Corrigendum: Alterations of the Gut Microbiota in Multiple System Atrophy Patients
- 1Department of Neurology, Xiangya Hospital, Central South University, Changsha, China
- 2National Clinical Research Center for Geriatric Disorders, Central South University, Changsha, China
- 3Key Laboratory of Hunan Province in Neurodegenerative Disorders, Central South University, Changsha, China
- 4Laboratory of Medical Genetics, Central South University, Changsha, China
- 5Department of Neurology, Xinjiang Medical University, Urumchi, China
Multiple system atrophy (MSA) is a fatal neurodegenerative disease, and the pathogenesis is still quite challenging. Emerging evidence has shown that the brain–gut–microbiota axis served a pivotal role in neurological diseases; however, researches utilizing metagenomic sequencing to analyze the alteration in gut microbiota of MSA patients were quite rare. Here, we carried out metagenomic sequencing in feces of 15 MSA patients and 15 healthy controls, to characterize the alterations in gut microbial composition and function of MSA patients in mainland China. The results showed that gut microbial community of MSA patients was significantly different from healthy controls, characterized by increased genus Akkermansia and species Roseburia hominis, Akkermansia muciniphila, Alistipes onderdonkii, Streptococcus parasanguinis, and Staphylococcus xylosus, while decreased genera Megamonas, Bifidobacterium, Blautia, and Aggregatibacter and species Bacteroides coprocola, Megamonas funiformis, Bifidobacterium pseudocatenulatum, Clostridium nexile, Bacteroides plebeius, and Granulicatella adiacens. Further, functional analysis based on the KEGG database revealed aberrant functional pathways in fecal microbiome of MSA patients. In conclusion, our findings provided evidence for dysbiosis in gut microbiota of Chinese MSA cohorts and helped develop new testable hypotheses on pathophysiology of MSA.
Introduction
Multiple system atrophy (MSA) is a sporadic, adult-onset, progressive neurodegenerative disease, characterized by diverse combinations of parkinsonian features, cerebellar ataxia, autonomic failure, and pyramidal features. Clinically, MSA is divided into two subtypes: MSA with predominant parkinsonism (MSA-P) and MSA with predominant cerebellar ataxia (MSA-C) (Fanciulli and Wenning, 2015). The typical neuropathology of MSA is oligodendroglial cytoplasmic inclusions (GCIs), mainly composed of α-synuclein (Papp et al., 1989; Gai et al., 1998). Till now, environmental factors (such as nicotine use and alcohol consumption) and genetic factors (such as mutations in COQ2, SHC2, and SNCA) are thought to contribute to the risk of MSA together. However, the explicit etiology and pathogenic mechanisms remain unclear (Fanciulli and Wenning, 2015). Due to the variable manifestations, the diagnostic accuracy of MSA remains a challenge. The definite diagnosis of MSA requires the neuropathologic evidence of widespread α-synuclein-positive glial cytoplasmic inclusions along with olivopontocerebellar atrophy or striatonigral degeneration in postmortem examination (Trojanowski and Revesz, 2007). MSA is a rare severe neurodegenerative disease with 6- to 10-year mean survival time from symptom onset; however, there is no cured therapy yet and only symptomatic treatment is available, which is also quite disappointing (Flabeau et al., 2010; Fanciulli and Wenning, 2015).
Microbiota, defined as the entirety of microorganisms in a specific habitat, is gaining increasing importance (Whiteside et al., 2015). It has been shown to serve pivotal roles in numerous diseases, such as obesity, cardiovascular diseases, dermatosis, hepatic diseases, and osteoarticular diseases (Collins et al., 2015; Li et al., 2016, 2017; Liu et al., 2017; Tan L. et al., 2018; Zhou et al., 2019). The brain–gut–microbiota axis has put forward a new promising direction for neuroscience. The gut microbiota could interact with brain through immune, neural, and neuroendocrine pathways to regulate brain development, function, and behavior (Dinan and Cryan, 2017). Therefore, microbiota may serve pivotal roles in pathogenesis of neurological diseases, which has been proven in Parkinson’s disease, myasthenia gravis, multiple sclerosis, etc. (Keshavarzian et al., 2015; Jangi et al., 2016; Qiu et al., 2018). As for MSA, intestinal inflammation has been suggested to be related with MSA pathogenesis (Cao et al., 2018); thus, the gut microbiota may also participate in pathogenesis of MSA. Engen et al. (2017) discovered the alteration of gut microbiota in American MSA patients through 16SrDNA sequencing in feces and sigmoid mucosa. Tan et al. used 16SrDNA sequencing, revealing that the fecal microbiota was different between MSA patients and healthy persons in ethnic Chinese of Malaysia (Tan A.H. et al., 2018). The studies focusing on the change in gut microbiota of MSA patients were quite rare.
Traditionally, 16SrDNA sequencing is the main approach for investigating the microbiota based on amplifying and sequencing the hypervariable loci in 16SrDNA gene of bacteria (18SrDNA for fungi). This method requires low cost and input template DNA concentrations; however, it cannot characterize bacteria to their species/strain levels and is limited when used in analyzing the functions of the microbiota. Metagenomics is defined as sequencing the entire DNA (or RNA) content of microbiota in a sample through high-throughput techniques. Metagenomics, besides identifying microbial taxa to their species/strain levels, possesses more superiority in analyzing the function of genes, the structure and organization of genomes, community structure and evolutionary relationships within the sample, and identification of new genes and biocatalysts (Cao et al., 2017; Martinez et al., 2017; Roumpeka et al., 2017). However, there were no researches utilizing metagenomic sequencing to analyze the relationships between gut microbiota and MSA pathogenesis yet. Here, we carried out metagenomic sequencing to compare the microbiota community in feces of MSA patients to healthy controls of mainland China and further explore the key alterations of functional pathways in MSA patients’ gut microbiota.
Materials and Methods
Subjects
Multiple system atrophy patients (n = 15; 8 males, 7 females; mean age 56.7 ± 8.4 years) were recruited from the Department of Neurology of Xiangya Hospital; meanwhile, healthy control volunteers (n = 15; 10 males, 5 females; mean age 53.8 ± 7.5 years) were recruited from the Physical Examination Center of Xiangya Hospital. The study was approved by the Ethics Committee of Xiangya Hospital of Central South University in China. All subjects gave written informed consent in accordance with the Declaration of Helsinki.
