, J. O., and LeWitt, P. A. (2017). Increased oxidative stress markers in cerebrospinal fluid from healthy subjects with Parkinson’s disease-associated LRRK2 gene mutations. Front. Aging Neurosci. 9:89. doi: 10.3389/fnagi.2017.00089
Luan, H., Liu, L. F., Tang, Z., Zhang, M., Chua, K. K., Song, J. X., et al. (2015). Comprehensive urinary metabolomic profiling and identification of potential noninvasive marker for idiopathic Parkinson’s disease. Sci. Rep. 5:13888. doi: 10.1038/srep13888
Ma, J., Gao, J., Wang, J., and Xie, A. (2019). Prion-like mechanisms in Parkinson’s disease. Front. Neurosci. 13:552. doi: 10.3389/fnins.2019.00552
Mallah, K., Quanico, J., Raffo-Romero, A., Cardon, T., Aboulouard, S., Devos, D., et al. (2019). Matrix-assisted laser desorption/ionization-mass spectrometry imaging of lipids in experimental model of traumatic brain injury detecting acylcarnitines as injury related markers. Anal. Chem. 91, 11879–11887. doi: 10.1021/acs.analchem.9b02633
Mallett, V., Ross, J. P., Alcalay, R. N., Ambalavanan, A., Sidransky, E., Dion, P. A., et al. (2016). GBA p.T369M substitution in Parkinson disease: polymorphism or association? A meta-analysis. Neurol. Genet. 2:e104. doi: 10.1212/NXG.0000000000000104
Marcovina, S. M., Sirtori, C., Peracino, A., Gheorghiade, M., Borum, P., Remuzzi, G., et al. (2013). Translating the basic knowledge of mitochondrial functions to metabolic therapy: role of L-carnitine. Transl. Res. 161, 73–84. doi: 10.1016/j.trsl.2012.10.006
Matheoud, D., Cannon, T., Voisin, A., Penttinen, A. M., Ramet, L., Fahmy, A. M., et al. (2019). Intestinal infection triggers Parkinson’s disease-like symptoms in Pink1(-/-) mice. Nature 571, 565–569. doi: 10.1038/s41586-019-1405-y
Matheoud, D., Sugiura, A., Bellemare-Pelletier, A., Laplante, A., Rondeau, C., Chemali, M., et al. (2016). Parkinson’s disease-related proteins PINK1 and Parkin repress mitochondrial antigen presentation. Cell 166, 314–327.
Mertsalmi, T., Pekkonen, E., and Scheperjans, F. (2020). Antibiotic exposure and risk of Parkinson’s disease in Finland: a nationwide case-control study. Mov. Disord. 35, 431–442. doi: 10.1002/mds.27924
Migdalska-Richards, A., and Schapira, A. H. (2016). The relationship between glucocerebrosidase mutations and Parkinson disease. J. Neurochem. 139(Suppl. 1) 77–90. doi: 10.1111/jnc.13385
Murota, K., Nakamura, Y., and Uehara, M. (2018). Flavonoid metabolism: the interaction of metabolites and gut microbiota. Biosci. Biotechnol. Biochem. 82, 600–610. doi: 10.1080/09168451.2018.1444467
Musgrove, R. E., Horne, J., Wilson, R., King, A. E., Edwards, L. M., and Dickson, T. C. (2014). The metabolomics of alpha-synuclein (SNCA) gene deletion and mutation in mouse brain. Metabolomics 10, 114–122. doi: 10.1007/s11306-013-0561-6
Muthukumaran, K., Leahy, S., Harrison, K., Sikorska, M., Sandhu, J. K., Cohen, J., et al. (2014). Orally delivered water soluble coenzyme Q10 (Ubisol-Q10) blocks on-going neurodegeneration in rats exposed to paraquat: potential for therapeutic application in Parkinson’s disease. BMC Neurosci. 15:21. doi: 10.1186/1471-2202-15-21
Nickels, S. L., Walter, J., Bolognin, S., Gérard, D., Jaeger, C., Qing, X., et al. (2019). Impaired serine metabolism complements LRRK2-G2019S pathogenicity in PD patients. Parkinsonism Relat. Disord. 67, 48–55. doi: 10.1016/j.parkreldis.2019.09.018
Okuzumi, A., Hatano, T., Ueno, S. I., Ogawa, T., Saiki, S., Mori, A., et al. (2019). Metabolomics-based identification of metabolic alterations in PARK2. Ann. Clin. Transl. Neurol. 6, 525–536. doi: 10.1002/acn3.724
