- 1Programa de Pós-Graduação em Neurociências, Instituto de Ciências Básicas da Saúde (ICBS), Universidade Federal do Rio Grande do Sul, Porto Alegre, Brazil
- 2Integrated Program in Neuroscience (IPN), McGill University, Montreal, QC, Canada
- 3Department of Psychiatry, Faculty of Medicine, McGill University, Montreal, QC, Canada
- 4Programa de Pós-Graduação em Psicologia, Instituto de Psicologia, Universidade Federal do Rio Grande do Sul, Porto Alegre, Brazil
- 5Ludmer Centre for Neuroinformatics and Mental Health, Douglas Hospital Research Centre, McGill University, Montreal, QC, Canada
- 6Mood Disorders Program, Department of Psychiatry & Behavioural Neurosciences, McMaster University, Hamilton, ON, Canada
- 7Department of Psychology, Neuroscience & Behaviour, McMaster University, Hamilton, ON, Canada
- 8Centre for Molecular Medicine and Therapeutics, BC Children's Hospital Research Institute, Department of Medical Genetics, The University of British Columbia, Vancouver, BC, Canada
- 9Departamento de Ciências Fisiológicas, Universidade Federal do Espírito Santo, Vitoria, Brazil
- 10Singapore Institute for Clinical Sciences, Agency for Science, Technology and Research (A*STAR), Singapore, Singapore
Variations in serotoninergic signaling have been related to behavioral outcomes. Alterations in the genome, such as DNA methylation and histone modifications, are affected by serotonin neurotransmission. The amygdala is an important brain region involved in emotional responses and impulsivity, which receives serotoninergic input. In addition, studies suggest that the serotonin transporter gene network may interact with the environment and influence the risk for psychiatric disorders. We propose to investigate whether/how interactions between the exposure to early life adversity and serotonin transporter gene network in the amygdala associate with behavioral disorders. We constructed a co-expression-based polygenic risk score (ePRS) reflecting variations in the function of the serotonin transporter gene network in the amygdala and investigated its interaction with postnatal adversity on attention problems in two independent cohorts from Canada and Singapore. We also described how interactions between ePRS-5-HTT and postnatal adversity exposure predict brain gray matter density and variation in DNA methylation across the genome. We observed that the expression-based polygenic risk score, reflecting the function of the amygdala 5-HTT gene network, interacts with postnatal adversity, to predict attention and hyperactivity problems across both cohorts. Also, both postnatal adversity score and amygdala ePRS-5-HTT score, as well as their interaction, were observed to be associated with variation in DNA methylation across the genome. Variations in gray matter density in brain regions linked to attentional processes were also correlated to our ePRS score. These results confirm that the amygdala 5-HTT gene network is strongly associated with ADHD-related behaviors, brain cortical density, and epigenetic changes in the context of adversity in young children.
Introduction
Attention Deficit Hyperactivity Disorder (ADHD) is a prevalent psychiatric condition characterized by symptoms of inattention, impulsivity, and hyperactivity (American Psychiatric Association, 2013). ADHD is a developmental disorder with symptoms often evident before 6 years of age (Faraone et al., 2003). Both genetic and environmental factors make a substantial contribution to the etiology of ADHD (Matthews et al., 2014). Childhood adversities are associated with the development of ADHD (Boecker et al., 2014; Björkenstam et al., 2017; Bock et al., 2017) while candidate gene studies suggest that low serotonergic activity is associated with increased impulsive-aggressive behavior (Faraone et al., 2003). Alterations in serotonin synthesis, breakdown and transport have been associated to ADHD-related phenotypes (Oades, 2010).
Serotonin or 5-hydroxytryptamine belongs to the indolamine family of neurotransmitters, and influences physiological processes, including autonomic function, motor activity, hormone secretion, and behavioral processes such as cognition, emotion, reward and attention (Carlsson, 1987; Greengard, 2001). The synthesis of 5-HT occurs in serotonergic neurons (Strüder and Weicker, 2001) where 5-HT is stored into presynaptic vesicles by a vesicular monoamine transporter (Sakowski et al., 2006). After being released, 5-HT is recycled through a process of active re-uptake by the serotonin transporter (5-HTT) (Hoffman et al., 1998). The 5-HTT gene, also known as SLC6A4, is localized in the long arm of chromosome 17. This gene encodes an integral membrane protein that transports the neurotransmitter serotonin from synaptic spaces into presynaptic neurons, and represents a primary mechanism for the regulation of serotonergic activity (Heils et al., 2002). 5-HTT is expressed in brain regions implicated in attention and memory such as the amygdala, hippocampus, and prefrontal cortex (Frankle et al., 2004; Oquendo et al., 2007; Puig and Gulledge, 2011). The amygdala is predominantly modulated by the 5-HT system, and plays an important role in attentional processes (Pessoa, 2011). Several studies have observed that variations specifically in this gene are associated with impulsivity, hyperactivity, and ADHD (Halperin et al., 1997; Manor et al., 2001; Seeger et al., 2001). Likewise, Genetic variation in SLC6A4 also associates with individual differences in DNA methylation, a functional epigenetic modification of DNA, and SLC6A4 gene expression. Interestingly, such genetic effects on DNA methylation emerged as a function of exposure to adversity early in life (Beach et al., 2010; Vijayendran et al., 2012); highlighting the importance of the interplay between the genome and the environment in the regulation of SLC6A4 gene expression.
A large number of studies focuses on heritability and candidate genes involved in ADHD. However, dysfunctions usually involve several molecular pathways that may result from variants in many genes, each of them contributing with only weak effects to the phenotype (Gaiteri et al., 2014). Analyzing the effect of genes co-expressed with a gene of interest allows the integration of information on gene networks and the functional relationships between these genes (Silveira et al., 2017; Miguel et al., 2019). Multivariate approaches like parallel ICA (p-ICA) are used to identify relationships between clusters of functionally related SNPs that are statistically correlated and phenotype components, such as brain structure (Pearlson et al., 2015; Khadka et al., 2016). Studies have been using p-ICA to correlate biological pathways with variations in gray matter density, which represents an important tool to identify an anatomical-functional basis for a behavioral phenotype (Khadka et al., 2016; Miguel et al., 2019). Additionally, stressors in early life are known to alter the expression of genes and cellular processes that influence behavior, and one of the molecular processes that contributes to this change is DNA methylation (Vinkers et al., 2015; Hing et al., 2018). However, the mechanisms linking these associations need to be further investigated.
Based on this evidence, we hypothesized that early life adversity would interact with individual differences in genetic variation within the SLC6A4 gene network to predict attention-related problems in childhood. We constructed an expression-based polygenic risk score (ePRS) that reflects the function of the amygdala 5-HTT gene network, and described how this polygenic predictor interacted with postnatal adversity to predict attention/hyperactivity and brain gray matter density. We also investigated the association between postnatal adversity and the ePRS of the 5-HTT gene network on variations in DNA methylation across the genome.
Methods and Materials
Participants
We used data from two prospective birth cohorts based in Canada (Maternal Adversity, Vulnerability and Neurodevelopment—MAVAN) and in Singapore (Growing Up in Singapore Toward Healthy Outcomes—GUSTO), to analyze the gene network by environment interaction effects on behavioral outcomes.
The study sample MAVAN is a birth cohort that followed up children from birth up to 6 years of age in Montreal (Quebec), and Hamilton (Ontario), Canada (O'Donnell et al., 2014). Mothers aged 18 years or above, with singleton pregnancies, and fluent in French or English were included in the study. Severe maternal chronic illness, placenta previa, and history of incompetent cervix, impending delivery, or a fetus/infant affected by a major anomaly or gestational age <37 weeks were the exclusion criteria. Approval for the MAVAN project was obtained by the ethics committees and university affiliates (McGill University and Université de Montréal, the Royal Victoria Hospital, Jewish General Hospital, Centre hospitalier de l'Université de Montréal and Hôpital Maisonneuve-Rosemount) and St. Joseph's Hospital and McMaster University, Hamilton, Ontario, Canada. Informed consent was obtained from all participants. In GUSTO, the replication cohort, pregnant women aged 18 years and above were recruited at the National University Hospital (NUH) and KK Women's and Children's Hospital (KKH), being of Chinese, Malay or Indian ethnicity with homogeneous parental ethnic background. Mothers receiving chemotherapy, psychotropic drugs or who had type I diabetes mellitus were excluded. Informed written consent was obtained from each participant (Soh et al., 2014).
Genotyping
Genome-wide platforms (PsychArray/PsychChip, Illumina) were used to genotype 242,211 autosomal SNPs of buccal epithelial cells of children in MAVAN, according to manufacturer's guidelines. SNPs with a low call rate (<95%), low p-values on Hardy-Weinberg Equilibrium (HWE) exact test (p <1e-40), and minor allele frequency (MAF <5%) were removed. Afterwards, imputation using the Sanger Imputation Service was performed and SNPs with an info score >0.80 were retained for the analysis, resulting in 20,790,893 SNPs (McCarthy et al., 2017).
In GUSTO, genotying was performed using Infinium OmniExpressExome array and split by ethnicity for quality checks. Non-autosomal SNPs, SNPs with call rates <95%, minor allele frequencies <5%, and failed Hardy-Weinberg equilibrium p-value of 10–6 were removed. Variants discordant with their respective subpopulation in the 1,000 G reference panel were removed (Chinese: EAS with a threshold of 0.20; Malays: EAS with a threshold of 0.30; Indian: SAS with a threshold of 0.20). Samples with call rate <99%, cryptic relatedness and sex/ ethnic discrepancies were excluded. The resulting data were pre-phased using SHAPEIT v2.837 with family trio information. We then used Sanger Imputation Service for imputation, choosing 1,000 G Phase 3 as reference panel and imputed “with PBWT, no pre-phasing” as the pipeline. Imputed data that were non-monomorphic, had biallelic SNPs and an INFO score >0.80 were retained. Imputed genotyping data that were common in all three ethnicities (5,771,259 SNPs) were used for further analyses.
The population structure of the MAVAN and GUSTO cohorts were evaluated using principal component analysis of all autosomal SNPs that passed the quality control without low allele frequency (MAF>5%) and not in high linkage disequilibrium (r2 >0.2) across 500 kb regions (Price et al., 2006; Wray et al., 2014). Based on the inspection of the scree plot, the first three principal components were the most informative of population structure in both cohorts and were included in all subsequent analyses.
DNA Methylation Analyses
DNA methylation analyses were done in buccal epithelial cells (Catch-All Swabs, Epicenter, USA) in MAVAN children at a mean age of 6.99 years. Details of DNA extraction, preprocessing and quality control procedures were described in Garg et al. (2018). Briefly, genomic DNA (750 ng) was bisulfite converted using the EZ-DNA Methylation Kit (Zymo Research, USA) and hybridized on Infinium HumanMethylation450 beadchip array (450 K, Illumina). Data was processed in R using the Minfi package (Aryee et al., 2014). Samples that failed standard Minfi quality control procedure were removed. All remaining samples had a high call rate (>95%). Probes with a low call rate (<75%), a high detection p-value (p > 0.05) and a low number of beads (<3 in >5% of the cohort) were also removed. We also used ComBat (Johnson et al., 2007) to iteratively adjust our data for unwanted technical variation associated with experimental batch, array row (sentrix row), and plate position (sample column). We also predicted buccal epithelial cell content of each sample (Smith et al., 2015) and included the proportion of buccal cells per sample as a covariate in all models describing variation in DNA methylation levels. A full explanation of the DNA methylation analyses is provided in Garg et al. (2018).