Multiple system atrophy patients were diagnosed by at least two neurologists according to the second consensus statement of diagnostic criteria for MSA (Gilman et al., 2008). SCA (spinocerebellar ataxia) 1, 2, 3, 6, 7, and 17 and DRPLA (dentatorubral-pallidoluysian atrophy) were genetically excluded. The inclusion criteria of healthy control group subjects were as follows: (1) age, sex, and BMI were matched to MSA groups; (2) no constipation and diarrhea; and (3) no neurological diseases. Exclusion criteria for both group subjects were as follows: (1) the use of antibiotics, glucocorticoids, probiotics, and immunosuppressants within the 2 months before the sample collection; (2) hypertension, diabetes, obesity, and metabolic syndrome; and (3) gastrointestinal diseases and autoimmune diseases. Besides, both group subjects were from the Han nationality in central south area of China.
Clinical information of all subjects was collected through face-to-face interviews between subjects and neurological specialists. All subjects finished a food frequency questionnaire (FFQ) through recalling the dietary frequency in the period of 1 year before the interview. Besides, we especially recorded the intake of the fish oil in the dietary supplement, since the unsaturated fatty acid could affect the gut microbiota composition (Zmora et al., 2019). In addition, we calculated the dietary diversity score (DDS) of each subject based on the FFQ results. According to the Chinese Dietary Guidelines, all food items were categorized into nine groups: grains, vegetables, fruit, meat, fish, eggs, beans, dairy, and oil (Zhang et al., 2017). If the participant ate any food from these nine categories at least once a week, one point was given in that category. Otherwise, the score was zero. Consuming different food in the same category would not count repeatedly. The DDS was equal to the sum points of the nine categories. The symptoms of MSA subjects were assessed through the Unified Multiple System Atrophy Rating Scale (UMSARS) and the cognitive status was evaluated by the Mini Mental State Examination (MMSE). The constipation of all subjects was assessed by the Wexner constipation score.
Sample Collection and DNA Extraction
Each MSA patient and healthy subject provided a fresh stool sample in the morning using a fecal collection container, which was immediately transported on ice to the laboratory and preserved at −80°C. QIAamp Fast DNA Stool Mini Kit (Qiagen, Germany) was used to extract DNA from fecal samples. The DNA integrity and concentration were tested by 1% agarose gel electrophoresis (AGE).
Metagenomic Sequencing
DNA Library Construction and Sequencing
The metagenomic libraries were constructed by using KAPA Hyper Prep Kit (Kapa Biosystems, United States) according to manufacturer’s instructions, with an average of 350 bp insert size. The quality was assessed on a Qubit@ 2.0 Fluorometer (Thermo Scientific, United States) and the Agilent Bioanalyzer 2100 system (Agilent, United States). Sequencing was performed on an Illumina HiSeq2500 platform.
Bioinformatics and Statistical Analysis
Reads that mapped to human genome (hg19) were removed from raw reads and then low-quality reads plus reads with adaptors were filtrated through Trimmomatic software (version 0.38). The quality of Illumina raw reads and clean reads was analyzed by using FastQC software (version 0.11.8). Species taxonomic assignment was achieved through aligning clean reads to clade-specific marker genes available on NCBI using MetaPhlAn2 software (version 2.0). Partial least square-discriminant analysis (PLS-DA) was performed through R software (version 3.4.1). LDA effect size (LEfSe) analysis was utilized to identify bacterial taxa with significantly different abundance between MSA and healthy groups based on LEfSe software (version 1.0). The alpha cutoff was set to 0.05 and effect size cutoff was set to 2.0. Because LEfSe required all the pairwise comparison to reject the null hypothesis to detect a biomarker, no multiple testing corrections were needed (Segata et al., 2011). Differential abundance analysis between MSA-C and MSA-P subtypes was performed through the Welch’s t-test and p-values were corrected through Storey’s false discovery rate (FDR) control. An FDR q value < 0.05 was considered statistically significant.
De novo assembly was carried out through Megahit software (version 0.3.0) to obtain contigs (>500 bp) with five k-mer parameters 21, 41, 61, 81, and 99. We use Prodigal software (version 2.6.3) to perform gene (open reading frame, ORF) prediction on contigs from each sample (genes shorter than 100 bp were removed) and translation to protein sequences. Then CD-HIT software (version 4.6.8) was utilized to cluster predicted genes and redundant sequences were removed based on 95% identity and 90% coverage. The longest sequence of each cluster was selected to construct non-redundant gene catalog and the final non-redundant gene catalog contains 1,925,921 microbial genes. Next, we utilized Kallisto software (version 0.44.0) to calculate gene reads counts and perform normalization on reads counts to obtain relative gene abundance through the TMM method implemented in edgeR software (version 3.14.0). Functional annotations were carried out through DIAMOND software (version 0.8.22) against the KEGG database (Kanehisa et al., 2004) and dbCAN database (Yin et al., 2012) (e value≤1e−5). Then, we calculated the abundance of each functional feature by summing the abundance of genes annotated to this feature. For functional features with an abundance median≥1e−8 in both groups, differential abundance analysis was performed using the Welch’s t-test in STAMP software (version 2.1.3). p-values were corrected through Storey’s FDR method and we applied a cutoff of FDR q value < 0.1 to indicate results remaining noteworthy. Demographic and clinical characteristics were compared with Student’s t-test, Fisher exact test, and Mann–Whitney U test for quantitative and categorical variables, based on the SPSS software (version 19.0).
Results
The Basic Characteristics of Subjects
The demographic and clinical characteristics of subjects are summarized in Table 1. In total, 15 MSA patients and 15 healthy controls were recruited in bioinformatic analysis. There was no difference observed in age, gender, and BMI between two groups. As for the diet pattern, no significant difference was detected in all food category consumption and dietary diversity scores. No subject consumed the fish oil as the dietary supplement (Supplementary Table S1). MSA patients including 11 MSA-C subtypes and 4 MSA-P subtypes had an average disease duration of 2.3 ± 0.8 years, mean UMSARS I scores of 19.7 ± 5.2, mean UMSARS II scores of 21.2 ± 6.9, mean UMSARS IV scores of 3.6 ± 0.7, and average MMSE scores of 26.3 ± 2.4. Besides, MSA patients had higher Wexner constipation scores than healthy controls (7.3 vs. 1.2, p-value < 0.001).
Taxonomy Annotation and Microbiota Composition
Metagenomic sequencing resulted in an average of 37.28 ± 3.56 million clean reads each sample. The specific quality of clean reads in each sample was shown in Supplementary Table S2. After taxonomy annotation based on NCBI, we summarized the microbiota composition of each sample on phylum (top 10) and genus (top 20) levels in Figure 1. In the stacked bar chart, each bar represented different bacterial taxon and the length represented the relative abundance. The results showed that Firmicutes, Bacteroidetes, and Proteobacteria were dominant phyla in both MSA and healthy groups. The samples were then analyzed with PLS-DA on the genus level. The score plots revealed a clear discrimination of microbial profile between MSA and healthy control groups (Figure 2), which reflected the alterations in MSA patients’ gut microbial community.
