- 1School of Informatics, Xiamen University, Xiamen, China
- 2Department of Ultrasound, The First Affiliated Hospital of Xiamen University, Xiamen, China
- 3Department of Computing and Mathematics, Manchester Metropolitan University, Manchester, United Kingdom
- 4Department of Electrical Engineering and Computer Science, Cleveland State University, Cleveland, OH, United States
Alzheimer’s disease (AD), which most commonly occurs in the elder, is a chronic neurodegenerative disease with no agreed drugs or treatment protocols at present. Amnestic mild cognitive impairment (aMCI), earlier than AD onset and later than subjective cognitive decline (SCD) onset, has a serious probability of converting into AD. The SCD, which can last for decades, subjectively complains of decline impairment in memory. Distinct altered patterns of default mode network (DMN) subnetworks connected to the whole brain are perceived as prominent hallmarks of the early stages of AD. Nevertheless, the aberrant phase position connectivity (PPC) connected to the whole brain in DMN subnetworks remains unknown. Here, we hypothesized that there exist distinct variations of PPC in DMN subnetworks connected to the whole brain for patients with SCD and aMCI, which might be acted as discriminatory neuroimaging biomarkers. We recruited 27 healthy controls (HC), 20 SCD and 28 aMCI subjects, respectively, to explore aberrant patterns of PPC in DMN subnetworks connected to the whole brain. In anterior DMN (aDMN), SCD group exhibited aberrant PPC in the regions of right superior cerebellum lobule (SCL), right superior frontal gyrus of medial part (SFGMP), and left fusiform gyrus (FG) in comparison of HC group, by contrast, no prominent difference was found in aMCI group. It is important to note that aMCI group showed increased PPC in the right SFGMP in comparison with SCD group. For posterior DMN (pDMN), SCD group showed decreased PPC in the left superior parietal lobule (SPL) and right superior frontal gyrus (SFG) compared to HC group. It is noteworthy that aMCI group showed decreased PPC in the left middle frontal gyrus of orbital part (MFGOP) and right SFG compared to HC group, yet increased PPC was found in the left superior temporal gyrus of temporal pole (STGTP). Additionally, aMCI group exhibited decreased PPC in the left MFGOP compared to SCD group. Collectively, our results have shown that the aberrant regions of PPC observed in DMN are related to cognitive function, and it might also be served as impressible neuroimaging biomarkers for timely intervention before AD occurs.
Introduction
Alzheimer’s disease (AD), which occurs more commonly in the elder, is a chronic neurodegenerative disease of impaired cognitive and memory (Ren et al., 2019; Wessels et al., 2019). Nevertheless, there are no agreed drugs or treatment protocols for patients with AD. Therefore, the timely detection and treatment of the early stages of AD is an urgent and realistic issue, which can improve symptoms of illness and alleviate the progression of the disease (Yang et al., 2019). Amnestic mild cognitive impairment (aMCI), as the phase close to AD, has a 10–15% probability of converting into AD per annum (Yang et al., 2017). Subjective cognitive decline (SCD) is referring to the fact that impaired cognition appealed by patients is entirely subjective without objective behavior of cognitive decline (Funaki et al., 2019), which has the certain likelihood of the development into aMCI and AD stages (Caillaud et al., 2019; Kim et al., 2020). Thus, from the above, we aim to adopt aMCI and SCD phases which may evolve into AD to explore the neural mechanism of the early stage of AD.
Resting-state functional magnetic resonance imaging (rs-fMRI), as one of the neuroimaging techniques, can offer a noninvasive method for the assessment of the cognitive mechanism of AD to a certain degree (Ferenci et al., 2002). Besides, in most studies of the early stage of AD based on rs-fMRI, the default mode network (DMN) has been emphasized highly for a long time (Banks et al., 2018). Anatomically, it includes the anterior DMN (aDMN) which is primarily composed of the ventromedial prefrontal cortex (vmPFC), and posterior DMN (pDMN) which mainly focuses on the posterior cingulate cortex (PCC) (Xu et al., 2016). Notice that the former mainly concerns memory extinction and self-referential mental idealization, while the latter is closely related to the function of episodic memory retrieval (Yang et al., 2017, 2018). Previously, it has been reported that amyloid deposits are detected with a great probability in the PCC which served as a primary part of DMN (Wang et al., 2013). The previous work has also indicated that the regions of DMN are abnormal and may serve as prominent hallmarks in the early stage of AD such as mild cognitive impairment (MCI) and SCD (Huang et al., 2018a; Scherr et al., 2019; Xie et al., 2019). More specifically, these disconnected areas that are connected from the DMN to the whole brain are considered to be associated with cognitive disorder (Huang et al., 2019; Zhao et al., 2020).
Hilbert transform (HT), which is characterized by rapidly and accurately describing the instantaneous position phase, is suitable for the analysis of non-stationary signals (Peng et al., 2005; Qian et al., 2015). A former study has suggested that frequency domain estimations such as the instantaneous phase position would provide a higher correlation between two signals than time-domain signals (Mandel and Atkins, 2016). Previous studies based on electroencephalography (EEG) have shown that prominently increased and decreased alpha spectral powers are found using HT in patients with AD in comparison with healthy controls (HC) (Babiloni et al., 2006; Fraga et al., 2013; Wang et al., 2020). Furthermore, it has been reported that time series from EEG was employed to obtain the altered instantaneous phase position of DMN using the HT method, which can be served as biomarkers (Thatcher et al., 2014; Wang et al., 2019). A previous investigation has also revealed that the brain activation patterns of DMN measured by HT were altered between the eye open and the closed eye (Wang et al., 2015). Besides, the former work on depressive disorder has indicated that the signals, extracted from the interesting regions of rs-fMRI, can reveal the aberrant brain regions using the HT method (Yu et al., 2018). Until now no experiments of HT in AD based on rs-fMRI, especially in DMN subnetworks, have been reported, which may be a new way to explore the neural mechanism of the early stage of AD.
Accordingly, our target aims to analyze the altered patterns of instantaneous phase position connectivity (PPC) in DMN subnetworks (include aDMN and pDMN networks) connected to the whole brain, and to explore whether there exists a relationship between the cognitive function and the aberrant regions. We hypothesized that there exist distinct variations of PPC in DMN subnetworks for patients SCD and aMCI, which might act as discriminatory neuroimaging biomarkers.
