- 1Laboratoire Parcours Santé Systémique (EA4129), Université Claude Bernard-Lyon 1 & Centre PSYRENE, Lyon, France
- 2CREATIS (CNRS UMR5220 & INSERM U1206), Université Claude Bernard-Lyon 1, Villeurbanne, France
- 3Faculty of Medicine, Abu-Haidar Neuroscience Institute, American University of Beirut, Beirut, Lebanon
- 4Service de Psychopathologie du Développement, Hôpital Femme-Mère-Enfant, Hospices Civils de Lyon, Bron, France
- 5Service de Psychopathologie de l'Enfant et de l'Adolescent, Hôpital Neurologique, Hospices Civils de Lyon, Bron, France
- 6CERMEP—Imagerie du Vivant, Université de Lyon, Bron, France
Objectives: The main goal of this study was to investigate and compare the neural substrate of two children's profiles of high intelligence quotient (HIQ).
Methods: Two groups of HIQ children were included with either a homogeneous (Hom-HIQ: n = 20) or a heterogeneous IQ profile (Het-HIQ: n = 24) as defined by a significant difference between verbal comprehension index and perceptual reasoning index. Diffusion tensor imaging was used to assess white matter (WM) microstructure while tract-based spatial statistics (TBSS) analysis was performed to detect and localize WM regional differences in fractional anisotropy (FA), mean diffusivity, axial (AD), and radial diffusivities. Quantitative measurements were performed on 48 regions and 21 fiber-bundles of WM.
Results: Hom-HIQ children presented higher FA than Het-HIQ children in widespread WM regions including central structures, and associative intra-hemispheric WM fasciculi. AD was also greater in numerous WM regions of Total-HIQ, Hom-HIQ, and Het-HIQ groups when compared to the Control group. Hom-HIQ and Het-HIQ groups also differed by their hemispheric lateralization in AD differences compared to Controls. Het-HIQ and Hom-HIQ groups showed a lateralization ratio (left/right) of 1.38 and 0.78, respectively.
Conclusions: These findings suggest that both inter- and intra-hemispheric WM integrity are enhanced in HIQ children and that neural substrate differs between Hom-HIQ and Het-HIQ. The left hemispheric lateralization of Het-HIQ children is concordant with their higher verbal index while the relative right hemispheric lateralization of Hom-HIQ children is concordant with their global brain processing and adaptation capacities as evidenced by their homogeneous IQ.
Introduction
Children with very high intelligence quotient (HIQ) [superior to 130 as measured by the fourth version of the Wechsler Intelligence Scale for Children (WISC-IV) (Wechsler, 2005)] are referred to as “gifted,” “talented,” as well as “high potential.” However, these terms imply different characteristics and conceptions that remain misunderstood. Qualitatively, HIQ children are perceived as having all or part of the following characteristics: faster processing speed based on a rich vocabulary range, greater attention, and visuo-spatial abilities, fast and large memory, superior problem solving capacities, mental flexibility, and reasoning strategies (Vaivre-Douret, 2011). Nonetheless, these exceptional characteristics are not always associated with learning or educational achievements. This paradox was investigated during the last decades but remains misunderstood (Barchmann and Kinze, 1990; Reis and McCoach, 2000).
Although gifted in certain areas of the curriculum, some HIQ children may present different disabilities in reading, writing, coordination, attention, and/or managing their emotions and relationships. Indeed, many HIQ children cases are referred to pediatric or child neuropsychiatry departments for socio-emotional problems such as anxiety, social withdrawal, low self-esteem, excessive perfectionism (Guignard et al., 2012), deficit of attention and/or school underachievement (Guénolé et al., 2015). In contrast, other HIQ children, corresponding better to the “talented” or “gifted” appellation, seem to be well adapted to their environment. These clinical observations have led us to define two profiles of HIQ children. On one hand, the homogeneous HIQ profile (Hom-HIQ) usually presents quite well-controlled behavior and successful curriculum. On the other hand, the heterogeneous HIQ profile (Het-HIQ) can show emotional and social maladjustment as well as learning troubles that can be related to a “dyssynchrony syndrome” (Terrassier, 2009; Guénolé et al., 2015). Such heterogeneity, also called “developmental asynchrony” by Alsop (2003) and Silverman (2011), can be detected by the Wechsler's IQ test. Indeed, a significant difference between verbal comprehension index (VCI) and perceptual reasoning index (PRI) values of WISC-IV as well as standard levels in working memory index (WMI) and processing speed index (PSI) are considered as significant markers indicative of a heterogeneous profile (Berk, 1982; Bessou et al., 2005; Sweetland et al., 2006; Guénolé et al., 2013).
Diffusion tensor imaging (DTI) is a unique non-invasive technique that provides microstructural information related to white matter (WM) integrity (Basser et al., 1994; Basser and Jones, 2002) and allows the identification of neural pathways (Beaulieu, 2002). In addition to various clinical applications, DTI has been used to study normal development of WM that is thought to play a key role in cognitive functions (Qiu et al., 2008; Lenroot and Giedd, 2010; Schmithorst and Yuan, 2010). Indeed, as distant brain regions become more efficiently interconnected, it is expected that the ability to transfer and analyze information also becomes more efficient. Thus, the high sensitivity of DTI to detect WM changes has led to recent investigations of neural connections in children and adolescents with specific abilities such as arithmetic (Tsang et al., 2009; Van Beek et al., 2014), executive functions (Seghete et al., 2013) as well as general intelligence (Schmithorst et al., 2005; Gläscher et al., 2010; Barbey et al., 2013; Dunst et al., 2014). Particularly, Clayden et al. (2012) demonstrated that increased anisotropy in the splenium of the corpus callosum and in the left inferior longitudinal and arcuate fasciculi positively predicted intelligence. Navas-Sánchez et al. (2014) also reported a positive correlation between fractional anisotropy (FA) values and intellectual capabilities in the corpus callosum. Overall, these findings demonstrate that intra-hemispheric long tracts connecting fronto-occipital and temporo-parietal regions as well as inter-hemispheric fibers are crucial for the integration of information.
The objective of this study was to identify and characterize WM microstructural differences between two HIQ children profiles in comparison with age-matched control subjects. To this end, tract-based spatial statistic (TBSS) was used to perform automated analysis of WM integrity (Smith et al., 2006). FA constitutes a sensitive marker of microstructural changes. Mean diffusivity (MD) as well as the axial (AD) and radial (RD) diffusivities (Tournier et al., 2011), allow a better interpretation of potential changes in terms of axonal integrity and myelination (Song et al., 2002).
We first hypothesized that HIQ children would show greater global WM integrity related to their general intelligence, when compared to control subjects. Second, the comparison of two profiles of HIQ children, namely Hom-HIQ, and Het-HIQ based on their PRI differences, would show local WM changes, potentially linked to their verbal and/or visuo-spatial abilities.