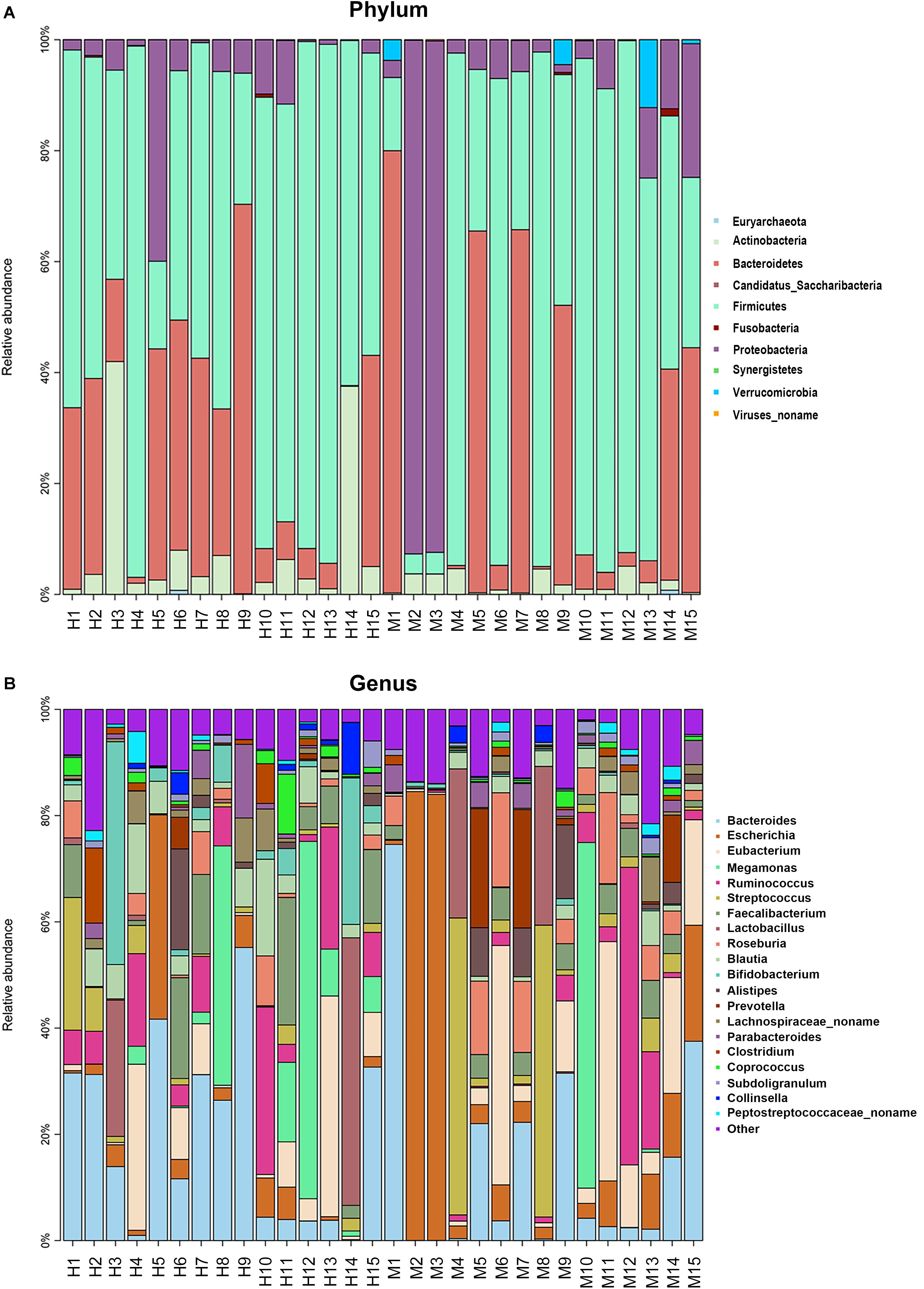
Figure 1. The microbiota composition of each sample. Each bar represented different bacterial taxon and the length represented the relative abundance. (A) The relative abundance of top 10 taxa on phylum level. (B) The relative abundance of top 20 taxa on genus level. H, healthy group; M, MSA group.
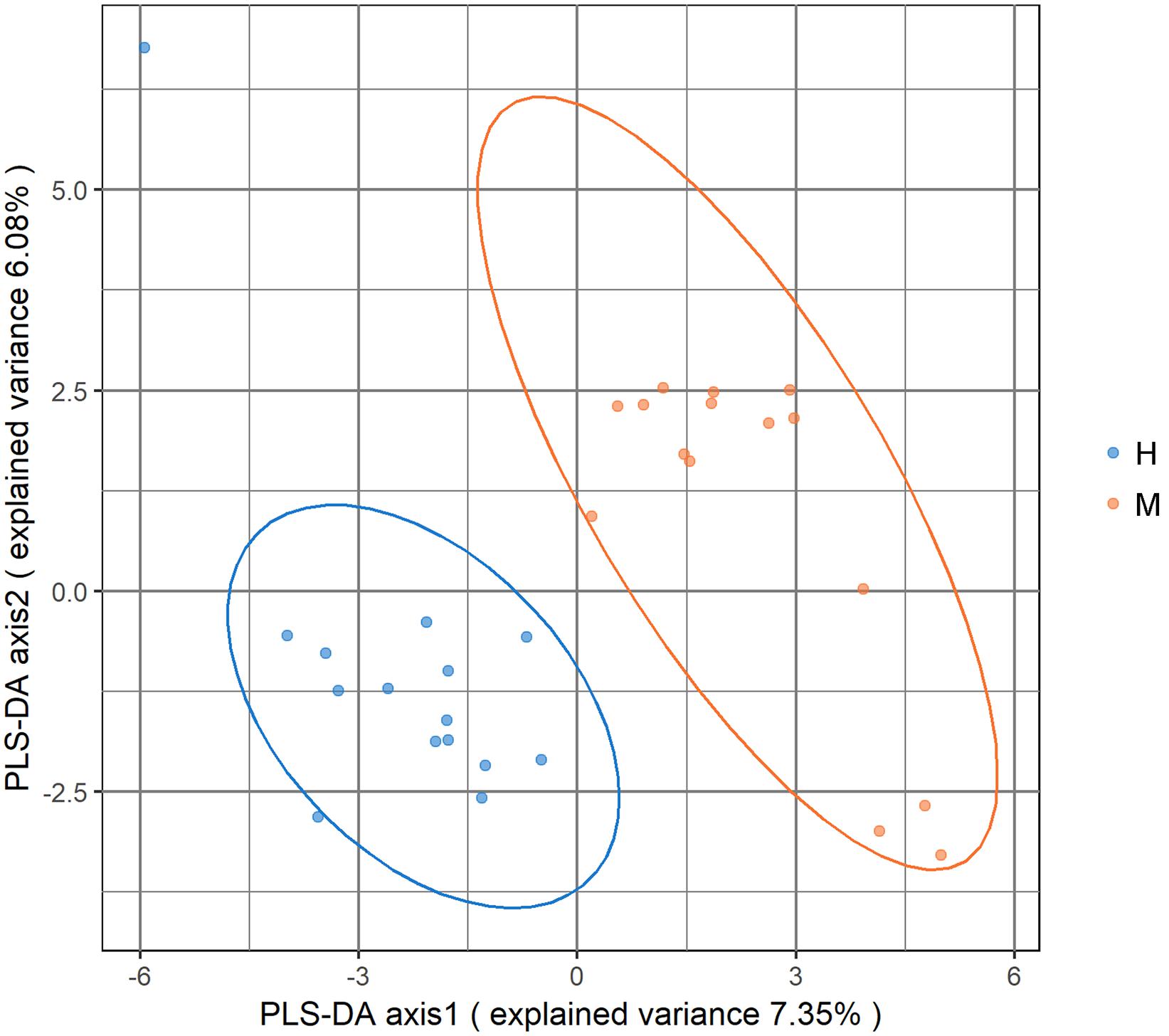
Figure 2. Score plot of partial least square-discriminant analysis (PLS-DA) showed that the MSA and healthy groups were separated into two clusters. Each dot represented one sample.