Materials and Methods
Participant
Our experimental participants in this work are briefly summarized as follows: All subjects are obtained from the public database of the second phase of Alzheimer’s Disease Neuroimaging Initiative (ADNI-2)1. The emphasis of ADNI-2 is to survey neurological biomarkers of cognitive disorder. Furthermore, it was announced in 2011 which had been lasting for 5 years to implement. To further explore the gap between the healthy subjects and patients with MCI, consider joining the subjects with SCD for the first time in ADNI-2. All recruited subjects are consist of three parts, HC (n = 28), SCD (n = 23), and aMCI (n = 29), respectively. It deserves to be further mentioned that we have precluded three subjects as the result of undue head movement (cumulative translation or rotation >1.5 mm or 1.5 degrees were executed in our work, n = 3). At the same time, we strictly control registration quality relying on artificial visual recognition due to the poor registration of certain subjects (n = 2). To sum up, we recruited 75 subjects, including 27 HC, 20 SCD, and 28 aMCI participants. The detail data selection process is shown in Figure 1.
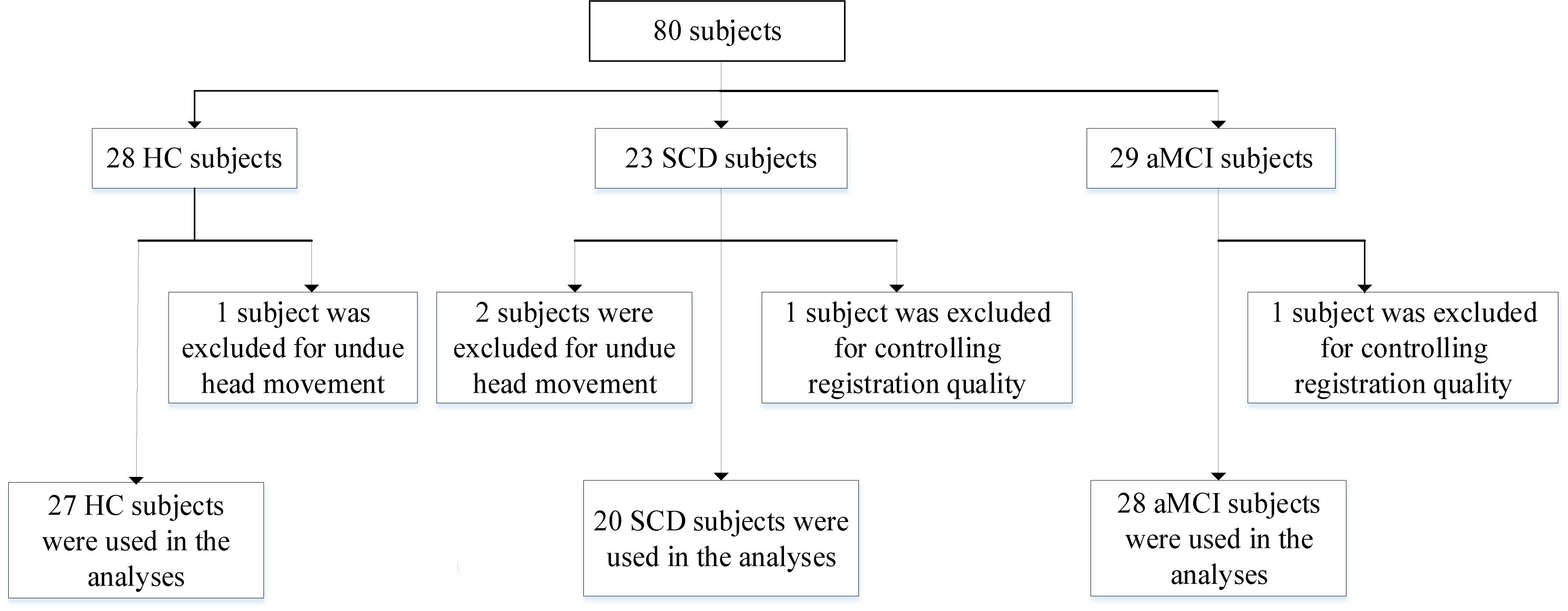
Figure 1. A flow chart depicting the data selection process. HC, healthy controls; SCD, subjective cognitive decline; aMCI, amnestic mild cognitive impairment.
MRI Data Acquisition
The obtained participants underwent rs-fMRI on a clinical 3.0 tesla (T) scanner (Philips Medical Systems). The echo-plane imaging (EPI) sequence consists of 140 volumes in which subjects were required to lie flat, close eyes, avoid conceptual work, and not turn the head during the process of data acquisition. Here, the specific parameters can be briefly described as: flip angle (FA) = 80 degree, matrix = 64 × 64, voxel size = 3.31 mm × 3.31 mm × 3.31 mm, repetition time (TR) = 3000 ms, echo time (TE) = 30 ms, slice thickness = 3.3 mm, respectively. T1-weighted structural MRI were acquired from magnetization-prepared rapid gradient-echo (MPRAGE) sequence (Chen et al., 2016), likewise, detailed parameters are represented as: matrix = 256 × 256, layer thickness = 1.2 mm, voxel size = 1 × 1 × 1.2 mm3, TR = 6.81 ms, FA = 9 degree, TE = 3.16 ms, respectively.
Data Preprocessing
The data preprocessing with aspect to the rs-fMRI of this work adopted Resting-State fMRI Data Analysis Toolkit plus (RESTplus)2, which is based on MATLAB2012a3 and Statistical Parametric Mapping (SPM12)4. Briefly steps on data preprocessing involving as follows: We discarded the first 5 of 140 points in time for each subject as the result of the instability of MRI signal induced by machine or human. Then, the remaining volumes were calibrated for controlling the effects of between slices or between subject volumes. It is stressed that we have ruled out the data on the condition of cumulative translation more than 1.5 mm or angular motion more than 1.5 degrees. Next in the normalization process, registration from the original space to Montreal Neurological Institute (MNI) space using T1-weight volumes was implemented to reduce the diversities between various subjects. To directly circumvent the subject variations, the images generated above have been smoothed using a Gaussian kernel of 6 × 6 × 6 of full width at half maximum (FWHW). Following this, to eliminate the influence of nuisance variables (Fox et al., 2009; Huang et al., 2018b), comprising of six head motion parameters, global mean signal, white matter signal and cerebrospinal fluid signal, were ruled out, respectively. At last, subject volumes were filtered at 0.01–0.08 Hz due to noise interferences, which may be induced by heartbeat and breathing.