Materials and Methods
Subjects
Participants in this study were children followed either at the children psychiatry department of Lyon's Neurological Hospital or the PSYRENE Center, both specialized in HIQ evaluation and psychological follow-up. Children were also recruited by placing ads in public schools (mostly for the recruitment of control subjects). Prior to enrollment, all children received a medical examination and were fully informed along with their parents of the study details. Children with any neurological, psychiatric disorders, learning disabilities such as dyslexia or dyspraxia, or MRI contra-indications were excluded from the study. None of the children received any medical treatments. Ethical committee approval (“CPP Sud-Est IV”) and written informed consent from the children and their parents were obtained. Three other subjects were excluded from this study: one for his inability to perform the MRI exam and two for motion artifacts.
All children underwent the WISC-IV with its four subscales [Verbal comprehension index (VCI), Perceptual reasoning index (PRI), Working memory index (WMI), and Processing speed index (PSI)] to calculate the FSIQ. Children scoring 130 or greater in VCI or PRI were included in HIQ groups. Children with a VCI significantly higher than the PRI were included in the Het-HIQ group, while those with no significant difference between VCI and PRI were included in the Hom-HIQ group. The significance of VCI-PRI differences was defined by the WISC-IV score with a range of 15–46 in the Het-HIQ group. Since the number of Het-HIQ children with significantly higher PRI than VCI was under represented (3 subjects out of 27), these subjects were excluded for group homogeneity purposes.
A total of 44 right-handed (according to the Edinburgh handedness scale Oldfield, 1971) HIQ children were included in this study. The study consisted of 8 girls and 36 boys aged between 8 and 12 years old [mean (± SD) age = 10.4 ± 1.3 y]. The Hom-HIQ group was composed of 20 children [5 girls and 15 boys; mean (±SD) age = 10.2 ± 1.2 y] with a FSIQ of 139.9 ± 11.1. The Het-HIQ group was composed of 24 children [3 girls and 21 boys, mean (±SD) age = 10.5 ± 1.4 y] with a FSIQ of 129.4 ± 10.6. Thirteen age-matched subjects [6 girls and 7 boys, mean (±SD) age = 10.5 ± 1.2 y] with a FSIQ of 105.2 ± 8.8 served as control subjects. Except for the two subjects excluded for motion artifacts, the quality of MRI data was optimal.
Behavioral Assessment
All children were assessed using the adapted French version of the Child Behavior Checklist (CBCL) for children from ages 4 to 16 (Achenbach, 1991). The CBCL is a 113-item parental report inventory that uses a Likert response format (not true, somewhat true, very true) for a variety of behaviors. The total Competence score was obtained from three subscales: activities, social, and school. The total Problem score was obtained from eight subscales: (I) withdrawn, (II) somatic complaints, (III) anxious, (IV) social, (V) thought, and (VI) attention problems, (VII) delinquent and (VIII) aggressive behavior. Two other subtotal scores, internal and external, were obtained from subscales (I+II+III) and (VII+VIII), respectively. Total scores were calculated by summing items, which was transformed by square root to approximate a normal distribution. Individual raw scores were converted into T-scores.
The Conners' Parent Rating Scale (CPRS) (Conners et al., 1998) was used to assess symptoms of Attention Deficit Hyperactivity Disorder (ADHD), and included six subscales: hyperactivity/impulsivity, psychosomatic, learning problems, anxiety, and conduct disorder. Individual raw scores were converted into T-scores.
Image Acquisition
MRI examinations were performed without any sedation at the MRI department of CERMEP-Imagerie du Vivant, on a 1.5T Siemens Sonata system (Erlangen, Germany) with an 8-channel head-coil and 40 mT/m gradients. The MRI protocol included a 3D T1-weighted magnetization prepared rapid gradient echo (MPRAGE) sequence (time of repetition/time of echo/time for inversion [TR/TE/TI] = 1,970/3.93/1,100 ms; flip angle = 15°; matrix size = 256 × 256; field of view = 256 × 256 mm; slice thickness = 1 mm; voxel size = 1 × 1 × 1 mm3; acquisition time = 8 min).
DTI protocol was based on a 2D multi-slice spin-echo echo-planar imaging (EPI) sequence (TR/TE = 6,900/86 ms, matrix size = 96 × 96, FOV = 240 × 240 mm, acquisition time = 7 min). Fifty-one contiguous axial slices, of 2.5 mm thickness were acquired in the anterior commissure—posterior commissure (AC-PC) plane. Twenty-four diffusion gradient directions (b = 1,000 s/mm2) were applied with a nominal isotropic resolution of 2.5 mm3. The b0 image was acquired four times to increase signal to noise ratio while the other directions were acquired twice. This protocol follows international recommendations on DTI acquisition (Jones, 2004).
Both T1-weighted, and DTI data were visually inspected (by S.H, D.S-M, and O.R) in order to detect artifacts arising from subject motion or scanner malfunction, and confirm the lack of visually detectable abnormalities.
Data Processing
Analysis of diffusion tensor data was performed using the Functional Magnetic Resonance Imaging of the Brain (FMRIB) Software Library (FSL) (Smith et al., 2004). Eddy current correction using FMRIB's Diffusion Toolbox (FDT) was first applied followed by a non-brain voxels extraction using the Brain Extraction Tool (BET) with a factor of 0.35. FA and MD as well as AD and RD maps were then generated. Individual DTI maps were visually inspected for the presence of significant residual motion or other artifacts.
TBSS analysis of FA maps first consisted in aligning all subjects' data into a common space by means of a nonlinear registration. Since children brain anatomy is slightly different from adult's, the common template used in FSL was thereby not adapted for our subjects. Therefore, all subjects' FA images were aligned to every other one in order to find the most “typical” subject as a target image to align all other. A mean FA image was then created and used to obtain a mean skeleton of the major WM tracts on which all aligned subjects FA data were then projected. The resulting data were next fed into a voxel-wise statistical analysis, performed to identify FA differences in areas between HIQ and Control subjects, and between Hom-HIQ and Het-HIQ groups, including age and sex as covariates. For a better assessment, TBSS was also applied to diffusion-derived data other than FA. The nonlinear warps and skeleton projection were therefore applied to AD, RD, and MD maps. The resulting warped maps were then merged and projected onto the original mean FA skeleton. Voxel-wise analysis was finally performed exactly as for the FA maps.