Differential Microbial Taxa Analysis
We utilized the LEfSe method to elucidate which taxa were driving divergence between MSA and healthy groups. We set the LDA score cutoff as 2.0 to distinguish the significant bacterial difference on different taxonomic levels. The results are illustrated in Figure 3 and showed a remarkable difference in gut microbiota between groups (Figures 3A,B). The phylum Verrucomicrobia was increased whereas phylum Actinobacteria was decreased in MSA patients. At the genus level, MSA group possessed more abundant Akkermansia, while Megamonas, Bifidobacterium, Blautia, and Aggregatibacter were more abundant in the healthy group. Further, to analyze at the species level, the relative abundance of Roseburia hominis, Akkermansia muciniphila, Alistipes onderdonkii, Streptococcus parasanguinis, and Staphylococcus xylosus in the MSA group was higher than those in the healthy group, while the healthy group had more abundant Bacteroides coprocola, Megamonas funiformis, Bifidobacterium pseudocatenulatum, Clostridium nexile, Bacteroides plebeius, and Granulicatella adiacens. In addition, we did not detect the differential taxa at an FDR q value cutoff of 0.05 between MSA-C and MSA-P subtypes. Since only four MSA-P patients were included in our study, differential microbiome analysis between MSA subtypes needs further verification in larger samples.
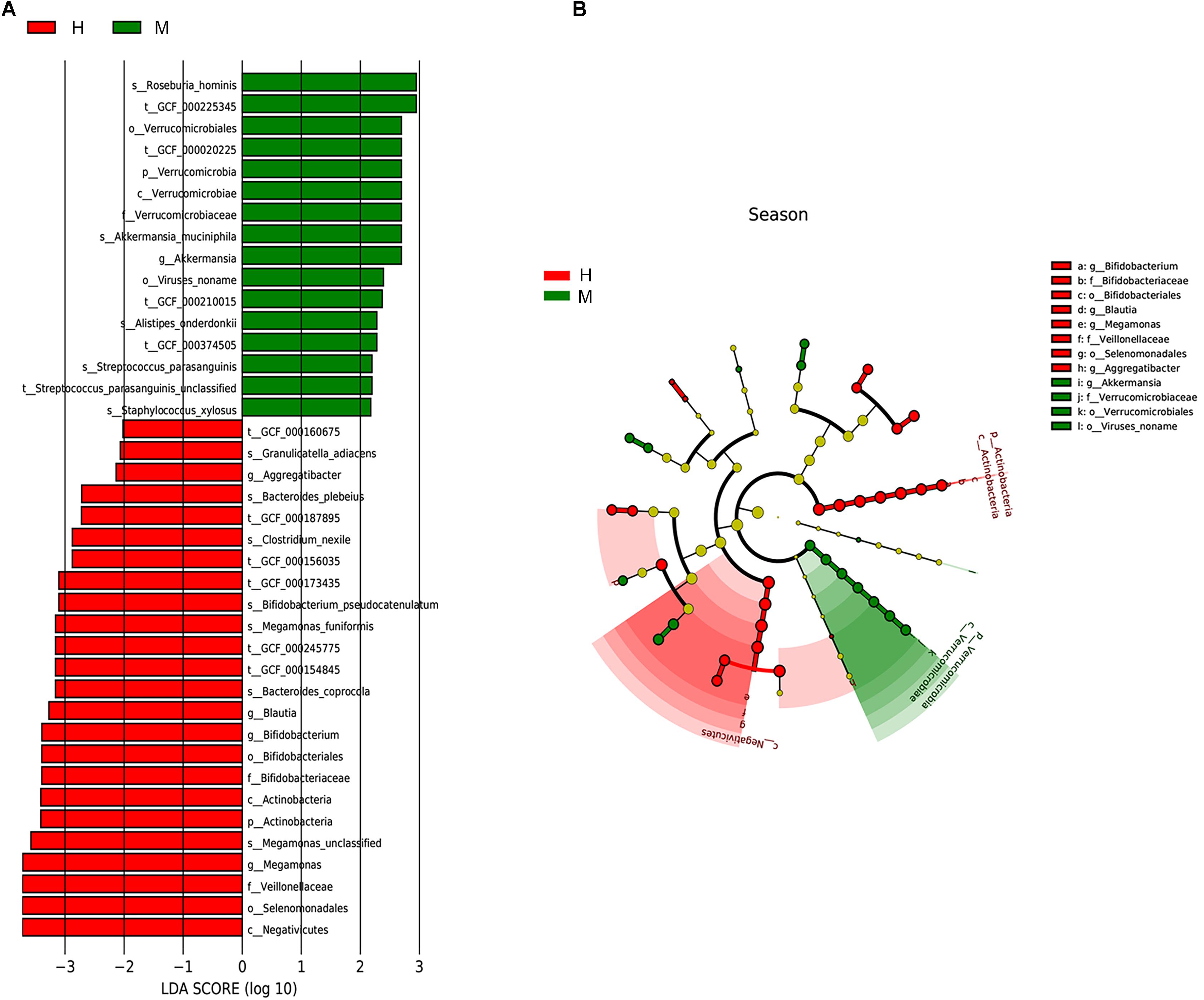
Figure 3. Taxonomic differences of gut microbiota in MSA and healthy groups predicted on different levels. (A) Histogram of the LDA scores computed for taxa differentially abundant between groups. (B) Cladogram indicated the phylogenetic distribution of fecal microbiota associated with MSA and healthy subjects. Each dot represented a taxonomic entity and the taxonomic levels ranged from phylum to species from the inner to outer circles.
