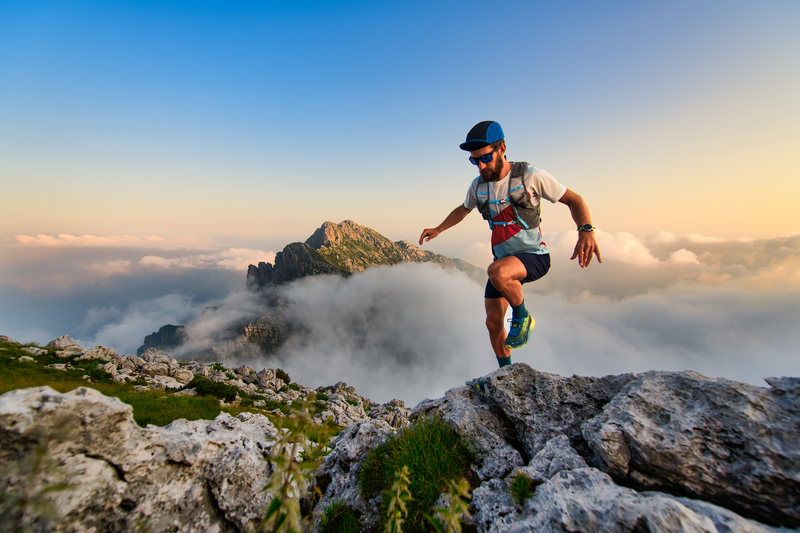
95% of researchers rate our articles as excellent or good
Learn more about the work of our research integrity team to safeguard the quality of each article we publish.
Find out more
ORIGINAL RESEARCH article
Front. Neurosci. , 15 November 2019
Sec. Neurodegeneration
Volume 13 - 2019 | https://doi.org/10.3389/fnins.2019.01228
Background and Purpose: Amyloid imaging, gray matter (GM) morphometry and diffusion tensor imaging (DTI) have all been used as predictive biomarkers in dementia. Our objective was to define the imaging profile of healthy elderly controls as a function of their cognitive trajectories and explore whether amyloid burden and white matter (WM) microstructure changes are associated with subtle decrement of neuropsychological performances in old age.
Materials and Methods: We performed a 4.5-year longitudinal study in 133 elderly individuals who underwent cognitive testing at inclusion and follow-up, amyloid PET, MRI including DTI sequences at inclusion, and APOE epsilon 4 genotyping. All cases were assessed using a continuous cognitive score (CCS) taking into account the global evolution of neuropsychological performances. Data processing included region of interest analysis of amyloid PET analysis, GM densities and tract-based spatial statistics (TBSS)-DTI. Regression models were built to explore the association between the CCS and imaging parameters controlling for significant demographic and clinical covariates.
Results: Amyloid uptake was not related to the cognitive outcome. In contrast, GM densities in bilateral hippocampus were associated with worst CCS at follow-up. In addition, radial and axial diffusivities in left hippocampus were negatively associated with CCS. Amyloid load was associated with decreased VBM and increased radial and axial diffusivity in the same area. These associations persisted when adjusting for gender and APOE4 genotype. Importantly, they were absent in amygdala and neocortical areas studied.
Conclusion: The progressive decrement of neuropsychological performances in normal aging is associated with volume loss and WM microstructure changes in hippocampus long before the emergence of clinically overt symptoms. Higher amyloid load in hippocampus is compatible with cognitive preservation in cases with better preservation of GM densities and WM microstructure in this area.
The current use of Alzheimer disease (AD) related amyloid/tau/neurodegeneration profile is made with the a priori idea that the first cognitive changes follows the elevation of brain amyloid and accumulation of neurofibrillary tangles (Soldan et al., 2019). In healthy individuals older than 80 years, the concomitant presence of positive biomarkers for amyloid, tau and neurodegeneration is the rule (Jack et al., 2017), still these cases perform within the normal range. However, cognitive trajectories in normal aging are variable ranging from stability over time, initial fluctuations and, in a limited number of cases, progressive worsening of neuropsychological performances that may take place long before the transition to the mild cognitive impairment (MCI) state. Although crucial for our understanding of the initial stages of neurodegeneration, the neuroimaging correlates of the individual trajectories within the normal range are poorly explored. The variability of clinical definitions before the appearance of the first cognitive deficits as well as limited follow-up period in this long asymptomatic phase are two major methodological problems facing this endeavor.