The employed voxel-wise analysis was based on a non-parametric approach using the permutation test theory with a standard generalized linear model design matrix. By allowing inference on the statistical maps when the null distribution is not known, this approach provides an easily implementable solution to the multiple testing problems. The permutation testing was performed using the Randomise module of FSL. Group comparisons were performed between each group of HIQ children and control subjects with age and gender as co-factors. The threshold-free cluster enhancement (TFCE) option of Randomise was applied on the resulting statistical maps. This method enhances cluster-like structures in an image without having to define an initial cluster-forming threshold or to carry out a large amount of data smoothing. The resulting statistical parameter maps were corrected for sex and age and for multiple comparisons by the family-wise error rate (FEW-corrected p < 0.05). The anatomical location of significant clusters was identified based on WM atlases (JHU ICBM-DTI81 White Mater Labels and JHU White-Matter Tractography Atlas) in FSL. In order to confirm the voxel-wise analysis results, quantitative values of diffusion metrics were extracted from 48 regions of interest (ROI) and 21 fiber-bundles of the JHU atlases, by multiplying atlas labels with the TBSS skeleton obtained from all 57 subjects.
Statistical analysis was performed using the R library (https://www.r-project.org). ANOVA followed by Tukey's post-hoc tests were performed on demographic data, IQ, CBCL, and CPRS scores, and DTI metrics (ROI and fiber bundles) to detect group-wise differences between the four children groups (Total-HIQ, Hom-HIQ, Het-HIQ, and Control) with age, gender and CBCL as co-factors.
Results
Descriptive statistics of age and IQ scores are reported in Table 1. As groups were age-matched, there were no significant differences in age between any of the HIQ groups and the Control group. PRI values were significantly lower in Het-HIQ compared to Hom-HIQ groups, whereas no significant differences were observed for VCI values. As reported in Table 2, no significant differences were found in mean CBCL and CPRS T-scores when comparing HIQ and Control groups. However, some differences were found using an ANOVA test between mean Het-HIQ and Hom-HIQ groups in two CBCL subscales (I and V), namely the withdrawn (p = 0.07) and thought (p = 0.03) problems, as reported in Table 2.
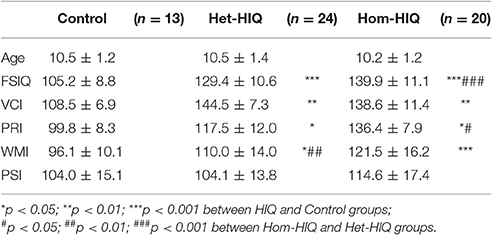
Table 1. Descriptive statistics (Mean ± SD) in Control, heterogeneous-HIQ (Het-HIQ) and homogeneous-HIQ (Hom-HIQ) groups.

Table 2. Normalized T-scores (Mean ± SD) measured in Competence and Problem subscales of the Child Behavior Checklist (CBCL) and in Conners' Parent Rating Scale (CPRS) questionnaires in Control, heterogeneous-HIQ (Het-HIQ) and homogeneous-HIQ (Hom-HIQ) groups.
Voxel-Wise Analysis
Figures 1, 2 illustrate the significant AD and FA differences observed when comparing HIQ and Control groups. No significant differences were reported for MD and RD diffusion metrics in any comparison.
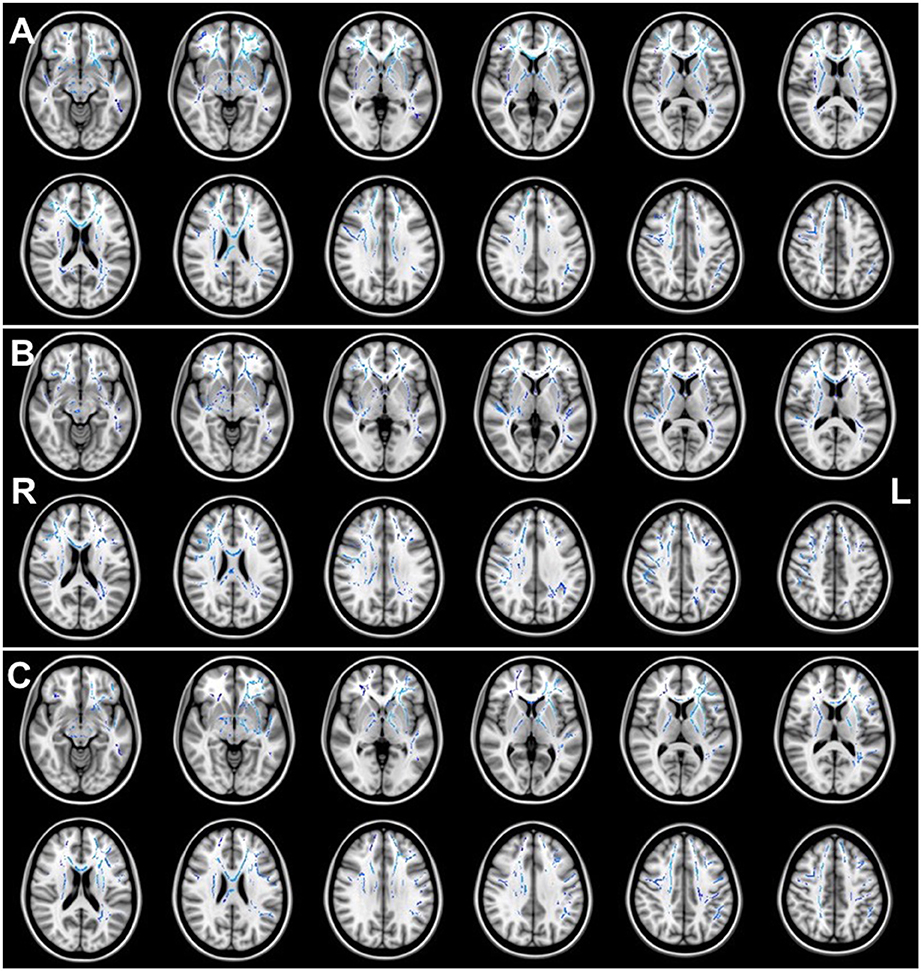
Figure 1. Regions of significant (p < 0.05) greater AD (blue) when comparing (A) the Total-HIQ group (Tot-HIQ), (B) the homogeneous-HIQ (Hom-HIQ) group and (C) the heterogeneous-HIQ (Het-HIQ) group with the Control group.
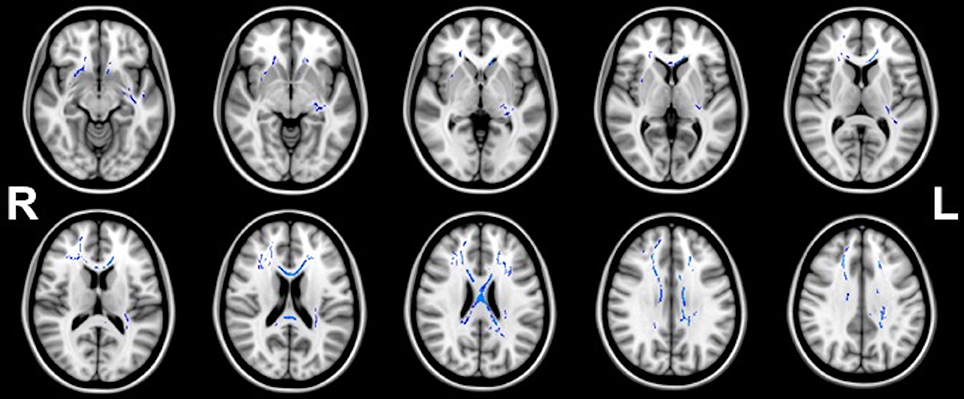
Figure 2. Regions (blue) of significant (p < 0.05) greater FA in homogeneous-HIQ (Hom-HIQ) compared to heterogeneous-HIQ (Het-HIQ) children.