Ou, R., Cao, B., Wei, Q., Hou, Y., Xu, Y., Song, W., et al. (2017). Serum uric acid levels and freezing of gait in Parkinson’s disease. Neurol. Sci. 38, 955–960. doi: 10.1007/s10072-017-2871-3
Paisan-Ruiz, C., Nath, P., Washecka, N., Gibbs, J. R., and Singleton, A. B. (2008). Comprehensive analysis of LRRK2 in publicly available Parkinson’s disease cases and neurologically normal controls. Hum. Mutat. 29, 485–490. doi: 10.1002/humu.20668
Pannkuk, E. L., Laiakis, E. C., Authier, S., Wong, K., and Fornace, A. J. Jr. (2015). Global metabolomic identification of long-term dose-dependent urinary biomarkers in nonhuman primates exposed to ionizing radiation. Radiat. Res. 184, 121–133. doi: 10.1667/rr14091.1
Parajuli, L. K., Wako, K., Maruo, S., Kakuta, S., Taguchi, T., Ikuno, M., et al. (2020). Developmental changes in dendritic spine morphology in the striatum and their alteration in an A53T alpha-synuclein transgenic mouse model of Parkinson’s disease. eNeuro 7:ENEURO.0072-20.2020. doi: 10.1523/ENEURO.0072-20.2020
Parker, A., Fonseca, S., and Carding, S. R. (2020). Gut microbes and metabolites as modulators of blood-brain barrier integrity and brain health. Gut Microbes 11, 135–157. doi: 10.1080/19490976.2019.1638722
Penn, A., Roberts, T., Hodder, J., Allen, P., Zhu, G., and Martin, W. J. N. (1995). Generalized mitochondrial dysfunction in Parkinson’s disease detected by magnetic resonance spectroscopy of muscle. Neurology 45, 2097–2099.
Pickrell, A. M., and Youle, R. J. (2015). The roles of PINK1, parkin, and mitochondrial fidelity in Parkinson’s disease. Neuron 85, 257–273. doi: 10.1016/j.neuron.2014.12.007
Polymeropoulos, M. H., Lavedan, C., Leroy, E., Ide, S. E., Dehejia, A., Dutra, A., et al. (1997). Mutation in the alpha-synuclein gene identified in families with Parkinson’s disease. Science 276, 2045–2047. doi: 10.1126/science.276.5321.2045
Puspita, L., Chung, S. Y., and Shim, J. W. (2017). Oxidative stress and cellular pathologies in Parkinson’s disease. Mol. Brain 10:53. doi: 10.1186/s13041-017-0340-9
Qamhawi, Z., Towey, D., Shah, B., Pagano, G., Seibyl, J., Marek, K., et al. (2015). Clinical correlates of raphe serotonergic dysfunction in early Parkinson’s disease. Brain 138, 2964–2973. doi: 10.1093/brain/awv215
Qiao, C., Sun, M., Jia, X., Shi, Y., Zhang, B., Zhou, Z., et al. (2020). Sodium butyrate causes α-synuclein degradation by an Atg5-dependent and PI3K/Akt/mTOR-related autophagy pathway. Exp. Cell Res. 387:111772. doi: 10.1016/j.yexcr.2019.111772
Reza, M., Finlay, B., and Pettersson, S. (2019). Gut microbes, ageing & organ function: a chameleon in modern biology? EMBO Mol. Med. 11:e9872. doi: 10.15252/emmm.201809872
Rocha, E. M., De Miranda, B., and Sanders, L. H. (2018). Alpha-synuclein: pathology, mitochondrial dysfunction and neuroinflammation in Parkinson’s disease. Neurobiol. Dis. 109, 249–257. doi: 10.1016/j.nbd.2017.04.004
Roede, J., Uppal, K., Park, Y., Lee, K., Tran, V., Walker, D., et al. (2013). Serum metabolomics of slow vs. rapid motor progression Parkinson’s disease: a pilot study. PLoS One 8:e77629. doi: 10.1371/journal.pone.0077629
Saiki, S., Hatano, T., Fujimaki, M., Ishikawa, K. I., Mori, A., Oji, Y., et al. (2017). Decreased long-chain acylcarnitines from insufficient beta-oxidation as potential early diagnostic markers for Parkinson’s disease. Sci. Rep. 7:7328. doi: 10.1038/s41598-017-06767-y
Sampson, T. R., Debelius, J. W., Thron, T., Janssen, S., Shastri, G. G., Ilhan, Z. E., et al. (2016). Gut microbiota regulate motor deficits and neuroinflammation in a model of Parkinson’s disease. Cell 167, 1469–1480.e12.