Statistical Analysis
For this study, the variance (ANOVA) and the chi-square test within the Statistical Package for the Social Sciences (SPSS) software version 22.0 were conducted to determine whether there exist prominent differences (p < 0.05) as to the data of demographic and neurocognitive between the HC, SCD and aMCI groups.
To better illustrate the differences of PPC in aDMN and pDMN networks between HC, SCD, and aMCI groups, one-way ANOVA, which is integrated into the software of Data Processing and Analysis for Brain Imaging (DPABI)5, was adopted after controlling the gender and age. As mentioned in former literature, multiple comparisons at cluster level using non-parametric permutation test can availably control the false positive rate existing in statistics (Winkler et al., 2016), and 1000 permutation times and the prominent cluster size >30 voxels (810 mm3, p < 0.05) were performed in this work. We have employed the two-sample T-test for calculating the differences between two groups (i.e., SCD and HC groups, aMCI and HC groups, aMCI and SCD groups), and that mask used in two-sample T-test was derived from ANOVA analysis. Besides, according to the recent report, there have identified that the non-parametric permutation test with Threshold-Free Cluster Enhancement (TFCE) can effectively achieve a good balance between family wise error (FWE) rate and reliability (Chen et al., 2018). Therefore, permutation test associated with TFCE, all integrated into PLAM module of DPABI, were employed as the method of multiple comparisons of this work, and we set cluster size >10 voxels (270 mm3, p < 0.05) as a prominent cluster (Xue et al., 2019).
PPC of Two Time Series Using Hilbert Transform
The HT can analyze nonlinear and non-stationary signals, and it is fully self-adaptive and suitable for mutation signals. Besides, it is characterized by rapidly and accurately describing the instantaneous phase position which was adopted to explore the altered patterns of PPC of DMN subnetworks in this work (Martis et al., 2012). Hence, the HT was adopted using complex demodulation to compute the instantaneous phase position difference between each pair of the time series. Among them, one series is extracted from aDMN (or pDMN), the other series is extracted from the voxel within the whole brain. The HT that we follow can be briefly described as follows: Given a time series name as x(t), and the HT later referred to as H(t), is shown in formula (1).
We first take two time series which represent aDMN (or pDMN) signal and the voxel signal of the brain as inputs to the formula (1) respectively. Next, using analytic signal Z(t) of x(t) to generate information about the phase position and amplitude, as shown in formula (2). Therefore, two analytic signals of aDMN (or pDMN) and the voxel of the brain are obtained.
where A(t) represents signal amplitude, and ϕx(t) is the instantaneous phase position of the signal. Then, we obtain phase difference D(t) according to phase positions of two signals, consisting of ϕx1(t) and ϕx2(t), and it is shown in formula (3). Here, we set the phase lock ratio as m = n = 1, and it indicates that the increase phase of two phase position is consistent.
Phase synchronization exponent of two signals can be seen from the formula (4).
Note that < ⋅ >t is the average at each time point with values ranging from 0 to 1. Furthermore, λ = 0 indicates no phase synchronization of two signals, whereas λ = 1 shows phase synchronization of two signals. That is, the larger the value of λ, the stronger the synchronization between two signals. Moreover, the seed-based method was conducted to analyze the altered PPC of DMN subnetworks. To identify the seed regions about aDMN and pDMN, 10-mm spherical regions of interest in aDMN (MNI space: 0, 52, −6) and pDMN (MNI space: 0, −53, 26) were adopted in our work (Zhang and Raichle, 2010; Xue et al., 2019). Collectively, we applied phase position synchronization generated by HT to investigate the altered connectivity between the DMN subnetworks and the whole brain, and aim to explore the relationship between the aberrant regions and cognitive function.
Results
Demographic and Neurocognitive Characteristics
Here, the demographic and neurocognitive characteristics of experimental participants were described in Table 1. The prominent differences verified by ANOVA analysis were the Age (F = 8.248, p = 0.016), the MMSE score (F = 9.129, p < 0.01), and CDR score (F = 68.98, p < 0.01), respectively, yet no prominent difference on the Gender (F = 2.026, p > 0.05). The results showed that the MMSE values of the HC group (29.14 ± 1.49), SCD group (28.94 ± 0.83) and aMCI group (26.87 ± 2.72) decreased successively. It is noteworthy that lower MMSE scores suggest the severe form of cognitive impairment, whereas higher CDR scores show much more serious for dementia.
The Aberrant PPC of aDMN Network in Patients With SCD and aMCI
The studies we have performed indicated that eight prominent clusters, comprising of right superior cerebellum lobule (SCL), right rectus (REC), left fusiform gyrus (FG), left inferior frontal gyrus of triangular part (IFGTP), left middle temporal gyrus (MTG), right middle frontal gyrus (MFG), left MFG and right superior frontal gyrus of medial part (SFGMP), were revealed according to one-way ANOVA analysis. Besides, patients with SCD exhibited aberrant PPC in the clusters of right SCL, right SFGMP and left FG as compared with HC group, by contrast, no significant difference was found in patients with aMCI resulted from two-sample T-test. Notably, aside from decreased PPC found in the left FG, the clusters of increased PPC were involved in the right SCL and right SFGMP. It is important to note that patients with aMCI showed increased PPC in the right SFGMP in comparison with the SCD group (TFCE-FWE corrected, cluster size ≥ 10 voxels, p < 0.05). In particular, we emphasize that our experiments are after controlling the effects of age and gender (see Table 2 and Figures 2–4).

Figure 2. The prominent differences of the brain in PPC of the aDMN network using one-way ANOVA analysis. L, left; R, right; MTG, middle frontal gyrus; REC, rectus; FG, fusiform gyrus; IFGTP, inferior frontal gyrus of triangular part; SCL, superior cerebellum lobule; MFG, middle frontal gyrus; SFGMP, superior frontal gyrus of medial part.