Among the different imaging technics that could be used to predict the cognitive trajectories in normal aging, amyloid positron emission tomography (PET) imaging, magnetic resonance imaging (MRI), and diffusion tensor imaging (DTI) assessing white matter (WM) and gray matter (GM) microstructure are likely to address at least partly temporally distinct processes (Bosch et al., 2012). Based on 1209 cognitively intact individuals aged 50–95, Jack et al. (2015) reported that hippocampal volume loss might occur before abnormal amyloid PET occurrence. Unlike hippocampal volume decrease that usually becomes significant after 60 years of age and is APOE4-independent, amyloid PET positivity is more frequent after 70 years of age with an increase of amyloid burden in the presence of APOE4 allele. These data indicated that Aß accumulation arises later in life on a background of preexisting structural deficits that are associated with normal aging and not with amyloid pathology per se (Jack et al., 2015). Early studies exploring the relationship between WM and amyloid have combined DTI and ex vivo histopathology. A positive relationship between the presence of amyloid deposits and WM microstructure abnormalities has been reported (Song et al., 2004; Sun et al., 2005; Shu et al., 2013; Zerbi et al., 2013; Racine et al., 2014; Wolf et al., 2015; Vipin et al., 2019). Importantly, the imaging parameters cited above are partly interdependent. The presence of hippocampal atrophy has been shown to impact on the association between DTI findings and amyloid positivity (Kantarci et al., 2014). Low amyloid burden is associated with higher FA values as a possible compensatory phenomenon at early stages of brain aging (Wolf et al., 2015).
Although within normal age-adjusted performances, elderly individuals with slowly declining cognitive abilities may exhibit a distinct pattern of amyloid deposition, volumetric and WM microstructure changes (Downer et al., 2017; Lin et al., 2017; Min et al., 2018). The present longitudinal study of a community-based cohort of 133 highly educated elderly controls focuses on their cognitive trajectories during a 4.5 year follow-up and explores their association with patterns of amyloid deposition, GM densities, and DTI markers and in this context. All of the cases were assessed with PET amyloid scans (for early amyloid burden), tract-based spatial statistics DTI (for WM microstructure), and GM densities (for brain atrophy) at inclusion and were classified according to their neuropsychological performances over the follow-up period.
Our main hypothesis is that cognitive fluctuations within the normal range are not innocuous and may be associated with significant GM and WM damage in key cortical areas for AD pathogenesis. We also hypothesized that amyloid load did not affect cognition in this highly selected series of healthy elders.
The study was approved by the local Ethics Committee and all participants gave written informed consent prior to inclusion. Individuals were selected from an ongoing cohort study on cognitively intact elders, as described in detail previously (Xekardaki et al., 2015; Zanchi et al., 2017; van der Thiel et al., 2018). Cases with three neurocognitive assessments at baseline, 18 months and 54 months, brain amyloid PET, structural brain MRI and DTI at inclusion and APOE status were considered. Exclusion criteria included psychiatric or neurologic disorders, sustained head injury, history of major medical disorders (neoplasm or cardiac illness), alcohol or drug abuse, regular use of neuroleptics, antidepressants or psychostimulants and contraindications to PET or MR imaging. To control for the confounding effect of vascular pathology on DTI findings, individuals with subtle cardiovascular symptoms, hypertension (non-treated), and a history of stroke or transient ischemic episodes were also excluded from the present study. The final sample included 133 elderly individuals (mean age 76.8 ± 4.0 years, 84 females).
At baseline, all individuals were evaluated with an extensive neuropsychological battery, including the mini-mental state examination (MMSE) (Folstein et al., 1975), the Hospital Anxiety and Depression Scale [HAD (Zigmond and Snaith, 1983], and the Lawton instrumental activities of daily living [IADL (Barberger-Gateau et al., 1992)]. Cognitive assessment included (a) attention (Digit-Symbol-Coding (Wechsler, 1997), Trail Making Test A (Reitan, 1958), (b) working memory [verbal: Digit Span Forward (Wechsler, 1955)], visuo-spatial: visual memory span (Corsi) (Milner, 1971), (c) episodic memory [verbal: RI-48 Cued Recall Test (Buschke et al., 1997)], visual: Shapes Test (Baddley et al., 1994), (d) executive functions [Trail Making Test B (Reitan, 1958), Wisconsin Card Sorting Test and Phonemic Verbal Fluency Test (Heaton, 1981)], (e) language [Boston Naming (Kaplan et al., 1983)], (f) visual gnosis (Ghent Overlapping Figures), (g) praxis: ideomotor (Schnider et al., 1997), reflexive (Poeck, 1985), and constructional [Consortium to Establish a Registry for Alzheimer’s Disease (CERAD), Figures copy (Welsh et al., 1994)]. All individuals were also evaluated with the Clinical Dementia Rating scale (CDR) (Hughes et al., 1982). In agreement with the criteria of Petersen et al. (Petersen et al., 2001), participants with a CDR of 0.5 but no dementia and a score exceeding 1.5 standard deviations below the age-appropriate mean in any of the cognitive tests were classified as MCI and were excluded. Participants with neither dementia nor MCI were classified as cognitively healthy controls and underwent full neuropsychological assessment at follow-ups, on average 18 and 54 months later.
The subtle cognitive decline was defined according to a continuous cognitive score (CCS), computed as follows. Most of the cognitive performances, discrete or continuous, cannot be linearly combined by adding the individual scores to a unique composite cognitive score. Thus, all values were converted to z scores. Subsequently, we summed the number of cognitive tests at follow-up with performances at least 0.5 standard deviation (SD) higher compared with the first evaluation, leading to the number of tests with improved performances (range, 0–14). Similarly, we summed the number of cognitive tests at follow-up with performances at least 0.5 SDs lower compared with the first evaluation, yielding the number of tests with decreased performances (range 0–14). Finally, the number of tests with improved minus the number of tests with decreased performances results in a final CCS. Change in cognition between inclusion and last follow-up was defined as the sum of the CCSs at two follow-ups.