5-HTT Co-expressed Genes and ePRS
The expression-based polygenic risk score was created considering genes co-expressed with the serotonin transporter gene (5-HTT-ePRS) in amygdala, according to the protocol previously described by Silveira et al. (2017) and Miguel et al. (2019). The genetic score from the 5-HTT gene network was created using: GeneNetwork (http://genenetwork.org), BrainSpan (http://www.brainspan.org), NCBI Variation Viewer (https://www.ncbi.nlm.nih.gov/variation/view), and The Genotype-Tissue Expression (GTEx) (https://gtexportal.org/home/) (Lonsdale et al., 2013). GeneNetwork was used to generate a list of genes coexpressed with 5-HTT in the amygdala in mice (Mulligan et al., 2016). Only genes with absolute value of co-expression correlation higher or equal to 0.5 were retained. The gene list generated by GeneNetwork was then filtered using BrainSpan to identify consensus transcripts enriched in the fetal and childhood human brain (Miller et al., 2014). Since we were interested in genes that were active during early developmental periods, we selected autosomal transcripts expressed in the amygdala at least 1.5-fold more during fetal and child development (all fetal samples and up to the first 5 years of age) as compared to adult samples. Based on their functional annotation in the National Center for Biotechnology Information, U.S. National Library of Medicine (NCBI Variation Viewer), using GRCh37.p13, we gathered all the SNPs from these genes and merged this list with the SNPs from the GTEx data in human amygdala to form a list of common SNPs. The list of common SNPs was then subjected to linkage disequilibrium clumping (r2 < 0.25), which resulted in 463 SNPs. In ePRS calculation, alleles at a given cis-SNP were weighed by the estimated effect of the genotype on gene expression (expression quantitative trait loci from GTeX, in which the effect allele is the alternative allele). Final ePRS was obtained by summation over all SNPs accounting for the sign of correlation coefficient between the genes and 5HTT gene expression. For more information on ePRS calculation, see Hari Dass et al. (2019). The summation of these values from the total number of SNPs provides the amygdala 5-HTT-ePRS score (Table 1 shows the final gene list). Figure 1 illustrates the steps involved in the creation of the ePRS.
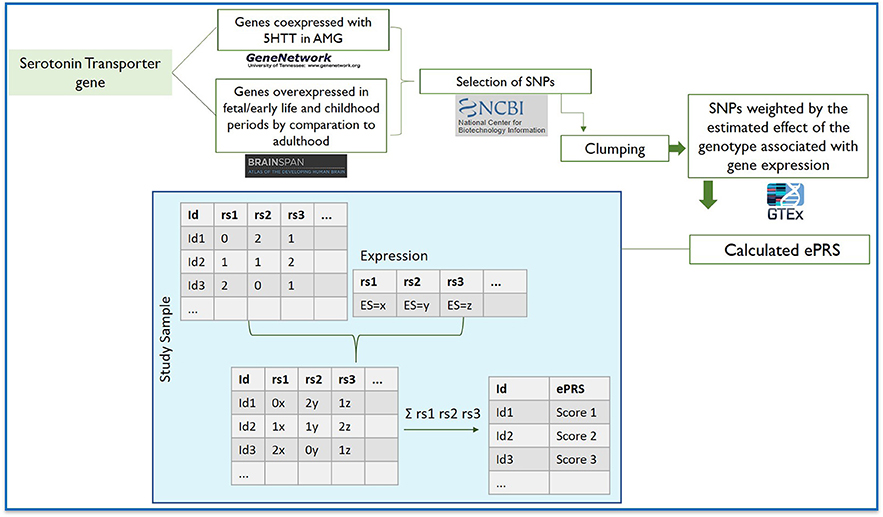
Figure 1. Flowchart depicting the steps involved in creating the expression-based polygenic risk score based on genes co-expressed with serotonin transporter gene in amygdala using gene co-expression databases: GeneNetwork was used to generate a co-expression matrix with 5-HTT gene in the amygdala in mice (absolute value of the co-expression correlation r ≥ 0.5); BrainSpan was then used to identify consensus human transcripts from this list; BrainSpan was also used for selecting genes differentially expressed at ≥1.5-fold during child and fetal development as compared to adulthood within the same brain areas. Based on their functional annotation in the National Center for Biotechnology Information, U.S. National Library of Medicine, we gathered all the existing SNPs from these genes and subjected this list of SNPs to linkage disequilibrium clumping. We used a count function of the number of alleles at a given SNP (rs1, rs2…) weighted by the estimated effect of the genotype associated with gene expression. The sum of these values from the total number of SNPs provides the amygdala 5-HTT ePRS score.
Postnatal Adversity Score
The postnatal adversity score was created combining multiple indicators of adversity. The instruments included as indicators of postnatal adversity were the following: (1) The Health and well-being questionnaire: short versions of multiple measures (Kramer et al., 2001): (a) Presence of chronic disease during pregnancy or severe acute conditions; (b) A subscale from the Daily Hassles was used to measure how often, and to what degree, the woman had lacked money for basic needs since the beginning of pregnancy (Kanner et al., 1981); (c) The Marital Strain Scale of Pearlin and Schooler was used to assess chronic stress with the romantic partner (Pearlin and Schooler, 1978); (d) The Abuse Assessment Screen was used to assess conjugal violence (Newberger et al., 1992; Parker et al., 1993); (e) Questions about anxiety during pregnancy (Lobel et al., 1992; Parker et al., 1993). (2) Smoking during pregnancy: composed of yes or no questions. (3) Household gross income (Daveluy et al., 1998). (4) Depression questionnaire (Centre of Epidemiological Studies) (CES-D): a 20 Likert-Scale item instrument applied to assess the symptoms of depression (Radolf, 1977). (5) Child Health Questionnaire: Includes questions on acute, chronic conditions, and hospitalizations (Plante et al., 2002). (6) Maternal mental health: Beck Depression Inventory, a 21-question self-report questionnaire (Beck et al., 1961); Edinburgh Postnatal Depression Scale (EPDS), a 10-item self-report scale designed to screen for postpartum depression (Cox et al., 1987); State-Trait Anxiety Inventory (STAI), a self-report instrument consisted of two forms of 20 items each to measure psychic components of state and trait anxiety (Spielberger, 2010). (7) Attachment: The Preschool Separation—Reunion Procedure (PSRP) was applied at 36 months and used to measure attachment security in preschool-aged children. The task consists of a baseline interaction, followed by two separation and reunion episodes lasting 5 min; scoring was based on video coding (reliability k = 0.83). Four categories were assessed: secure, ambivalent, avoidant and disorganized (Cassidy and Marvin, 1992). (8) Family Assessment Device: 60-item self-report instrument developed to assess the six dimensions of the family functioning outlined in the McMaster Model of Family Functioning (Moss et al., 2004). A general functioning scale assesses overall health-pathology (Kabacoff et al., 1990). In GUSTO the indicators of adversity were similar, except that there was no information on attachment styles in this cohort. For each continuous variable we applied 15th or 85th percentile cut-off to categorize subjects in exposed or not to that event. The total score represents the summation of all points. The earliest postnatal time point available for each variable was used for the calculation of the adversity score.
Behavioral Outcome: Child Behavior Checklist
The Child Behavior Checklist (CBCL) questionnaire which includes 100 items was used to evaluate emotional, behavioral, and social difficulties in preschool children. The focus of this study was in scales related with attention and hyperactivity problems, so we chose ADHD Problems, Attention Problems and Externalizing Problem scales for this work. An externalizing scale is computed by summing scores on items related to attention problems and aggressive behaviors (Achenbach, 2011).
A total of 137 children from the MAVAN cohort and 401 from the GUSTO cohort had complete data (genotype, information on early life environment, and CBCL scores) and were included in the study.
Gray Matter Density
Structural MRI of the whole brain was acquired using a 3T trio Siemens scanner available at Cerebral Imaging Center, Douglas Research Centre (Montreal, Canada) (1 mm isotropic 3D MPRAGE, sagittal acquisition, 256 × 256 mm grid, TR= 2,300 ms, TE = 4 ms, FA = 9°) and a GE MR750 Discovery 3T MRI scanner at the Imaging Research Centre, St. Joseph's Healthcare (Hamilton, Canada) (3D inversion recovery-prepped, T1-weighted anatomical data set, fSPGR, axial acquisition, TE/TR/flip angle = 3.22/10.308/9, 512 × 512 matrix with 1 mm slice thickness and 24 cm FOV. T1-weighted images were processed by computational Anatomy Toolbox (CAT12) from the Statistical Parametric Mapping software (SPM12). In the preprocessing step, the images were normalized and segmented into gray matter and white matter. After a high-dimensional Diffeomorphic Anatomical Registration Through Exponentiated Lie Algebra (DARTEL) normalization, a smoothing process was applied using 8 mm full width half maximum kernel.
Enrichment Analysis
The gene network data were retrieved from GeneMANIA (Warde-Farley et al., 2010) (https://genemania.org) database and the gene-gene interaction networks were constructed and visualized in the Cytoscape software (Saito et al., 2012). Enrichment analysis was performed using MetaCore™ (Clarivate Analytics). Data for analysis of the co-expression data during development was extracted from BrainSpan (Miller et al., 2014). Epigenes (genes and proteins involved in epigenetic regulation) within the networks were investigated using the Epifactors Database (http://epifactors.autosome.ru/) (Medvedeva et al., 2015). Localization of epigenes on cell classes in the brain were made using cell types enrichment (https://www.brainrnaseq.org/) (Zhang et al., 2014). Enrichment analysis for molecular function, biological process, protein class, cell components and pathways were performed in Panther (http://pantherdb.org/) (Mi et al., 2019).
Statistical Analysis
Data was analyzed using R (https://www.rstudio.com/) (R Foundation for Statistical Computing, 2018). Significance levels for all measures were set at α < 0.05. Linear regression analysis was applied to examine the effects of interaction between the polygenic score and the adversity score on the behavioral outcomes (CBCL), adjusting for sex and population stratification. For the analyses with a significant interaction term, simple slope analyses were conducted to describe the differences. Population stratification was composed by models adjusted by principal components (Patterson et al., 2006; Price et al., 2006). For that, population structure was analyzed to identify the presence of a systematic difference in allele frequencies between subpopulations in a population. We added the principal components to adjust for false results due to ancestry differences. We pruned our datasets to common variants (MAF>0.05) that were not in linkage disequilibrium (r2 < 0.20) with a sliding window (50 kilobases) approach that examined linkage disequilibrium in increments of 5 SNPs using PLINK 1.9 (Price et al., 2006). Principal component analysis was performed using SMARTPCA on this pruned dataset and generated a scree plot (see Hari Dass et al., 2019, for scree plot for the MAVAN cohort). Based on the inspection of the scree plot, the first three principal components were the most informative of population structure in both cohorts and were included in all analyses.
A multivariate approach called parallel independent component analysis (pICA) was applied to identify relationships between two different data modalities focusing on inter-related patterns in a data driven manner (Price et al., 2006). The goal of this method is to discover independent components from these two data modalities, in addition to the relationship between them (Liu and Calhoun, 2014). The pICA is a variant of ICA for multimodality processing that extracts maximally independent components within each data modality separately, while also maximizing the association between modalities using an entropy term based on information theory, thus enhancing the interconnection by maximizing the linkage function in a joint estimation process (Liu and Calhoun, 2014; Pearlson et al., 2015).
Using this approach we sought to find the relationship between the SNP-based ePRS-5-HTT (or genotype x GTEx gene expression slope at each SNP comprised by the ePRS-5-HTT) and the voxel-based gray matter in the whole brain, instead of investigating the relationship between the crude genotype and the gray matter-voxel-based measures. The groups for comparison (25 children with high adversity score and 24 children with low adversity score) were defined by the postnatal environment aggregated with population stratification (ethnicity) for adjustment. The number of independent components estimated using minimum description length criteria (Calhoun et al., 2001) was 17 for genetic data and 8 for MRI data. Loading coefficients, which describe the presence of the identified component across participants (Liu et al., 2012) were extracted for each component, modality, and participant. The mean participant-specific loading coefficients of these components between children from high and low-adversity-score groups was compared using Student's t-test.
Further we explored if variation in the levels of DNA methylation was predicted by postnatal adversity score, ePRS-5-HTT, or their interaction. We used the following models of linear regression analyses to test these associations:
1. CpG ~ sex + PC1 + PC2 + PC3 + A postnatal
2. CpG ~ sex + PC1 + PC2 + PC3 + ePRS
3. CpG ~ sex + PC1 + PC2 + PC3 + A_ postnatal * ePRS
CpG represents methylation levels at a single variably methylated probe; sex is the biological sex of participant; PC(1, 2, or 3) represents the population stratification principal components from the genetic data, and A_ postnatal represents the postnatal adversity score.
For each variably methylated CpG we compared the Akaike information criterion (AIC) across the three models to identify the model that best explained variation in DNA methylation at a given CpG. We utilized AIC since it permits the comparison of non-nested models.