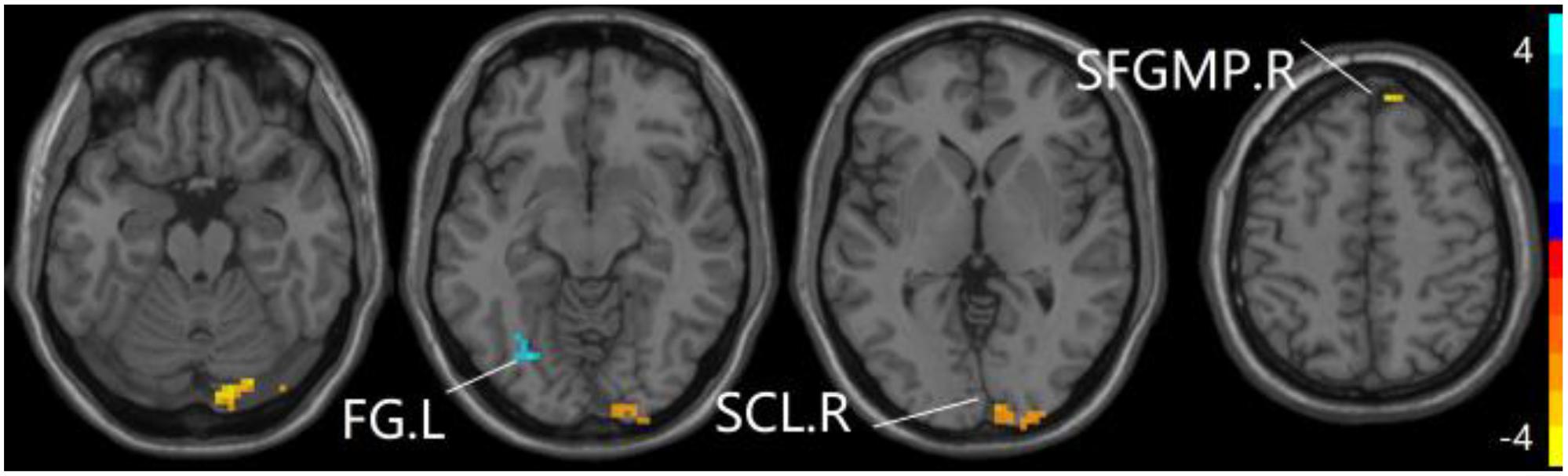
Figure 3. Compared to HC group, the SCD group exhibits prominent differences in PPC of the aDMN network based on two-sample T-test. L, left; R, right; FG, fusiform gyrus; SCL, superior cerebellum lobule; SFGMP, superior frontal gyrus of medial part.
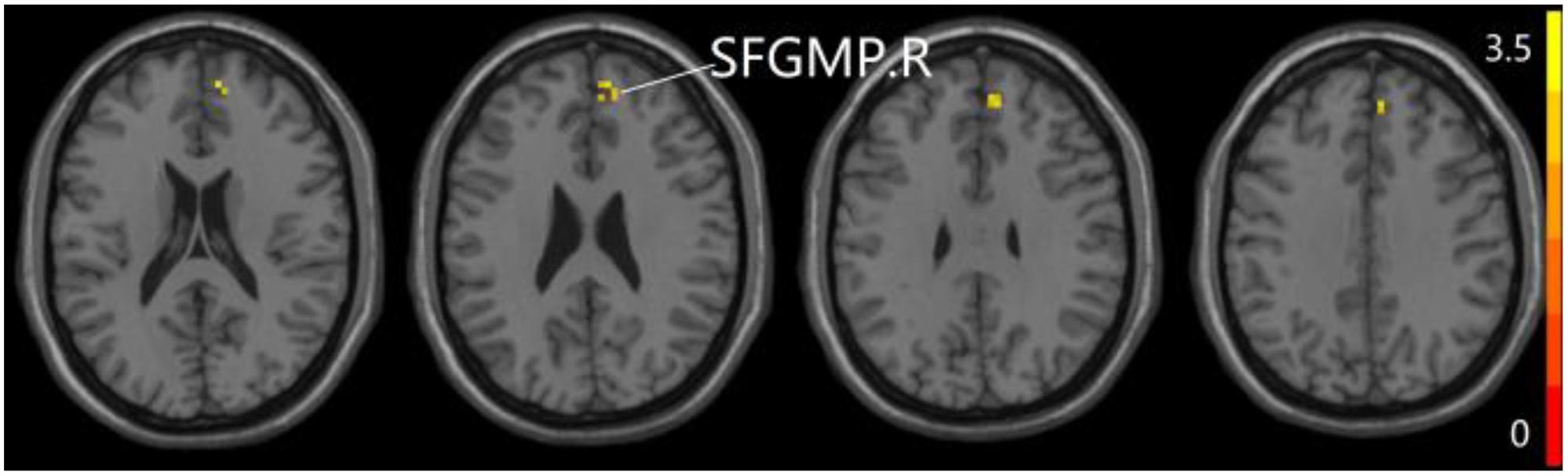
Figure 4. Compared to SCD group, the aMCI group exhibits prominent differences in PPC of the aDMN network based on two-sample T-test. R, right; SFGMP, superior frontal gyrus of medial part.
The Aberrant PPC of pDMN Network in Patients With SCD and aMCI
For pDMN network, the one-way ANOVA analysis we have done suggested the prominent differences in four clusters, consisting of the left superior temporal gyrus of temporal pole (STGTP), left MFL, left superior parietal lobule (SPL) and right superior frontal gyrus (SFG). Compared to HC group, patients with SCD showed decreased PPC in the left SPL and right SFG. It is noteworthy that aMCI group showed decreased PPC in the left middle frontal gyrus of orbital part (MFGOP) and right SFG, yet increased PPC was found in the left STGTP. Additionally, aMCI group exhibited decreased PPC in the left MFGOP as the comparison with SCD group (TFCE-FWE corrected, cluster size ≥ 10 voxels, p < 0.05). The experimental data used in the PPC analysis are after controlling the influences of gender and age (see Table 3 and Figures 5–8).

Figure 5. The prominent differences of the brain in PPC of the pDMN network based on one-way ANOVA analysis. L, left; R, right; STGTP, superior temporal gyrus of temporal pole; MFL, middle frontal lobule; SPL, superior parietal lobule; SFG, superior frontal gyrus.
