- 1Department of Neurology, The First Affiliated Hospital of Chongqing Medical University, Chongqing, China
- 2Institute of Neuroscience and the Collaborative Innovation Center for Brain Science, Chongqing Medical University, Chongqing, China
- 3School of Clinical Medicine, Ningxia Medical University, Yinchuan, China
- 4Department of Psychiatry, The First Affiliated Hospital of Chongqing Medical University, Chongqing, China
- 5Department of Neurology, The Second Affiliated Hospital of Chongqing Medical University, Chongqing, China
Depression is a common and disabling mental disorder characterized by high disability and mortality, but its physiopathology remains unclear. In this study, we combined a non-targeted gas chromatography-mass spectrometry (GC-MS)-based metabolomic approach and isobaric tags for relative and absolute quantitation (iTRAQ)-based proteomic analysis to elucidate metabolite and protein alterations in the hippocampus of rat after chronic social defeat stress (CSDS), an extensively used animal model of depression. Ingenuity pathway analysis (IPA) was conducted to integrate underlying relationships among differentially expressed metabolites and proteins. Twenty-five significantly different expressed metabolites and 234 differentially expressed proteins were identified between CSDS and control groups. IPA canonical pathways and network analyses revealed that intracellular second messenger/signal transduction cascades were most significantly altered in the hippocampus of CSDS rats, including cyclic adenosine monophosphate (cAMP), phosphoinositol, tyrosine kinase, and arachidonic acid systems. These results provide a better understanding of biological mechanisms underlying depression, and may help identify potential targets for novel antidepressants.
Introduction
Depression is one of the most prevalent psychiatric and disability diseases conditions in the world today, but its physiopathology remains poorly elucidated (Health TNIoM, 2016; World Health Organization [WHO], 2017). Among various animal models, social defeat stress is one of the most widely used for investigating possible causes of and treatments for depression (Becker et al., 2008; Ota et al., 2014). Chronic social stress requires learned social defeat for a long period, usually combined with repeated exposure to sensory stimuli from aggressive rodents (Denmark et al., 2010). The repeated exposure of rodents to social defeat causes a solid depression-like behavior marked by anhedonia, body weight alteration, and altered protein expression in various brain areas (Vyas et al., 2002; Radley and Morrison, 2005; Denmark et al., 2010).
Hippocampus is a key brain area for emotion and motivation generation and regulation, and plays a critical influence in the etiology of depression (Campbell and Macqueen, 2004; McEwen et al., 2012). Neuropathological studies of hippocampus showed atrophy of hippocampal neurons (Sousa et al., 2000; Harrison, 2002; Lucassen et al., 2006; McEwen, 2010). However, how these changes in hippocampus lead to depression still unclear (Christoffel et al., 2011). Revealing these molecular events is essential for understanding the pathogenesis of depression.
In recent decades, omics technologies have become a powerful tool to uncover the physiopathology of neuropsychiatric disorders (Zheng et al., 2013a, 2016a; Zhou et al., 2015). Metabolomics can quantify low-molecular-weight metabolites in specific biological sample, and is widely used to capture disease-specific metabolite signatures (Lindon and Nicholson, 2008; Koike et al., 2014; Proitsi et al., 2015). Proteomics – the analysis of protein expression in biological samples – can provide insights into the pathophysiological mechanisms of several disease states (Taurines et al., 2011). Previously, our team completed a series of research on depression in clinical patients and animal models using a single omics technology (Zheng et al., 2013b, 2016b; Chen et al., 2015; Liu et al., 2016; Wang et al., 2016; Zhou et al., 2018). Recently, researchers have started to use combinatorial omics approaches to study the physiopathology of various diseases, including cancer, cardiovascular disease, and psychiatric disorders (Mayr et al., 2008; Ma et al., 2012; Wesseling et al., 2013). In those studies, metabolomics informed functional interpretations of proteomic results, and proteomics helped to a better understanding of metabolomics results by emphasizing the involvement of specific enzymatic and/or enzymatic pathways. With respect to depression, significantly perturbed energy metabolism in the cerebellum and dysfunction of amino acid metabolism and lipid metabolism in the hippocampus of chronically stressed rats has been observed, and disturbance of phospholipid metabolism in the plasma of MDD patients has been found in previous studies using integrated analysis of proteomic and metabolomic profiles (Shao et al., 2015; Gui et al., 2018; Zhang et al., 2018). Hence, combining proteomic analysis with metabolic profiling is promising and may help to elucidate complete biological mechanisms (Cavill et al., 2016).
The purpose of this research was to elucidate metabolite and protein alterations induced by depression in rat hippocampus after CSDS. We combined a non-targeted GC-MS-based metabolomic approaches with iTRAQ-based proteomic analysis. Furthermore, IPA was conducted to integrate potential relationships among altered metabolites and proteins to better understand the physiopathology of depression.
Materials and Methods
Animals and Ethics Statement
This research (including stress and behavioral tests) was conducted in accordance with recommendations of the Guide for the Care and Use of Laboratory Animals, and approved by the Ethics Committee of Chongqing Medical University. All procedures contributing to this work comply with the ethical standards of the relevant national and institutional committees on animal experimentation. As described in our previous study, thirty-five male SD rats, 250–300 g, were kept in a separate cage as experimental intruder or control animals (Liu et al., 2018). Male LE rats, 380–450 g, were housed in pairs with ligatured female LEs served as resident rats. All animals lived under a 12-h day–and-night regime (lights on at 19:00) in controlled environmental conditions (21 ± 1°C, 55 ± 5% relative humidity) to ensure that all manipulations and tests were performed during the active phase (subjective night) of rats. Food and water were available ad libitum. Before starting the experimental procedures, all animals were adapted to light regime, controlled environmental conditions, as well as handling and presence of the experimenter in the room for 10 days.
Using measurements of body weight, sucrose preference, and activity, outliers at baseline were removed, leaving twenty-eight SD rats that were body weight matched and randomly divided into the CSDS group or CON group.
Experimental Procedures
Social-Defeat Procedure
The social defeat pattern included two consecutive periods (a total 60 min) in LE rats’ home cages. During period I (5 min), rats were allowed physical confrontation. In period I, when the interaction became too strong or once the SD rat surrendered or acquired a supine position over 5 seconds, the SD rat was transported to a small wire mesh protective cage (10 cm × 10 cm × 15 cm) within the LE’s home and spend the remaining 60 min (period II). Hence, the wire mesh cage allowed for comprehensive visual, olfactory, as well as auditory exposure to LE rat without direct physical contact. CON group rats were placed in the empty LE rats’ home cages for 60 min. The 60-min social defeat exposure was repeated once daily for 5 days during weeks 1 and 3, and once daily for 3 days during week 2. The overall experimental procedure is shown in Figure 1.
Behavioral Tests
Weight measurement and SPT were conducted at baseline and weekly during for 3 weeks. In addition, during the whole experiment, a series of behavioral tests were carried out in a soundproof room between 09:00 and 15:00 with a red light (1–2 lx), as mentioned in earlier publication (Liu et al., 2018). LAT was conducted only at baseline to test the activity of rats prior to stress. OFT, EPM, and FST were conducted after the CSDS procedure. Prior to LAT, FST, OFT, and EPM testing, each rat was individually transported to the testing room and adapted for 6–7 min. The testing order was alternated between CSDS and CON groups.
Sample Preparation
After a short period of anesthesia, two hippocampi were quickly dissected and separately immersed into liquid nitrogen and maintained at -80°C before analysis.
Metabolomic Analysis
Details of GC-MS approaches and procedures for metabolomic analysis were provided in our previous study (Liu et al., 2018). Metabolomic analysis was performed using SIMCA software (version 14.0) for principal component analysis (PCA) and OPLS-DA. The criterion for identifying significantly different metabolites was variable influence of projection (VIP) >1 and p-values <0.05. Heatmap and unsupervised hierarchical clustering analysis of differentially expressed metabolites were constructed using R (version 3.4.0). The MetaboAnalyst online tool (version 4.0) was performed to explore biological patterns, functions, and pathways of identified differentially expressed metabolites.