Seventy-seven 18F-Florbetapir- (Amyvid) and fifty-six 18F-Flutemetanol-PET (Vizamyl) data were acquired on 2 different instruments (Siemens BiographTM mCT scanner and GE Healthcare Discovery PET/CT 710 scanner) of varying resolution and following different platform-specific acquisition protocols. The 18F-Florbetapir images were acquired 50–70 min after injection and the 18F-Flutemetanol images 90–120 min after injection. PET images were reconstructed using the parameters recommended by the ADNI protocol aimed at increasing data uniformity across the multicenter acquisitions. More information on the different imaging protocols for PET acquisition can be found on the ADNI web site1.
At baseline, imaging data were acquired on a 3T MRI scanner (TRIO SIEMENS Medical Systems, Erlangen, Germany). The structural high-resolution T1-weighted anatomical scan was performed with the following fundamental parameters: 256 × 256 matrix, 176 slices, 1 mm isotropic, TR = 2.27 ms). An additional DTI sequence was acquired (b = 0 and 30 diffusion directions with b = 1000 s/mm2, 128 × 128 matrix, voxel size 2.0 mm3 × 2.0 mm3 × 2.0 mm3, TE = 74.5 ms, TR = 15809 ms and 1 average). Additional sequences included axial fast spin-echo T2w imaging and susceptibility weighted imaging to exclude brain disease, such as ischemic stroke, subdural hematomas, or space-occupying lesions.
Whole blood samples were collected at baseline for all subjects for APOE genotyping. Standard DNA extraction was performed using either 9 ml EDTA tubes (Sarstedt, Germany) or Oragene Saliva DNA Kit (DNA Genotek, Inc., Ottawa, ON, Canada) which were stored at −20°C. APOE genotyping was done on the LightCycler (Roche Diagnostics, Basel, Switzerland) as described previously (Nauck et al., 2000). Subjects were classified according to the presence of an APOEε4 allele (ε4/ε3, ε3/ε3, and ε3/ε2 carrier).
All scans were intensity normalized using a modified Centiloid pons as reference region created by Lilja et al. (2018). The voxel-wise analysis was carried out using the FSL software package2, and the voxel-wise FSL General Linear Model was applied by using permutation-based non-parametric testing with the FSL Randomize Tool with the threshold-free cluster enhancement (TFCE) correction for multiple comparisons (Smith and Nichols, 2009) considering fully corrected p values <0.05 as significant. The analysis was performed across all participants across the entire brain using the CCS as explanatory variable, and age, gender, education, APOE status and tracer as non-explanatory variables.
Preprocessing of the DTI data was performed by using the standard procedure of TBSS, as described in detail before (Smith et al., 2006), in the FSL software package2. All subjects’ fractional anisotropy (FA) data were projected onto a mean FA skeleton using a non-linear spatial registration. The FA represents an index for the amount of diffusion asymmetry within a voxel. The tract skeleton is the basis for voxel-wise cross-subject statistics and reduces potential misregistrations as the source for false-positive or false-negative analysis results. The other DTI-derived parameters, axial diffusivity (the first eigenvalue, AR) and radial diffusivity (the average of the second and third eigenvalues, RD), were analyzed in the same way by using spatial transformation parameters that were estimated in the initial FA analysis.
We performed a ROI analysis on amyloid load and GM densities in 9 regions of particular interest in the context of cognitive decline (posterior cingulate cortex, mesial temporal lobe, parietal lobe, as well as hippocampus, amygdala, and caudate nucleus bilaterally) with the occipital lobe as control region. The ROI analysis was also performed on FA, AD, and RD values in all of the above mentioned areas.
Male and female participants’ characteristics were compared using Fisher exact, Mann. Whitney U or t tests as appropriate. The significance level was set at P < 0.05. Simple and multiple linear regression models were used to predict CCS (dependent variable) with amyloid load, GM densities and DTI markers (FA, AD, and RD) after including APOE epsilon 4 status (presence/absence), and gender as independent predictors. Only covariates that were significantly associated with the CCS in univariate models were included in multivariate models. A threshold of P value less than 0.05 was applied for significance. The correlation between amyloid values and TBSS-DTI, GM densities was assessed using Spearman rank correlation coefficient. The “Stata” software release 16.0 was used for all analyses.
The main demographic data is summarized in Table 1. Importantly, no case evolved to MCI during the follow-up period. Men were significantly more educated than women (p = 0.0013). The CCS (combined on the basis of two follow-ups) decreased in men but remained fairly stable in women (p = 0.038). The mean SUVr in bilateral hippocampus was below 0.8 in all of the cases implying a low level of amyloid deposition in these cognitively preserved cases.
Table 1. Gender-related differences in demographic and clinical data, radiological parameters, and APOE status.