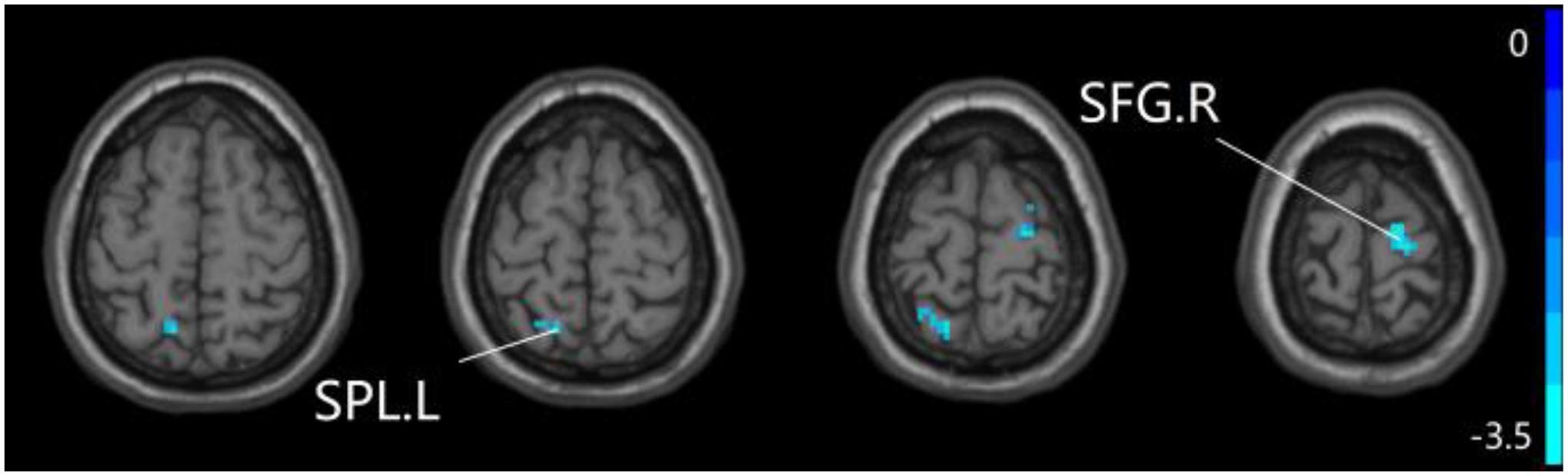
Figure 6. Compared to HC group, the SCD group exhibits prominent differences in PPC of the pDMN network based on two-sample T-test. L, left; R, right; SPL, superior parietal lobule; SFG, superior frontal gyrus.
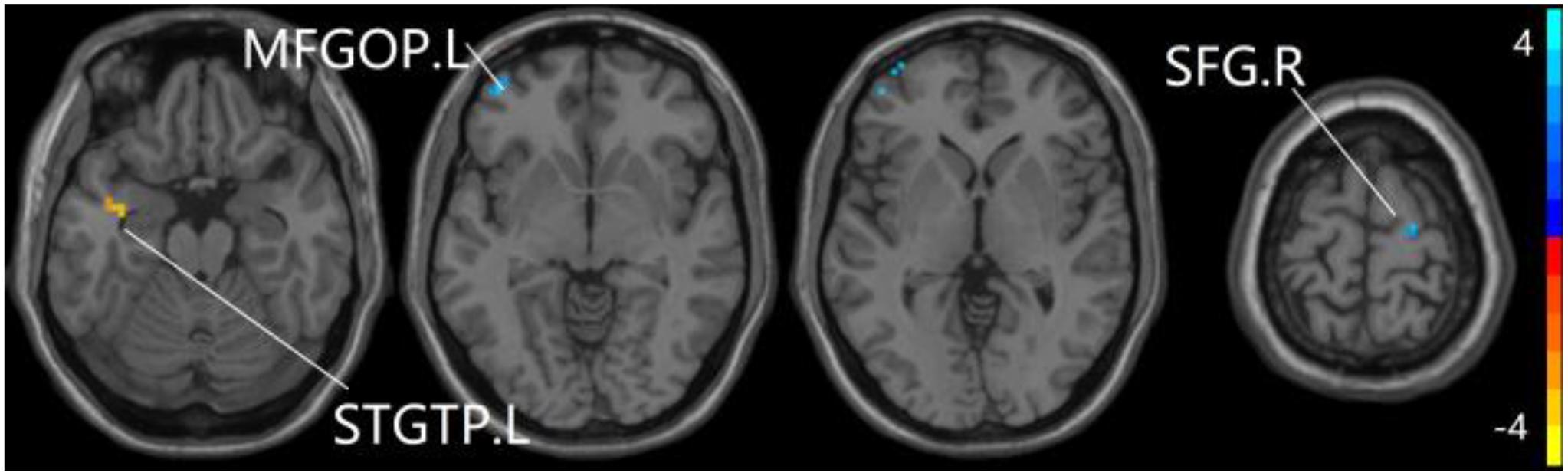
Figure 7. Compared to HC group, the aMCI group exhibits prominent differences in PPC of the pDMN network based on two-sample T-test. L, left; R, right; STGTP, superior temporal gyrus of temporal pole; SFG, superior frontal gyrus; MFGOP, middle frontal gyrus of orbital part.