Gene Prediction and Non-redundant Gene Catalog Construction
After de novo assembly, we obtained contigs with a mean number of 105.97 ± 47.78 thousand in each sample and the assembly result of each sample was illustrated in Supplementary Table S3. Then, we performed prediction on contigs to gain gene and protein sequences. The distribution of gene length in each sample is shown in Supplementary Figure S1 and Supplementary Table S4. After clustering on predicted genes, we selected the longest sequence of each cluster to construct a non-redundant catalog, and the final non-redundant gene catalog contained 1,925,921 microbial genes.
Differential Functional Pathways Analysis
To further investigate significantly altered functions between MSA patients and healthy controls’ gut microbiota, we annotated the gene catalog against the KEGG database and dbCAN database. Based on the KEGG database, the gene counts under each functional pathway were illustrated in Supplementary Figure S2 and the metabolism function catalog was with the most genes distributed. As illustrated in Figure 4 (p-value < 0.05, FDR q value < 0.1), the microbial gene functions including galactose metabolism (p-value = 0.001, FDR q value = 0.066), methane metabolism (p-value = 0.003, FDR q value = 0.066), and pantothenate and CoA biosynthesis (p-value = 0.002, FDR q value = 0.066) were much higher in fecal microbiome of the healthy group. In addition, a vital role of the human intestinal microbiota in hosts is to metabolize the dietary glycans and the carbohydrates of the host mucus (Koropatkin et al., 2012). Carbohydrate-active enzymes (CAZymes) encoded by the gut microbiota are responsible for synthesis, degradation, and modification of carbohydrates (Yin et al., 2012). The distribution of gene counts annotated under different CAZyme terms is shown in Supplementary Figure S3. However, there were no significantly differential CAZyme terms detected at an FDR cutoff of 0.1 between two groups.

Figure 4. Differential KEGG pathways at level 3 for the fecal microbiome of MSA and healthy groups. Mean proportions are shown in stacks for MSA (orange) and healthy (blue) groups. Difference in mean proportions = mean proportions in healthy group minus mean proportions in MSA groups. q values were calculated through Storey’s FDR method.
Discussion
Our study showed that MSA patients had a distinction in taxa composition and function of gut microbiota compared to healthy controls based on metagenomic sequencing of fecal samples. Although fecal microbiota may not reflect gut bacterial communities exactly, metagenomic sequencing based on stool could still be informative. It contained bacteria from mucosal desquamation and was the easiest sampling technique up to the standard of related investigations (Durban et al., 2011; Vazquez-Castellanos et al., 2015). Our study revealed that MSA patients presented a distinctive microbiota composition compared to healthy controls, characterized by high abundant genus Akkermansia and species R. hominis, A. muciniphila, A. onderdonkii, S. parasanguinis, and S. xylosus, while low abundant genera Megamonas, Bifidobacterium, Blautia, and Aggregatibacter and species B. coprocola, M. funiformis, B. pseudocatenulatum, C. nexile, B. plebeius, and G. adiacens. Functional analysis based on the KEGG database showed that pathways involving galactose metabolism, methane metabolism, and pantothenate and CoA biosynthesis were less abundant in fecal microbiome of MSA patients. In dbCAN database, we did not detect the significantly differential CAZyme terms between two groups.
Multiple system atrophy is a fatal neurodegenerative disease as well as synucleinopathy, with challenges in pathogenesis explication, diagnosis, and therapy. Recently, increasing evidence has shown that alteration of the gut microbiota could influence neural development, cognition, and behavior via the bidirectional interaction with the brain–gut–microbiota axis (Rogers et al., 2016). In another synucleinopathy, Parkinson’s disease, 16SrDNA sequencing of sigmoid mucosal and fecal samples revealed that Ralstonia, Akkermansia, Oscillospira, and Bacteroides were more abundant while Blautia, Coprococcus, Roseburia, and Faecalibacterium were less enriched in Parkinson’s disease patients (Keshavarzian et al., 2015). Besides, colonization with microbiota from Parkinson’s disease patients was found to exacerbate physical impairments in germ-free transgenic Parkinson’s disease mice (Sampson et al., 2016). As for MSA, Engen et al. found that at the family level, the abundance of Clostridiaceae and Rikenellaceae were higher while Lachnospiraceae (genera Ruminococcus, Roseburia, and Coprococcus) and Ruminococcaceae (genus Faecalibacterium) were lower in American MSA patients’ fecal sample (Engen et al., 2017). Tan et al. found that in ethnic Chinese of Malaysia, MSA patients had more abundant Bacteroides and less abundant Paraprevotella in fecal microbiota at the genus level (Tan A.H. et al., 2018). Both two studies were based on 16SrDNA sequencing.
Among different bacterial taxa based on our study, genus Akkermansia was also found to be increased in gut microbiota of Parkinson’s disease patients (Keshavarzian et al., 2015; Petrov et al., 2017). Akkermansia could produce short-chain fatty acids (SCFAs). SCFAs had several beneficial effects on host: maintaining epithelial barrier function, diminishing oxidative DNA damage, regulating cytokine production, anti-inflammatory effects, and stimulating immune function (Maslowski and Mackay, 2011). However, Akkermansia was reported to have proinflammatory properties, upregulating genes involved in antigen presentation pathway, B and T cell receptor signaling, IL-4 signaling, and complement and coagulation cascades (Derrien et al., 2011). These proinflammatory features may be due to its ability to disturb host mucus homeostasis, resulting in the breakdown of the gut barrier (Ganesh et al., 2013). Inflammation served a critical part in MSA pathogenesis (Fanciulli and Wenning, 2015; Zhou et al., 2018) and gut inflammation was shown to increase the risk of MSA. Besides, some gut-inflammation risk genes could also increase the risk of MSA, such as LRRK2 and NOD2; therefore, putative proinflammatory bacterial genera may participate in stimulating MSA process (Franke et al., 2010; Heckman et al., 2014; Engen et al., 2017; Cao et al., 2018; Villumsen et al., 2019). As for reduced bacteria, Blautia was a butyrate-producing genus (Keshavarzian et al., 2015) and butyrate was the most pronounced SCFA, which had anti-inflammation properties (Maslowski and Mackay, 2011; Pirozzi et al., 2018). Genus Bifidobacterium was also an inflammation-suppressing bacteria, and bioactive factors from Bifidobacterium were shown to improve epithelial cell barrier resistance and, thus, attenuated inflammation (Ewaschuk et al., 2008), which hinted the suppressive influence of Bifidobacterium for MSA. However, the detailed roles of these different bacterial taxa in pathogenesis of MSA still need further exploration. The bacterial alteration in MSA patients of mainland China identified from our study was different from that in American and ethnic Chinese of Malaysia (Engen et al., 2017; Tan A.H. et al., 2018). Numerous factors may be responsible for the discrepancy. First, there is the inherent discrepancy between 16SrRNA and metagenomic sequencing, which was reported previously in other studies (Shah et al., 2011; Poretsky et al., 2014; Hong et al., 2016). Then, bias due to the small sample size could not be excluded.