There was no significant association between amyloid load and CCS in all of the areas studied. In contrast, there were significant positive associations between ROI measures of GM densities in hippocampus bilaterally and CCS Figure 1; left: regression coefficient: 22.2 (CI: 7.55, 36.87), p = 0.003; right: regression coefficient: 24.32 (CI: 8.98, 39.67), p = 0.002]. Results from TBSS-DTI analysis showed significant negative associations between both radial [regression coefficient: −4657 (CI: −8598, −715), p = 0.021] and axial diffusivity [regression coefficient: −4774 (CI: −8494, −1053), p = 0.012] in left hippocampus and CCS scores (Figure 2). FA analysis did not reveal significant associations with the cognitive outcome. Importantly, these associations persisted after adjustment for gender (the only demographic variable that was associated with imaging parameters in this sample) and APOE 4 genotype.
Figure 1. Scatterplots illustrating the association between GM densities and CCS together with best fit equations for left (A) and right (B) hippocampus.
Figure 2. Scatterplots illustrating the association between radial (A) and axial (B) diffusivity and CCS together with best fit equations for left hippocampus.
We observed a significant positive correlation between GM densities and amyloid load in both left (rs: 0.32, p = 0.0002) and right (rs: 0.27, p = 0.0018) hippocampus (Figures 3A,B). Moreover, a negative association was found between amyloid values and both radial (rs: −0.44, p = 0.0001) and axial diffusivity (rs: −0.43, p = 0.0001) in left hippocampus (Figures 3C,D).
Figure 3. Scatterplots illustrating the association between amyloid load and GM densities (A,B), radial (C) as well as axial (D) diffusivities for left (A,C,D), and right (B) hippocampus. Best fit equations are integrated in each plot.
Our findings reveal that the subtle changes in cognitive performances that occur during a long follow-up in normal aging are associated with decreased GM densities and altered WM microstructure in hippocampus. They also indicate that the low amyloid load observed in our community-based cohort of healthy elders has no direct impact on cognition and did not affect GM and WM integrity. In contrast to the majority of previous investigations, we did not include AD or MCI cases, but focused solely on cognitively preserved elderly individuals without significant vascular burden that remained within the normal range during the study period. Even the cases with the more marked decrement of their CCS with respect to their own baseline evaluation did not satisfy the MCI criteria even 4.5 years post-inclusion. Subsequently, our observations concern the initial stages of brain aging long before the emergence of clinically overt cognitive dysfunction. In this critical period, cognitive trajectories are still not fully determined by the impact of neurodegenerative changes and compensation abilities may be sufficiently active to counterbalance the deleterious effect of lesion accumulation.
Current evidences about the role of amyloid accumulation in these individuals remain ambiguous. Although several data suggested that elevated amyloid levels at baseline (SUVr > 1.5) was associated with greater cognitive decline at follow-up (Petersen et al., 2016), the INSIGHT-pre-AD data published recently showed no association between this parameter and cognitive fate at 30-month follow-up in healthy controls (Dubois et al., 2018). Another recent contribution indicated that PIB PET β-amyloid’s relationship to cognitive decline was non-linear being more prominent at lower β-amyloid levels (Knopman et al., 2018). Consistent with the INSIGHT-pre-AD data, amyloid load was not related to the cognitive outcome in the present series. One possible explanation for this finding may reside to the low levels of mean SUVr in our cases (less than 0.8 in hippocampus). It is thus likely that such small amyloid uptake is not sufficient for a direct deleterious effect on cognitive performances.
With respect to MRI data, there was a significant association between GM densities in both hippocampus and cognitive status in the present series. Data on GM evolution in normal aging remains ambiguous. Regional GM decrements in right thalamus, left parahippocampal gyrus, inferior temporo-parietal lobules, anterior cingulum, and precentral gyrus have been documented (Lee et al., 2016; Fletcher et al., 2018; Squarzoni et al., 2018) but in certain cohorts GM densities were preserved or marginally affected independently of the cognitive performances of healthy controls (Hong et al., 2016; Takeuchi et al., 2017). Unlike FA, the increase of AD and RD in left hippocampus reflects the presence of early axonal and myelin damage in asymptomatic cases with progressive decrement of cognitive performances prior to the MCI status. This finding supports the idea that early changes of diffusivity parameters in hippocampus may be more efficient than FA as a surrogate marker of cognitive dysfunction in non-demented individuals (Fellgiebel and Yakushev, 2011).