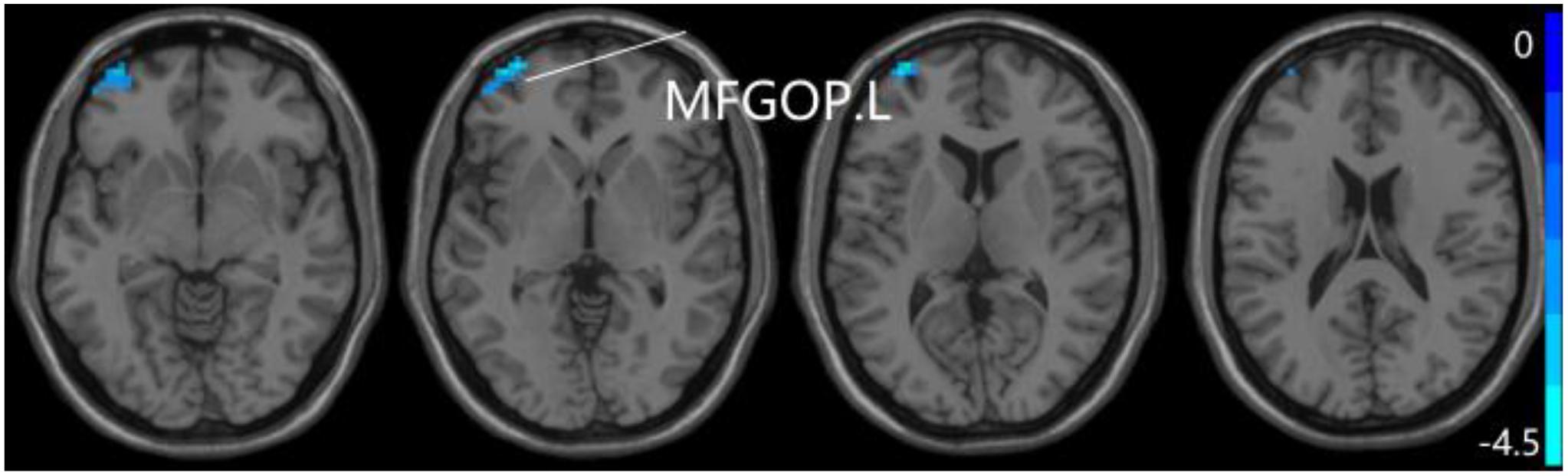
Figure 8. Compared to SCD group, the aMCI group exhibits prominent differences in PPC of the pDMN network based on two-sample T-test. L, left; MFGOP, middle frontal gyrus of orbital part.
Discussion
The work presented in this paper is that it focuses on investigating aberrant patterns of PPC of the DMN subnetworks connected to the whole brain between HC, SCD, and aMCI groups, and analyzing whether the aberrant areas are related with cognitive function. The result was consistent with the hypothesis that the SCD and aMCI groups exhibited distinct abnormal PCC in DMN subnetworks and the alerted regions were related to cognitive function. Besides, the altered regions in DMN subnetworks might regard as neuroimaging biomarkers and may be used to better understand the neural mechanism for the early stages of AD.
For aDMN, patients with SCD have prominently altered regions of the right SCL, right SFGMP and left FG resulted from the two-sample T-test. Based on earlier finds, SCL is involved in articulatory control and non-motor cognitive function (Ferenci et al., 2002). The left FG plays a critical role in semantic dementia and is repeatedly reported to be involved in visual word processing (Peterburs et al., 2019). SFG, which is located on top of the brain, plays a role in several higher-level cognitive processes and working memory according to a previous report (Alagapan et al., 2019). Combining the altered regions mentioned above, these regions are related to language and memory, which are consistent with the manifestation of cognitive function (Samaras et al., 2014). Interestingly, no prominent regions were found in aDMN network in aMCI group, while aMCI group exhibited prominent regions in left STGTP, left MFGOP and right SFG in pDMN. A former study has reported that STG is anchored in the auditory association cortex involving spoken word recognition and MFG is related to working memory (Fegen et al., 2015; Kajikawa et al., 2015). Hence, the altered regions we found might involve in language cognitive disorder, and pDMN first appears abnormal connections compared to aDMN, which might suggest that the aDMN and pDMN have different manifestations in the early stages of AD.
We found that both aMCI and SCD groups showed a prominent region in SFG that is connected to DMN subnetworks, and according to the previous research that the altered functional connectivity between the pDMN and the SFG might be a compensatory response of brain (Xue et al., 2019). We assume that the aberrant PPC in SFG connected to the DMN may also be caused by the brain’s compensation. Compared to HC group, aMCI group showed a prominent region in STG which did not belong to the altered region of SCD group, while SCD group showed prominent regions in SCL which did not belong to the altered region of aMCI group. It can be deduced that SCL and STG, related to articulatory control and working memory, respectively, are sensitive and might as neuroimaging biomarkers to distinguish the SCD and aMCI. Interestingly, compared to patients with SCD, DMN subnetworks in aMCI group showed obvious differences in right SFGMP and left MFGOP belonging to FG. In previous studies, aMCI group showed more widespread topological changes involving the frontal lobes (Barban et al., 2017), and aberrant connectivity was also found in patients with SCD between DMN and FG due to cognitive impairment (Xue et al., 2019). Besides, an increasing trend of values of amplitude of low-frequency fluctuation (ALFF) and fractional ALFF were detected in FG (Yang et al., 2018). Therefore, changes in FG may be related to cognitive dysfunction.
Conclusion
Our study mainly shows that the PPC of the DMN subnetworks which are connected to the whole brain has different disconnection patterns in SCD and aMCI stages. Moreover, the significant difference in DMN subnetworks varies considerably, which might act as neuroimaging biomarkers of sensitivity for timely detection of the early stage of AD.
Data Availability Statement
Publicly available datasets were analyzed in this study. This data can be found here: http://adni.loni.usc.edu/.
Author Contributions
CC was responsible for writing the manuscript and doing the experiment. CH, CY, and XZ instructed the experiment. WZ, XH, FR, DH, YX, and JY participated in the experiment of manuscript and checked the English grammar. YP developed the idea for the study and analyzed most of the data.
Funding
This work was supported by the Natural Science Foundation of Fujian Province, China (No. 2017J01372) and the Foundation of Fujian Educational Committee (No. JK2015019).
Conflict of Interest
The authors declare that the research was conducted in the absence of any commercial or financial relationships that could be construed as a potential conflict of interest.