Results
Baseline comparisons between low and high ePRS groups (median split) were done in the two cohorts. Differences in means on the main confounding variables were tested using Student's t-test for independent samples and Chi-square Test was applied for categorical variables. No differences were found in relation to the main confounding variables in MAVAN (Table 2). In GUSTO (Table 2), we observed a higher prevalence of breastfeeding for at least 3 months in the low ePRS group (66%) vs. high ePRS group (56%). To confirm our results we adjusted the interaction by breastfeeding in GUSTO. The same results were observed.
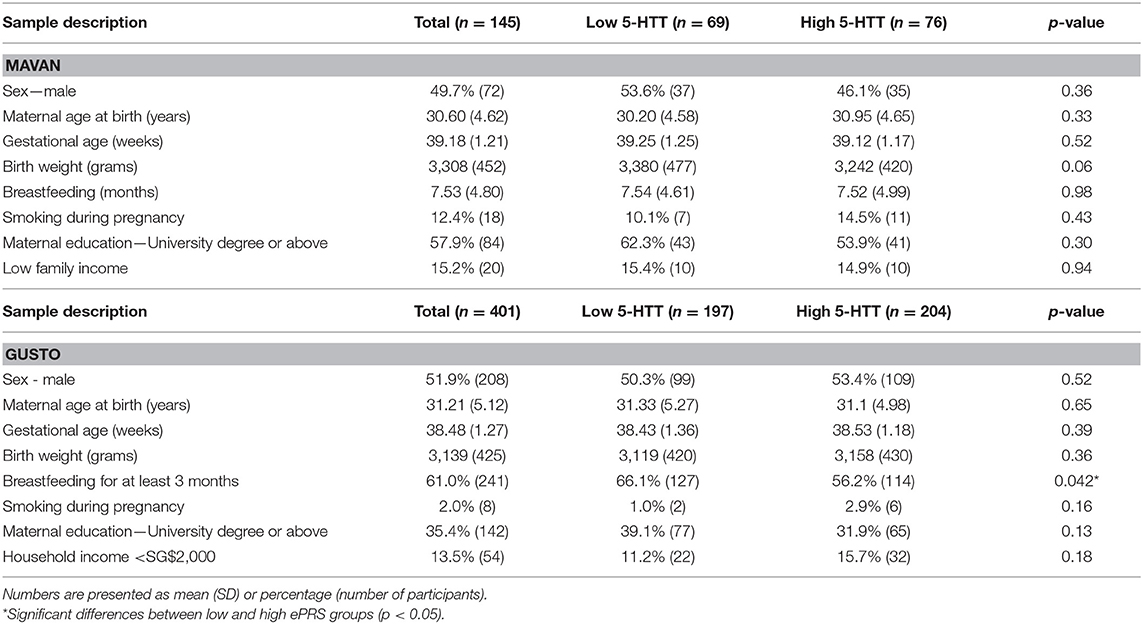
Table 2. Description of the baseline characteristics of the MAVAN and GUSTO samples according to high and low amygdala ePRS-5-HTT.
Interaction Between ePRS-5-HTT in the Amygdala and Postnatal Adversity Score Moderates the Behavior in Children
In MAVAN children, we found a significant effect of interaction between the adversity score and the amygdala-based ePRS-5-HTT on domains of the CBCL related to attentional problems and hyperactivity: ADHD Problems at 48 months (β = –71.19, p = 0.0009) and 60 months (β = –62.32, p = 0.006); Attention Problems at 48 months (β = –39.07, p = 0.010) and a trend at 60 months (β = –27.81, p = 0.07); and Externalizing Problem at 48 months (β = –131.06, p = 0.03) and 60 months (β = –151.64, p = 0.01). Simple slope analysis showed that increased postnatal adversity exposure is associated with more ADHD problems (48 months: β = 0.93, p = 0.0001; 60 months: β = 1.07, p < 0.0001), attentional problems (β = 0.56, p = 0.0004); externalizing problems (48 months: β = 1.62, p = 0.01; 60 months: β = 1.91, p = 0.002), as the ePRS-5-HTT score decreases (Figure 2). After correction for multiple testing using Bonferroni-Holm method, ADHD Problems at 48 and 60 months were still significant.
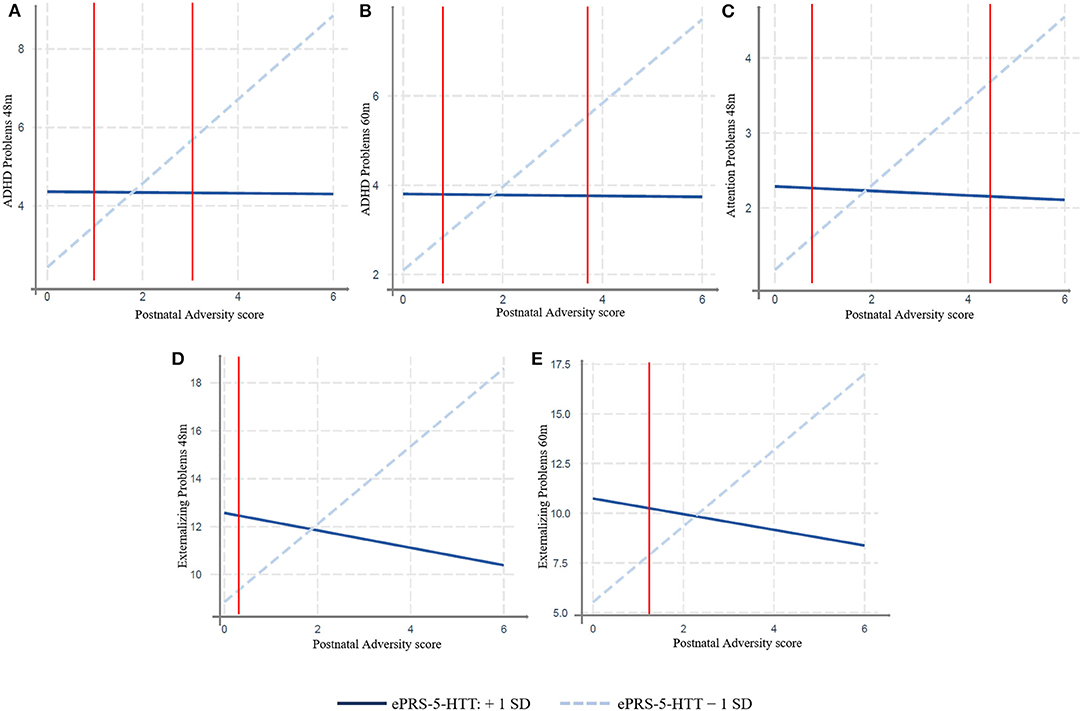
Figure 2. Interaction between Postnatal Adversity Score and amygdala-based ePRS-5-HTT on: (A) ADHD problems 48 and (B) 60 months (N = 137), (C) Attention Problems at 48 months (D) Externalizing Problem at 48 months (E) and 60 months. Increased postnatal adversity exposure is associated with more problematic behavior outcomes as the ePRS decreases. MAVAN cohort, N = 125–137.
We replicated our findings in a different population. More specifically, we analyzed whether the interaction between the adversity score and the amygdala-based ePRS-5-HTT would predict attention problems in children in the GUSTO cohort, and similar results were found. We observed significant effect of interactions between adversity score and the amygdala-based ePRS-5-HTT on CBCL scales: ADHD problems at 4 years (β = −32.79, p = 0.02) and attention problems at 4 years (β = −31.77, p = 0.0014) and externalizing problems at 4 years (β = –87.06, p = 0.031; Table 3). A simple slope analysis showed that increased postnatal adversity exposure is associated with more ADHD problems (β = 0.89, p < 0.0001), as well as with higher attentional (β = 0.70, p < 0.0001), and externalizing problems (β = 2.72, p < 0.0001) as the ePRS-5-HTT score decreases (Figure 3). After correction for multiple testing, all the associations remained significant.
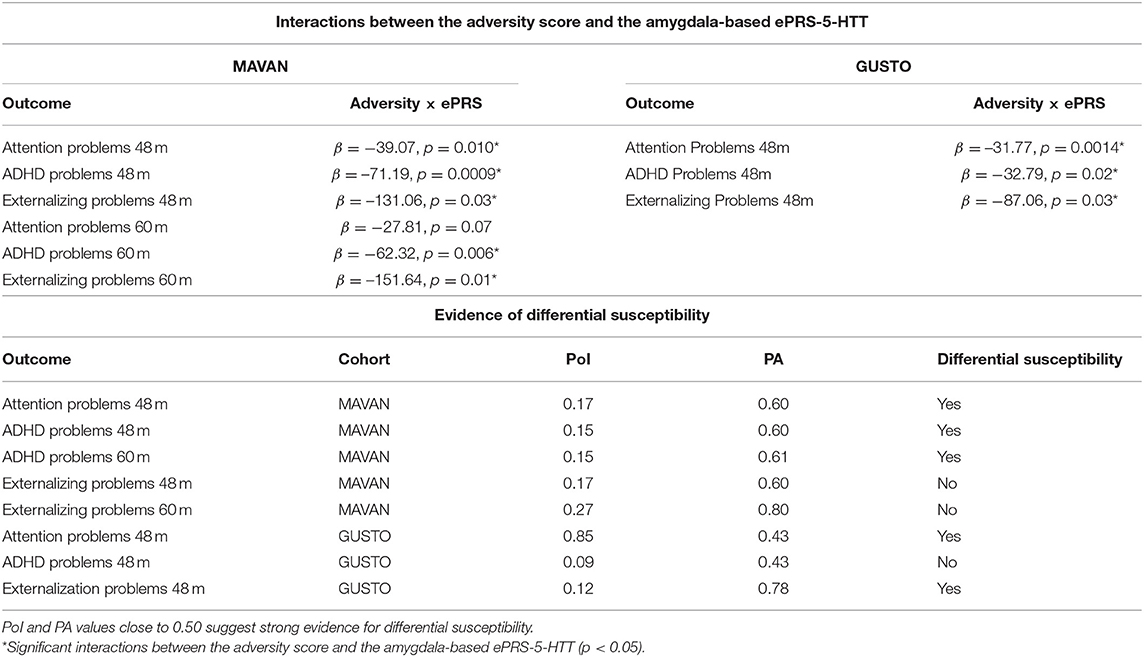
Table 3. Results of interactions between the adversity score and the amygdala-based ePRS-5-HTT on CBCL behavior of children in MAVAN and GUSTO cohorts; and evidence of differential susceptibility.
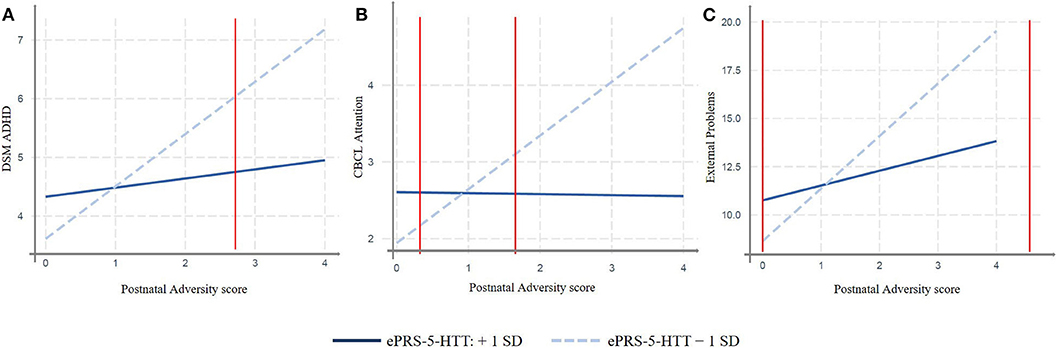
Figure 3. Interaction effect between Postnatal Adversity Score and amygdala-based ePRS-5-HTT on: (A) ADHD problems at 4 years, (B) attention problems at 4 years, and (C) Externalizing Problems at 4 years. Increased postnatal adversity exposure is associated with outcomes related to more Behavioral problems as ePRS decreases. GUSTO cohort, N = 401.