Our functional analysis revealed the aberrant functional pathways of MSA patients’ gut microbiota based on the KEGG database, which were all related with metabolism. Galactose metabolism was attached to the carbohydrate metabolism, and methane metabolism was related to the energy metabolism. Moreover, pantothenate and CoA biosynthesis belonged to the metabolism of cofactors and vitamins. The results revealed the aberrant metabolism in MSA patients’ gut microbiota. The host–microbe metabolic axis is a multi-directional interactive chemical highway between specific host and microbe functional pathways. The gut microbiota could produce a large array of metabolites that are necessary for host health, such as bile acids, choline, SCFAs, aromatic amino acids, etc. Microbe-produced metabolites could affect host metabolic phenotype, energy homeostasis, immune, inflammation, etc., and hence regulate disease process (Nicholson et al., 2012). Energy failure and inflammation are significant contributors to MSA pathogenesis (Jellinger, 2014). For more details about the functional alteration in gut microbiota of MSA patients and its roles in the MSA process, multi-omics combination including metabonomics, transcriptomics, proteomics, etc., was required. In addition, a function experiment based on the germ-free mice was also necessary.
There were still big challenges in MSA pathogenesis interpretation, precise diagnosis, and efficient treatment. Going into the roles of gut microbiota in MSA may shed light on its pathogenesis. Besides, the bacterial taxa with differential abundance between MSA patients and healthy persons might be utilized as novel biomarkers, contributing to the clinical diagnosis. In addition, rebalancing the gut microbiota dysbiosis through pre-/probiotics may be a novel therapeutic target for this untreated disease. However, several limitations need to be taken into account in our study. Firstly, the sample size in our study was small due to the low morbidity and rapidly progressive process of MSA (Geser et al., 2006; Fanciulli and Wenning, 2015). Thus, the larger-scale researches including different population were required. Then, our study was a cross-sectional study and whether the change of gut microbiota was the initiating stimulator or the result of MSA pathogenesis remained unclear. Therefore, investigation on the gut microbiota in longitudinal studies was indispensable across different periods of MSA process.
Conclusion
In conclusion, we found altered gut microbiota composition and functional pathways in MSA patients. Our study provided microbial basis for the further multi-omics research and functional experiments to clarify roles of gut microbiota in MSA, which may improve our understanding of MSA pathogenesis and facilitate the development of accurate diagnosis methods, as well as novel therapeutic strategies targeting modifying gut microbiota in MSA patients.
Data Availability Statement
The raw data supporting the conclusions of this manuscript are available in the SRA database under the accession number PRJNA532538.
Ethics Statement
This study was approved by the Ethics Committee of Xiangya Hospital of Central South University in China. All subjects gave written informed consent in accordance with the Declaration of Helsinki.
Author Contributions
LW, XZ, and HJ designed the project. LW, XZ, CW, ZC, HP, XuH, YP, PW, and TL organized and executed the experiment. LW, XZ, HY, YS, XiH, KXu, YX, and LH analyzed and interpreted the data. LW wrote the manuscript. KXi, BT, and HJ revised the manuscript. HJ agreed to be accountable for the content of the work. All authors approved the final version of the manuscript.
Funding
This study was supported by the National Key Research and Development Program of China (Nos. 2016YFC0901504 and 2016YFC0905100 to HJ and No. 2016YFC1306000 to BT), the National Natural Science Foundation of China (Nos. 81771231, 81471156, and 81974176 to HJ, No. 81600995 to YS, No. 81901169 to ZC, and No. 81901305 to CW), the Key Research and Development Program of Hunan Province (No. 2018SK2092 to HJ), the Scientific Research Foundation of Health Commission of Hunan Province (No. B2019183 to HJ), and the Clinical and Rehabilitation Fund of Peking University Weiming Biotech Group (No. xywm2015I10 to HJ).
Conflict of Interest
The authors declare that the research was conducted in the absence of any commercial or financial relationships that could be construed as a potential conflict of interest.
Acknowledgments
We thank all of the participants for their involvement in this study. We are also grateful to Dr. Peng Zheng for a critical review of the manuscript.