Proteomic Analysis
As we previously described, iTRAQ-based proteomic measurements were made using an iTRAQ Reagent-8plex Multiplex Kit (Zhang et al., 2018). Two pooled samples were obtained corresponding to 9 or 8 rats in each group (CSDS group: n = 9; CON group: n = 8), then each pooled sample was divided into three equal parts as replicates. Subsequently, peptides were mixed and fractionated by strong cation exchange chromatography, liquid chromatography–tandem mass spectrometry analysis, analysis by Q Exactive, and sequence database searching and analysis. Compared with the CON group, final proteins considered to be differentially expressed and chosen for further analysis by p-values <0.05 and 1.2-fold changes (>1.20 or <0.83) for proteomic analysis (Ren et al., 2013). Heatmap and hierarchical clustering analysis of feature proteins between two sample sets were constructed using R (version 3.4.0). The DAVID online tool (version 6.8) was used to annotate the function of differentially expressed proteins. The STRING online tool (version 9.0) was used to obtain differentially expressed protein interaction data.
Statistical Analysis
The results of behavioral tests including body weight, SPT, OFT, EPM, and FST are presented with mean ± standard deviation and calculated by IBM SPSS (version 21.0). For all analyses, p-values <0.05 were considered to have statistical significance.
Integrated Analysis of Metabolomics and Proteomics
Ingenuity pathway analysis (IPA Ingenuity Systems, Redwood City, CA, United States) software was used to analyze and integrative metabolites and proteins data (including canonical pathway and molecular interaction network). The network score was based on a hypergeometric distribution and calculated using the right-tail Fisher exact test.
Results
After screening, there were 28 rats (20 CSDS and 8 CON) for subsequent experiments. No significant differences in LAT, body weight, or SPT were found between CSDS and CON groups at baseline. After 3 weeks of social-defeat exposure, the 20 CSDS rats were divided into susceptible and resilient subgroups according to their SPT. In this research, only susceptible rats (9 CSDS) were used for further analysis, with no significant differences in baseline body weight (281.94g vs. 289.06g, p = 0.392), SPT (84.01% ± 9.83% vs. 85.02% ± 7.70%, p = 0.819), or LAT (1544.20 cm vs. 1624.64 cm, p = 0.700) between CSDS (n = 9) and CON (n = 8) groups (Supplementary Figure S1).
The results of behavioral tests were reported in our previous publication (Liu et al., 2018). Briefly, the CSDS group gained less body weight than the CON group after 1 week (293.38g vs. 314.99g, t = -2.70, p < 0.05), 2 weeks (305.04g vs. 335.83g, t = -3.52, p < 0.05), and 3 weeks (324.83g vs. 356.28g, t = -3.12, p < 0.05) (Supplementary Figure S1A). The sucrose preference of CSDS group was lower than that of CON group (85.98% vs. 91.41%, p < 0.05; Supplementary Figure S1B). In FST, immobility time of CSDS group was longer than that of CON group (130.63 vs. 112.39, p < 0.05; Supplementary Figure S1C), indicating aggravated despair behavior. In OFT, total distance (2804.82 vs. 3249.95, t = -1.16, p > 0.05; Supplementary Figure S1D), time spent in the inner squares, and number of rearing behaviors (8.94 vs. 9.91, t = -0.26, p > 0.05; 13.78 vs. 14.25, t = -0.14, p > 0.05; Supplementary Figures S1E,F) did not differ between the two groups, indicating no anxiety-like behavior in this model. In EPM, time spent in open arms and frequency (67.78 vs. 40.81, z = -0.55, p > 0.05; 3.44 vs. 2.50, t = 0.80, p > 0.05; Supplementary Figures S1G,I), as well as time spent in closed arms and frequency (120.06 vs. 158.44, t = -0.27, p > 0.05; 11.00 vs. 11.63, t = -1.34, p > 0.05; Supplementary Figures S1H,J) did not differ between the two groups.
GC-MS-Based Metabolomic Analyses
Using GC-MS metabolomic profiling of hippocampus from CSDS and CON groups, 413 metabolites were identified and used for subsequent multi-variate analysis. PCA score indicated clear segregation between CSDS group and CON group (R2X = 0.431, Q2 = 0.147; Figure 2A). In addition, the resulting OPLS-DA scores plot clearly distinguished CSDS group from CON group (R2X = 0.896, R2Y = 0.890, Q2 = 0.762; Figure 2B). The results of permutation tests showed no overfitting in the OPLS-DA model (R2 = 0.52, Q2 = -0.115; Figure 2C). Based on the OPLS-DA model, 25 differentially expressed metabolites were identified between CSDS and CON groups using criteria of VIP > 1 and p-values < 0.05 (Supplementary Table S1). Heatmap visualization of 25 differential metabolomics data is displayed in Figure 2D. Overviews of pathway and enrichment analyses based on metabolite alterations are displayed in Figures 2E,F.
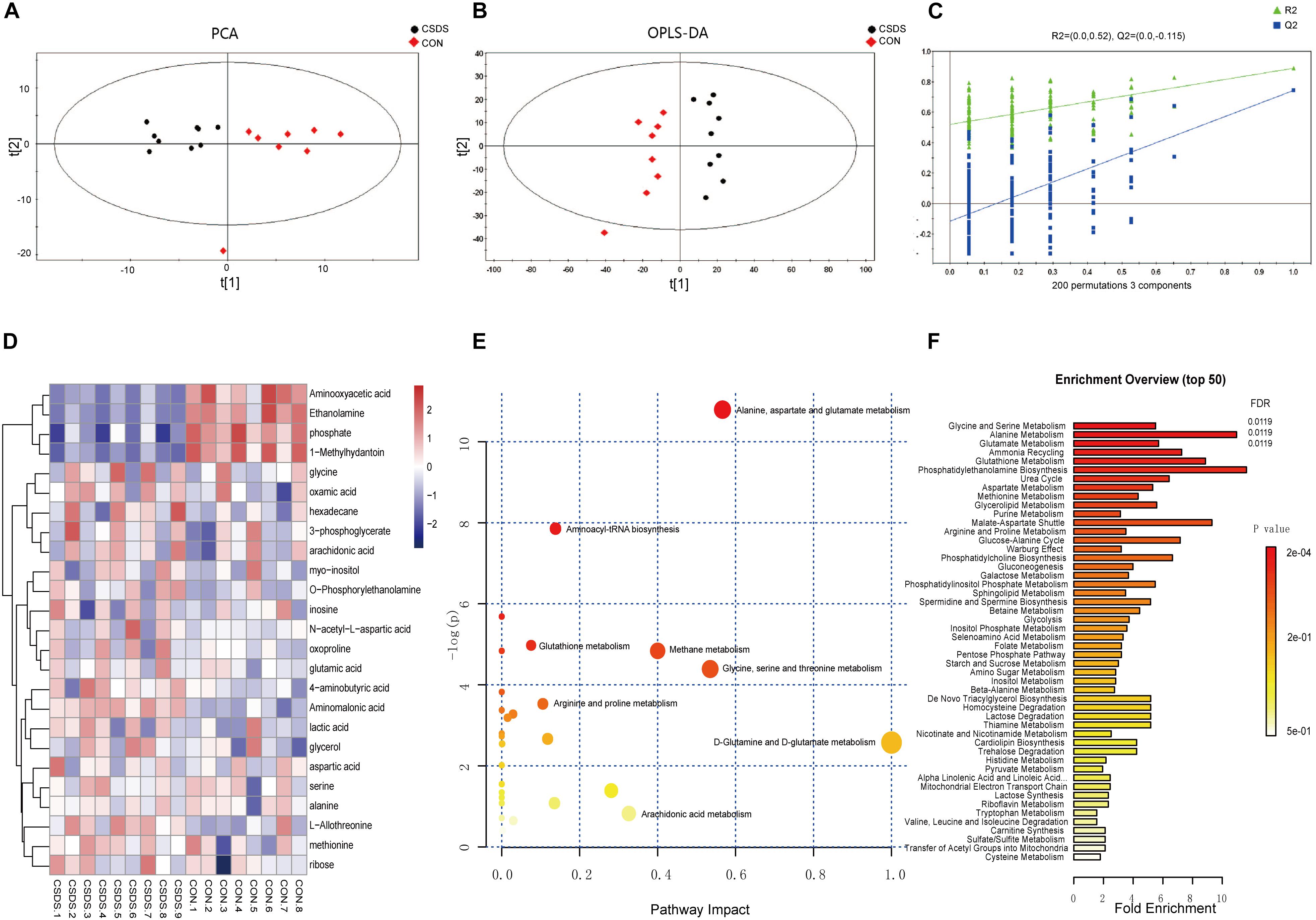
Figure 2. Metabolomic analysis of hippocampi from CSDS and CON group rats. (A) Principal component analysis (PCA) scores plot of CSDS group rats (black dots) and CON group rats (red diamonds). (B) Orthogonal partial least-squares discriminant analysis (OPLS-DA) scores of CSDS group rats (black dots) and CON group rats (red diamonds). (C) A 200-iteration permutation test showing the original values (top right) of R2 (green triangles) and Q2 (blue squares) was significantly higher than corresponding permuted values (bottom left), demonstrating the OPLS-DA model was not-overfitted. (D) Heatmap of 25 differentially expressed metabolites in the CSDS model: rows, metabolites; columns, samples. (E) Overview of pathway analysis based on metabolite alterations. (F) Overview of enrichment analysis based on metabolite alterations.