We also analyzed whether these interactions were consistent with the differential susceptibility model (Roisman et al., 2012). We verified if regions of significance were inside the possible range of value for the environmental score, calculated proportion of interaction (PoI) and proportion affected (PA). Table 3 shows these results in MAVAN and GUSTO cohorts. In every case we find some evidence (PoI and PA values close to 0.50 suggest strong evidence for differential susceptibility) for differential susceptibility. All three criteria showed that interactions were consistent with differential susceptibility. These results suggest that the same genetic background associated with the 5-HTT gene network is affected by the exposure to adversity but also more protected in supportive environments with regards to ADHD and Attention Problems outcomes. Pol and PA are consistent with differential susceptibility in MAVAN and GUSTO cohort (see Table 3).
These results suggest a presence of a strong genome x environment interaction effect on behavior outcomes in children. Exposure to postnatal adversity and decreased ePRS-5-HTT are associated with negative outcomes in CBLC at different ages in different cohorts. This suggests that the amygdala 5-HTT ePRS is a strong predictor of attention-related processes in the context of variation in the environmental quality in line with the differential susceptibility paradigm.
Postnatal Adversity, ePRS-5-HTT, and Their Interaction Contribute to DNA Methylation Variations
We identified a total of 54,295 variably methylated probes cross the genome. For each probe we compared AIC across three models to explore the prediction of the DNA methylation by postnatal adversity, ePRS or their interaction. We observed that postnatal adversity explained DNA methylation levels better than other predictors for 47% of all variably methylated sites. The ePRS-5-HTT main effect was a better predictor for 43% of the variably methylated sites and the interaction between the two factors—for 10% of CpGs (Figure 4).
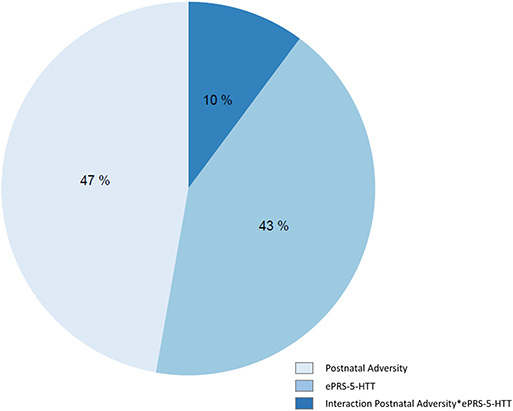
Figure 4. Variations in DNA methylation levels were predicted by postnatal adversity score, ePRS-5-HTT, and the interaction between postnatal adversity score and ePRS-5-HTT. Each variable on chart denotes one model. AIC was used to compare three models for each CpG and select the best one. Percentages on chart represent the number of CpGs that are best explained (lowest AIC) by a specific model. All models were adjusted for population stratification and sex.
Enrichment Analysis of the 5-HTT Co-expression Networks
We performed enrichment analysis on the genes selected for composing the genetic score (complete list of genes on Table 1). The relationship between genes was performed using GeneMANIA, and the interaction networks were constructed in the Cytoscape software. Figure 5 shows the gene interactions. Using Cytoscape, we calculated the degrees and betweenness of the 5-HTT network. Nodes above the threshold (mean + 1 SD) were considered central nodes (high betweenness indicated bottlenecks and high degrees indicated hubs) (Neves de Oliveira et al., 2018). The two most important hubs and bottlenecks of this list included: HNRNPA1 (Heterogeneous Nuclear Ribonucleoprotein A1), a protein coding gene, member of a family of ubiquitously expressed heterogeneous nuclear ribonucleoproteins, which are RNA-binding proteins that associate with pre-mRNAs in the nucleus and influence pre-mRNA processing, as well as other aspects of mRNA metabolism and transport; and PRKDC, a protein kinase, which encodes the catalytic subunit of the DNA-dependent protein kinase. AURKA (Aurora kinase) is the most import hub gene of the gene network co-expressed with 5-HTT in amygdala. The protein encoded by this gene is a cell cycle-regulated kinase that appears to be involved in microtubule formation and/or stabilization at the spindle pole during chromosome segregation. The most important bottleneck is RAD54L. The protein encoded by this gene is involved in homologous recombination and repair of DNA (Stelzer et al., 2016).
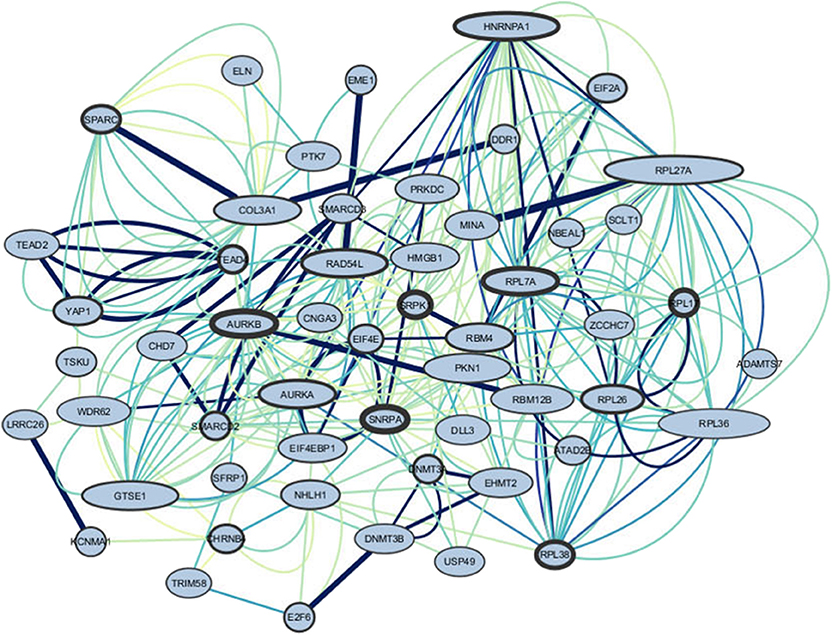
Figure 5. Genes interactions of the amygdala 5-HTT co-expression network. The border of the genes represents the out-degree of these nodes, meaning the number of outgoing relationships. The larger the border, the stronger the relationship of the gene to other genes. The size of the nodes represents the in-degree, i.e., the number of incoming relationships with neighbors. The bigger the node, the higher the relationships of other genes to the target gene. The edges represent co-expression. Color and thickness were used to identify the most co-expressed genes, darker, and thicker represent higher co-expression.
BrainSpan data was used to correlate the expression levels of the genes included in the ePRS-5-HTT in the amygdala in two periods (a) infancy and early childhood, and (b) adulthood. We observed large clusters of highly co-expressed genes in both periods (Figure 6). However, different gene clusters were observed during infancy/early childhood and during adulthood, suggesting that there is a developmental influence on the design of the clusters of co-expression of these genes.
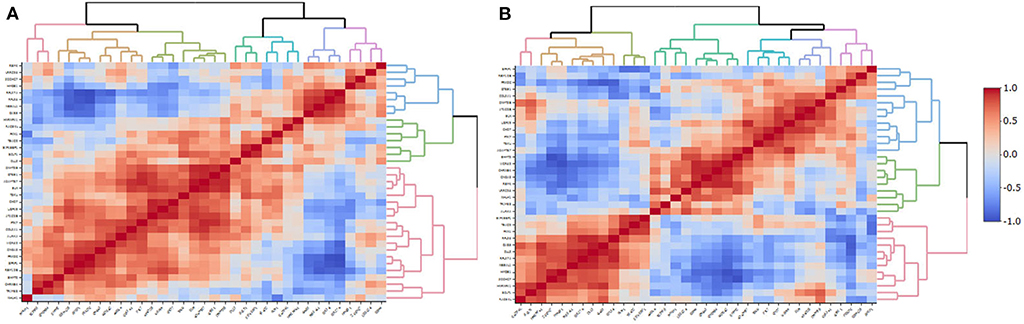
Figure 6. Co-expression of the genes included in the ePRS-5-HTT in the amygdala in the (A) infancy and early childhood, and (B) adulthood periods in humans. Each vertical line represents correlation with a unique gene. Genes from the same expression quantification tend to cluster together and could be visualized in red (positive correlation), or blue (negative correlation) patterns. Infancy and early childhood period ranges from 0 to 4 years of age (N = 7); Adulthood ranges from 20 to 40 years (N = 6). Data for this analysis was extracted from BrainSpan.
Enrichment analyses performed in Metacore® shows two important pathways related with DNA damage and epigenetic regulation of gene expression (see all results in Table 4). The enrichment analysis for gene ontology shows enrichment processes related to chromosome organization, DNA conformational changes, DNA methylation; and nervous system development (such as generation of neurons and developmental process). Interestingly, of the 35 genes on the list of genes co-expressed with 5-HTT in the amygdala, 10 are classified as epifactors and are involved in histone phosphorylation and methylation, chromatin remodeling and DNA methylation (Figure 7). We also analyzed the cell classes in the brain where these epifactors are more expressed, and most of them are localized in astrocytes, neurons, and oligodendrocyte precursor cells (Figure 7). Enrichment analyses performed in Panther shows molecular functions related with transporter activity and binding, biological process related with developmental processes, cellular processes, and metabolic processes. At same time, proteins classes were enriched for transporter, nucleic acid binding, and transferases. Finally, this gene network was enriched in pathways related to muscarinic acetylcholine and nicotine receptors signaling pathways (Figure 8).
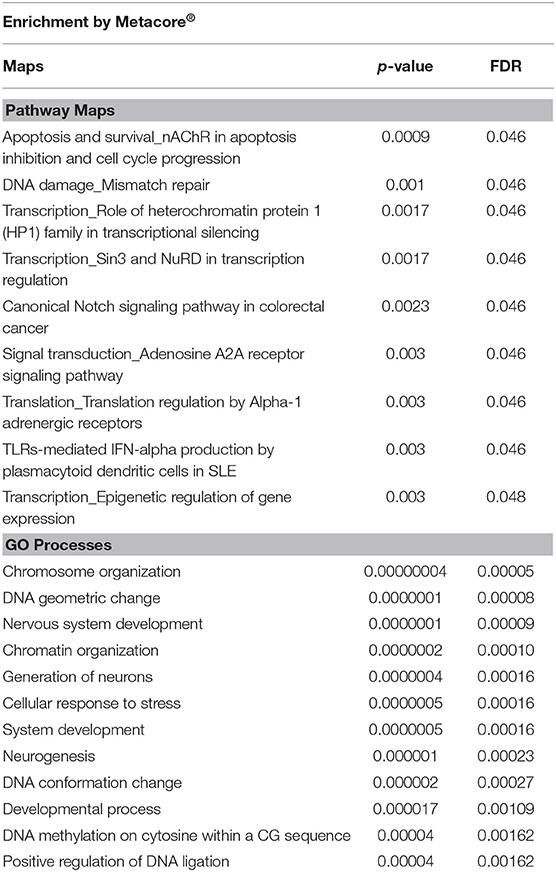
Table 4. Pathway Maps and Gene Ontology Processes related to genes included in the expression-based polygenic risk score of the 5-HTT in the amygdala.