Supplementary Material
The Supplementary Material for this article can be found online at: https://www.frontiersin.org/articles/10.3389/fnins.2019.01102/full#supplementary-material
References
Cao, B., Chen, Y., Zhou, Q., Zhang, L., Ou, R., Wei, Q., et al. (2018). Functional variant rs3135500 in NOD2 increases the risk of multiple system atrophy in a chinese population. Front. Aging Neurosci. 10:150. doi: 10.3389/fnagi.2018.00150
Cao, Y., Fanning, S., Proos, S., Jordan, K., and Srikumar, S. (2017). A review on the applications of next generation sequencing technologies as applied to food-related microbiome studies. Front. Microbiol. 8:1829. doi: 10.3389/fmicb.2017.01829
Collins, K. H., Paul, H. A., Reimer, R. A., Seerattan, R. A., Hart, D. A., and Herzog, W. (2015). Relationship between inflammation, the gut microbiota, and metabolic osteoarthritis development: studies in a rat model. Osteoarthr. Cartil. 23, 1989–1998. doi: 10.1016/j.joca.2015.03.014
Derrien, M., Van Baarlen, P., Hooiveld, G., Norin, E., Muller, M., and de Vos, W. M. (2011). Modulation of mucosal immune response, tolerance, and proliferation in mice colonized by the mucin-degrader Akkermansia muciniphila. Front. Microbiol. 2:166. doi: 10.3389/fmicb.2011.00166
Dinan, T. G., and Cryan, J. F. (2017). Gut-brain axis in 2016: brain-gut-microbiota axis - mood, metabolism and behaviour. Nat. Rev. Gastroenterol. Hepatol. 14, 69–70. doi: 10.1038/nrgastro.2016.200
Durban, A., Abellan, J. J., Jimenez-Hernandez, N., Ponce, M., Ponce, J., Sala, T., et al. (2011). Assessing gut microbial diversity from feces and rectal mucosa. Microb. Ecol. 61, 123–133. doi: 10.1007/s00248-010-9738-y
Engen, P. A., Dodiya, H. B., Naqib, A., Forsyth, C. B., Green, S. J., Voigt, R. M., et al. (2017). The Potential role of gut-derived inflammation in multiple system atrophy. J. Parkinsons Dis. 7, 331–346. doi: 10.3233/jpd-160991
Ewaschuk, J. B., Diaz, H., Meddings, L., Diederichs, B., Dmytrash, A., Backer, J., et al. (2008). Secreted bioactive factors from Bifidobacterium infantis enhance epithelial cell barrier function. Am. J. Physiol. Gastrointest. Liver Physiol. 295, G1025–G1034. doi: 10.1152/ajpgi.90227.2008
Fanciulli, A., and Wenning, G. K. (2015). Multiple-system atrophy. N. Engl. J. Med. 372, 249–263. doi: 10.1056/NEJMra1311488
Flabeau, O., Meissner, W. G., and Tison, F. (2010). Multiple system atrophy: current and future approaches to management. Ther. Adv. Neurol. Disord. 3, 249–263. doi: 10.1177/1756285610375328
Franke, A., McGovern, D. P., Barrett, J. C., Wang, K., Radford-Smith, G. L., Ahmad, T., et al. (2010). Genome-wide meta-analysis increases to 71 the number of confirmed Crohn’s disease susceptibility loci. Nat. Genet. 42, 1118–1125. doi: 10.1038/ng.717
Gai, W. P., Power, J. H., Blumbergs, P. C., and Blessing, W. W. (1998). Multiple-system atrophy: a new alpha-synuclein disease? Lancet 352, 547–548. doi: 10.1016/s0140-6736(05)79256-4
Ganesh, B. P., Klopfleisch, R., Loh, G., and Blaut, M. (2013). Commensal Akkermansia muciniphila exacerbates gut inflammation in Salmonella typhimurium-infected gnotobiotic mice. PLoS One 8:e74963. doi: 10.1371/journal.pone.0074963
Geser, F., Wenning, G. K., Seppi, K., Stampfer-Kountchev, M., Scherfler, C., Sawires, M., et al. (2006). Progression of multiple system atrophy (MSA): a prospective natural history study by the European MSA study group (EMSA SG). Mov. Disord. 21, 179–186. doi: 10.1002/mds.20678
Gilman, S., Wenning, G. K., Low, P. A., Brooks, D. J., Mathias, C. J., Trojanowski, J. Q., et al. (2008). Second consensus statement on the diagnosis of multiple system atrophy. Neurology 71, 670–676. doi: 10.1212/01.wnl.0000324625.00404.15
Heckman, M. G., Schottlaender, L., Soto-Ortolaza, A. I., Diehl, N. N., Rayaprolu, S., Ogaki, K., et al. (2014). LRRK2 exonic variants and risk of multiple system atrophy. Neurology 83, 2256–2261. doi: 10.1212/wnl.0000000000001078
Hong, X., Chen, J., Liu, L., Wu, H., Tan, H., Xie, G., et al. (2016). Metagenomic sequencing reveals the relationship between microbiota composition and quality of Chinese Rice Wine. Sci. Rep. 6:26621. doi: 10.1038/srep26621
Jangi, S., Gandhi, R., Cox, L. M., Li, N., von Glehn, F., Yan, R., et al. (2016). Alterations of the human gut microbiome in multiple sclerosis. Nat. Commun. 7:12015. doi: 10.1038/ncomms12015
Jellinger, K. A. (2014). Neuropathology of multiple system atrophy: new thoughts about pathogenesis. Mov. Disord. 29, 1720–1741. doi: 10.1002/mds.26052
Kanehisa, M., Goto, S., Kawashima, S., Okuno, Y., and Hattori, M. (2004). The KEGG resource for deciphering the genome. Nucleic Acids Res. 32, D277–D280. doi: 10.1093/nar/gkh063
Keshavarzian, A., Green, S. J., Engen, P. A., Voigt, R. M., Naqib, A., Forsyth, C. B., et al. (2015). Colonic bacterial composition in Parkinson’s disease. Mov. Disord. 30, 1351–1360. doi: 10.1002/mds.26307
Koropatkin, N. M., Cameron, E. A., and Martens, E. C. (2012). How glycan metabolism shapes the human gut microbiota. Nat. Rev. Microbiol. 10, 323–335. doi: 10.1038/nrmicro2746
Li, J., Zhao, F., Wang, Y., Chen, J., Tao, J., Tian, G., et al. (2017). Gut microbiota dysbiosis contributes to the development of hypertension. Microbiome 5:14. doi: 10.1186/s40168-016-0222-x
Li, Y., Luo, W., Deng, Z., and Lei, G. (2016). Diet-intestinal microbiota axis in osteoarthritis: a possible role. Mediators Inflamm. 2016:3495173. doi: 10.1155/2016/3495173
Liu, R., Hong, J., Xu, X., Feng, Q., Zhang, D., Gu, Y., et al. (2017). Gut microbiome and serum metabolome alterations in obesity and after weight-loss intervention. Nat. Med. 23, 859–868. doi: 10.1038/nm.4358
Martinez, K. B., Leone, V., and Chang, E. B. (2017). Microbial metabolites in health and disease: navigating the unknown in search of function. J. Biol. Chem. 292, 8553–8559. doi: 10.1074/jbc.R116.752899
Maslowski, K. M., and Mackay, C. R. (2011). Diet, gut microbiota and immune responses. Nat. Immunol. 12, 5–9. doi: 10.1038/ni0111-5