iTRAQ-Based Proteomic Analyses
Using iTRAQ-based proteomic profiling of hippocampus from CSDS and CON groups, 4950 proteins were detected with at least one unique peptide and a 1% FDR. A volcano plot of all proteins is displayed in Figure 3A. Based on the criteria mentioned above, 234 differentially expressed proteins were identified and chosen for further analysis between CSDS group and CON group (Supplementary Table S2). Among them, 66 proteins were increased and 168 were decreased expression. Heatmap visualization of 234 differentially expressed proteins is displayed in Figure 3B. As shown in the Figure 3B, CSDS group and CON group were clearly separated, and three replicates of each group showed good reproducibility. According to hierarchical clustering analysis, proteins were resulted into three clusters. The proteins in cluster 1 were most associated with positive regulation of neuron death, while those in cluster 2 were most associated with translation, and those in cluster 3 were most associated with protein transport. Gene Ontology (GO) analysis annotated 221 proteins and then resulted them into 19 significant GO terms for biological processes (Figure 3D), 29 for cellular components (Figure 3E), and 14 for molecular function (Figure 3F). A total of 196 altered proteins were annotated by STRING. The Protein-Protein interaction (PPI) network of these proteins is showed in Figure 3C. The PPI network analysis revealed that MAP3K5, PIK3R2, RAC1, PIP4K2C, and PPP2R2B participated in various biological progress such as single-organism cellular process or single-organism transport, and MAP3K5, PIK3R2, RAC1, PIP4K2C, and BAD participated in neurotrophin signaling pathway, which may play a critical influence in the pathogenesis of depression. The significantly different canonical KEGG pathways are displayed in Figure 3G.
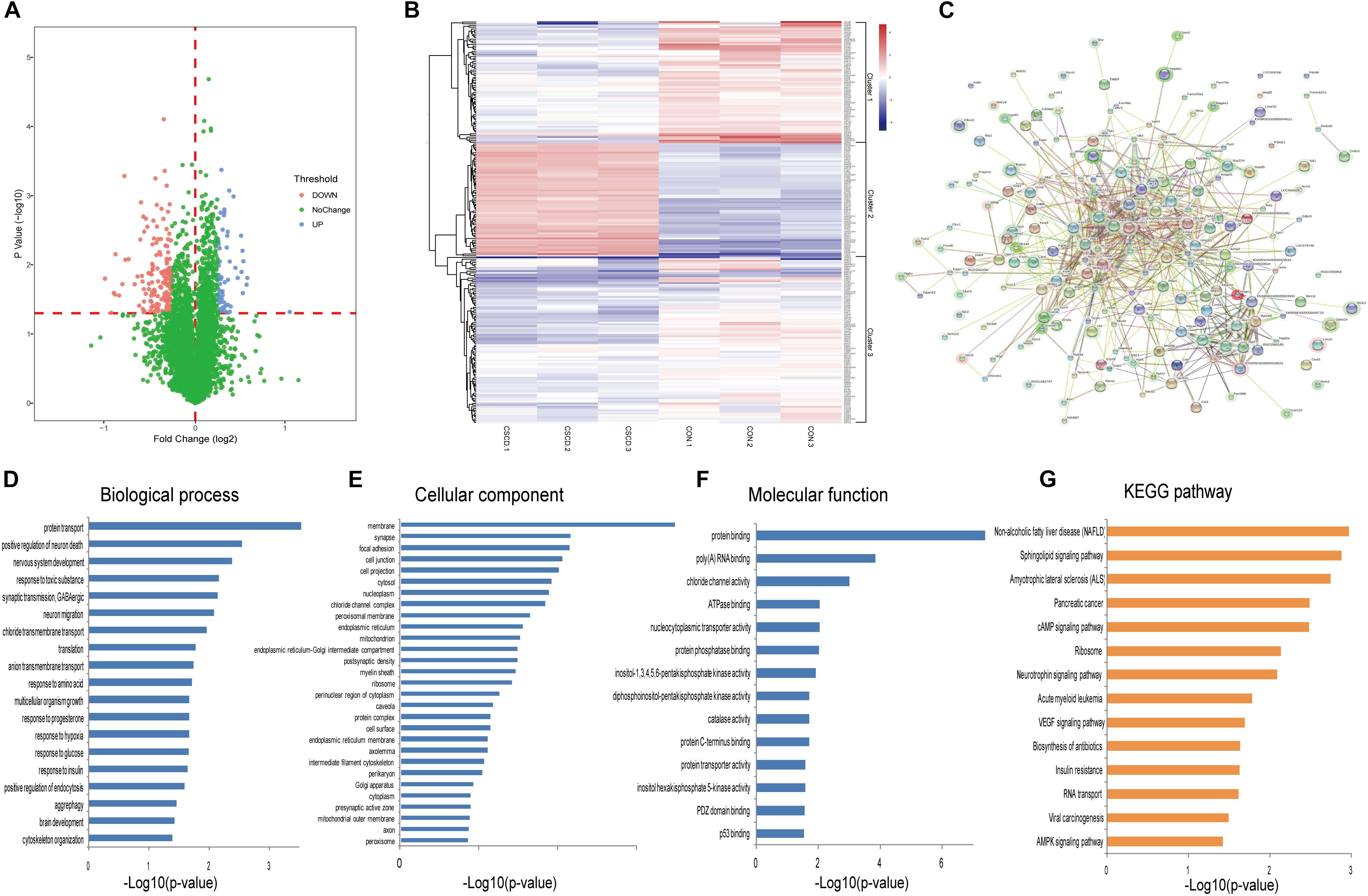
Figure 3. Proteomic analysis of hippocampi from CSDS and CON groups rats. (A) Volcano plot of all proteins. (B) Heatmap of 234 differentially expressed proteins in the CSDS model: rows, proteins; columns, samples. (C) Protein-Protein interaction (PPI) network of 196 differently expressed proteins which was performed with STRING. Nodes represent proteins. Edges represent protein-protein associations. (D–F) Distribution of altered proteins for biological processes, cellular components, and molecular functions. Only GO terms that were significantly overrepresented (p < 0.05) are shown. (G) Significantly enriched pathways identified by KEGG pathway analysis (p < 0.05).
Integrated Pathway and Network Analyses
The 25 differentially expressed metabolites and 234 differentially expressed proteins were submitted to IPA for integration analysis. According to canonical pathway analysis, the top five significantly different canonical pathways were Rac signaling, ceramide signaling, folate transformation I, S-methyl-5-thio-a-D-ribose 1-phosphate degradation, and regulation of eIF4 and p70S6K signaling (Supplementary Table S3). In the network function analysis, “cellular movement, cell death and survival, molecular transport” changed most significantly, with a score of 43 (Figure 4A). The “molecular transport, RNA trafficking, protein trafficking” and “auditory disease, hereditary disorder, neurological disease” networks were also identified as top-ranked enriched networks based on changed products, with scores of 41 and 27 respectively (Figures 4B,C). Molecular and cellular functions related to submitted metabolites and proteins were molecular transport, amino acid metabolism, small molecule biochemistry, cellular assembly and organization, and cellular function and maintenance.