Whether amyloid load impacts on hippocampal volumes in cognitively preserved individuals is still matter of debate. Previous studies implied that this could be the case only in some vulnerable individuals (Nosheny et al., 2015; Khan et al., 2017; Duarte-Abritta et al., 2018). Equally ambiguous is the association between DTI parameters and amyloid load in normal aging. Some authors pointed to a RD increase with high amyloid uptake (Wolf et al., 2015) whereas others found compensatory increase of FA associated with impaired diffusion in amyloid positive cases (Racine et al., 2014). Negative data were also reported supporting an independent effect of DTI changes and amyloid deposits on cognitive decline in preclinical AD cases (Kantarci et al., 2014). Our correlation analysis showed that PET amyloid uptake was negatively related to both AD and RD in left hippocampus and positively associated with GM densities in hippocampus bilaterally. These results imply that higher amyloid load in hippocampus is compatible with cognitive preservation in cases with better preservation of GM densities and WM microstructure in this area. The absence of a direct toxic effect of amyloid on WM microstructure and GM densities supports the dissociation between neurodegeneration, WM microstructure changes and amyloid pathology in the initial phases of brain aging (Insel et al., 2015). In the same line, Wang et al., showed that elevated CSF tau in healthy elders is associated with cortical thinning but not the classical hippocampal atrophy. An inverse pattern was observed in cases with decreased CSF Aß42 implying the presence of a spatially distinct neurodegeneration related to Aß and tau pathology in preclinical AD (Wang et al., 2015). Analyzing 570 ADNI controls, Edmonds et al. (2015) reported that among the cases with only one abnormal biomarker at baseline that later progress to MCI, neurodegeneration alone was much more frequent than amyloidosis or subtle cognitive deficits which were equally common. Besson et al. (2015) postulated that most preclinical AD cases have either neurodegeneration or Aß deposition but not both. These observations point to the necessity to add imaging modalities such as tau imaging and fluorodeoxyglucose PET in order to assess the complex relationships between GM densities/DTI parameters and the development of AD pathology in normal brain aging.
Strengths of the present study includes the combination of three imaging modalities in the initial stages of normal brain aging (amyloid PET, GM morphometry, DTI), longitudinal assessment of neuropsychological performances using a detailed battery, and exclusion of cases with cardiovascular risk factors or significant vascular pathologies that could affect DTI data. Two limitations should, however, be taken into account. We report here the results of a cohort of highly educated elders without significant vascular burden that is clearly not representative of the whole spectrum of normal aging. The paucity of DTI alterations and in particular FA decrease, contrasts with previous reports in brain aging (for review see Squarzoni et al., 2018) and may reflect a selection bias and should thus be interpreted with caution. Finally, although our follow-up lasted for 4.5 years, we cannot exclude that early amyloid burden impacts on DTI-assessed longitudinal WM changes at later time points (Vipin et al., 2019).
Our data shed new light into the complex association between neuroimaging markers and cognitive trajectories in normal brain aging. Although not yet considered pathological, the continuous decrement of cognitive performances in this community-based cohort is associated with substantial changes in morphometry and WM diffusivity parameters in hippocampus. The low amyloid load in these cases did not affect cognition. Its association with higher GM densities and lower RD and AD values in hippocampus support the dissociation between amyloid pathology and neurodegeneration in normal brain aging.
The raw data supporting the conclusions of this manuscript will be made available by the authors, without undue reservation, to any qualified researcher.
The studies involving human participants were reviewed and approved by the Commission Cantonale d’Ethique de la Recherche (CCER) Geneva. The patients/participants provided their written informed consent to participate in this study.
SH, M-LM, JL, and FH performed and analyzed the data. SH, PG, and M-LM wrote the manuscript. CR and VG were involved in participants recruitment, follow-up, and data acquisition. All authors read and approved the final manuscript.
This research was carried out with help of an unrestricted grant from the Association Suisse pour la Recherche sur Alzheimer, a unrestricted grant from the Schmidheiny Foundation, and the Swiss National Foundation grant (Grant No. 320030-169390).
The authors declare that the research was conducted in the absence of any commercial or financial relationships that could be construed as a potential conflict of interest.
Baddley, A., Emslie, H., and Nimmo-Smith, I. (1994). A Test of Visual and Verbal Recall and Recognition. Bury St. Edmunds: Thames Valley Test Company.
Barberger-Gateau, P., Commenges, D., Gagnon, M., Letenneur, L., Sauvel, C., and Dartigues, J. F. (1992). Instrumental activities of daily living as a screening tool for cognitive impairment and dementia in elderly community dwellers. J. Am. Geriatr. Soc. 40, 1129–1134. doi: 10.1111/j.1532-5415.1992.tb01802.x
Besson, F. L., La Joie, R., Doeuvre, L., Gaubert, M., Mézenge, F., Egret, S., et al. (2015). Cognitive and brain profiles associated with current neuroimaging biomarkers of preclinical alzheimer’s disease. J. Neurosci. 35, 10402–10411. doi: 10.1523/jneurosci.0150-15.2015
Bosch, B., Arenaza-Urquijo, E. M., Rami, L., Sala-Llonch, R., Junqué, C., Solé-Padullés, C., et al. (2012). Multiple DTI index analysis in normal aging, amnestic MCI and AD. Relationship with neuropsychological performance. Neurobiol. Aging 33, 61–74. doi: 10.1016/j.neurobiolaging.2010.02.004
Buschke, H., Sliwinski, M. J., Kuslansky, G., and Lipton, R. B. (1997). Diagnosis of early dementia by the double memory test: encoding specificity improves diagnostic sensitivity and specificity. Neurology 48, 989–997.