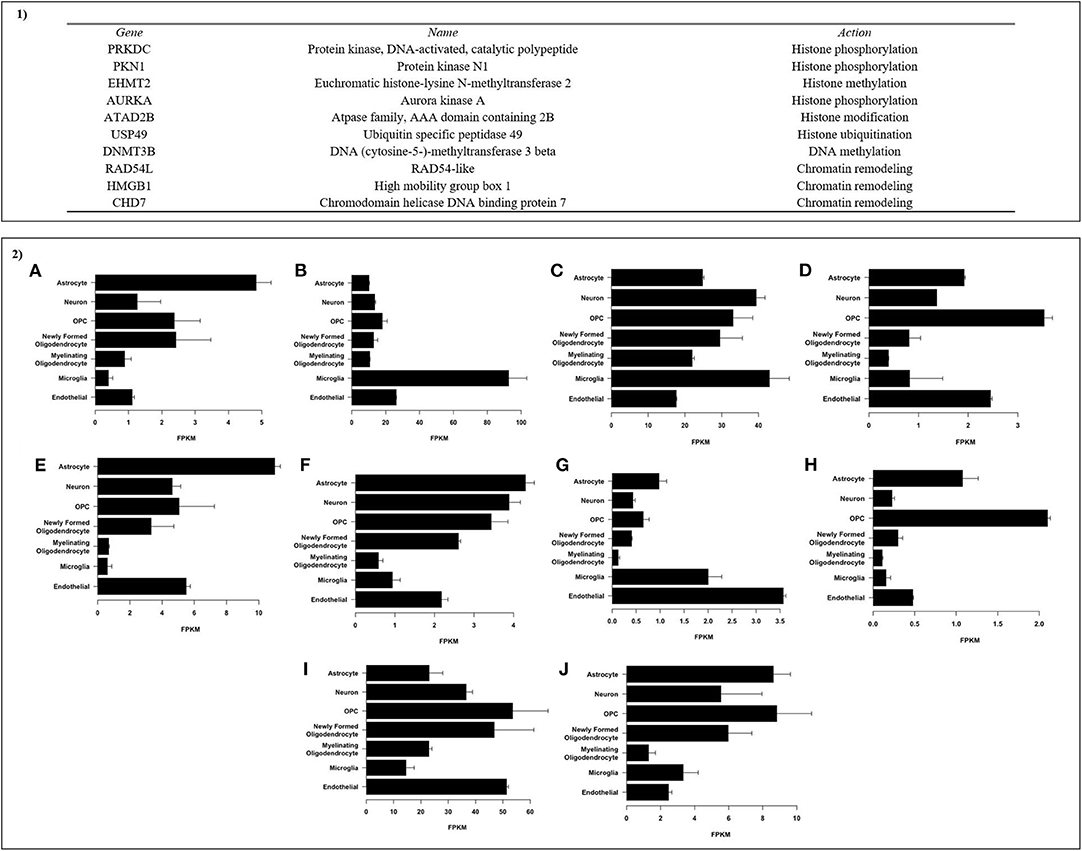
Figure 7. Epifactors included in the expression-based polygenic risk score of the 5-HTT in the amygdala: (1) Genes classified as epifactors, involved in histone phosphorylation and methylation, chromatin remodeling, and DNA methylation; (2) Cell classes in the brain where these epifactors are expressed. Each graphic represents a gene: (A) PRKDC, (B) PKN1, (C) EHMT2, (D) AURKA, (E) ATAD2B, (F) USP49, (G) DNMT3B, (H) RAD54L, (I) HMGB1, and (J) CHD7.
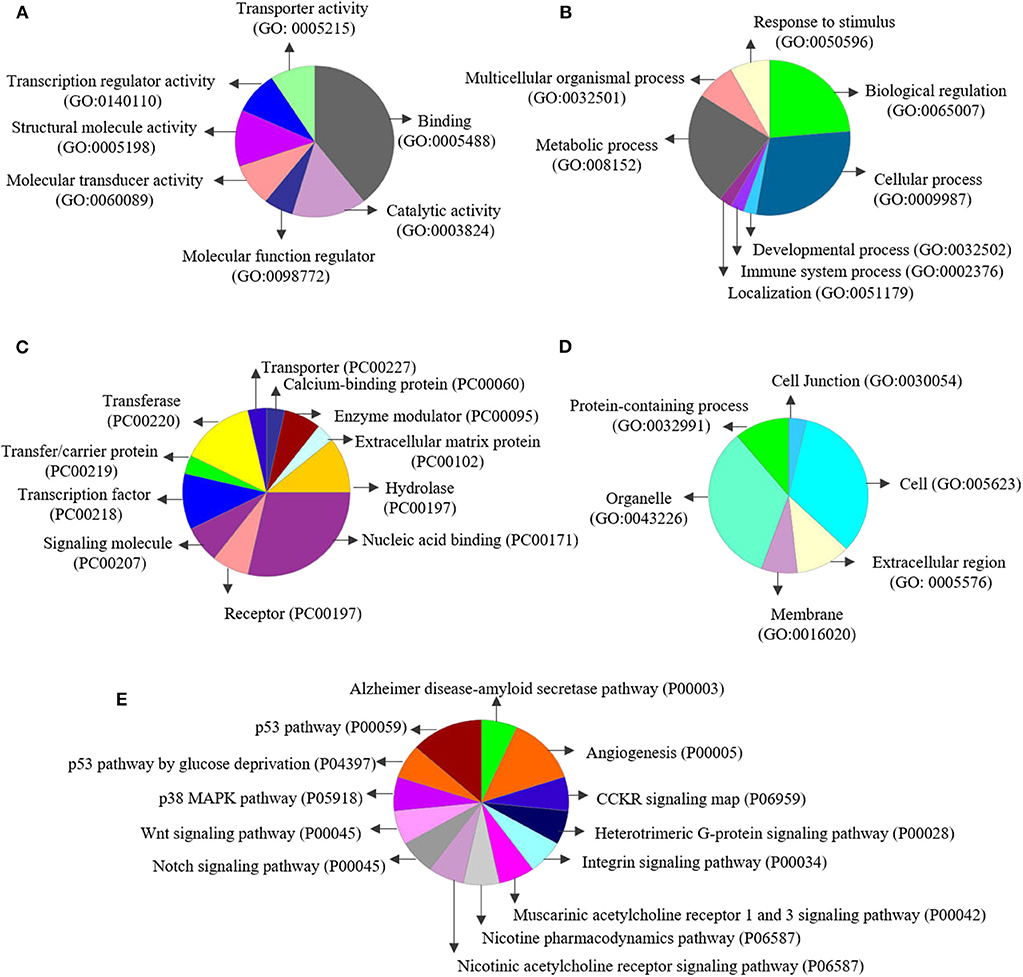
Figure 8. Enrichment analysis performed using Panther (http://pantherdb.org/): Enrichment for (A) molecular functions, (B) biological processes, (C) protein classes, (D) cell components, and (E) pathways.
SNP-Based ePRS-5-HTT and the Voxel-Based Gray Matter Density
In order to perform an anatomical-functional correlation of our findings, we analyzed the relationship between brain gray matter density and the SNPs used to create the ePRS-5-HTT in 49 children from MAVAN that had MRI and genotype data available. The Parallel ICA identified seven significant relationships between regional gray matter volume and SNP-based ePRS. The most significant relationships between regional gray matter density and SNP-based ePRS-5-HTT data was on the genetic component 12 and MRI component 5 (r =0.704, p = 1.62e-08). The comparison of mean loading coefficients of these components between children from high- and low adversity score groups by Student's t-test indicated no statistically significant differences (Figure 9), suggesting that SNPs on the ePRS-5-HTT list moderate gray matter density in specific brain regions, but this effect is not moderated by the adversity experienced on these parameters.
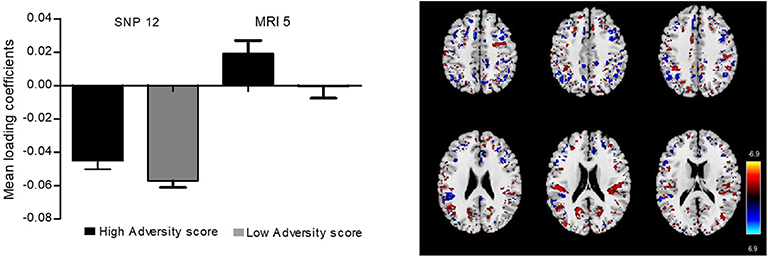
Figure 9. A bar plot of the mean loading coefficients of brain magnetic resonance imaging (MRI) component and genetic component. Student's t-test was performed to compare loading coefficients means between groups of high and low adversity score for SNPs (single nucleotide polymorphisms) and MRI.
To define the significant SNPs in each component, we used a threshold of higher than 2.5 and lower than 2.5 (Z-Threshold>±2.5). In component 12, we found 14 significant SNPs, and the enrichment analysis by Metacore® demonstrated that these SNPs are involved especially in regulation of neuronal migration [false discovery rate (FDR) = 0.0082], cerebral cortex development (FDR = 0.02), neurogenesis (FDR = 0.025), regulation of cell migration (FDR = 0.030), and cell development (FDR = 0.04). This group of SNPs were related to gray matter density located mainly in cortical areas, such as superior, middle and inferior frontal gyrus, temporal gyrus, precuneus, and inferior parietal lobule (MRI component 5). All the significant brain regions and SNPs are listed in Tables 5, 6.
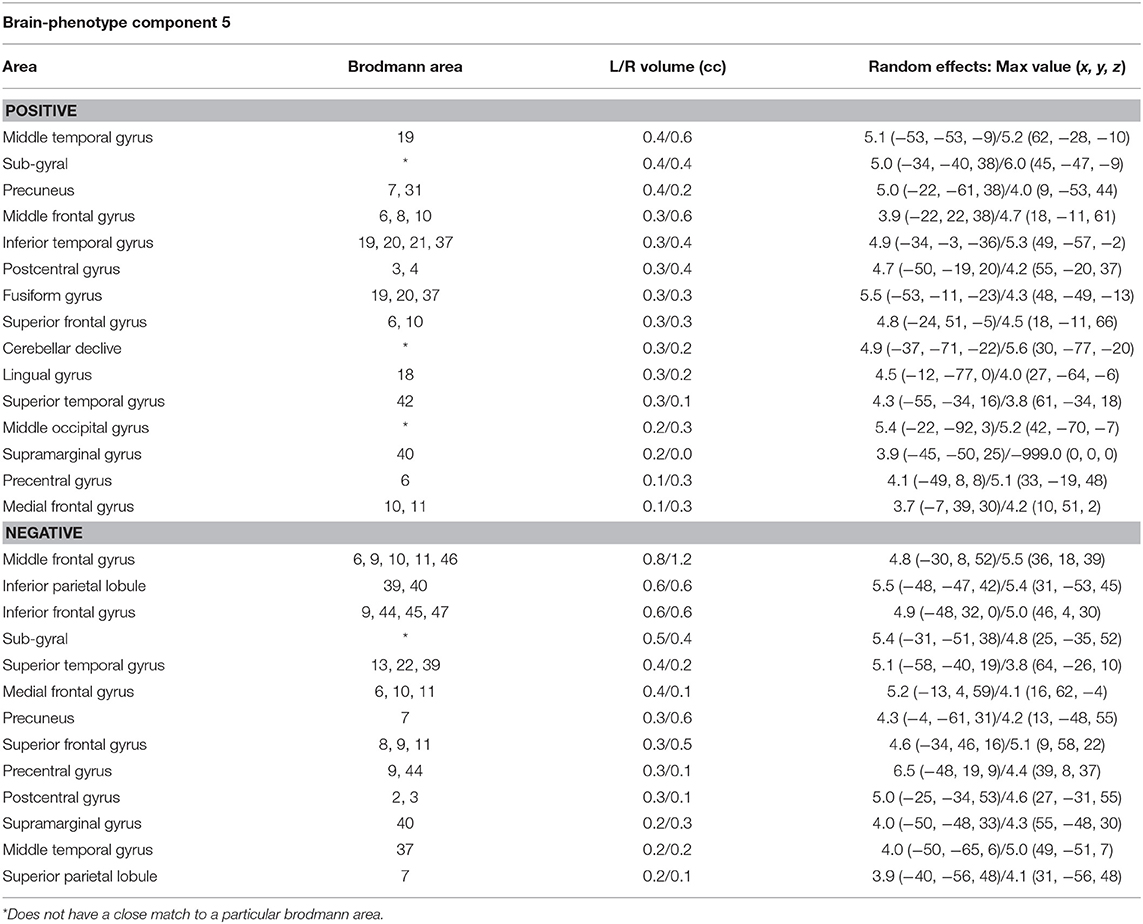
Table 5. Significant brain regions associated with gray matter density and SNP-based ePRS-5-HTT data on MRI component 5 by Parallel ICA.
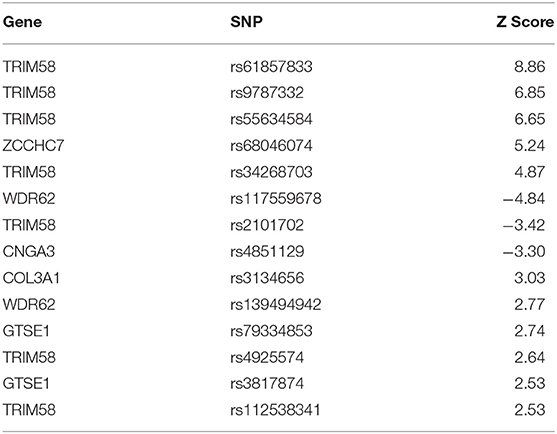
Table 6. Dominant SNPs (Z-Threshold = 2.5) associated with gray matter density on SNP component 12 by Parallel ICA.