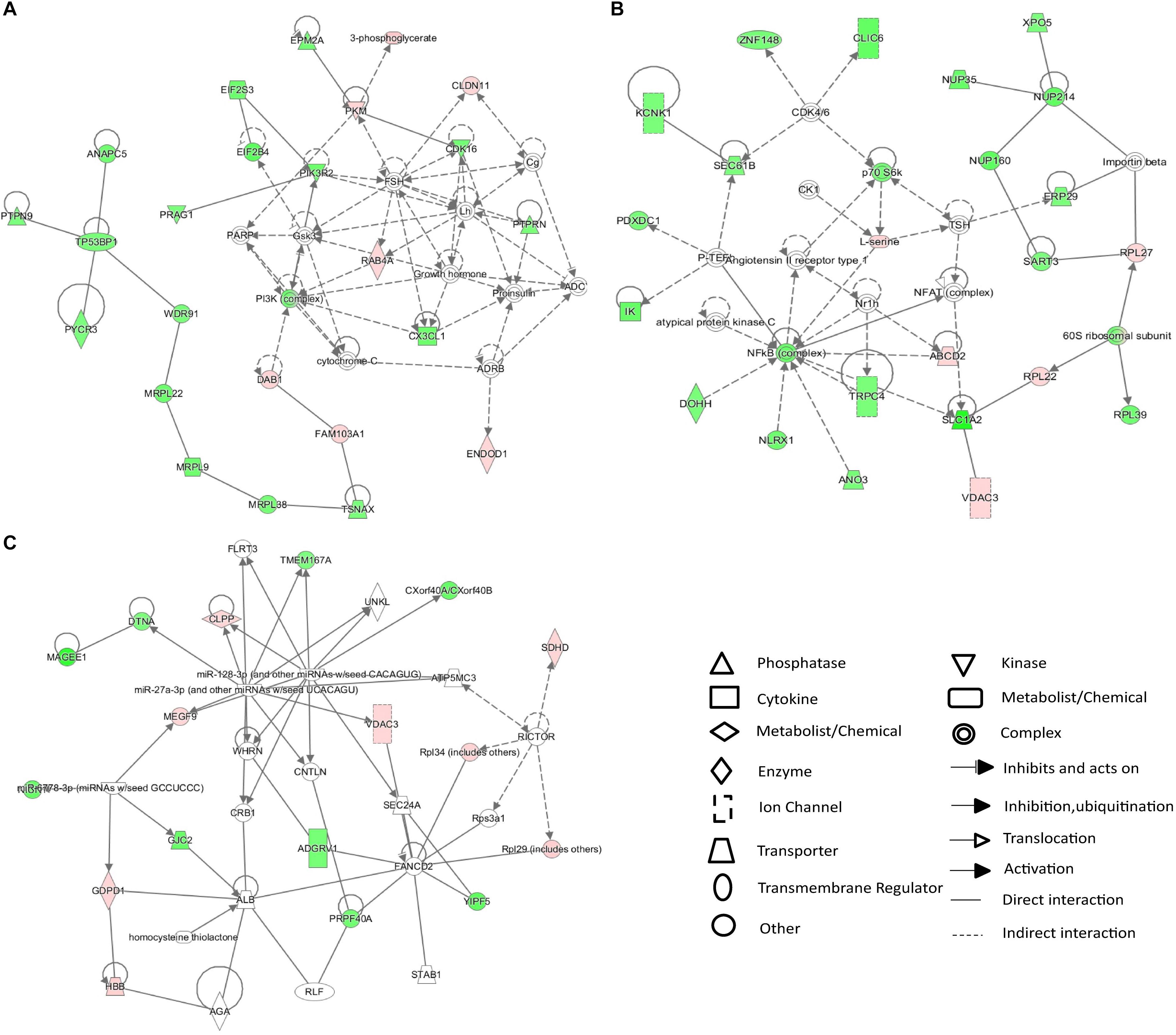
Figure 4. IPA network analysis of differentially expressed proteins and metabolites in the CSDS model group. (A–C) Top-ranked enriched networks based on changed products. Red, significantly increased; green, significantly decreased.
Discussion
In the current research, we conducted an integrated analysis of GC–MS-based metabolomics and iTRAQ-based proteomics to investigate molecular changes in the hippocampus of rats induced by CSDS. Twenty-five significantly different metabolites and 234 differentially expressed proteins were identified between CSDS and control groups. IPA canonical pathway and network analyses of integrated metabolites and proteins revealed that biomolecular changes in the hippocampus of CSDS rats are mainly related to intracellular second messenger/signal transduction cascades (Figure 5).
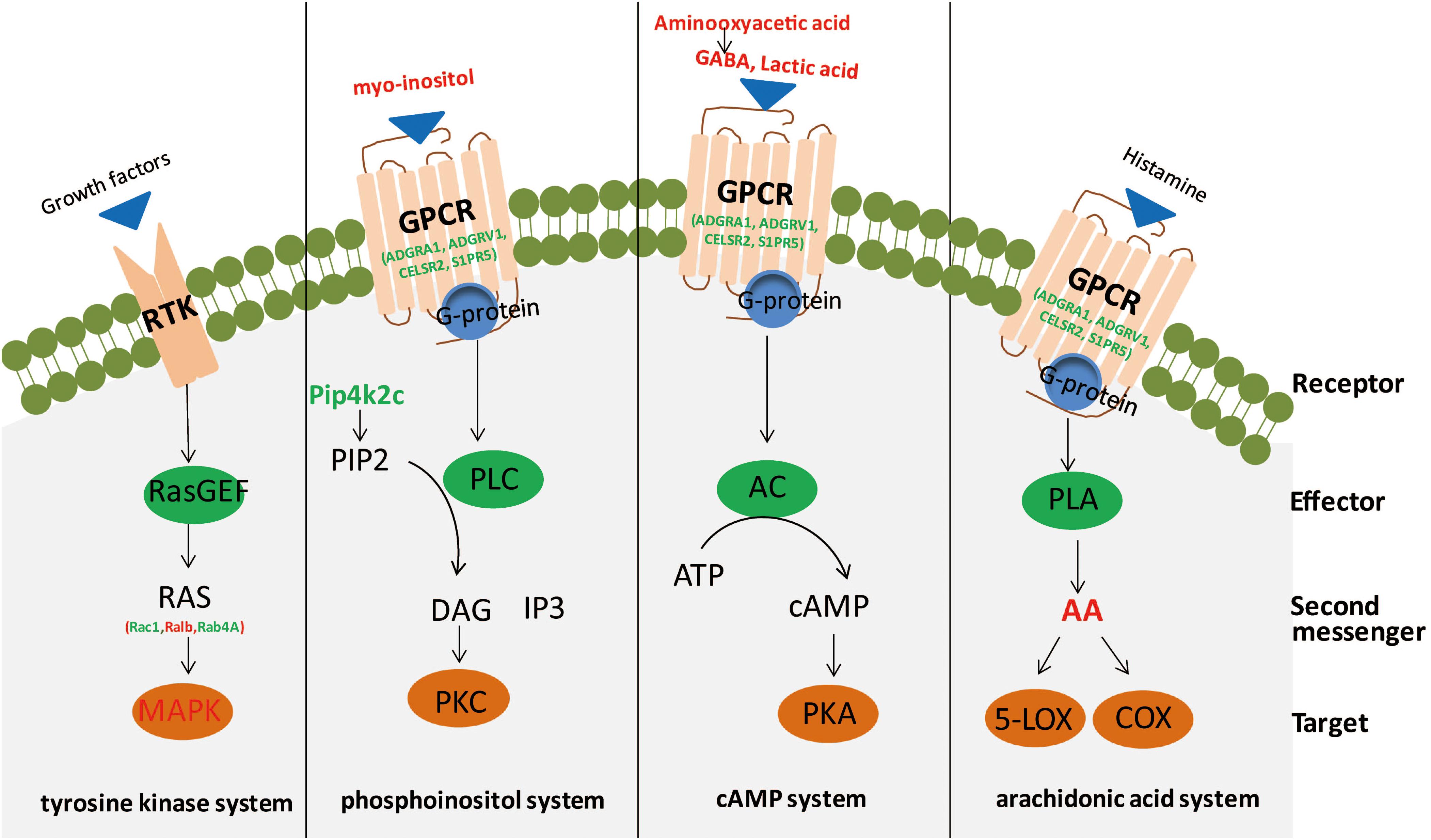
Figure 5. Second messengers disseminate information received from cell-surface receptors. A simplistic diagram of second messenger systems, including tyrosine kinase, phosphoinositol, cyclic adenosine monophosphate (cAMP), and arachidonic acid (AA) systems. In the tyrosine kinase system, binding of growth factors to a receptor tyrosine kinase (RTK; the receptor) can activate RasGEF (the effector) to produce RAS (the second messenger) to activate mitogen-activated protein kinase (MAPK; the target). For the phosphoinositol system, binding of ligands to a GPCR (receptor) activates phospholipase C (PLC; effector), to generate 2 s messengers, DAG and IP3, which activate protein kinase C (PKC; target) and release calcium from intracellular stores. Red, significantly increased; green, significantly decreased. In the cAMP system, binding of agonists to a GPCR (receptor) can activate adenylyl cyclase (AC; effector) to produce cAMP (second messenger) to activate protein kinase A (PKA; target). In the AA system, binding of ligands to a GPCR (receptor) can activate phospholipase A (PLA; effector) to generate AA (second messenger), which activates targets cyclooxygenase (COX) or 5-lipoxygenase (5-LOX).