Axial Diffusivity (AD)
When compared to the Control group, TBSS analysis showed significant higher AD values in Total-HIQ, Hom-HIQ, and Het-HIQ groups as illustrated in Figure 1. For quantification purposes, the percentage of significant voxels was calculated in each ROI of the ICBM-DTI81 JHU atlas (Table 3). The three groups showed a large number of significant voxels (above 50% of ROI volume) in many WM regions, including mainly the central structures such as the fornix and the corpus callosum as well as the brainstem (cerebral peduncles and medial lemnisci) and cerebellar structures (cerebellar peduncles). In the corpus callosum, a decreasing gradient was observed from genu to splenium that was slightly more pronounced in the Het-HIQ group. The larger changes included different regions of the corona radiata and the capsule as well as different parts of WM fasciculi, namely the uncinate (UNC), the superior fronto-occipital (SFO), the posterior thalamic radiations (PTR), the cortico-spinal tract (CST) and the superior longitudinal (SLF) (Table 3).
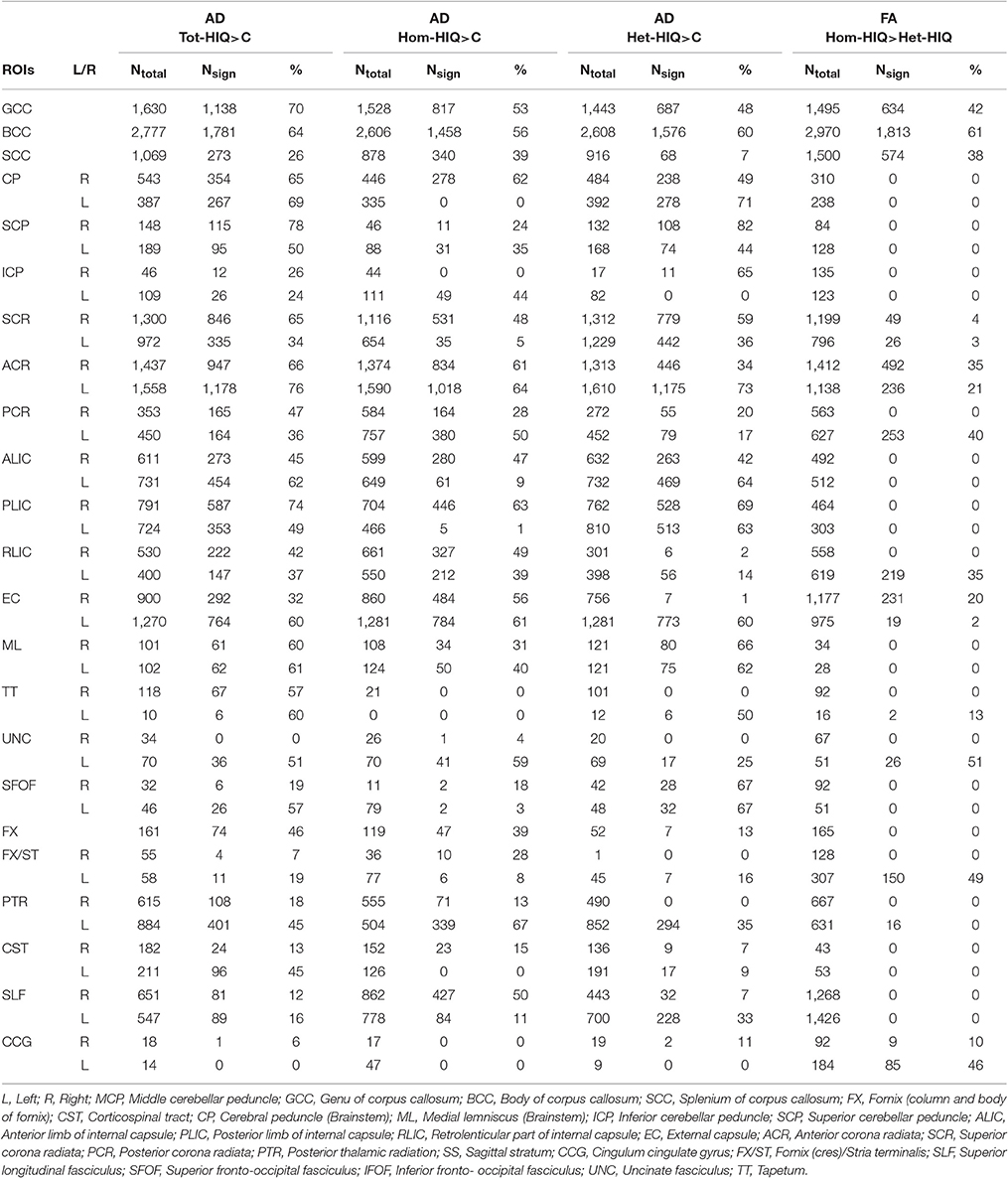
Table 3. Number of significant voxels (Nsign) and percentage (%) of significant voxels per total number of voxels (Ntotal) per ROI of the JHU atlas (ICBM-DTI81 White Matter Labels) with significant changes in AD values when comparing Total-HIQ (Tot-HIQ), homogeneous-HIQ (Hom-HIQ) and heterogeneus-HIQ (Het-HIQ) groups with the Control (C) group, and in FA values when comparing Hom-HIQ and Het-HIQ groups.
When comparing the localization of these AD increases, we observed a significant difference in hemispheric lateralization. As illustrated in Figure 1, 56% of significant voxels were found in the right hemisphere of Hom-HIQ group while 58% were found in the left hemisphere of Het-HIQ, leading to a left/right ratio of 0.78 and 1.38 in Hom-HIQ and Het-HIQ groups, respectively.
Fractional Anisotropy (FA)
TBSS analysis showed significant higher FA values in Hom-HIQ compared to Het-HIQ groups (Figure 2). FA differences were observed mainly in the corpus callosum and several central regions such as the anterior and posterior parts of the corona radiata (CR), and the internal and external capsules. Further, several parts of WM fasciculi such as the left uncinate (UNC), the left fornix stria terminalis (FX/ST) and the cingulum cingulate gyrus (CCG) were significantly detected. No significant decreases of FA were observed.