Discussion
The main aim of this study was to analyze whether a genetic score based on genes co-expressed with the serotonin transporter in the amygdala moderates the impact of adversity exposure in early life on behaviors related to attention and hyperactivity, as well as comorbidities associated with this condition. We demonstrated that the expression-based polygenic risk score reflecting the function of an amygdala 5-HTT gene network interacts with postnatal adversity exposure, influencing attention and hyperactivity problems in different cohorts and different ages. We found a correlation between the postnatal adversity score, amygdala ePRS-5-HTT scores and their interaction on variations of DNA methylation across the genome. Additionally, we observed that SNPs used to create the ePRS-5-HTT are positively and negatively correlated with variations in gray matter density. These results together confirm that the gene network co-expressed with 5-HTT is strongly associated with behavioral, brain cortical density, and epigenetic changes in the context of adversity, that strongly correlate with their impact on attentional problems in young children.
The attention deficit hyperactivity disorder is characterized by symptoms of inattention, impulsivity, and hyperactivity. Often, ADHD is associated with emotions and behavior dysfunctions, such as affect dysregulation, irritability, and mood (Matthews et al., 2014). We demonstrated that early life adversity is strongly associated not only with attention and hyperactivity problems, but also with behaviors correlated with ADHD, such as externalizing behaviors and total problems (as measured through the CBCL). Externalizing behavioral problems reflect the child negatively acting on the external environment and consist of disruptive, hyperactive, and aggressive behaviors (Nolan et al., 1996; Liu, 2004). Our finding corroborates the associations between ADHD problems and comorbidities, primarily related to externalizing behavior problems.
The serotonergic system is associated with several behavioral processes, in domains such as cognition, emotion, reward, and attention (Carlsson, 1987; Greengard, 2001). Variations in the 5-HTT gene have been reported to be associated with impulsivity, hyperactivity, and ADHD (Halperin et al., 1997; Manor et al., 2001; Seeger et al., 2001; Heils et al., 2002). The amygdala is a complex structure rich in serotonergic terminals, and it is involved in several processes related to emotion, mechanisms of vigilance, and attention (Pessoa, 2011). While several studies have focused on the effects of serotonergic function on comorbidities, our approach presents a biologically defined gene network of genes co-expressed with the serotonin transporter in the amygdala. We demonstrated that variation across the 5HTT gene network moderated the effects of postnatal adversity on attention and hyperactivity problems. Furthermore, we observed that the interaction between genetic variation and environment on attention and hyperactivity problems provided support for the differential susceptibility hypothesis. These results suggest that individuals whose behavior is negatively affected by environmental adversity could be the same individuals to benefit most from an enriched/supportive environment (Belsky et al., 2009). Belsky et al. have suggested that children more sensitive to context might be more affected by developmental factors, being that an exposure to adversity or to more enriched environments in early life (Belsky, 1997). These results provide evidence that the 5-HTT gene network is associated with vulnerability and resilience to environmental variations, which can have implications for both preventive and therapeutic interventions.
Our findings showed that the postnatal adversity score and gene network of 5-HTT in the amygdala predict variation in DNA methylation across the genome. In fact, several studies have demonstrated that trauma in early life affects DNA methylation (Beach et al., 2010; Ouellet-Morin et al., 2013). Infant attachment is also associated with variations in genome-wide DNA methylation (Garg et al., 2018) and the early social environment has persisting influence until adult life on the DNA methylation variation across the genome (O'Donnell et al., 2018). The serotoninergic system, especially the serotonin transporter, regulates a number of epigenetic modifications (Ismaylova et al., 2018). However, we show that a broader analysis of 5-HTT gene network variation is associated with epigenetic changes. Interestingly, several genes included in the 5-HTT gene network are associated with epigenetic processes. As shown in Figure 7, 10 of the 35 genes in the amygdala 5HTT gene network are associated with histone phosphorylation and methylation, chromatin remodeling, and DNA methylation. This explains the strong association between the ePRS and variations of DNA methylation observed in our model. Although the ePRS-5-HTT in the amygdala and postnatal adversity explain most of the variations observed, it is important to emphasize that the interaction between these two factors also predicts variations in DNA methylation across the genome. These results may explain, at least in part, the mechanism by which adversity exerts its long-term effects, especially in attention hyperactivity problems.
We also analyzed the relationship between the SNPs used to create the ePRS-5-HTT in amygdala and brain gray matter density, in order to obtain anatomical-functional resolution in our findings. We observed an association between these SNPs and gray matter density in cortical areas involved in memory, attention, information processing, and decision-making, such as precuneus, superior and inferior temporal gyrus, frontal gyrus, and inferior parietal lobule, respectively (Shapiro et al., 2002; du Boisgueheneuc et al., 2006; Wallentin et al., 2006; Vickery and Jiang, 2009; Tops and Boksem, 2011). Clinical studies have been demonstrated that smaller volumes of frontal, parietal and occipital cortices were associated with the higher rates of ADHD (Botellero et al., 2017; Suffren et al., 2017). In fact, ADHD is a disorder associated with cognitive control and reward response. Theories that address ADHD as a disorder of executive control have considered atypical activity or connectivity specifically in prefrontal regions and posterior cortical regions (Miller and Cohen, 2001; Aron et al., 2004; Matthews et al., 2014). At the same time, theories that consider ADHD as a sensory and reward dysfunction have associated this disorder with brain regions involved in motivation, reward and emotional regulation, and with dysregulation of emotional control (Matthews et al., 2014). Even though we analyzed brain gray matter density, not activity or connectivity, it is interesting that the results pointed to an association between SNPs of genes co-expressed with 5-HTT in amygdala and gray matter density in cortical regions associated with executive control, suggesting that both theories may be important for typical behaviors observed in this disorder, and that this gene network could have an important role in this moderations.
These results together point to an association between genes and the environment: the gene network co-expressed with 5-HTT in the amygdala was consistently associated with behavioral outcomes, variations of gray matter density and DNA methylation. In fact, the biological processes enriched for this gene network show a high association with nervous system development and epigenetic pathways. Previous studies have shown that ADHD patients with higher SLC6A4 promoter methylation status had significantly worse hyperactive-impulsive symptoms, suggesting the potential role of epigenetics pathways in attention problems (Park et al., 2015). Furthermore, epigenes of this gene network were enriched in different cells, such as astrocytes, neurons and precursors of oligodendrocytes, which demonstrates the wide distribution of these factors. Interestingly, this gene network was also enriched for pathways of the cholinergic system, which have been associated with a number of cognitive functions, including memory, attention, and emotional processing (Sarter et al., 2001, 2006; Bentley et al., 2003). The presence of these pathways demonstrates that complex dysfunctions are probably associated not only with a gene, but possibly with a network of genes, and several systems, cells and brain regions (Gaiteri et al., 2014). Exposure to stress has been shown to affect methylation of Lys4 in histone 3 (Han et al., 2012). Methylation of this amino acid residue has been reported to affect the activity of DNMT3B (Liang et al., 2002; Chen et al., 2003) one of the enzymes coded by a gene in the ePRS-5-HTT network. This is a possible mechanism through which early adversity could epigenetically influence the function of this network and, thus, interact with our genetic score.
In conclusion, our present findings provide support for the impact of exposure to postnatal adversity on attention deficit hyperactivity disorder and externalizing problems, showing that the 5-HTT gene network is an important moderator of these effects. Our data also supports the hypothesis that this gene network has important impact in brain regions related with attention and cognitive processes. Additionally, the 5-HTT gene network and postnatal adversity are associated with variation in DNA methylation, which may explain the mechanism of long-term effects of postnatal adversity. Our study extends the knowledge of how exposure to postnatal adversity affects behavior and highlights the importance of analyzing not just a candidate gene in psychiatric disorders, but an entire gene network.
Data Availability Statement
The raw data supporting the conclusions of this article will be made available by the authors, without undue reservation, to any qualified researcher upon reasonable request.
Ethics Statement
The studies involving human participants were reviewed and approved by MAVAN: (McGill University and Université de Montréal, the Royal Victoria Hospital, Jewish General Hospital, Centre Hospitalier de l'Université de Montréal and Hôpital Maisonneuve-Rosemount) and St. Joseph's Hospital and McMaster University, Hamilton, Ontario, Canada. GUSTO: National Healthcare Group Domain Specific Review Board and the Sing Health Centralized Institutional Review Board. Written informed consent to participate in this study was provided by the participants' legal guardian/next of kin.
Author Contributions
RL, PS, and CD designed the experiments. RS, GH, MK, KO'D, PS, and MM performed the data collection. CD and AB performed the enrichment analysis. SP performed the ePRS. ZW, AC, IP, and EM performed statistical analysis. BB, DA, CD, and PS analyze the results and wrote the manuscript with RL.
Funding
This research was supported by National Research Council of Brazil (CNPq), Coordination of Improvement of Higher Level Personnel (CAPES), and the JPB Foundation through a grant to The JPB Research Network on Toxic Stress: A Project of the Center on the Developing Child at Harvard University.
Conflict of Interest
The authors declare that the research was conducted in the absence of any commercial or financial relationships that could be construed as a potential conflict of interest.
References
Achenbach, T. M. (2011). “Child behavior checklist,” in Encyclopedia of Clinical Neuropsychology, eds J. S. Kreutzer, J. DeLuca, and B. Caplan (New York, NY: Springer), 546–552.
American Psychiatric Association (2013). Diagnostic and Statistical Manual of Mental Disorders. Arlington, TX: APA. doi: 10.1176/appi.books.9780890425596
Aron, A. R., Robbins, T. W., and Poldrack, R. A. (2004). Inhibition and the right inferior frontal cortex. Trends Cogn. Sci. 18, 177–185. doi: 10.1016/j.tics.2013.12.003
Aryee, M. J., Jaffe, A. E., Corrada-Bravo, H., Ladd-Acosta, C., Feinberg, A. P., Hansen, K. D., et al. (2014). Minfi: a flexible and comprehensive bioconductor package for the analysis of infinium DNA methylation microarrays.” Bioinformatics 30, 363–369. doi: 10.1093/bioinformatics/btu049
Beach, S. R. H., Brody, G. H., Todorov, A. A., Gunter, T. D., and Philibert, R. A. (2010). Methylation at SLC6A4 is linked to family history of child abuse: an examination of the iowa adoptee sample Am. J. Med. Genet. B Neuropsychiatr. Genet. 153, 710–713. doi: 10.1002/ajmg.b.31028
Beck, A. T., Ward, C. H., Mendelson, M., Mock, J., and Erbaugh, J. (1961). Inventory for Measuring Depression. Arch Gen Psychiatry. 4, 53–63.
Belsky, J. (1997). Variation in susceptibility to environmental influence: an evolutionary argument. Psychol. Inq. 8, 182–186. doi: 10.1207/s15327965pli0803_3
Belsky, J., Jonassaint, C., Pluess, M., Stanton, M., Brummett, B., and Williams, R. (2009). Vulnerability genes or plasticity genes? Mol. Psychiatry 14, 746–754. doi: 10.1038/mp.2009.44
Bentley, P., Vuilleumier, P., Thiel, C. M., Driver, J., and Dolan, R. J. (2003). Cholinergic enhancement modulates neural correlates of selective attention and emotional processing. NeuroImage 20, 58–70. doi: 10.1016/s1053-8119(03)00302-1
Björkenstam, E., Björkenstam, C., Jablonska, B., and Kosidou, K. (2017). Cumulative exposure to childhood adversity, and treated attention deficit/hyperactivity disorder: a cohort study of 543 650 adolescents and young adults in sweden. Psychol. Med. 48, 498–507. doi: 10.1017/S0033291717001933
Bock, J., Breuer, S., Poeggel, G., and Braun, K. (2017). Early life stress induces attention-deficit hyperactivity disorder (ADHD)-like behavioral and brain metabolic dysfunctions: functional imaging of methylphenidate treatment in a novel rodent model. Brain Struct. Funct. 222, 765–780. doi: 10.1007/s00429-016-1244-7
Boecker, R., Holz, N. E., Buchmann, A. F., Blomeyer, D., Plichta, M. M., Wolf, I., et al. (2014). Impact of early life adversity on reward processing in young adults: EEG-FMRI results from a prospective study over 25 years. PLoS ONE 9:e112155. doi: 10.1371/journal.pone.0104185
Botellero, V. L., Skranes, J., Bjuland, K. J., Håberg, A. K., Lydersen, S., Brubakk, A. M., et al. (2017). A longitudinal study of associations between psychiatric symptoms and disorders and cerebral gray matter volumes in adolescents born very preterm. BMC Pediat. 17:45. doi: 10.1186/s12887-017-0793-0
Calhoun, V. D., Adali, T., Pearlson, G. D., and Pekar, J. J. (2001). A method for making group inferences from functional MRI data using independent component analysis. Hum. Brain Mapp. 140, 140–151. doi: 10.1002/hbm.1048
Carlsson, A. (1987). Perspectives on the discovery of central monoaminergic neurotransmission. Ann. Rev. Neurosci. 10, 19–40. doi: 10.1146/annurev.ne.10.030187.000315
Cassidy, J., and Marvin, R. S. (1992). Attachment Organization in 2 1/2 to 4 1/2 Years Olds: Coding Manual. Available online at: http://is.muni.cz/el/1423/jaro2014/PSY103/um/46958257/Handbook_of_Attachment_ch.18.pdf (accessed February 03, 2020).