Footnotes
- ^ http://adni.loni.usc.edu/
- ^ http://restfmri.net/forum/RESTplusV1.2
- ^ http://www.mathworks.com/products/matlab/
- ^ https://www.fil.ion.ucl.ac.uk/spm/
- ^ http://rfmri.org/dpabi
References
Alagapan, S., Lustenberger, C., Hadar, E., Shin, H. W., and Frhlich, F. (2019). Low-frequency direct cortical stimulation of left superior frontal gyrus enhances working memory performance. Neuroimage 184, 697–706. doi: 10.1016/j.neuroimage.2018.09.064
Babiloni, C., Binetti, G., Cassetta, E., Dal Forno, G., Del Percio, C., Ferreri, F., et al. (2006). Sources of cortical rhythms change as a function of cognitive impairment in pathological aging: a multicenter study. Clin. Neurophysiol. 117, 252–268. doi: 10.1016/j.clinph.2005.09.019
Banks, S. J., Zhuang, X., Bayram, E., Bird, C., Cordes, D., Caldwell, J. Z. K., et al. (2018). Default mode network lateralization and memory in healthy aging and Alzheimer’s disease. J. Alzheimers Dis. 66, 1223–1234. doi: 10.3233/jad-180541
Barban, F., Mancini, M., Cercignani, M., Adriano, F., Perri, R., Annicchiarico, R., et al. (2017). A pilot study on brain plasticity of functional connectivity modulated by cognitive training in mild Alzheimer’s disease and mild cognitive impairment. Brain Sci. 7:50. doi: 10.3390/brainsci7050050
Caillaud, M., Hudon, C., Boller, B., Brambati, S., Duchesne, S., Lorrain, D., et al. (2019). Evidence of a relation between hippocampal volume, white matter hyperintensities, and cognition in subjective cognitive decline and mild cognitive impairment. J. Gerontol. B Psychol. Sci. Soc. Sci. 26:gbz120. doi: 10.1093/geronb/gbz120
Chen, J., Duan, X., Shu, H., Wang, Z., Long, Z., Liu, D., et al. (2016). Differential contributions of subregions of medial temporal lobe to memory system in amnestic mild cognitive impairment: insights from fMRI study. Sci. Rep. 6:26148. doi: 10.1038/srep26148
Chen, X., Lu, B., and Yan, C. G. (2018). Reproducibility of R-fMRI metrics on the impact of different strategies for multiple comparison correction and sample sizes. Hum. Brain Mapp. 39, 300–318. doi: 10.1002/hbm.23843
Fegen, D., Buchsbaum, B. R., and D’Esposito, M. (2015). The effect of rehearsal rate and memory load on verbal working memory. Neuroimage 105, 120–131. doi: 10.1016/j.neuroimage.2014.10.034
Ferenci, P., Lockwood, A., Mullen, K., Tarter, R., Weissenborn, K., and Blei, A. T. (2002). Hepatic encephalopathy–definition, nomenclature, diagnosis, and quantification: final report of the working party at the 11th World Congresses of Gastroenterology, Vienna, 1998. Hepatology 35, 716–721. doi: 10.1053/jhep.2002.31250
Fox, M. D., Zhang, D., Snyder, A. Z., and Raichle, M. E. (2009). The global signal and observed anticorrelated resting state brain networks. J. Neurophysiol. 101, 3270–3283. doi: 10.1152/jn.90777.2008
Fraga, F. J., Falk, T. H., Kanda, P. A., and Anghinah, R. (2013). Characterizing Alzheimer’s disease severity via resting-awake EEG amplitude modulation analysis. PLoS One 8:e72240. doi: 10.1371/journal.pone.0072240
Funaki, K., Nakajima, S., Noda, Y., Wake, T., Ito, D., Yamagata, B., et al. (2019). Can we predict amyloid deposition by objective cognition and regional cerebral blood flow in patients with subjective cognitive decline? Psychogeriatrics 19, 325–332. doi: 10.1111/psyg.12397
Huang, C., Tian, G., Lan, Y., Peng, Y., Ng, E. Y. K., Hao, Y., et al. (2019). A new pulse coupled neural network (PCNN) for brain medical image fusion empowered by shuffled frog leaping algorithm. Front. Neurosci. 13:210. doi: 10.3389/fnins.2019.00210
Huang, C., Xiaoying, S., Yisha, L., Lu, L., Haidong, C., Wenliang, C., et al. (2018a). A hybrid active contour segmentation method for myocardial D-SPECT images. IEEE Access 6, 39334–39343. doi: 10.1109/access.2018.2855060
Huang, C., Xie, Y., Lan, Y., Hao, Y., Chen, F., Cheng, Y., et al. (2018b). A new framework for the integrative analytics of intravascular ultrasound and optical coherence tomography images. IEEE Access 6, 36408–36419. doi: 10.1109/access.2018.2839694
Kajikawa, Y., Frey, S., Ross, D., Falchier, A., Hackett, T. A., and Schroeder, C. E. (2015). Auditory properties in the parabelt regions of the superior temporal gyrus in the awake macaque monkey: an initial survey. J. Neurosci. 35, 4140–4150. doi: 10.1523/JNEUROSCI.3556-14.2015
Kim, J. G., Ahn, C., Shin, H., Kim, W., Lim, T. H., Jang, B. H., et al. (2020). Efficacy of the cooling method for targeted temperature management in post-cardiac arrest patients: a systematic review and meta-analysis. Resuscitation 148, 14–24. doi: 10.1016/j.resuscitation.2019.12.025
Mandel, J. E., and Atkins, J. H. (2016). Hilbert-Huang transform yields improved minute volume estimates from respiratory inductance plethysmography during transitions to paradoxical breathing. Anesth. Analg. 122, 126–131. doi: 10.1213/ANE.0000000000000969
Martis, R., Acharya, U. R., Tan, J. H., Petznick, A., Yanti, R., Chua, K., et al. (2012). Application of empirical mode decomposition (EMD) for automated detection of epilepsy using EEG signals. Int. J. Neural Syst. 22:1250027. doi: 10.1142/S012906571250027X
Peng, Z. K., Tse, P. W., and Chu, F. L. (2005). A comparison study of improved Hilbert–Huang transform and wavelet transform: application to fault diagnosis for rolling bearing. Mech. Syst. Signal Process. 19, 974–988. doi: 10.1016/j.ymssp.2004.01.006
Peterburs, J., Blevins, L. C., Sheu, Y. S., and Desmond, J. E. (2019). Cerebellar contributions to sequence prediction in verbal working memory. Brain Struct. Funct. 224, 485–499. doi: 10.1007/s00429-018-1784-0
Qian, L., Zhang, Y., Zheng, L., Shang, Y., Gao, J. H., and Liu, Y. (2015). Frequency dependent topological patterns of resting-state brain networks. PLoS One 10:e0124681. doi: 10.1371/journal.pone.0124681
Ren, C., Li, D., Zhou, Q., and Hu, X. (2019). Mitochondria-targeted TPP-MoS2 with dual enzyme activity provides efficient neuroprotection through M1/M2 microglial polarization in an Alzheimer’s disease model. Biomaterials 232:119752. doi: 10.1016/j.biomaterials.2019.119752
Samaras, K., Lutgers, H. L., Kochan, N. A., Crawford, J. D., Campbell, L. V., Wen, W., et al. (2014). The impact of glucose disorders on cognition and brain volumes in the elderly: the Sydney memory and ageing study. Age (Dordr.) 36, 977–993. doi: 10.1007/s11357-013-9613-0
Scherr, M., Utz, L., Tahmasian, M., Pasquini, L., Grothe, M. J., Rauschecker, J. P., et al. (2019). Effective connectivity in the default mode network is distinctively disrupted in Alzheimer’s disease-A simultaneous resting-state FDG-PET/fMRI study. Hum. Brain Mapp. doi: 10.1002/hbm.24517 [Epub ahead of print].