Downer, B., Chen, N. W., Raji, M., and Markides, K. S. (2017). A longitudinal study of cognitive trajectories in mexican americans age 75 and older. Int. J. Geriatr. Psychiatry 32, 1122–1130. doi: 10.1002/gps.4575
Duarte-Abritta, B., Villarreal, M. F., Abulafia, C., Loewenstein, D., Curiel Cid, R. E., Castro, M. N., et al. (2018). Cortical thickness, brain metabolic activity, and in vivo amyloid deposition in asymptomatic, middle-aged offspring of patients with late-onset alzheimer’s disease. J. Psychiatr. Res. 107, 11–18. doi: 10.1016/j.jpsychires.2018.10.008
Dubois, B., Epelbaum, S., Nyasse, F., Bakardjian, H., Gagliardi, G., Uspenskaya, O., et al. (2018). Cognitive and neuroimaging features and brain β-amyloidosis in individuals at risk of alzheimer’s disease (insight-pread): a longitudinal observational study. Lancet Neurol. 17, 335–346. doi: 10.1016/s1474-4422(18)30029-2
Edmonds, E. C., Delano-Wood, L., Galasko, D. R., Salmon, D. P., Bondi, M. W. and Disease Neuroimaging Initiative Alzheimer’, (2015). Subtle cognitive decline and biomarker staging in preclinical alzheimer’s disease. J. Alzheimers Dis. 47, 231–242. doi: 10.3233/jad-150128
Fellgiebel, A., and Yakushev, I. (2011). Diffusion tensor imaging of the hippocampus in mci and early alzheimer’s disease. J. Alzheimers Dis. 26(Suppl. 3), 257–262. doi: 10.3233/JAD-2011-0001
Fletcher, E., Gavett, B., Harvey, D., Farias, S. T., Olichney, J., Beckett, L., et al. (2018). Brain volume change and cognitive trajectories in aging. Neuropsychology 32, 436–449. doi: 10.1037/neu0000447
Folstein, M. F., Folstein, S. E., and McHugh, P. R. (1975). Mini-mental state. A practical method for grading the cognitive state of patients for the clinician. J. Psychiatr. Res. 12, 189–198.
Heaton, R. K. (1981). Wisconsing Card Sorting Test Manual. Odessa: Psychological Assessment Resources Inc.
Hong, Y. J., Kim, C. M., Jang, E. H., Hwang, J., Roh, J. H., and Lee, J. H. (2016). White matter changes may precede gray matter loss in elderly with subjective memory impairment. Dement. Geriatr. Cogn. Disord. 42, 227–235. doi: 10.1159/000450749
Hughes, C. P., Berg, L., Danziger, W. L., Coben, L. A., and Martin, R. L. (1982). A new clinical scale for the staging of dementia. Br. J. Psychiatry 140, 566–572. doi: 10.1192/bjp.140.6.566
Insel, P. S., Mattsson, N., Donohue, M. C., Mackin, R. S., Aisen, P. S., Jack, C. R., et al. (2015). The Transitional Association Between B -amyloid pathology and regional brain atrophy. Alzheimers Dement. 11, 1171–1179. doi: 10.1016/j.jalz.2014.11.002
Jack, C. R., Wiste, H. J., Weigand, S. D., Knopman, D. S., Vemuri, P., Mielke, M. M., et al. (2015). Age, sex, and apoe E 4 effects on memory, brain structure, and β-amyloid across the adult life span. JAMA Neurol. 72, 511–519. doi: 10.1001/jamaneurol.2014.4821
Jack, C. R., Wiste, H. J., Weigand, S. D., Therneau, T. M., Knopman, D. S., Lowe, V., et al. (2017). Age-specific and sex-specific prevalence of cerebral B -amyloidosis, tauopathy, and neurodegeneration in cognitively unimpaired individuals aged 50-95 years: a cross-sectional study. Lancet Neurol. 16, 435–444. doi: 10.1016/S1474-4422(17)30077-7
Kantarci, K., Schwarz, C. G., Reid, R. I., Przybelski, S. A., Lesnick, T. G., Zuk, S. M., et al. (2014). White matter integrity determined with diffusion tensor imaging in older adults without dementia: influence of amyloid load and neurodegeneration. JAMA Neurol. 71, 1547–1554. doi: 10.1001/jamaneurol.2014.1482
Kaplan, E. F., Goodglass, H., and Weintraub, S. (1983). The Boston Naming Test, 2nd Edn, Philadelphia: Lea & Febiger.