Among the 25 differentially expressed metabolites, 21 in the hippocampus were also considered as potential biomarkers of depression in earlier researches, but most of these in chronic unpredictable mild stress model (Kim et al., 2014; Du et al., 2016; Liu et al., 2016; Zhang et al., 2018). The remaining metabolites (myo-inositol, aminomalonic acid, ribose, and oxamic acid) are identified as potential biomarkers for the first time. Consistent with a previous study, most are primarily involved in amino acid metabolism, carbohydrate metabolism, and lipid metabolism (Zhang et al., 2018). GO enrichment analysis of the 234 differentially expressed proteins revealed that most proteins are associated with protein transport, positive regulation of neuron death, nervous system development, response to toxic substances and synaptic transmission, and GABAergic. KEGG pathway analysis based on differentially expressed proteins revealed that sphingolipid signaling, cAMP signaling, and neurotrophin signaling, which have previously been associated with depression, are the main signaling pathways activated in the hippocampus of CSDS rats (Gould and Manji, 2002; Niciu et al., 2013).
Ingenuity pathway analysis canonical pathway and network analyses of integrated metabolites and proteins revealed that intracellular second messenger/signal transduction cascades were the most significantly altered in the hippocampus of CSDS rats. Indeed, the results of IPA canonical analyses showed six of the top ten pathways are related to second messenger signaling, including Rac signaling, ceramide signaling, regulation of eIF4 and p70S6K signaling, mTOR signaling, nerve growth factor signaling, and PI3K/AKT signaling (Niciu et al., 2013; Newton et al., 2016). The results of IPA network analyses revealed that the network “cellular movement, cell death and survival, molecular transport” was the most altered between CSDS and CON groups. Our findings are consistent with previous studies using animal models of depression or clinical patients of MDD, which revealed imbalance in second messenger/signal transduction cascades (Niciu et al., 2013). Several researchers have examined the critical influence of second messenger/signal transduction cascades in the physiopathology and treatment of depression, e.g., cAMP/PKA/CREB, neurotrophin-mediated (MAPK and others), p11, Wnt/Fz/Dvl/GSK3β, NFκB/Δfobs, mTOR, and eEF2K/CAMKIII, resulting in the second-messenger imbalance hypothesis (Wachtel, 1989, 1990; Niciu et al., 2013). In addition, previous studies found that more direct targeting of second messenger/signal transduction intermediate products may offer a faster and more solid effects than currently available antidepressants (Li et al., 2010; Autry et al., 2011; Monteggia et al., 2013).
Several distinct secondary messenger systems, such as cAMP, phosphoinositol, tyrosine kinase, and AA systems, are reportedly associated with depression (Odagaki et al., 2001; Gould and Manji, 2002; Skelin et al., 2011; Niciu et al., 2013; Fujita et al., 2017). The cAMP second messenger system plays a pivotal role in neuroplasticity and depression; in addition, studies have shown that antidepressant treatment can alter the expression of components of this signaling pathway in rodents (Popoli et al., 2000; Fujita et al., 2017). In this research, we found that GABA, lactic acid, and aminooxyacetic acid were significantly elevated in the hippocampus. Among these three metabolites, GABA and lactic acid can participate in the cAMP signaling pathway through binding and activation of GPCRs, and aminooxyacetic acid can inhibit GABA-T activity, leading to a reduction of GABA breakdown (Wallach, 1961). Moreover, GPCRs (including ADGRA1, ADGRV1, CELSR2, and S1PR5) previously reported to be implicated in the physiopathology and pharmacology of depression were also significantly altered (Tomita et al., 2013). Whereas previous studies observed decreased activation of adenylate cyclase and PKA in depression (Gould and Manji, 2002; Akin et al., 2005), in this study, we found a significant decrease of PPP1R13B and PPP2R2B, two phosphate family proteins that counterbalance the action of PKA (Fimia and Sassone-Corsi, 2001), in the hippocampus. Unexpectedly, but consistent with earlier researches, we did not observe changes in cAMP levels (Belmaker et al., 1980; Maj et al., 1984). However, changes in receptor-mediated cAMP formation have been studied in depression (Dwivedi and Pandey, 2008). These researches indicate PKA and associated cAMP signaling molecules may act as crucial neurobiological factors in depression (Dwivedi and Pandey, 2008).
Alterations of phosphoinositol signaling in the cerebra and peripheral tissues of depressed patients have been extensively reported, and studies have found that mood stabilizers (e.g., lithium, and valproate) seem to partially repair these abnormalities (Lenox et al., 1992; Kofman and Belmaker, 1993; Yuan et al., 2001; Gould and Manji, 2002). In this study, significantly altered metabolites and proteins (including myo-inositol, PLD2, and PIP4K2C) involved in phosphoinositol second messenger systems were observed. Myo-inositol is a by-product of membrane-bound phospholipid metabolism and an important player in the phosphoinositide secondary messenger pathway (Yildiz-Yesiloglu and Ankerst, 2006). A previous study reported that elevated levels of myo-inositol could arise from disturbances in the coupling metabolism of the receptor-second messenger system complex, thus providing an important biological substrate for the onset of depression (Hemanth Kumar et al., 2012). Protein PIP4K2C may play a critical influence in the generation of phosphatidylinositol bisphosphate, which is a precursor for second messengers of phosphoinositide signaling pathways (Itoh et al., 1998; Bulley et al., 2015). Meanwhile, PLD2 is an isoenzyme of PLD, which catalyzes the hydrolysis of phosphatidylcholine to generate an important lipid second messenger, phosphatidic acid (Kolesnikov et al., 2012; Zhu et al., 2018). These results are consistent with our previous plasma proteomic and metabolomic study, which found the dysregulation of phospholipid metabolism may aggravate inflammation in the central nervous system, ultimately leading to depression (Gui et al., 2018).
Small G proteins, which function as monomeric small GTPases, form one of the two classes of G proteins. In this study, elevated levels of three small GTPases, Rac1, RALB, and RAB4A, were observed. In addition, a significantly altered protein level of MAP3K5, a member of the MAP kinase kinase kinase family and part of the mitogen-activated protein kinase pathway, was observed. These factors are reportedly involved in signal transduction as second messengers for the tyrosine kinase system. AA, a polyunsaturated fatty acid that plays an important role in regulating inflammation signals and (possibly) vulnerability to depression, participated in cellular signaling as a lipid second messenger (Janssen-Timmen et al., 1994; Lotrich et al., 2013; Newton et al., 2016).
Furthermore, there are other metabolites and proteins involved in second messenger systems, including serine, O-phosphorylethanolamine, NAA, and protein PIK3R2. Serine and O-phosphorylethanolamine are involved in sphingolipid metabolism and sphingolipid signaling. In recent studies, sphingolipids (more specifically ceramides) were proposed to act as lipid second messengers in intracellular signaling pathways and participate in the induction of apoptosis in many cells activated by GPCR or stress stimuli (Venkataraman and Futerman, 2000; Guzmán et al., 2001; Newton et al., 2016). Recent reports suggest NAA is a main format of storage and transport of acetyl-coenzyme A in the neural system, where it is proposed to act as a second messenger relaying extracellular signals to the intracellular milieu (Ariyannur et al., 2010; Pietrocola et al., 2015). A recent research demonstrated that changes in the PI3K/AKT pathway may have specific therapeutic effects on depression (Kitagishi et al., 2012). In our study, we observed decreased PIK3R2, a regulatory subunit of PI3K, which belongs to the PI3K family. PI3Ks are enzymes that play an essential influence in lipid second messengers production and numerous biological reaction transduction.
There are some limitations of this study. First, the total number of included samples is relatively small, which may restrict interpretation of our results. Second, a number of low polarity metabolites are not detected by GC–MS. Therefore, GC–MS combined with other metabolomics approaches should be considered in future metabolomic studies. Third, iTRAQ and label-free, two quantitative proteomic analysis methods, have their own superiorities. The combination of these two methods can be complementary and should be considered in future studies. Fourth, proteomic results have not been validated by targeted methods. Thus, further researches are needed to validate these findings.