Quantitative Analysis
Based on the JHU atlases, the diffusion metrics were measured in different ROIs (ICBM DTI-81) and fiber-bundles (White-Matter Tractography) in HIQ and Control groups to confirm our voxel-wise findings. When comparing Hom-HIQ and Het-HIQ groups, FA values were significantly increased in several ROIs, the body of corpus callosum, the left inferior cerebellar peduncle (ICP), the left posterior corona radiata (PCR), and the right fornix stria terminalis (FX/ST), and in one fiber bundle, the cingulum cingulate gyrus (CCG). When comparing with the Control group, AD values were significantly increased in 17 ROIs and six fiber bundles of the Tot-HIQ group, in four ROIs and three fiber bundles of the Hom-HIQ group, and four ROIs and two fiber bundles of the Het-HIQ group, as reported in Table 4.
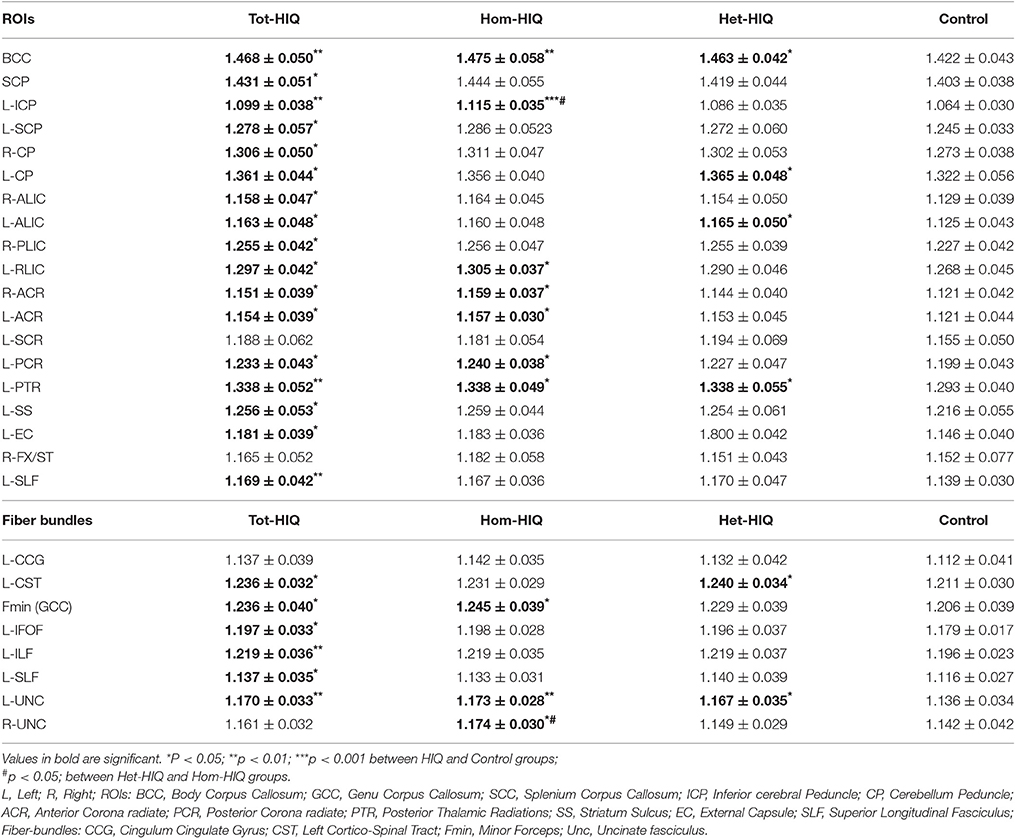
Table 4. Axial diffusivity (AD) values (Mean ± SD) in selected ROIs (ICBM-DTI81 White Matter Labels) and fiber-bundles (White-Matter Tractography) of JHU atlases applied to Control, heterogeneous-HIQ (Het-HIQ), homogeneous-HIQ (Hom-HIQ), and Total-HIQ (Tot-HIQ) groups.
Effects of full-scale IQ, and CBCL score (Achenbach) on AD values were estimated using General linear models for WM region reported in Table 4. The relative importance of these predictors was estimated for each WM region. A mean value of 77.88% (78.21% for JHU and 77.74% for ROI) and 22.12% (21.79% for JHU and 22.26% for ROI) was obtained for the full-scale IQ and CBCL score respectively. These results showed that differences observed in WM between HIQ and Control groups are mainly driven by IQ.
Discussion
In this work, TBSS analysis was performed to investigate the diffusion-related differences in HIQ children, and between two groups of HIQ, based on their IQ profile, namely homogeneous and heterogeneous. To our knowledge, this work is the first to investigate WM microarchitecture in these two profiles of HIQ children.
Global Changes with Intelligence
When compared to the Control group, both HIQ groups presented greater AD and FA in widespread WM regions of frontal, central and associative pathways. Central regions included mainly the body and the genu of the corpus callosum, the fornix and the cingulum as well as brainstem and cerebellar regions. These findings are in agreement with previous DTI studies on intelligence showing a bilateral fronto-parietal network as a neural substrate of enhanced information processing and intelligence (Dunst et al., 2014; Navas-Sánchez et al., 2014). Similarly, a previous fMRI study demonstrated the use of more extensive and bilateral cortical connections in gifted adolescents (Desco et al., 2011). Such improvement of bilateral and frontal WM organization contributes to improved cognitive performance in working memory, fluency, and executive functioning such as inhibition and task-switching (Seghete et al., 2013). Other studies suggested that corpus callosum related changes are crucial for higher cognitive capabilities (Singh and O'Boyle, 2004; Hutchinson et al., 2009; Prescott et al., 2010). Also, it has been demonstrated that the genu (Sidtis et al., 1981) and the splenium (Funnell et al., 2000) are involved in high order transfer of semantic information. In addition, the higher FA in the fornix may reflect a greater episodic memory (Gaffan and Wilson, 2008). Projection fibers of the capsule and the corona radiata also showed higher FA. These fibers regroup ascending fibers from the thalamus and descending fibers from the fronto-parietal cortex to subcortical nuclei. They constitute the backbone of perceptual and motor functions and other cognitive functions (Schmahmann and Pandya, 2008). Additionally, our study showed greater FA and AD in other regions of associative WM fasciculi, namely the uncinate, the superior longitudinal (SLF) and the superior fronto-occipital (SFOF). The SLF supports bidirectional networks involved in high order cognitive executive functions such as attention, inhibition as well as memory and language (Mesulam, 1998) while the uncinate (UNC) fasciculus may play a prominent role in semantic tasks (Catani and Mesulam, 2008; Catani et al., 2013). Thus, these findings show that both inter- and intra-hemispheric connections are enhanced in HIQ children suggesting that they may benefit from a better WM integrity, providing higher performances in most abilities.