Khan, W., Giampietro, V., Banaschewski, T., Barker, G. J., Bokde, A. L., Büchel, C., et al. (2017). A multi-cohort study of apoe ε4 and amyloid-B effects on the hippocampus in alzheimer’s disease. J. Alzheimers Dis. 56, 1159–1174. doi: 10.3233/JAD-161097
Knopman, D. S., Lundt, E. S., Therneau, T. M., Vemuri, P., Lowe, V. J., Kantarci, K., et al. (2018). Joint associations of B -amyloidosis and cortical thickness with cognition. Neurobiol. Aging 65, 121–131. doi: 10.1016/j.neurobiolaging.2018.01.017
Lee, Y. M., Ha, J. K., Park, J. M., Lee, B. D., Moon, E., Chung, Y. I., et al. (2016). Impact of apolipoprotein E4 polymorphism on the gray matter volume and the white matter integrity in subjective memory impairment without white matter hyperintensities: voxel-based morphometry and tract-based spatial statistics study under 3-tesla mri. J. Neuroimaging 26, 144–149. doi: 10.1111/jon.12207
Lilja, J., Leuzy, A., Chiotis, K., Savitcheva, I., Sörensen, J., and Nordberg, A. (2018). Spatial normalization of 18F-flutemetamol PET images using an adaptive principal-component template. J. Nucl. Med. 60, 285–291. doi: 10.2967/jnumed.118.207811
Lin, F. V., Wang, X., Wu, R., Rebok, G. W., Chapman, B. P. and Disease Neuroimaging Initiative Alzheimer’, (2017). Identification of successful cognitive aging in the alzheimer’s disease neuroimaging initiative study. J. Alzheimers Dis. 59, 101–111. doi: 10.3233/jad-161278
Milner, B. (1971). Interhemispheric differences in the localization of psychological processes in man. Br. Med. Bull. 27, 272–277. doi: 10.1093/oxfordjournals.bmb.a070866
Min, M., Shi, T., Sun, C., Liang, M., Zhang, Y., Wu, Y., et al. (2018). The association between orthostatic hypotension and dementia: a meta-analysis of prospective cohort studies. Int. J. Geriatr. Psychiatry 33, 1541–1547. doi: 10.1002/gps.4964
Nauck, M., Hoffmann, M. M., Wieland, H., and März, W. (2000). Evaluation of the apo E genotyping kit on the lightcycler. Clin. Chem. 46, 722–724.
Nosheny, R. L., Insel, P. S., Truran, D., Schuff, N., Jack, C. R., Aisen, P. S., et al. (2015). Variables associated with hippocampal atrophy rate in normal aging and mild cognitive impairment. Neurobiol. Aging 36, 273–282. doi: 10.1016/j.neurobiolaging.2014.07.036
Petersen, R. C., Doody, R., Kurz, A., Mohs, R. C., Morris, J. C., Rabins, P. V., et al. (2001). Current concepts in mild cognitive impairment. Arch. Neurol. 58, 1985–1992.
Petersen, R. C., Wiste, H. J., Weigand, S. D., Rocca, W. A., Roberts, R. O., Mielke, M. M., et al. (2016). Association of elevated amyloid levels with cognition and biomarkers in cognitively normal people from the community. JAMA Neurol. 73, 85–92. doi: 10.1001/jamaneurol.2015.3098
Poeck, K. (1985). “Clues to the nature of disruption to limb praxis,” in Neuropsychological Studies of Apraxia and Related Disorders, ed. E. A. Roy, (Amsterdam: North-Holland), 99–109. doi: 10.1016/s0166-4115(08)61138-3
Racine, A. M., Adluru, N., Alexander, A. L., Christian, B. T., Okonkwo, O. C., Oh, J., et al. (2014). Associations between white matter microstructure and amyloid burden in preclinical alzheimer’s disease: a multimodal imaging investigation. Neuroimage Clin. 4, 604–614. doi: 10.1016/j.nicl.2014.02.001
Reitan, R. M. (1958). Validity of the trail making test as an indicator of organic brain damage. Percept. Mot. Skills 8, 271–276. doi: 10.2466/pms.1958.8.3.271
Schnider, A., Hanlon, R. E., Alexander, D. N., and Benson, D. F. (1997). Ideomotor apraxia: behavioral dimensions and neuroanatomical basis. Brain Lang. 58, 125–136. doi: 10.1006/brln.1997.1770
Shu, X., Qin, Y. Y., Zhang, S., Jiang, J. J., Zhang, Y., Zhao, L. Y., et al. (2013). Voxel-based diffusion tensor imaging of an app/ps1 mouse model of alzheimer’s disease. Mol. Neurobiol. 48, 78–83. doi: 10.1007/s12035-013-8418-6
Smith, S. M., Jenkinson, M., Johansen-Berg, H., Rueckert, D., Nichols, T. E., Mackay, C. E., et al. (2006). Tract-based spatial statistics: voxelwise analysis of multi-subject diffusion data. Neuroimage 31, 1487–1505. doi: 10.1016/j.neuroimage.2006.02.024
Smith, S. M., and Nichols, T. E. (2009). Threshold-free cluster enhancement: addressing problems of smoothing, threshold dependence and localisation in cluster inference. Neuroimage 44, 83–98. doi: 10.1016/j.neuroimage.2008.03.061
Soldan, A., Pettigrew, C., Fagan, A. M., Schindler, S. E., Moghekar, A., Fowler, C., et al. (2019). Atn profiles among cognitively normal individuals and longitudinal cognitive outcomes. Neurology 92, e1567–e1579. doi: 10.1212/WNL.0000000000007248
Song, S. K., Kim, J. H., Lin, S. J., Brendza, R. P., and Holtzman, D. M. (2004). Diffusion tensor imaging detects age-dependent white matter changes in a transgenic mouse model with amyloid deposition. Neurobiol. Dis. 15, 640–647. doi: 10.1016/j.nbd.2003.12.003