Conclusion
In summary, we applied complementary omics strategies (GC–MS-based metabolomics and iTRAQ-based proteomics) to comprehensively elucidate metabolite and protein alterations in the hippocampus of rat after CSDS. The results of this study suggest that disturbances in several different secondary messenger systems (cAMP, phosphoinositol, AA, and tyrosine kinase) are involved in the hippocampus of CSDS rodents. An imbalance of second messengers may be related to the physiopathology of depression. The results of our study contribute to a better understanding of biological mechanisms and physiopathology of depression, and may contribute to identify novel targets for antidepressant treatment.
Author Contributions
L-NY and PX conceived and designed the study. L-NY, J-CP, L-XL, X-YZ, Y-QZ, and Y-YL collected the data. L-NY, J-CP, and L-XL analyzed the data. L-NY wrote the first draft of the manuscript. L-NY, G-WW, and PX interpreted the data and wrote the final version. All authors approved final version of the manuscript.
Funding
This work was supported by the National Key Research and Development Program of China (Grant No. 2017YFA0505700), and the National Natural Science Foundation of China (Grant Nos. 8170051561 and 81873800). The funders had no role in the study design, data collection and analysis, decision to publish, or preparation of the manuscript.
Conflict of Interest Statement
The authors declare that the research was conducted in the absence of any commercial or financial relationships that could be construed as a potential conflict of interest.
Supplementary Material
The Supplementary Material for this article can be found online at: https://www.frontiersin.org/articles/10.3389/fnins.2019.00247/full#supplementary-material
FIGURE S1 | Results of Behavioral tests. (A) Body weight change between CSDS group (n = 9) and CON group (n = 8). (B) Sucrose preference changes between CSDS and CON groups. (C) Immobility time in the forced swim test. (D–F) Total distance, time spent in the inner squares, and rearing frequency in the open field test, respectively. (G–J) Open arms time, closed arms time, number of open arms entries, and number of closed arms entries in the elevated plus-maze, respectively. ∗P < 0.05.
TABLE S1 | Identified differential metabolites in the hippocampus between the CSDS and CON groups.
TABLE S2 | Identified differential proteins in hippocampus between the CSDS and CON groups.
TABLE S3 | Top ten canonical pathways by IPA analysis of integrated data from proteomics and metabolomics.
Abbreviations
AA, arachidonic acid; cAMP, cyclic adenosine monophosphate; CON, non-stress control; CSDS, chronic social defeat stress; EPM, elevated plus maze; FDR, false discovery rate; FST, forced swim test; GABA, 4-aminobutyric acid; GABA-T, 4-aminobutyrate aminotransferase; GC-MS, gas chromatography-mass spectrometry; GPCRs, G protein-coupled receptors; IPA, ingenuity pathway analysis; iTRAQ, isobaric tags for relative and absolute quantitation; LAT, locomotor activity test; LC–MS, liquid chromatography-mass spectrometry; LE, long-evans; MDD, major depressive disorder; NAA, N-acetyl-L-aspartic acid; NMR, nuclear magnetic resonance; OFT, open field test; OPLS-DA, orthogonal partial least-squares discriminant analysis; PCA, principal components analysis; PI3K, phosphoinositide-3-kinase; PKA, protein kinase A; PLD, phospholipase D; SD, sprague-dawley; SPT, sucrose preference test; VIP, variable importance on projection.
References
Akin, D., Manier, D. H., Sanders-Bush, E., and Shelton, R. C. (2005). Signal transduction abnormalities in melancholic depression. Int. J. Neuropsychopharmacol. 8, 5–16. doi: 10.1017/s146114570400478x
Ariyannur, P. S., Moffett, J. R., Manickam, P., Pattabiraman, N., Arun, P., Nitta, A., et al. (2010). Methamphetamine-induced neuronal protein NAT8L is the NAA biosynthetic enzyme: implications for specialized acetyl coenzyme A metabolism in the CNS. Brain Res. 1335, 1–13. doi: 10.1016/j.brainres.2010.04.008
Autry, A. E., Adachi, M., Nosyreva, E., Na, E. S., Los, M. F., Cheng, P. F., et al. (2011). NMDA receptor blockade at rest triggers rapid behavioural antidepressant responses. Nature 475, 91–95. doi: 10.1038/nature10130
Becker, C., Zeau, B., Rivat, C., Blugeot, A., Hamon, M., and Benoliel, J. J. (2008). Repeated social defeat-induced depression-like behavioral and biological alterations in rats: involvement of cholecystokinin. Mol. Psychiatry 13, 1079–1092. doi: 10.1038/sj.mp.4002097
Belmaker, R. H., Zohar, J., and Ebstein, R. P. (1980). Cyclic nucleotides in mental disorder. Adv. Cyclic Nucleotide Res. 12, 187–198.
Bulley, S. J., Clarke, J. H., Droubi, A., Giudici, M. L., and Irvine, R. F. (2015). Exploring phosphatidylinositol 5-phosphate 4-kinase function. Adv. Biol. Regul. 57, 193–202. doi: 10.1016/j.jbior.2014.09.007
Campbell, S., and Macqueen, G. (2004). The role of the hippocampus in the pathophysiology of major depression. J. Psychiatry Neurosci. 29, 417–426.
Cavill, R., Jennen, D., Kleinjans, J., and Briede, J. J. (2016). Transcriptomic and metabolomic data integration. Brief. Bioinform. 17, 891–901. doi: 10.1093/bib/bbv090
Chen, G., Yang, D., Yang, Y., Li, J., Cheng, K., Tang, G., et al. (2015). Amino acid metabolic dysfunction revealed in the prefrontal cortex of a rat model of depression. Behav. Brain Res. 278, 286–292. doi: 10.1016/j.bbr.2014.05.027
Christoffel, D. J., Golden, S. A., and Russo, S. J. (2011). Structural and synaptic plasticity in stress-related disorders. Rev. Neurosci. 22, 535–549. doi: 10.1515/rns.2011.044
Denmark, A., Tien, D., Wong, K., Chung, A., Cachat, J., Goodspeed, J., et al. (2010). The effects of chronic social defeat stress on mouse self-grooming behavior and its patterning. Behav. Brain Res. 208, 553–559. doi: 10.1016/j.bbr.2009.12.041
Du, H., Wang, K., Su, L., Zhao, H., Gao, S., Lin, Q., et al. (2016). Metabonomic identification of the effects of the Zhimu-Baihe saponins on a chronic unpredictable mild stress-induced rat model of depression. J. Pharm. Biomed. Anal. 128, 469–479. doi: 10.1016/j.jpba.2016.06.019
Dwivedi, Y., and Pandey, G. N. (2008). Adenylyl cyclase-cyclicAMP signaling in mood disorders: role of the crucial phosphorylating enzyme protein kinase A. Neuropsychiatr. Dis. Treat. 4, 161–176. doi: 10.2147/NDT.S2380
Fujita, M., Richards, E. M., Niciu, M. J., Ionescu, D. F., Zoghbi, S. S., Hong, J., et al. (2017). cAMP signaling in brain is decreased in unmedicated depressed patients and increased by treatment with a selective serotonin reuptake inhibitor. Mol. Psychiatry 22, 754–759. doi: 10.1038/mp.2016.171
Gould, T. D., and Manji, H. K. (2002). Signaling networks in the pathophysiology and treatment of mood disorders. J. Psychosom. Res. 53, 687–697. doi: 10.1016/S0022-3999(02)00426-9
Gui, S. W., Liu, Y. Y., Zhong, X. G., Liu, X., Zheng, P., Pu, J. C., et al. (2018). Plasma disturbance of phospholipid metabolism in major depressive disorder by integration of proteomics and metabolomics. Neuropsychiatr. Dis. Treat. 14, 1451–1461. doi: 10.2147/ndt.s164134
Guzmán, M., Galve-Roperh, I., and Sanchez, C. (2001). Ceramide: a new second messenger of cannabinoid action. Trends Pharmacol. Sci. 22, 19–22. doi: 10.1016/S0165-6147(00)01586-8
Harrison, P. J. (2002). The neuropathology of primary mood disorder. Brain 125, 1428–1449. doi: 10.1093/brain/awf149
Health TNIoM (2016). Depression. Available at: https://www.nimh. nih.gov/health/topics/depression/index.shtml [accessed September 28, 2018].