Local Changes with HIQ Profiles
When comparing Hom-HIQ and Het-HIQ groups to the Control group, we found AD increases in numerous WM regions. However, their spatial distribution was clearly different between the two HIQ groups. The Het-HIQ group showed more AD changes in the left hemisphere in contrast to the Hom-HIQ group which showed more AD differences in the right hemisphere.
The left hemisphere is well known to play a major role in lingual functions (Szaflarski et al., 2012) with a strong involvement of the SLF, the SFOF, and UNC fasciculi (Catani et al., 2002). In Het-HIQ group, AD is only significantly increased in the left UNC fasciculus while in Hom-HIQ group, it is also increased the right UNC, which is involved in emotion processing and memory, and plays an important role in lexical retrieval, semantic associations and naming aspects of the language (Grossman and Ash, 2004; Agosta et al., 2010; Galantucci et al., 2011). Thus, the left lateralization of increased diffusivity observed in Het-HIQ children can be associated to their high language performances (high VCI) in contrast to their relatively low visuo-spatial capacities (low PRI). Indeed, the right hemisphere is more specialized in visuo-spatial capacities such as facial recognition and attention monitoring (Gazzaniga, 2000; de Schotten et al., 2011; Caeyenberghs and Leemans, 2014).
Recently, Marinsek et al. (2014) proposed that both hemispheres diverge by their reasoning strategies. On one hand, the left hemisphere is considered as an interpreter, tending to reduce uncertainty by creating explanations, filling gaps of information, and making inference (Braun and Suffren, 2011). On the other hand, the right hemisphere is considered as a realist, striving to reduce inconsistencies between hypotheses and reality by detecting conflicts, updating information, supporting mental set shifting (Goel et al., 2013), and monitoring and inhibiting thoughts and behavior (Chatham et al., 2012). Moreover, Vendetti et al. (2015) found hemispheric differences in relational reasoning. Indeed, the left hemisphere has been shown to excel in relational encoding, particularly when the information is ordered linearly. It interacts more with itself, whereas the right hemisphere demonstrates connectivity patterns associated with both hemispheres (Gotts et al., 2013), and acts more as a monitor of evaluation and inhibition.
However, the complementarity or independence of hemispheric specialization remains an open question (Badzakova-Trajkov et al., 2016). The causal hypothesis suggests that strengths in language and weaknesses in visuo-spatial abilities of Het-HIQ may result from an earlier development of language, which thereby reinforces a hemispheric asymmetry specialization. Also, disabilities observed in Het-HIQ children may derive from an initial sub-development of the right hemisphere due to genetic and/or environmental causes.
Methodological Consideration
FA is a useful measure of brain WM integrity that can be derived from DTI. It provides a simple and robust mean to assess the degree of anisotropic diffusion occurring within a region. FA is determined by both microscopic factors, such as myelination and axonal density, and macroscopic factors such as crossing fibers. Thus, the greater values of FA in HIQ children could be interpreted in terms of higher directionally coherent organization of fibers within voxels (Beaulieu, 2002), increased fiber tracts myelination (Jones et al., 2013), or higher axonal density and/or diameter (Mori and Zhang, 2006). However, increased FA could also be associated with less integrity, due to neurodegenerative processes in crossing fibers' regions (Douaud et al., 2011). Nevertheless, this effect is unlikely to occur in children. Furthermore, the increased FA observed in HIQ children is supported by the higher AD found in all HIQ groups compared to controls. AD quantifies the amount of water diffusion along the primary axis of the axon. It is believed to better reflect axonal density and/or caliber, while RD constitutes a more specific marker of myelin integrity (Song et al., 2005; Jones et al., 2013). Based on these assumptions, our findings suggest that HIQ children might present a greater axonal density or caliber than control subjects. The higher degree of axonal density or caliber could increase the signal transmission speed and thereby might account for more efficient brain functioning.
Among the diffusion metrics, significant differences were observed in FA and AD, but not in MD and RD. We can hypothesize that RD values might be decreased in HIQ children, leading to insignificant changes in MD but significant increase in FA. Such a hypothetical RD decrease would reinforce the argument of a higher degree of myelination in relation with intelligence (Dunst et al., 2014).
Nevertheless, this study may suffer from the relatively small number of children in each group. This is mostly related to the targeted population of children, which is difficult to recruit. Also, the ratio of girls and boys was different between HIQ and Control groups. This bias is probably related to girls' observed ability of “over-adaptation” which leads to less clinical consultations, thus reducing the number of girls diagnosed as HIQ, and particularly as Het-HIQ. In contrast, our control group was well balanced in that matter. This potential bias was taken into account by including age and sex as covariates in the voxel-wise statistical analysis.
Conclusion
Our findings demonstrate that increased WM integrity is correlated with higher intelligence capabilities, particularly in main central structures such as the corpus callosum, but also in WM associative and projection pathways. Our results highlight significant differences in WM tissue integrity between HIQ profiles, mainly in terms of hemispheric lateralization. Regions of higher diffusivity were more distributed in the right hemisphere of HIQ children with homogeneous IQ and in the left hemisphere of HIQ children with heterogeneous IQ. These findings suggest a potential relationship between intelligence profile and lateralization. The left hemispheric specialization of Het-HIQ children suggests a better capacity in language and may reflect their independent and self-connected behavior. In contrast, the bilateral and right hemispheric organization of Hom-HIQ children can support their greater capacities in exploration, adaptation and learning from environmental stimulations.
Ethics Statement
This study was carried out in accordance with the recommendations of CPP Sud-Est IV (France) with written informed consent from all subjects. All subjects gave written informed consent in accordance with the Declaration of Helsinki. The protocol was approved by the CPP Sud-Est IV (France).
Author Contributions
FN coordinated the organization and implementation of the study, recruited the children, provided the clinical expertise in the interpretation of the results, and participated to the paper writing. SH performed the voxelwise analysis and participated to the statistical analysis, and to the paper writing. GK performed the regional analysis and participated to the statistical analysis, and to the paper writing. CS helped in the interpretation of the results and participated to the paper writing. PF participated to the coordination of the study. OR participated to the coordination of the study, recruited children and provided the clinical expertise in the interpretation of the results. DSM supervised MR acquisition, provided methodological expertise in the interpretation of the results, and participated to the paper writing.
Conflict of Interest Statement
The authors declare that the research was conducted in the absence of any commercial or financial relationships that could be construed as a potential conflict of interest.
Acknowledgments
This work was made possible by APICIL Foundation and SHIRE International financial supports. We thank Antoine Streichenberger and Diane de Fremonville for their assistance with data analysis. We thank Dr. Ribal Haddad for English proofing the manuscript.