Sanchez-Guajardo, V., Tentillier, N., and Romero-Ramos, M. J. N. (2015). The relation between α-synuclein and microglia in Parkinson’s disease: recent developments. Neuroscience 302, 47–58. doi: 10.1016/j.neuroscience.2015.02.008
Santos Garcia, D., de Deus Fonticoba, T., Suarez Castro, E., Borrue, C., Mata, M., Solano Vila, B., et al. (2019). Non-motor symptoms burden, mood, and gait problems are the most significant factors contributing to a poor quality of life in non-demented Parkinson’s disease patients: results from the COPPADIS study cohort. Parkinsonism Relat. Disord. 66, 151–157. doi: 10.1016/j.parkreldis.2019.07.031
Sasmita, A. O. (2019). Modification of the gut microbiome to combat neurodegeneration. Rev. Neurosci. 30, 795–805. doi: 10.1515/revneuro-2019-0005
Scheperjans, F., Aho, V., Pereira, P. A., Koskinen, K., Paulin, L., Pekkonen, E., et al. (2015). Gut microbiota are related to Parkinson’s disease and clinical phenotype. Mov. Disord. 30, 350–358. doi: 10.1002/mds.26069
Segain, J., Raingeard de la Blétière, D., Bourreille, A., Leray, V., Gervois, N., Rosales, C., et al. (2000). Butyrate inhibits inflammatory responses through NFkappaB inhibition: implications for Crohn’s disease. Gut 47, 397–403. doi: 10.1136/gut.47.3.397
Sidransky, E., and Lopez, G. (2012). The link between the GBA gene and parkinsonism. Lancet Neurol. 11, 986–998. doi: 10.1016/S1474-4422(12)70190-4
Singleton, A. B., Farrer, M. J., and Bonifati, V. (2013). The genetics of Parkinson’s disease: progress and therapeutic implications. Mov. Disord. 28, 14–23.
Spencer, B., Emadi, S., Desplats, P., Eleuteri, S., Michael, S., Kosberg, K., et al. (2014). ESCRT-mediated uptake and degradation of brain-targeted α-synuclein single chain antibody attenuates neuronal degeneration in vivo. Mol. Ther. 22, 1753–1767. doi: 10.1038/mt.2014.129
Spillantini, M. G., and Goedert, M. (2018). Neurodegeneration and the ordered assembly of α-synuclein. Cell Tissue Res. 373, 137–148.
Spillantini, M. G., Schmidt, M. L., Lee, V. M.-Y., Trojanowski, J. Q., Jakes, R., and Goedert, M. J. N. (1997). α-Synuclein in Lewy bodies. Nature 388, 839–840.
Stauch, K. L., Villeneuve, L. M., Purnell, P. R., Ottemann, B. M., Emanuel, K., and Fox, H. S. (2016). Loss of Pink1 modulates synaptic mitochondrial bioenergetics in the rat striatum prior to motor symptoms: concomitant complex I respiratory defects and increased complex II-mediated respiration. Proteomics Clin. Appl. 10, 1205–1217. doi: 10.1002/prca.201600005
Stoessel, D., Schulte, C., Teixeira Dos Santos, M. C., Scheller, D., Rebollo-Mesa, I., Deuschle, C., et al. (2018). Promising metabolite profiles in the plasma and CSF of early clinical Parkinson’s disease. Front. Aging Neurosci. 10:51. doi: 10.3389/fnagi.2018.00051
Sugeno, N., Hasegawa, T., Tanaka, N., Fukuda, M., Wakabayashi, K., Oshima, R., et al. (2014). Lys-63-linked ubiquitination by E3 ubiquitin ligase Nedd4-1 facilitates endosomal sequestration of internalized α-synuclein. J. Biol. Chem. 289, 18137–18151. doi: 10.1074/jbc.M113.529461
Tan, A., Mahadeva, S., Marras, C., Thalha, A., Kiew, C., Yeat, C., et al. (2015). Helicobacter pylori infection is associated with worse severity of Parkinson’s disease. Parkinsonism Relat. Disord. 21, 221–225. doi: 10.1016/j.parkreldis.2014.12.009