Chen, T., Ueda, Y., Dodge, J. E., Wang, Z., and Li, E. (2003). Establishment and maintenance of genomic methylation patterns in mouse embryonic stem cells by dnmt3a and dnmt3b. Mol. Cell. Bio. 23, 5594–5605. doi: 10.1128/mcb.23.16.5594-5605.2003
Cox, J. L., Holden, J. M., and Sagovsky, R. (1987). Detection of postnatal depression. development of the 10-item edinburgh postnatal depression scale. Br. J. Psychiatry 150, 782–786. doi: 10.1192/bjp.150.6.782
Daveluy, C., Pica, L., Audet, N., Courtemanche, R., and Lapoint, F. (1998). Enquéte Sociale Et De Santé 1998, 2éme Édition. Québec, QC: Institut de la statistique du Québec.
du Boisgueheneuc, F., Levy, R., Volle, E., Seassau, M., Duffau, H., Kinkingnehun, S., et al. (2006). Functions of the left superior frontal gyrus in humans: a lesion study. Brain 129, 3315–3328. doi: 10.1093/brain/awl244
Faraone, S. V., Sergeant, J., Gillberg, C., and Biederman, J. (2003). The worldwide prevalence of ADHD: is it an American condition? World Psychiatry 2, 104–113.
Frankle, W. G., Huang, Y., Hwang, D. R., Talbot, P. S., Slifstein, M., Van Heertum, R., et al. (2004). Comparative evaluation of serotonin transporter radioligands 11C-DASB and 11C-McN 5652 in healthy humans. J. Nucl. Med. 45, 682–695.
Gaiteri, C., Ding, Y., French, B., Tseng, G. C., and Sibille, E. (2014). Beyond modules & hubs: the potential of gene coexpression networks for investigating molecular mechanisms of complex brain disorders. Genes Brain Behav. 13, 13–24. doi: 10.1111/gbb.12106
Garg, E., Chen, L., Nguyen, T. T. T., Pokhvisneva, I., Chen, L. M., Unternaehrer, E., et al. (2018). The early care environment and dna methylome variation in childhood. Dev. Psychopathol. 30, 891–903. doi: 10.1017/S0954579418000627
Greengard, P. (2001). The neurobiology of slow synaptic transmission. Science 294, 1024–1030. doi: 10.1126/science.294.5544.1024
Halperin, J. M., Newcorn, J. H., Schwartz, S. T., Sharma, V., Siever, L. J., Koda, V. H., et al. (1997). Age-related changes in the association between serotonergic function and aggression in boys with ADHD. Biol. Psychiatry 41, 682–689. doi: 10.1016/S0006-3223(96)00168-0
Han, Y., Han, D., Yan, Z., Boyd-Kirkup, J. D., Green, C. D., Khaitovich, P., et al. (2012). Stress-associated H3K4 methylation accumulates during postnatal development and aging of rhesus macaque brain. Aging Cell 11, 1055–1064. doi: 10.1111/acel.12007
Hari Dass, S. A., McCracken, K., Pokhvisneva, I., Chen, L. M., Garg, E., Nguyen, T. T. T., et al. (2019). A biologically-informed polygenic score identifies endophenotypes and clinical conditions associated with the insulin receptor function on specific brain regions. EBioMed. 42, 188–202. doi: 10.1016/j.ebiom.2019.03.051
Heils, A., Teufel, A., Petri, S., Stöber, G., Riederer, P., Bengel, D., et al. (2002). Allelic variation of human serotonin transporter gene expression. J. Neurochem. 66, 2621–2624. doi: 10.1046/j.1471-4159.1996.66062621.x
Hing, B., Braun, P., Cordner, Z. A., Ewald, E. R., Moody, L., McKane, M., et al. (2018). Chronic social stress induces DNA methylation changes at an evolutionary conserved intergenic region in chromosome X. Epigenetics 13, 627–641. doi: 10.1080/15592294.2018.1486654
Hoffman, B. J., Hansson, S. R., Mezey, E., and Palkovits, M. (1998). Localization and dynamic regulation of biogenic amine transporters in the mammalian central nervous system. Front. Neuroendocrinol. 19, 187–231. doi: 10.1006/frne.1998.0168
Ismaylova, E., Lévesque, M. L., Pomares, F. M., Szyf, M., Nemoda, Z., Fahim, C., et al. (2018). Serotonin transporter promoter methylation in peripheral cells and neural responses to negative stimuli: a study of adolescent monozygotic twins. Transl. Psychiatry 8:147. doi: 10.1038/s41398-018-0195-6
Johnson, W. E., Li, C., and Rabinovic, A. (2007). Adjusting batch effects in microarray expression data using empirical bayes methods. Biostatistics 8, 118–127. doi: 10.1093/biostatistics/kxj037
Kabacoff, R. I., Miller, I. W., Bishop, D. S., Epstein, N. B, and Keitner, G. I. (1990). A psychometric study of the mcmaster family assessment device in psychiatric, medical, and nonclinical samples. J. Fam. Psychol. 3, 431–439. doi: 10.1037/h0080547
Kanner, A. D., Coyne, J. C., Schaefer, C., and Lazarus, R. S. (1981). Comparison of two modes of stress measurement: daily hassles and uplifts versus major life events. J. Behav. Med. 4, 1–39. doi: 10.1007/bf00844845
Khadka, S., Pearlson, G. D., Calhoun, V. D., Liu, J., Gelernter, J., Bessette, K. L., et al. (2016). Multivariate imaging genetics study of MRI gray matter volume and SNPs reveals biological pathways correlated with brain structural differences in attention deficit hyperactivity disorder. Front. Psychiatry 7:128. doi: 10.3389/fpsyt.2016.00128
Kramer, M. S., Goulet, L., Lydon, J., Séguin, L., McNamara, H., Dassa, C., et al. (2001). Socio-economic disparities in preterm birth: causal pathways and mechanisms. Paediatr. Perinat. Epidemiol. 15, 104–123. doi: 10.1046/j.1365-3016.2001.00012.x
Liang, G., Chan, M. F., Tomigahara, Y., Tsai, Y. C., Gonzales, F. A., Li, E., et al. (2002). Cooperativity between DNA methyltransferases in the maintenance methylation of repetitive elements. Mol. Cell. Biol. 22, 480–491. doi: 10.1128/mcb.22.2.480-491.2002
Liu, J. (2004). Childhood externalizing behavior: theory and implications jianghong. J. Child and Adolesc. Psychiatr. Nurs. 17, 93–103. doi: 10.1111/j.1744-6171.2004.tb00003.x
Liu, J., and Calhoun, V. D. (2014). A review of multivariate analyses in imaging genetics. Front. Neuroinform. 8:29. doi: 10.3389/fninf.2014.00029
Liu, J., Ghassemi, M. M., Michael, A. M., Boutte, D., Wells, W., Perrone-Bizzozero, N., et al. (2012). An ICA with reference approach in identification of genetic variation and associated brain networks. Front. Hum. Neurosci. 6:21. doi: 10.3389/fnhum.2012.00021
Lobel, M., Dunkel-Schetter, C., and Scrimshaw, S. C. (1992). Prenatal maternal stress and prematurity: a prospective study of socieconomically disadvantaged women. Health Psychol. 11, 32–40. doi: 10.1037//0278-6133.11.1.32
Lonsdale, J., Thomas, J., Salvatore, M., Phillips, R., Lo, E., Shad, S., et al. (2013). The genotype-tissue expression (GTEx) project. Nat. Genet. 45, 580–585. doi: 10.1038/ng.2653
Manor, I., Eisenberg, J., Tyano, S., Sever, Y., Cohen, H., Ebstein, R. P., et al. (2001). Family-based association study of the serotonin transporter promoter region polymorphism (5-HTTLPR) in attention deficit hyperactivity disorder. Am. J. Med. Genet. 105, 91–95.
Matthews, M., Nigg, J. T., and Fair, D. A. (2014). Attention deficit hyperactivity disorder marguerite. Curr. Top. Behav. Neurosci. 16, 235–266. doi: 10.1007/978-3-662-45758-0_249
McCarthy, S., Das, S., Kretzschmar, W., Delaneau, O., Wood, A. R., Teumer, A., et al. (2017). A Reference panel of 64,976 haplotypes for genotype imputation. Nat Genet. 48, 1279–1283. doi: 10.1038/ng.3643
Medvedeva, Y. A., Lennartsson, A., Ehsani, R., Kulakovskiy, I. V., Vorontsov, I. E., Panahandeh, P., et al. (2015). EpiFactors: a comprehensive database of human epigenetic factors and complexes. Database 2015, 1–10. doi: 10.1093/database/bav067
Mi, H., Muruganujan, A., Ebert, D., Huang, X., and Thomas, P. D. (2019). PANTHER Version 14: more genomes, a new PANTHER GO-slim and improvements in enrichment analysis tools. Nucleic Acids Res. 47, D419–D426. doi: 10.1093/nar/gky1038
Miguel, P. M., Pereira, L. O., Barth, B., de Mendonça Filho, E. J., Pokhvisneva, I., Nguyen, T. T. T., et al. (2019). Prefrontal cortex dopamine transporter gene network moderates the effect of perinatal hypoxic-ischemic conditions on cognitive flexibility and brain gray matter density in children. Biol. Psychiatry 86, 621–630. doi: 10.1016/j.biopsych.2019.03.983
Miller, E. K., and Cohen, J. D. (2001). An integrate theory of PFC function. Ann. Rev. Neurosc. 24, 167–202. doi: 10.1146/annurev.neuro.24.1.167
Miller, J. A., Ding, S. L., Sunkin, S. M., Smith, K. A., Ng, L., Szafer, A., et al. (2014). Transcriptional landscape of the prenatal human brain. Nature 508, 199–206. doi: 10.1038/nature13185
Moss, E., Bureau, J. F., Cyr, C., Mongeau, C., and St-Laurent, D. (2004). Correlates of attachment at age 3: construct validity of the preschool attachment classification system. Dev. Psychol. 40, 323–334. doi: 10.1037/0012-1649.40.3.323
Mulligan, M. K., Mozhui, K., Prins, P., and Williams, R. W. (2016). Genenetwork: a toolbox for systems genetics. Methods Mol. Biol. 1488, 75–120. doi: 10.1007/978-1-4939-6427-7_4
Neves de Oliveira, B. H., Dalmaz, C., and Zeidán-Chuliá, F. (2018). Network-based identification of altered stem cell pluripotency and calcium signaling pathways in metastatic melanoma. Med. Sci. 6:23. doi: 10.3390/medsci6010023
Newberger, E. H., Barkan, S. E., Lieberman, E. S., McCormick, M. C., Yllo, K., Gary, L. T., et al. (1992). Abuse of pregnant women and adverse birth outcome: current knowlege and implication for practice. Obstet. Gynecol. Surv. 267, 2370–2372.