Abbreviations
AD, axial diffusivity; ADHD, attention deficit hyperactivity disorder; CBCL, child behavior checklist; CPRS, Conners' parent rating scale; DTI, diffusion tensor imaging; FSIQ, full scale intelligence quotient; Het-HIQ, heterogeneous-high intelligence quotient; HIQ, high intelligence quotient; Hom-HIQ, homogeneous-high intelligence quotient; MD, mean diffusivity; PRI, perceptual reasoning index; PSI, processing speed index; RD, radial diffusivity; TBSS, tract-based spatial statistic; TE, time of echo; TI, time for inversion. TR, time of repetition; VCI, verbal comprehension index; WISC, Wechsler intelligence scale for children; WM, white matter; FA, fractional anisotropy; WMI, working memory index.
References
Achenbach, T. M. (1991). Manual for the Child Behavior Checklist/4-18 and 1991 Profile. Burlington, VT: Department of Psychiatry, University of Vermont.
Agosta, F., Henry, R. G., Migliaccio, R., Neuhaus, J., Miller, B. L., Dronkers, N. F., et al. (2010). Language networks in semantic dementia. Brain 133, 286–299. doi: 10.1093/brain/awp233
Alsop, G. (2003). Asynchrony: intuitively valid and theoretically reliable. Roeper Rev. 25, 118–127. doi: 10.1080/02783190309554213
Badzakova-Trajkov, G., Corballis, M. C., and Häberling, I. S. (2016). Complementarity or independence of hemispheric specializations? A brief review. Neuropsychologia 93(Pt B), 386–393. doi: 10.1016/j.neuropsychologia.2015.12.018
Barbey, A. K., Colom, R., and Grafman, J. (2013). Architecture of cognitive flexibility revealed by lesion mapping. Neuroimage 82, 547–554. doi: 10.1016/j.neuroimage.2013.05.087
Barchmann, H., and Kinze, W. (1990). Behaviour and achievement disorders in children with high intelligence. Acta Paedopsychiatr. 53, 168–72.
Basser, P. J., and Jones, D. K. (2002). Diffusion-tensor MRI: theory, experimental design and data analysis - a technical review. NMR Biomed. 15, 456–467. doi: 10.1002/nbm.783
Basser, P. J., Mattiello, J., and LeBihan, D. (1994). MR diffusion tensor spectroscopy and imaging. Biophys. J. 66, 259–267. doi: 10.1016/S0006-3495(94)80775-1
Beaulieu, C. (2002). The basis of anisotropic water diffusion in the nervous system - a technical review. NMR Biomed. 15, 435–455. doi: 10.1002/nbm.782
Berk, R. A. (1982). Verbal-performance IG discrepancy score: a comment on reliability, abnormality, and validity. J. Clin. Psychol. 38, 638–41.
Bessou, A., Montlahuc, C., Louis, J., Fourneret, P., and Revol, O. (2005). Profil psychométrique de 245 enfants intellectuellement précoces au WISC-III. Approch. Neuropsychol. Apprentissages chez l'enfant 81, 23–28.
Braun, C. M. J., and Suffren, S. (2011). A general neuropsychological model of delusion. Cogn. Neuropsychiatry 16, 1–39. doi: 10.1080/13546800903442314
Caeyenberghs, K., and Leemans, A. (2014). Hemispheric lateralization of topological organization in structural brain networks. Hum. Brain Mapp. 35, 4944–4957. doi: 10.1002/hbm.22524
Catani, M., Howard, R. J., Pajevic, S., and Jones, D. K. (2002). Virtual in vivo interactive dissection of white matter fasciculi in the human brain. Neuroimage 17, 77–94. doi: 10.1006/nimg.2002.1136
Catani, M., and Mesulam, M. (2008). The arcuate fasciculus and the disconnection theme in language and aphasia: history and current state. Cortex 44, 953–961. doi: 10.1016/j.cortex.2008.04.002
Catani, M., Thiebaut de Schotten, M., Slater, D., and Dell'Acqua, F. (2013). Connectomic approaches before the connectome. Neuroimage 80, 2–13. doi: 10.1016/j.neuroimage.2013.05.109
Chatham, C. H., Claus, E. D., Kim, A., Curran, T., Banich, M. T., and Munakata, Y. (2012). Cognitive control reflects context monitoring, not motoric stopping, in response inhibition. PLoS ONE 7:e31546. doi: 10.1371/journal.pone.0031546
Clayden, J. D., Jentschke, S., Mu-oz, M., Cooper, J. M., Chadwick, M. J., Banks, T., et al. (2012). Normative development of white matter tracts: similarities and differences in relation to age, gender, and intelligence. Cereb. Cortex 22, 1738–1747. doi: 10.1093/cercor/bhr243
Conners, C. K., Sitarenios, G., Parker, J. D., and Epstein, J. N. (1998). Revision and restandardization of the Conners Teacher Rating Scale (CTRS-R): factor structure, reliability, and criterion validity. J. Abnorm. Child Psychol. 26, 279–291. doi: 10.1023/A:1022606501530
de Schotten, M. T., Dell'Acqua, F., Forkel, S. J., Simmons, A., Vergani, F., Murphy, D. G. M., et al. (2011). A lateralized brain network for visuospatial attention. Nat. Neurosci. 14, 1245–1246. doi: 10.1038/nn.2905
Desco, M., Navas-Sanchez, F. J., Sanchez-González, J., Reig, S., Robles, O., Franco, C., et al. (2011). Mathematically gifted adolescents use more extensive and more bilateral areas of the fronto-parietal network than controls during executive functioning and fluid reasoning tasks. Neuroimage 57, 281–292. doi: 10.1016/j.neuroimage.2011.03.063
Douaud, G., Jbabdi, S., Behrens, T. E. J., Menke, R. A., Gass, A., Monsch, A. U., et al. (2011). DTI measures in crossing-fibre areas: increased diffusion anisotropy reveals early white matter alteration in MCI and mild Alzheimer's disease. Neuroimage 55, 880–890. doi: 10.1016/j.neuroimage.2010.12.008
Dunst, B., Benedek, M., Koschutnig, K., Jauk, E., and Neubauer, A. C. (2014). Sex differences in the IQ-white matter microstructure relationship: a DTI study. Brain Cogn. 91, 71–78. doi: 10.1016/j.bandc.2014.08.006
Funnell, M. G., Corballis, P. M., and Gazzaniga, M. S. (2000). Insights into the functional specificity of the human corpus callosum. Brain 123(Pt 5), 920–926. doi: 10.1093/brain/123.5.920
Gaffan, D., and Wilson, C. R. E. (2008). Medial temporal and prefrontal function: recent behavioural disconnection studies in the macaque monkey. Cortex 44, 928–935. doi: 10.1016/j.cortex.2008.03.005
Galantucci, S., Tartaglia, M. C., Wilson, S. M., Henry, M. L., Filippi, M., Agosta, F., et al. (2011). White matter damage in primary progressive aphasias: a diffusion tensor tractography study. Brain 134, 3011–3029. doi: 10.1093/brain/awr099
Gazzaniga, M. S. (2000). Cerebral specialization and interhemispheric communication: does the corpus callosum enable the human condition? Brain 123(Pt 7), 1293–1326.