Thellung, S., Corsaro, A., Nizzari, M., Barbieri, F., and Florio, T. (2019). Autophagy activator drugs: a new opportunity in neuroprotection from misfolded protein toxicity. Int. J. Mol. Sci. 20:901. doi: 10.3390/ijms20040901
Tofaris, G., Layfield, R., and Spillantini, M. (2001). Alpha-synuclein metabolism and aggregation is linked to ubiquitin-independent degradation by the proteasome. FEBS Lett. 509, 22–26. doi: 10.1016/s0014-5793(01)03115-5
Trezzi, J. P., Galozzi, S., Jaeger, C., Barkovits, K., Brockmann, K., Maetzler, W., et al. (2017). Distinct metabolomic signature in cerebrospinal fluid in early Parkinson’s disease. Mov. Disord. 32, 1401–1408. doi: 10.1002/mds.27132
Troisi, J., Landolfi, A., Vitale, C., Longo, K., Cozzolino, A., Squillante, M., et al. (2019). A metabolomic signature of treated and drug-naive patients with Parkinson’s disease: a pilot study. Metabolomics 15:90. doi: 10.1007/s11306-019-1554-x
Trupp, M., Jonsson, P., Ohrfelt, A., Zetterberg, H., Obudulu, O., Malm, L., et al. (2014). Metabolite and peptide levels in plasma and CSF differentiating healthy controls from patients with newly diagnosed Parkinson’s disease. J. Parkinsons Dis. 4, 549–560. doi: 10.3233/JPD-140389
Tufi, R., Gandhi, S., de Castro, I. P., Lehmann, S., Angelova, P. R., Dinsdale, D., et al. (2014). Enhancing nucleotide metabolism protects against mitochondrial dysfunction and neurodegeneration in a PINK1 model of Parkinson’s disease. Nat. Cell Biol. 16, 157–166. doi: 10.1038/ncb2901
Tuttle, M. D., Comellas, G., Nieuwkoop, A. J., Covell, D. J., Berthold, D. A., Kloepper, K. D., et al. (2016). Solid-state NMR structure of a pathogenic fibril of full-length human α-synuclein. Nat. Struct. Mol. Biol. 23, 409–415.
Tyurina, Y. Y., Polimova, A. M., Maciel, E., Tyurin, V. A., Kapralova, V. I., Winnica, D. E., et al. (2015). LC/MS analysis of cardiolipins in substantia nigra and plasma of rotenone-treated rats: implication for mitochondrial dysfunction in Parkinson’s disease. Free Radic. Res. 49, 681–691. doi: 10.3109/10715762.2015.1005085
Uemura, N., Koike, M., Ansai, S., Kinoshita, M., Ishikawa-Fujiwara, T., Matsui, H., et al. (2015). Viable neuronopathic Gaucher disease model in medaka (Oryzias latipes) displays axonal accumulation of alpha-synuclein. PLoS Genet. 11:e1005065. doi: 10.1371/journal.pgen.1005065
Ueno, S. I., Hatano, T., Okuzumi, A., Saiki, S., Oji, Y., Mori, A., et al. (2020). Nonmercaptalbumin as an oxidative stress marker in Parkinson’s and PARK2 disease. Ann. Clin. Transl. Neurol. 7, 307–317. doi: 10.1002/acn3.50990
Van Den Berge, N., Ferreira, N., Gram, H., Mikkelsen, T. W., Alstrup, A. K. O., Casadei, N., et al. (2019). Evidence for bidirectional and trans-synaptic parasympathetic and sympathetic propagation of alpha-synuclein in rats. Acta Neuropathol. 138, 535–550. doi: 10.1007/s00401-019-02040-w
Van Laar, T., Boertien, J., and Herranz, A. H. (2019). Faecal transplantation, pro- and prebiotics in Parkinson’s disease; hope or hype? J. Parkinsons Dis. 9, S371–S379. doi: 10.3233/jpd-191802
Vascellari, S., Palmas, V., Melis, M., Pisanu, S., Cusano, R., Uva, P., et al. (2020). Gut microbiota and metabolome alterations associated with Parkinson’s disease. mSystems 5:e00561-20.