Squarzoni, P., Duran, F. L. S., Busatto, G. F., and Alves, T. C. T. F. (2018). Reduced gray matter volume of the thalamus and hippocampal region in elderly healthy adults with no impact of apoe ε4: a longitudinal voxel-based morphometry study. J. Alzheimers Dis. 62, 757–771. doi: 10.3233/jad-161036
Sun, S. W., Song, SK, Harms, M. P., Lin, Sj, Holtzman, Dm, Merchant, KM, and Kotyk, J. J. (2005). Detection of age-dependent brain injury in a mouse model of brain amyloidosis associated with alzheimer’s disease using magnetic resonance diffusion tensor imaging. Exp. Neurol. 191, 77–85. doi: 10.1016/j.expneurol.2004.09.006
Takeuchi, H., Taki, Y., Nouchi, R., Yokoyama, R., Kotozaki, Y., Nakagawa, S., et al. (2017). Global associations between regional gray matter volume and diverse complex cognitive functions: evidence from a large sample study. Sci. Rep. 7:10014. doi: 10.1038/s41598-017-10104-8
van der Thiel, M., Rodriguez, C., Giannakopoulos, P., Burke, M. X., Lebel, R. M., Gninenko, N., et al. (2018). Brain perfusion measurements using multidelay arterial spin-labeling are systematically biased by the number of delays. Am. J. Neuroradiol. 39, 1432–1438. doi: 10.3174/ajnr.A5717
Vipin, A., Ng, K. K., Ji, F., Shim, H. Y., Lim, J. K. W., Pasternak, O., et al. (2019). Amyloid burden accelerates white matter degradation in cognitively normal elderly individuals. Hum. Brain Mapp. 40, 2065–2075. doi: 10.1002/hbm.24507
Wang, L., Benzinger, T. L., Hassenstab, J., Blazey, T., Owen, C., Liu, J., et al. (2015). Spatially distinct atrophy is linked to β-amyloid and tau in preclinical alzheimer disease. Neurology 84, 1254–1260. doi: 10.1212/WNL.0000000000001401
Wechsler, D. (1955). Manual for the Wechsler Adult Intelligence Scale. New York, NY: Psychological Corporation.
Welsh, K. A., Butters, N., Mohs, R. C., Beekly, D., Edland, S., Fillenbaum, G., et al. (1994). The consortium to establish a registry for alzheimer’s disease (Cerad). part v. a normative study of the neuropsychological battery. Neurology 44, 609–614.
Wolf, D., Fischer, F. U., Scheurich, A., Fellgiebel, A. and Disease Neuroimaging Initiative Alzheimer’, (2015). Non-linear association between cerebral amyloid deposition and white matter microstructure in cognitively healthy older adults. J. Alzheimers Dis. 47, 117–127. doi: 10.3233/JAD-150049
Xekardaki, A., Rodriguez, C., Montandon, M. L., Toma, S., Tombeur, E., Herrmann, F. R., et al. (2015). Arterial spin labeling may contribute to the prediction of cognitive deterioration in healthy elderly individuals. Radiology 274, 490–499. doi: 10.1148/radiol.14140680
Zanchi, D., Giannakopoulos, P., Borgwardt, S., Rodriguez, C., and Haller, S. (2017). Hippocampal and amygdala gray matter loss in elderly controls with subtle cognitive decline. Front. Aging Neurosci. 9:50. doi: 10.3389/fnagi.2017.00050
Zerbi, V., Kleinnijenhuis, M., Fang, X., Jansen, D., Veltien, A., Van Asten, J., et al. (2013). Gray and white matter degeneration revealed by diffusion in an alzheimer mouse model. Neurobiol. Aging 34, 1440–1450. doi: 10.1016/j.neurobiolaging.2012.11.017
Keywords: amyloid deposition, APOE genotyping, magnetic resonance imaging, normal aging, positron emission tomography
Citation: Haller S, Montandon M-L, Rodriguez C, Garibotto V, Lilja J, Herrmann FR and Giannakopoulos P (2019) Amyloid Load, Hippocampal Volume Loss, and Diffusion Tensor Imaging Changes in Early Phases of Brain Aging. Front. Neurosci. 13:1228. doi: 10.3389/fnins.2019.01228
Received: 14 June 2019; Accepted: 30 October 2019;
Published: 15 November 2019.
Edited by:
Gianfranco Spalletta, Santa Lucia Foundation (IRCCS), ItalyReviewed by:
Fabrizio Piras, Santa Lucia Foundation (IRCCS), ItalyCopyright © 2019 Haller, Montandon, Rodriguez, Garibotto, Lilja, Herrmann and Giannakopoulos. This is an open-access article distributed under the terms of the Creative Commons Attribution License (CC BY). The use, distribution or reproduction in other forums is permitted, provided the original author(s) and the copyright owner(s) are credited and that the original publication in this journal is cited, in accordance with accepted academic practice. No use, distribution or reproduction is permitted which does not comply with these terms.
*Correspondence: Sven Haller, c3Zlbi5oYWxsZXJAbWUuY29t
Disclaimer: All claims expressed in this article are solely those of the authors and do not necessarily represent those of their affiliated organizations, or those of the publisher, the editors and the reviewers. Any product that may be evaluated in this article or claim that may be made by its manufacturer is not guaranteed or endorsed by the publisher.
Research integrity at Frontiers
Learn more about the work of our research integrity team to safeguard the quality of each article we publish.