Nicholson, J. K., Holmes, E., Kinross, J., Burcelin, R., Gibson, G., Jia, W., et al. (2012). Host-gut microbiota metabolic interactions. Science 336, 1262–1267. doi: 10.1126/science.1223813
Papp, M. I., Kahn, J. E., and Lantos, P. L. (1989). Glial cytoplasmic inclusions in the CNS of patients with multiple system atrophy (striatonigral degeneration, olivopontocerebellar atrophy and Shy-Drager syndrome). J. Neurol. Sci. 94, 79–100. doi: 10.1016/0022-510x(89)90219-0
Petrov, V. A., Saltykova, I. V., Zhukova, I. A., Alifirova, V. M., Zhukova, N. G., Dorofeeva, Y. B., et al. (2017). Analysis of gut microbiota in patients with Parkinson’s disease. Bull. Exp. Biol. Med. 162, 734–737. doi: 10.1007/s10517-017-3700-7
Pirozzi, C., Francisco, V., Guida, F. D., Gomez, R., Lago, F., Pino, J., et al. (2018). Butyrate modulates inflammation in chondrocytes via GPR43 receptor. Cell Physiol. Biochem. 51, 228–243. doi: 10.1159/000495203
Poretsky, R., Rodriguez, R. L., Luo, C., Tsementzi, D., and Konstantinidis, K. T. (2014). Strengths and limitations of 16S rRNA gene amplicon sequencing in revealing temporal microbial community dynamics. PLoS One 9:e93827. doi: 10.1371/journal.pone.0093827
Qiu, D., Xia, Z., Jiao, X., Deng, J., Zhang, L., and Li, J. (2018). Altered gut microbiota in myasthenia gravis. Front. Microbiol. 9:2627. doi: 10.3389/fmicb.2018.02627
Rogers, G. B., Keating, D. J., Young, R. L., Wong, M. L., Licinio, J., and Wesselingh, S. (2016). From gut dysbiosis to altered brain function and mental illness: mechanisms and pathways. Mol. Psychiatry 21, 738–748. doi: 10.1038/mp.2016.50
Roumpeka, D. D., Wallace, R. J., Escalettes, F., Fotheringham, I., and Watson, M. (2017). A review of bioinformatics tools for bio-prospecting from metagenomic sequence data. Front. Genet. 8:23. doi: 10.3389/fgene.2017.00023
Sampson, T. R., Debelius, J. W., Thron, T., Janssen, S., Shastri, G. G., Ilhan, Z. E., et al. (2016). Gut microbiota regulate motor deficits and neuroinflammation in a model of Parkinson’s disease. Cell 167, 1469.e12–1480.e12. doi: 10.1016/j.cell.2016.11.018
Segata, N., Izard, J., Waldron, L., Gevers, D., Miropolsky, L., Garrett, W. S., et al. (2011). Metagenomic biomarker discovery and explanation. Genome Biol. 12:R60. doi: 10.1186/gb-2011-12-6-r60
Shah, N., Tang, H., Doak, T. G., and Ye, Y. (2011). Comparing bacterial communities inferred from 16S rRNA gene sequencing and shotgun metagenomics. Pac. Symp. Biocomput. 16, 165–176. doi: 10.1142/9789814335058_0018
Tan, A. H., Chong, C. W., Song, S. L., Teh, C. S. J., Yap, I. K. S., Loke, M. F., et al. (2018). Altered gut microbiome and metabolome in patients with multiple system atrophy. Mov. Disord. 33, 174–176. doi: 10.1002/mds.27203
Tan, L., Zhao, S., Zhu, W., Wu, L., Li, J., Shen, M., et al. (2018). The Akkermansia muciniphila is a gut microbiota signature in psoriasis. Exp. Dermatol. 27, 144–149. doi: 10.1111/exd.13463
Trojanowski, J. Q., and Revesz, T. (2007). Proposed neuropathological criteria for the post mortem diagnosis of multiple system atrophy. Neuropathol. Appl. Neurobiol. 33, 615–620. doi: 10.1111/j.1365-2990.2007.00907.x
Vazquez-Castellanos, J. F., Serrano-Villar, S., Latorre, A., Artacho, A., Ferrus, M. L., Madrid, N., et al. (2015). Altered metabolism of gut microbiota contributes to chronic immune activation in HIV-infected individuals. Mucosal. Immunol. 8, 760–772. doi: 10.1038/mi.2014.107
Villumsen, M., Aznar, S., Pakkenberg, B., Jess, T., and Brudek, T. (2019). Inflammatory bowel disease increases the risk of Parkinson’s disease: a Danish nationwide cohort study 1977-2014. Gut 68, 18–24. doi: 10.1136/gutjnl-2017-315666
Whiteside, S. A., Razvi, H., Dave, S., Reid, G., and Burton, J. P. (2015). The microbiome of the urinary tract–a role beyond infection. Nat. Rev. Urol. 12, 81–90. doi: 10.1038/nrurol.2014.361
Yin, Y., Mao, X., Yang, J., Chen, X., Mao, F., and Xu, Y. (2012). dbCAN: a web resource for automated carbohydrate-active enzyme annotation. Nucleic Acids Res. 40, W445–W451. doi: 10.1093/nar/gks479
Zhang, Q., Chen, X., Liu, Z., Varma, D. S., Wan, R., and Zhao, S. (2017). Diet diversity and nutritional status among adults in southwest China. PLoS One 12:e0172406. doi: 10.1371/journal.pone.0172406
Zhou, R., Fan, X., and Schnabl, B. (2019). Role of the intestinal microbiome in liver fibrosis development and new treatment strategies. Transl. Res. 209, 22–38. doi: 10.1016/j.trsl.2019.02.005
Zhou, X., Wang, C., Chen, Z., Peng, Y., Peng, H., Hou, X., et al. (2018). Association of TNF-alpha rs1799964 and IL-1beta rs16944 polymorphisms with multiple system atrophy in Chinese Han population. Int. J. Neurosci. 128, 761–764. doi: 10.1080/00207454.2017.1418346
Keywords: multiple system atrophy, microbiota, metagenomics, functional pathways, inflammation
Citation: Wan L, Zhou X, Wang C, Chen Z, Peng H, Hou X, Peng Y, Wang P, Li T, Yuan H, Shi Y, Hou X, Xu K, Xie Y, He L, Xia K, Tang B and Jiang H (2019) Alterations of the Gut Microbiota in Multiple System Atrophy Patients. Front. Neurosci. 13:1102. doi: 10.3389/fnins.2019.01102
Received: 04 May 2019; Accepted: 30 September 2019;
Published: 18 October 2019.
Edited by:
Odete A. B. da Cruz e Silva, University of Aveiro, PortugalReviewed by:
Pilar Codoñer-Franch, University of Valencia, SpainYi-Chung Lee, Taipei Veterans General Hospital, Taiwan
Copyright © 2019 Wan, Zhou, Wang, Chen, Peng, Hou, Peng, Wang, Li, Yuan, Shi, Hou, Xu, Xie, He, Xia, Tang and Jiang. This is an open-access article distributed under the terms of the Creative Commons Attribution License (CC BY). The use, distribution or reproduction in other forums is permitted, provided the original author(s) and the copyright owner(s) are credited and that the original publication in this journal is cited, in accordance with accepted academic practice. No use, distribution or reproduction is permitted which does not comply with these terms.
*Correspondence: Hong Jiang, jianghong73868@126.com
†These authors have contributed equally to this work