Volf, J., Polansky, O., Varmuzova, K., Gerzova, L., Sekelova, Z., Faldynova, M., et al. (2016). Transient and prolonged response of chicken cecum mucosa to colonization with different gut microbiota. PLoS One 11:e0163932. doi: 10.1371/journal.pone.0163932
Wahlstrom, A., Sayin, S. I., Marschall, H. U., and Backhed, F. (2016). Intestinal crosstalk between bile acids and microbiota and its impact on host metabolism. Cell Metab. 24, 41–50. doi: 10.1016/j.cmet.2016.05.005
Wallen, Z., Appah, M., Dean, M., Sesler, C., Factor, S., Molho, E., et al. (2020). Characterizing dysbiosis of gut microbiome in PD: evidence for overabundance of opportunistic pathogens. NPJ Parkinsons Dis. 6:11. doi: 10.1038/s41531-020-0112-6
Wile, D. J., Agarwal, P. A., Schulzer, M., Mak, E., Dinelle, K., Shahinfard, E., et al. (2017). Serotonin and dopamine transporter PET changes in the premotor phase of LRRK2 parkinsonism: cross-sectional studies. Lancet Neurol. 16, 351–359. doi: 10.1016/S1474-4422(17)30056-X
Willis, G. L., Boda, J., and Freelance, C. B. (2018). Polychromatic light exposure as a therapeutic in the treatment and management of Parkinson’s disease: a controlled exploratory trial. Front. Neurol. 9:741. doi: 10.3389/fneur.2018.00741
Willkommen, D., Lucio, M., Moritz, F., Forcisi, S., Kanawati, B., Smirnov, K. S., et al. (2018). Metabolomic investigations in cerebrospinal fluid of Parkinson’s disease. PLoS One 13:e0208752. doi: 10.1371/journal.pone.0208752
Wilson, H., Dervenoulas, G., Pagano, G., Koros, C., Yousaf, T., Picillo, M., et al. (2019). Serotonergic pathology and disease burden in the premotor and motor phase of A53T alpha-synuclein parkinsonism: a cross-sectional study. Lancet Neurol. 18, 748–759. doi: 10.1016/S1474-4422(19)30140-1
Wilson, H., Giordano, B., Turkheimer, F. E., Chaudhuri, K. R., and Politis, M. (2018). Serotonergic dysregulation is linked to sleep problems in Parkinson’s disease. Neuroimage Clin. 18, 630–637. doi: 10.1016/j.nicl.2018.03.001
Xu, L., Lee, J. R., Hao, S., Ling, X. B., Brooks, J. D., Wang, S. X., et al. (2019). Improved detection of prostate cancer using a magneto-nanosensor assay for serum circulating autoantibodies. PLoS One 14:e0221051. doi: 10.1371/journal.pone.0221051
Younes-Mhenni, S., Frih-Ayed, M., Kerkeni, A., Bost, M., and Chazot, G. (2007). Peripheral blood markers of oxidative stress in Parkinson’s disease. Eur. Neurol. 58, 78–83.
Zampieri, S., Cattarossi, S., Bembi, B., and Dardis, A. (2017). GBA analysis in next-generation era: pitfalls, challenges, and possible solutions. J. Mol. Diagn. 19, 733–741. doi: 10.1016/j.jmoldx.2017.05.005
Zarranz, J. J., Alegre, J., Gomez-Esteban, J. C., Lezcano, E., Ros, R., Ampuero, I., et al. (2004). The new mutation, E46K, of alpha-synuclein causes Parkinson and Lewy body dementia. Ann. Neurol. 55, 164–173. doi: 10.1002/ana.10795
Keywords: Parkinson disease, metabolomics, genetic mutations, mitochondrial dysfunction, dysbacteriosis
Citation: Zhang Y, Li J, Zhang X, Song D and Tian T (2021) Advances of Mechanisms-Related Metabolomics in Parkinson’s Disease. Front. Neurosci. 15:614251. doi: 10.3389/fnins.2021.614251
Received: 05 October 2020; Accepted: 11 January 2021;
Published: 03 February 2021.
Edited by:
Deepti Lall, Cedars Sinai Medical Center, United StatesReviewed by:
Maria Shadrina, Institute of Molecular Genetics (RAS), RussiaCopyright © 2021 Zhang, Li, Zhang, Song and Tian. This is an open-access article distributed under the terms of the Creative Commons Attribution License (CC BY). The use, distribution or reproduction in other forums is permitted, provided the original author(s) and the copyright owner(s) are credited and that the original publication in this journal is cited, in accordance with accepted academic practice. No use, distribution or reproduction is permitted which does not comply with these terms.
*Correspondence: Tian Tian, ZmNjdGlhbnRAenp1LmVkdS5jbg==
Disclaimer: All claims expressed in this article are solely those of the authors and do not necessarily represent those of their affiliated organizations, or those of the publisher, the editors and the reviewers. Any product that may be evaluated in this article or claim that may be made by its manufacturer is not guaranteed or endorsed by the publisher.
Research integrity at Frontiers
Learn more about the work of our research integrity team to safeguard the quality of each article we publish.