Hemanth Kumar, B. S., Mishra, S. K., Rana, P., Singh, S., and Khushu, S. (2012). Neurodegenerative evidences during early onset of depression in CMS rats as detected by proton magnetic resonance spectroscopy at 7 T. Behav. Brain Res. 232, 53–59. doi: 10.1016/j.bbr.2012.03.011
Itoh, T., Ijuin, T., and Takenawa, T. (1998). A novel phosphatidylinositol-5-phosphate 4-kinase (phosphatidylinositol-phosphate kinase IIgamma) is phosphorylated in the endoplasmic reticulum in response to mitogenic signals. J. Biol. Chem. 273, 20292–20299. doi: 10.1074/jbc.273.32.20292
Janssen-Timmen, U., Tomic, I., Specht, E., Beilecke, U., and Habenicht, A. J. (1994). The arachidonic acid cascade, eicosanoids, and signal transduction. Ann. N. Y. Acad. Sci. 733, 325–334. doi: 10.1111/j.1749-6632.1994.tb17282.x
Kim, S. Y., Lee, D. W., Kim, H., Bang, E., Chae, J. H., and Choe, B. Y. (2014). Chronic repetitive transcranial magnetic stimulation enhances GABAergic and cholinergic metabolism in chronic unpredictable mild stress rat model: (1)H-NMR spectroscopy study at 11.7T. Neurosci. Lett. 572, 32–37. doi: 10.1016/j.neulet.2014.04.033
Kitagishi, Y., Kobayashi, M., Kikuta, K., and Matsuda, S. (2012). Roles of PI3K/AKT/GSK3/mTOR pathway in cell signaling of mental illnesses. Depress. Res. Treat. 2012:752563. doi: 10.1155/2012/752563
Kofman, O., and Belmaker, R. H. (1993). Ziskind-Somerfeld Research Award 1993. Biochemical, behavioral, and clinical studies of the role of inositol in lithium treatment and depression. Biol. Psychiatry 34, 839–852. doi: 10.1016/0006-3223(93)90052-F
Koike, S., Bundo, M., Iwamoto, K., Suga, M., Kuwabara, H., Ohashi, Y., et al. (2014). A snapshot of plasma metabolites in first-episode schizophrenia: a capillary electrophoresis time-of-flight mass spectrometry study. Transl. Psychiatry 4:e379. doi: 10.1038/tp.2014.19
Kolesnikov, Y. S., Nokhrina, K. P., Kretynin, S. V., Volotovski, I. D., Martinec, J., Romanov, G. A., et al. (2012). Molecular structure of phospholipase D and regulatory mechanisms of its activity in plant and animal cells. Biochemistry 77, 1–14. doi: 10.1134/s0006297912010014
Lenox, R. H., Watson, D. G., Patel, J., and Ellis, J. (1992). Chronic lithium administration alters a prominent PKC substrate in rat hippocampus. Brain Res. 570, 333–340. doi: 10.1016/0006-8993(92)90598-4
Li, N., Lee, B., Liu, R. J., Banasr, M., Dwyer, J. M., Iwata, M., et al. (2010). mTOR-dependent synapse formation underlies the rapid antidepressant effects of NMDA antagonists. Science 329, 959–964. doi: 10.1126/science.1190287
Lindon, J. C., and Nicholson, J. K. (2008). Spectroscopic and statistical techniques for information recovery in metabonomics and metabolomics. Annu. Rev. Anal. Chem. 1, 45–69. doi: 10.1146/annurev.anchem.1.031207.113026
Liu, L., Zhou, X., Zhang, Y., Liu, Y., Yang, L., Pu, J., et al. (2016). The identification of metabolic disturbances in the prefrontal cortex of the chronic restraint stress rat model of depression. Behav. Brain Res. 305, 148–156. doi: 10.1016/j.bbr.2016.03.005
Liu, L., Zhou, X., Zhang, Y., Pu, J., Yang, L., Yuan, S., et al. (2018). Hippocampal metabolic differences implicate distinctions between physical and psychological stress in four rat models of depression. Transl. Psychiatry 8:4. doi: 10.1038/s41398-017-0018-1
Lotrich, F. E., Sears, B., and McNamara, R. K. (2013). Elevated ratio of arachidonic acid to long-chain omega-3 fatty acids predicts depression development following interferon-alpha treatment: relationship with interleukin-6. Brain Behav. Immun. 31, 48–53. doi: 10.1016/j.bbi.2012.08.007
Lucassen, P. J., Heine, V. M., Muller, M. B., van der Beek, E. M., Wiegant, V. M., De Kloet, E. R., et al. (2006). Stress, depression and hippocampal apoptosis. CNS Neurol. Disord. Drug Targets 5, 531–546. doi: 10.2174/187152706778559273
Ma, Y., Zhang, P., Wang, F., Liu, W., Yang, J., and Qin, H. (2012). An integrated proteomics and metabolomics approach for defining oncofetal biomarkers in the colorectal cancer. Ann. Surg. 255, 720–730. doi: 10.1097/SLA.0b013e31824a9a8b
Maj, M., Ariano, M. G., Arena, F., and Kemali, D. (1984). Plasma cortisol, catecholamine and cyclic AMP levels, response to dexamethasone suppression test and platelet MAO activity in manic-depressive patients. A longitudinal study. Neuropsychobiology 11, 168–173. doi: 10.1159/000118071
Mayr, M., Yusuf, S., Weir, G., Chung, Y. L., Mayr, U., Yin, X., et al. (2008). Combined metabolomic and proteomic analysis of human atrial fibrillation. J. Am. Coll. Cardiol. 51, 585–594. doi: 10.1016/j.jacc.2007.09.055
McEwen, B. S. (2010). Stress, sex, and neural adaptation to a changing environment: mechanisms of neuronal remodeling. Ann. N. Y. Acad. Sci. 1204(Suppl.), E38–E59. doi: 10.1111/j.1749-6632.2010.05568.x
McEwen, B. S., Eiland, L., Hunter, R. G., and Miller, M. M. (2012). Stress and anxiety: structural plasticity and epigenetic regulation as a consequence of stress. Neuropharmacology 62, 3–12. doi: 10.1016/j.neuropharm.2011.07.014
Monteggia, L. M., Gideons, E., and Kavalali, E. T. (2013). The role of eukaryotic elongation factor 2 kinase in rapid antidepressant action of ketamine. Biol. Psychiatry 73, 1199–1203. doi: 10.1016/j.biopsych.2012.09.006
Newton, A. C., Bootman, M. D., and Scott, J. D. (2016). Second messengers. Cold Spring Harb. Perspect. Biol. 8:a005926. doi: 10.1101/cshperspect.a005926
Niciu, M. J., Ionescu, D. F., Mathews, D. C., Richards, E. M., and Zarate, C. A. Jr. (2013). Second messenger/signal transduction pathways in major mood disorders: moving from membrane to mechanism of action, part I: major depressive disorder. CNS Spectr. 18, 231–241. doi: 10.1017/s1092852913000059
Odagaki, Y., Garcia-Sevilla, J. A., Huguelet, P., La Harpe, R., Koyama, T., and Guimon, J. (2001). Cyclic AMP-mediated signaling components are upregulated in the prefrontal cortex of depressed suicide victims. Brain Res. 898, 224–231. doi: 10.1016/S0006-8993(01)02188-6
Ota, K. T., Liu, R. J., Voleti, B., Maldonado-Aviles, J. G., Duric, V., Iwata, M., et al. (2014). REDD1 is essential for stress-induced synaptic loss and depressive behavior. Nat. Med. 20, 531–535. doi: 10.1038/nm.3513
Pietrocola, F., Galluzzi, L., Bravo-San Pedro, J. M., Madeo, F., and Kroemer, G. (2015). Acetyl coenzyme A: a central metabolite and second messenger. Cell Metab. 21, 805–821. doi: 10.1016/j.cmet.2015.05.014
Popoli, M., Brunello, N., Perez, J., and Racagni, G. (2000). Second messenger-regulated protein kinases in the brain: their functional role and the action of antidepressant drugs. J. Neurochem. 74, 21–33. doi: 10.1046/j.1471-4159.2000.0740021.x
Proitsi, P., Kim, M., Whiley, L., Pritchard, M., Leung, R., Soininen, H., et al. (2015). Plasma lipidomics analysis finds long chain cholesteryl esters to be associated with Alzheimer’s disease. Transl. Psychiatry 5:e494. doi: 10.1038/tp.2014.127
Radley, J. J., and Morrison, J. H. (2005). Repeated stress and structural plasticity in the brain. Ageing Res. Rev. 4, 271–287. doi: 10.1016/j.arr.2005.03.004