Thatcher, R. W., North, D. M., and Biver, C. J. (2014). LORETA EEG phase reset of the default mode network. Front. Hum. Neurosci. 8:529. doi: 10.3389/fnhum.2014.00529
Wang, T., Luo, H., Zheng, X., and Xie, M. (2019). Crowdsourcing mechanism for trust evaluation in CPCS based on intelligent mobile edge computing. ACM Trans. Intell. Syst. Technol. 10:62.
Wang, T., Mei, Y., Jia, W., Zheng, X., Wang, G., and Xie, M. (2020). Edge-based differential privacy computing for sensor-cloud systems. J. Parallel Distrib. Comput. 136, 75–85. doi: 10.1016/j.jpdc.2019.10.009
Wang, X. H., Li, L., Xu, T., and Ding, Z. (2015). Investigating the temporal patterns within and between intrinsic connectivity networks under eyes-open and eyes-closed resting states: a dynamical functional connectivity study based on phase synchronization. PLoS One 10:e0140300. doi: 10.1371/journal.pone.0140300
Wang, Y., Risacher, S. L., West, J. D., McDonald, B. C., Magee, T. R., Farlow, M. R., et al. (2013). Altered default mode network connectivity in older adults with cognitive complaints and amnestic mild cognitive impairment. J. Alzheimers Dis. 35, 751–760. doi: 10.3233/JAD-130080
Wessels, A. M., Tariot, P. N., Zimmer, J. A., Selzler, K. J., Bragg, S. M., Andersen, S. W., et al. (2019). Efficacy and safety of lanabecestat for treatment of early and mild Alzheimer disease: the AMARANTH and DAYBREAK-ALZ randomized clinical trials. JAMA Neurol. [Epub ahead of print].
Winkler, A. M., Ridgway, G. R., Douaud, G., Nichols, T. E., and Smith, S. M. (2016). Faster permutation inference in brain imaging. Neuroimage 141, 502–516. doi: 10.1016/j.neuroimage.2016.05.068
Xie, Y., Liu, T., Ai, J., Chen, D., Zhuo, Y., Zhao, G., et al. (2019). Changes in centrality frequency of the default mode network in individuals with subjective cognitive decline. Front. Aging Neurosci. 11:118. doi: 10.3389/fnagi.2019.00118
Xu, X., Yuan, H., and Lei, X. (2016). Activation and connectivity within the default mode network contribute independently to future-oriented thought. Sci. Rep. 6:21001. doi: 10.1038/srep21001
Xue, C., Yuan, B., Yue, Y., Xu, J., Wang, S., Wu, M., et al. (2019). Distinct disruptive patterns of default mode subnetwork connectivity across the spectrum of preclinical Alzheimer’s disease. Front. Aging Neurosci. 11:307. doi: 10.3389/fnagi.2019.00307
Yang, H., Wang, C., Zhang, Y., Xia, L., Feng, Z., Li, D., et al. (2017). Disrupted causal connectivity anchored in the posterior cingulate cortex in amnestic mild cognitive impairment. Front. Neurol. 8:10. doi: 10.3389/fneur.2017.00010
Yang, J., Zeng, F., Ge, Y., Peng, K., Li, X., Li, Y., et al. (2019). Development of near-infrared fluorescent probes for use in Alzheimer’s disease diagnosis. Bioconjug. Chem. 31, 2–15. doi: 10.1021/acs.bioconjchem.9b00695
Yang, L., Yan, Y., Wang, Y., Hu, X., Lu, J., Chan, P., et al. (2018). Gradual disturbances of the amplitude of low-frequency fluctuations (ALFF) and fractional ALFF in Alzheimer spectrum. Front. Neurosci. 12:975. doi: 10.3389/fnins.2018.00975
Yu, H., Li, F., Wu, T., Li, R., Yao, L., Wang, C., et al. (2018). Functional brain abnormalities in major depressive disorder using the Hilbert-Huang transform. Brain Imaging Behav. 12, 1556–1568. doi: 10.1007/s11682-017-9816-6
Zhang, D., and Raichle, M. E. (2010). Disease and the brain’s dark energy. Nat. Rev. Neurol. 6, 15–28.
Keywords: subjective cognitive decline, amnestic mild cognitive impairment, default mode network, phase position connectivity, neuroimaging biomarkers
Citation: Cai C, Huang C, Yang C, Zhang X, Peng Y, Zhao W, Hong X, Ren F, Hong D, Xiao Y and Yan J (2020) Altered Patterns of Phase Position Connectivity in Default Mode Subnetwork of Subjective Cognitive Decline and Amnestic Mild Cognitive Impairment. Front. Neurosci. 14:185. doi: 10.3389/fnins.2020.00185
Received: 02 February 2020; Accepted: 19 February 2020;
Published: 20 March 2020.
Edited by:
Yizhang Jiang, Jiangnan University, ChinaReviewed by:
Wenzheng Bao, Xuzhou University of Technology, ChinaTian Wang, Huaqiao University, China
Copyright © 2020 Cai, Huang, Yang, Zhang, Peng, Zhao, Hong, Ren, Hong, Xiao and Yan. This is an open-access article distributed under the terms of the Creative Commons Attribution License (CC BY). The use, distribution or reproduction in other forums is permitted, provided the original author(s) and the copyright owner(s) are credited and that the original publication in this journal is cited, in accordance with accepted academic practice. No use, distribution or reproduction is permitted which does not comply with these terms.
*Correspondence: Chenxi Huang, supermonkeyxi@xmu.edu.cn; Chenhui Yang, ych987@126.com; Xiaodong Zhang, zxdon11@163.com