Nolan, T. M., Bond, L., Adler, R., Littlefield, L., Birleson, P., Marriage, K., et al. (1996). Child behaviour checklist classification of behaviour disorder. J. Paediatr. Child Health 32, 405–411. doi: 10.1111/j.1440-1754.1996.tb00939.x
Oades, R. D. (2010). The role of serotonin in attention-deficit hyperactivity disorder (ADHD). Handb. Behav. Neurosci. 21, 565–584. doi: 10.1016/S1569-7339(10)70101-6
O'Donnell, K. A., Gaudreau, H., Colalillo, S., Steiner, M., Atkinson, L., Moss, E., et al. (2014). The maternal adversity, vulnerability and neurodevelopment project: theory and methodology. Can. J. Psychiatry 59, 497–508. doi: 10.1177/070674371405900906
O'Donnell, K. J., Chen, L., MacIsaac, J. L., McEwen, L. M., Nguyen, T., Beckmann, K., et al. (2018). DNA methylome variation in a perinatal nurse-visitation program that reduces child maltreatment: a 27-year follow-up. Transl. Psychiatry 8:15. doi: 10.1038/s41398-017-0063-9
Oquendo, M. A., Hastings, R. S., Huang, Y. Y., Simpson, N., Ogden, R. T., Hu, X. Z., et al. (2007). Brain serotonin transporter binding in depressed patients with bipolar disorder using positron emission tomography. Arch. Gen. Psychiatry 64, 201–208. doi: 10.1001/archpsyc.64.2.201
Ouellet-Morin, I., Wong, C. C., Danese, A., Pariante, C. M., Papadopoulos, A. S., Mill, J., et al. (2013). Increased serotonin transporter gene (SERT) DNA methylation is associated with bullying victimization and blunted cortisol response to stress in childhood: a longitudinal study of discordant monozygotic twins. Psychol. Med. 43, 1813–1823. doi: 10.1017/S0033291712002784
Park, S., Lee, J. M., Kim, J. W., Cho, D. Y., Yun, H. J., Han, D. H., et al. (2015). Associations between serotonin transporter gene (SLC6A4) methylation and clinical characteristics and cortical thickness in children with ADHD. Psychol. Med. 45, 3009–3017. doi: 10.1017/S003329171500094X
Parker, B., McFarlane, J., Soeken, K., Torres, S., and Campbell, D. (1993). Physiocal and emotioan abus in pregnancy: a comparison of adult ans teenage women. Nurs. Res. 42, 173–178. doi: 10.1097/00006199-199305000-00009
Patterson, N., Price, A. L., and Reich, D. (2006). Population structure and eigenanalysis. PLoS Genet. 2:e190. doi: 10.1371/journal.pgen.0020190
Pearlin, L. I., and Schooler, C. (1978). The structure of coping. J. Health Soc. Behav. 19, 2–21. doi: 10.2307/2136319
Pearlson, G. D., Liu, J., and Calhoun, V. D. (2015). An introductory review of parallel independent component analysis (p-ICA) and a guide to applying p-ICA to genetic data and imaging phenotypes to identify disease-associated biological pathways and systems in common complex disorders. Front. Genet. 6:276. doi: 10.3389/fgene.2015.00276
Pessoa, L. (2011). Emotion and cognition and the amygdala: from ‘what is it?’ to ‘what’s to be done?” Neuropsychologia 48, 3416–3429. doi: 10.1016/j.neuropsychologia.2010.06.038
Plante, N., Courtemanche, R., and Groseilliers, L. D. (2002). “Survey description and methodology - part II – statistical methodology – logitudinal aspects of 1998 to 2000 rounds,” in Québec Longitudinal Study of Child Development (QLSCD 1998-2002) – From Birth to 29 Months (Québec, QC).
Price, A. L., Patterson, N. J., Plenge, R. M., Weinblatt, M. E., Shadick, N. A., and Reich, D. (2006). Principal components analysis corrects for stratification in genome-wide association studies. Nat. Genet. 38, 904–909. doi: 10.1038/ng1847
Puig, M. V., and Gulledge, A. T. (2011). Serotonin and prefrontal cortex function: neurons, networks, and circuits. Mol. Neurobiol. 44, 449–464. doi: 10.1007/s12035-011-8214-0
R Foundation for Statistical Computing (2018). R: A Language and Environment for Statistical Computing, Vol. 2. Avalaible online at: http://www.R-Project.org/ (accessed February 03, 2020).
Radolf, L. S. (1977). The CES-D Scale: a self-report depression scale for research in the general population. Appl. Psychol. Meas. 1, 385–401. doi: 10.1177/014662167700100306
Roisman, G. I., Newman, D. A., Fraley, R. C., Haltigan, J. D., Groh, A. M, Haydon, K. C., et al. (2012). Distinguishing differential susceptibility from diathesis-stress: recommendations for evaluating interaction effects. Dev. Psychopathol. 24, 389–409. doi: 10.1017/S0954579412000065
Saito, R., Smoot, M. E., Ono, K., Ruscheinski, J., Wang, P. L., Lotia, S., et al. (2012). A travel guide to cytoscape plugins. PLoS ONE 9, 1069–1076. doi: 10.1038/nmeth.2212
Sakowski, S. A., Geddes, T. J., Thomas, D. M., Levi, E., Hatfield, J. S., and Kuhn, D. M. (2006). Differential tissue distribution of tryptophan hydroxylase isoforms 1 and 2 as revealed with monospecific antibodies. Brain Res. 1085, 11–18. doi: 10.1016/j.brainres.2006.02.047
Sarter, M., Gehring, W. J., and Kozak, R. (2006). More attention must be Paid: the neurobiology of attentional effort. Brain Res. Rev. 51, 145–160. doi: 10.1016/j.brainresrev.2005.11.002
Sarter, M., Givens, B., and Bruno, J. P. (2001). The cognitive neuroscience of sustained attention: where top-down meets bottom-up. Brain Res. Brain Res. Rev. 35, 146–160. doi: 10.1016/s0165-0173(01)00044-3
Seeger, G., Schloss, P., and Schmidt, M. H. (2001). Marker gene polymorphisms in hyperkinetic disorder–predictors of clinical response to treatment with methylphenidate? Neurosci. Lett. 313, 45–48. doi: 10.1016/s0304-3940(01)02253-4
Shapiro, K., Hillstrom, A. P, and Husain, M. (2002). Control of visuotemporal attention by inferior parietal and superior temporal cortex. Science 12, 1320–1325. doi: 10.1016/s0960-9822(02)01040-0
Silveira, P. P., Pokhvisneva, I., Parent, C., Cai, S., Rema, A. S. S., Broekman, B. F. P., et al. (2017). Cumulative prenatal exposure to adversity reveals associations with a broad range of neurodevelopmental outcomes that are moderated by a novel, biologically informed polygenetic score based on the serotonin transporter solute carrier family C6, member 4 (SLC6A4) gene expression. Dev. Psychopathol. 29, 1601–1617. doi: 10.1017/S0954579417001262
Smith, A. K., Kilaru, V., Klengel, T., Mercer, K. B., Bradley, B., Conneely, K. N., et al. (2015). DNA extracted from saliva for methylation studies of psychiatric traits: evidence tissue specificity and relatedness to brain. Am. J. Med. Genet. B. Neuropsychiatr. Genet. 168B, 36–44. doi: 10.1002/ajmg.b.32278
Soh, S. E., Chong, Y. S., Kwek, K., Saw, S. M., Meaney, M. J., Gluckman, P. D., et al. (2014). Insights from the growing up in singapore towards healthy outcomes (GUSTO) cohort study. Ann. Nutr. Metab. 64, 218–25. doi: 10.1159/000365023
Spielberger, C. D. (2010). “State-trait anxiety inventory,” in The Corsini Encyclopedia of Psychology, eds I. B. Weiner and W. E. Craighead (New York, NY: John Wiley & Sons). doi: 10.1002/9780470479216.corpsy0943
Stelzer, G., Rosen, N., Plaschkes, I., Zimmerman, S., Twik, M., Fishilevich, S., et al. (2016). The genecards suite: from gene data mining to disease genome sequence analyses. Curr. Protoc. Bioinformatics 54–1.30.1–1.30.33. doi: 10.1002/cpbi.5
Strüder, H. K., and Weicker, H. (2001). Physiology and pathophysiology of the serotonergic system and its implications on mental and physical performance. Part I. Int. J. Sports Med. 22, 467–481. doi: 10.1055/s-2001-17605
Suffren, S., Angulo, D., Ding, Y., Reyes, P., Marin, J., Hernandez, J. T., et al. (2017). Long-Term attention deficits combined with subcortical and cortical structural central nervous system alterations in young adults born small for gestational age. Early. Hum. Dev. 110, 44–49. doi: 10.1016/j.earlhumdev.2017.04.016
Tops, M., and Boksem, M. A. (2011). A potential role of the inferior frontal gyrus and anterior insula in cognitive control, brain rhythms, and event-related potentials. Front. Psychol. 2:330. doi: 10.3389/fpsyg.2011.00330
Vickery, T. J., and Jiang, Y. V. (2009). Inferior parietal lobule supports decision making under uncertainty in humans. Cereb. Cortex. 19, 916–925. doi: 10.1093/cercor/bhn140
Vijayendran, M., Beach, S. R., Plume, J. M., Brody, G. H., and Philibert, R. A. (2012). Effects of genotype and child abuse on DNA methylation and gene expression at the serotonin transporter. Fron. Psychiatry. 3:55. doi: 10.3389/fpsyt.2012.00055
Vinkers, C. H., Kalafateli, A. L., Rutten, B. P., Kas, M. J., Kaminsky, Z., Turner, J. D., et al. (2015). Traumatic stress and human dna methylation: a critical review. Epigenomics 7, 593–608. doi: 10.2217/epi.15.11
Wallentin, M., Roepstorff, A., Glover, R., and Burgess, N. (2006). Parallel memory systems for talking about location and age in precuneus, caudate and broca's region. NeuroImage 32, 1850–1864. doi: 10.1016/j.neuroimage.2006.05.002
Warde-Farley, D., Donaldson, S. L., Comes, O., Zuberi, K., Badrawi, R., Chao, P., et al. (2010). The GeneMANIA prediction server: biological network integration for gene prioritization and predicting gene function. Nucleic. Acids. Res. 38 (Suppl. 2), 214–220. doi: 10.1093/nar/gkq537
Wray, N. R., Lee, S. H., Mehta, D., Vinkhuyzen, A. A., Dudbridge, F., and Middeldorp, C. M. (2014). Research review: polygenic methods and their application to psychiatric traits. J. Child. Psychol. Psychiatry 55, 1068–1087. doi: 10.1111/jcpp.12295
Keywords: early environment, serotonin transporter, ADHD, DNA methylation, ePRS, parallel independent component analysis, attention, impulsivity
Citation: de Lima RMS, Barth B, Arcego DM, de Mendonça Filho EJ, Clappison A, Patel S, Wang Z, Pokhvisneva I, Sassi RB, Hall GBC, Kobor MS, O'Donnell KJ, Bittencourt APSV, Meaney MJ, Dalmaz C and Silveira PP (2020) Amygdala 5-HTT Gene Network Moderates the Effects of Postnatal Adversity on Attention Problems: Anatomo-Functional Correlation and Epigenetic Changes. Front. Neurosci. 14:198. doi: 10.3389/fnins.2020.00198
Received: 16 October 2019; Accepted: 24 February 2020;
Published: 17 March 2020.
Edited by:
Deborah Suchecki, Federal University of São Paulo, BrazilReviewed by:
Carina Bodden, University of Melbourne, AustraliaTamas Kozicz, Mayo Clinic, United States
Copyright © 2020 de Lima, Barth, Arcego, de Mendonça Filho, Clappison, Patel, Wang, Pokhvisneva, Sassi, Hall, Kobor, O'Donnell, Bittencourt, Meaney, Dalmaz and Silveira. This is an open-access article distributed under the terms of the Creative Commons Attribution License (CC BY). The use, distribution or reproduction in other forums is permitted, provided the original author(s) and the copyright owner(s) are credited and that the original publication in this journal is cited, in accordance with accepted academic practice. No use, distribution or reproduction is permitted which does not comply with these terms.
*Correspondence: Patrícia Pelufo Silveira, patricia.silveira@mcgill.ca