Ren, Y., Hao, P., Dutta, B., Cheow, E. S., Sim, K. H., Gan, C. S., et al. (2013). Hypoxia modulates A431 cellular pathways association to tumor radioresistance and enhanced migration revealed by comprehensive proteomic and functional studies. Mol. Cell. Proteomics 12, 485–498. doi: 10.1074/mcp.M112.018325
Shao, W. H., Chen, J. J., Fan, S. H., Lei, Y., Xu, H. B., Zhou, J., et al. (2015). Combined metabolomics and proteomics analysis of major depression in an animal model: perturbed energy metabolism in the chronic mild stressed rat cerebellum. OMICS 19, 383–392. doi: 10.1089/omi.2014.0164
Skelin, I., Kovacevic, T., Sato, H., and Diksic, M. (2011). Upregulated arachidonic acid signalling in the olfactory bulbectomized rat model of depression. Neurochem. Int. 58, 483–488. doi: 10.1016/j.neuint.2010.12.022
Sousa, N., Lukoyanov, N. V., Madeira, M. D., Almeida, O. F., and Paula-Barbosa, M. M. (2000). Reorganization of the morphology of hippocampal neurites and synapses after stress-induced damage correlates with behavioral improvement. Neuroscience 97, 253–266. doi: 10.1016/S0306-4522(00)00050-6
Taurines, R., Dudley, E., Grassl, J., Warnke, A., Gerlach, M., Coogan, A. N., et al. (2011). Proteomic research in psychiatry. J. Psychopharmacol. 25, 151–196. doi: 10.1177/0269881109106931
Tomita, H., Ziegler, M. E., Kim, H. B., Evans, S. J., Choudary, P. V., Li, J. Z., et al. (2013). G protein-linked signaling pathways in bipolar and major depressive disorders. Front. Genet. 4:297. doi: 10.3389/fgene.2013.00297
Venkataraman, K., and Futerman, A. H. (2000). Ceramide as a second messenger: sticky solutions to sticky problems. Trends Cell Biol. 10, 408–412. doi: 10.1016/S0962-8924(00)01830-4
Vyas, A., Mitra, R., Shankaranarayana Rao, B. S., and Chattarji, S. (2002). Chronic stress induces contrasting patterns of dendritic remodeling in hippocampal and amygdaloid neurons. J. Neurosci. 22, 6810–6818. doi: 10.1523/JNEUROSCI.22-15-06810.2002
Wachtel, H. (1989). Dysbalance of neuronal second messenger function in the aetiology of affective disorders: a pathophysiological concept hypothesising defects beyond first messenger receptors. J. Neural Transm. 75, 21–29. doi: 10.1007/BF01250641
Wachtel, H. (1990). The second-messenger dysbalance hypothesis of affective disorders. Pharmacopsychiatry 23, 27–32. doi: 10.1055/s-2007-1014478
Wallach, D. P. (1961). Studies on the GABA pathway. I. The inhibition of gamma-aminobutyric acid-alpha-ketoglutaric acid transaminase in vitro and in vivo by U-7524 (amino-oxyacetic acid). Biochem. Pharmacol. 5, 323–331. doi: 10.1016/0006-2952(61)90023-5
Wang, W., Guo, H., Zhang, S. X., Li, J., Cheng, K., Bai, S. J., et al. (2016). Targeted metabolomic pathway analysis and validation revealed glutamatergic disorder in the prefrontal cortex among the chronic social defeat stress mice model of depression. J. Proteome Res. 15, 3784–3792. doi: 10.1021/acs.jproteome.6b00577
Wesseling, H., Chan, M. K., Tsang, T. M., Ernst, A., Peters, F., Guest, P. C., et al. (2013). A combined metabonomic and proteomic approach identifies frontal cortex changes in a chronic phencyclidine rat model in relation to human schizophrenia brain pathology. Neuropsychopharmacology 38, 2532–2544. doi: 10.1038/npp.2013.160
World Health Organization [WHO] (2017). Depression and Other Common Mental Disorders: Global Health Estimates. Geneva: WHO Press.
Yildiz-Yesiloglu, A., and Ankerst, D. P. (2006). Neurochemical alterations of the brain in bipolar disorder and their implications for pathophysiology: a systematic review of the in vivo proton magnetic resonance spectroscopy findings. Prog. Neuropsychopharmacol. Biol. Psychiatry 30, 969–995. doi: 10.1016/j.pnpbp.2006.03.012
Yuan, P. X., Huang, L. D., Jiang, Y. M., Gutkind, J. S., Manji, H. K., and Chen, G. (2001). The mood stabilizer valproic acid activates mitogen-activated protein kinases and promotes neurite growth. J. Biol. Chem. 276, 31674–31683. doi: 10.1074/jbc.M104309200
Zhang, Y., Yuan, S., Pu, J., Yang, L., Zhou, X., Liu, L., et al. (2018). Integrated metabolomics and proteomics analysis of hippocampus in a rat model of depression. Neuroscience 371, 207–220. doi: 10.1016/j.neuroscience.2017.12.001
Zheng, P., Chen, J. J., Huang, T., Wang, M. J., Wang, Y., Dong, M. X., et al. (2013a). A novel urinary metabolite signature for diagnosing major depressive disorder. J. Proteome Res. 12, 5904–5911. doi: 10.1021/pr400939q
Zheng, P., Wang, Y., Chen, L., Yang, D., Meng, H., Zhou, D., et al. (2013b). Identification and validation of urinary metabolite biomarkers for major depressive disorder. Mol. Cell. Proteomics 12, 207–214. doi: 10.1074/mcp.M112.021816
Zheng, P., Chen, J. J., Zhou, C. J., Zeng, L., Li, K. W., Sun, L., et al. (2016a). Identification of sex-specific urinary biomarkers for major depressive disorder by combined application of NMR- and GC-MS-based metabonomics. Transl. Psychiatry 6:e955. doi: 10.1038/tp.2016.188
Zheng, P., Zeng, B., Zhou, C., Liu, M., Fang, Z., Xu, X., et al. (2016b). Gut microbiome remodeling induces depressive-like behaviors through a pathway mediated by the host’s metabolism. Mol. Psychiatry 21, 786–796. doi: 10.1038/mp.2016.44
Zhou, J., Liu, Z., Yu, J., Han, X., Fan, S., Shao, W., et al. (2015). Quantitative proteomic analysis reveals molecular adaptations in the hippocampal synaptic active zone of chronic mild stress-unsusceptible rats. Int. J. Neuropsychopharmacol. 19:pyv100. doi: 10.1093/ijnp/pyv100
Zhou, X., Liu, L., Lan, X., Cohen, D., Zhang, Y., Ravindran, A. V., et al. (2018). Polyunsaturated fatty acids metabolism, purine metabolism and inosine as potential independent diagnostic biomarkers for major depressive disorder in children and adolescents. Mol. Psychiatry doi: 10.1038/s41380-018-0047-z [Epub ahead of print].
Keywords: depression, social defeat, proteomics, metabolomics, second-messenger
Citation: Yang L-N, Pu J-C, Liu L-X, Wang G-W, Zhou X-Y, Zhang Y-Q, Liu Y-Y and Xie P (2019) Integrated Metabolomics and Proteomics Analysis Revealed Second Messenger System Disturbance in Hippocampus of Chronic Social Defeat Stress Rat. Front. Neurosci. 13:247. doi: 10.3389/fnins.2019.00247
Received: 19 November 2018; Accepted: 01 March 2019;
Published: 22 March 2019.
Edited by:
Firas H. Kobeissy, University of Florida, United StatesReviewed by:
Oksana Sorokina, University of Edinburgh, United KingdomYing Xu, University at Buffalo, United States
Copyright © 2019 Yang, Pu, Liu, Wang, Zhou, Zhang, Liu and Xie. This is an open-access article distributed under the terms of the Creative Commons Attribution License (CC BY). The use, distribution or reproduction in other forums is permitted, provided the original author(s) and the copyright owner(s) are credited and that the original publication in this journal is cited, in accordance with accepted academic practice. No use, distribution or reproduction is permitted which does not comply with these terms.
*Correspondence: Peng Xie, xiepeng@cqmu.edu.cn
†These authors have contributed equally to this work