Gläscher, J., Rudrauf, D., Colom, R., Paul, L. K., Tranel, D., Damasio, H., et al. (2010). Distributed neural system for general intelligence revealed by lesion mapping. Proc. Natl. Acad. Sci. U.S.A. 107, 4705–4709. doi: 10.1073/pnas.0910397107
Goel, V., Vartanian, O., Bartolo, A., Hakim, L., Ferraro, A. M., Isella, V., et al. (2013). Lesions to right prefrontal cortex impair real-world planning through premature commitments. Neuropsychologia 51, 713–724. doi: 10.1016/j.neuropsychologia.2012.11.029
Gotts, S. J., Jo, H. J., Wallace, G. L., Saad, Z. S., Cox, R. W., and Martin, A. (2013). Two distinct forms of functional lateralization in the human brain. Proc. Natl. Acad. Sci. U.S.A. 110, E3435–E3444. doi: 10.1073/pnas.1302581110
Grossman, M., and Ash, S. (2004). Primary progressive aphasia: a review. Neurocase 10, 3–18. doi: 10.1080/13554790490960440
Guénolé, F., Speranza, M., Louis, J., Fourneret, P., Revol, O., and Baleyte, J.-M. (2015). Wechsler profiles in referred children with intellectual giftedness: associations with trait-anxiety, emotional dysregulation, and heterogeneity of Piaget-like reasoning processes. Eur. J. Paediatr. Neurol. 19, 402–410. doi: 10.1016/j.ejpn.2015.03.006
Guénolé, F., Louis, J., Creveuil, C., Baleyte, J.-M., Montlahuc, C., Fourneret, P., et al. (2013). Behavioral profiles of clinically referred children with intellectual giftedness. Biomed. Res. Int. 2013:540153. doi: 10.1155/2013/540153
Guignard, J.-H., Jacquet, A.-Y., and Lubart, T. I. (2012). Perfectionism and anxiety: a paradox in intellectual giftedness? PLoS ONE 7:e41043. doi: 10.1371/journal.pone.0041043
Hutchinson, A. D., Mathias, J. L., Jacobson, B. L., Ruzic, L., Bond, A. N., and Banich, M. T. (2009). Relationship between intelligence and the size and composition of the corpus callosum. Exp. Brain Res. 192, 455–464. doi: 10.1007/s00221-008-1604-5
Jones, D. K. (2004). The effect of gradient sampling schemes on measures derived from diffusion tensor MRI: a Monte Carlo study. Magn. Reson. Med. 51, 807–815. doi: 10.1002/mrm.20033
Jones, D. K., Knösche, T. R., and Turner, R. (2013). White matter integrity, fiber count, and other fallacies: the do's and don'ts of diffusion MRI. Neuroimage 73, 239–254. doi: 10.1016/j.neuroimage.2012.06.081
Lenroot, R. K., and Giedd, J. N. (2010). Sex differences in the adolescent brain. Brain Cogn. 72, 46–55. doi: 10.1016/j.bandc.2009.10.008
Marinsek, N., Turner, B. O., Gazzaniga, M., and Miller, M. B. (2014). Divergent hemispheric reasoning strategies: reducing uncertainty versus resolving inconsistency. Front. Hum. Neurosci. 8:839. doi: 10.3389/fnhum.2014.00839
Mesulam, M. M. (1998). From sensation to cognition. Brain 121(Pt 6), 1013–1052. doi: 10.1093/brain/121.6.1013
Mori, S., and Zhang, J. (2006). Principles of diffusion tensor imaging and its applications to basic neuroscience research. Neuron 51, 527–539. doi: 10.1016/j.neuron.2006.08.012
Navas-Sánchez, F. J., Alemán-Gómez, Y., Sánchez-Gonzalez, J., Guzmán-De-Villoria, J. A., Franco, C., Robles, O., et al. (2014). White matter microstructure correlates of mathematical giftedness and intelligence quotient. Hum. Brain Mapp. 35, 2619–2631. doi: 10.1002/hbm.22355
Oldfield, R. C. (1971). The assessment and analysis of handedness: the Edinburgh inventory. Neuropsychologia 9, 97–113. doi: 10.1016/0028-3932(71)90067-4
Prescott, J., Gavrilescu, M., Cunnington, R., O'Boyle, M. W., and Egan, G. F. (2010). Enhanced brain connectivity in math-gifted adolescents: an fMRI study using mental rotation. Cogn. Neurosci. 1, 277–288. doi: 10.1080/17588928.2010.506951
Qiu, D., Tan, L.-H., Zhou, K., and Khong, P.-L. (2008). Diffusion tensor imaging of normal white matter maturation from late childhood to young adulthood: voxel-wise evaluation of mean diffusivity, fractional anisotropy, radial and axial diffusivities, and correlation with reading development. Neuroimage 41, 223–232. doi: 10.1016/j.neuroimage.2008.02.023
Reis, S. M., and McCoach, D. B. (2000). The Underachievement of gifted students: what do we know and where do we go? Gift. Child Q. 44, 152–170. doi: 10.1177/001698620004400302
Schmahmann, J. D., and Pandya, D. N. (2008). Disconnection syndromes of basal ganglia, thalamus, and cerebrocerebellar systems. Cortex 44, 1037–1066. doi: 10.1016/j.cortex.2008.04.004
Schmithorst, V. J., Wilke, M., Dardzinski, B. J., and Holland, S. K. (2005). Cognitive functions correlate with white matter architecture in a normal pediatric population: a diffusion tensor MRI study. Hum. Brain Mapp. 26, 139–147. doi: 10.1002/hbm.20149
Schmithorst, V. J., and Yuan, W. (2010). White matter development during adolescence as shown by diffusion MRI. Brain Cogn. 72, 16–25. doi: 10.1016/j.bandc.2009.06.005
Seghete, K. L. M., Herting, M. M., and Nagel, B. J. (2013). White matter microstructure correlates of inhibition and task-switching in adolescents. Brain Res. 1527, 15–28. doi: 10.1016/j.brainres.2013.06.003
Sidtis, J. J., Volpe, B. T., Holtzman, J. D., Wilson, D. H., and Gazzaniga, M. S. (1981). Cognitive interaction after staged callosal section: evidence for transfer of semantic activation. Science 212, 344–346. doi: 10.1126/science.6782673
Silverman, L. K. (2011). The construct of asynchronous development. Peabody J. Educ. 72, 36–58. doi: 10.1080/0161956X.1997.9681865
Singh, H., and O'Boyle, M. W. (2004). Interhemispheric interaction during global-local processing in mathematically gifted adolescents, average-ability youth, and college students. Neuropsychology 18, 371–377. doi: 10.1037/0894-4105.18.2.371
Smith, S. M., Jenkinson, M., Johansen-Berg, H., Rueckert, D., Nichols, T. E., Mackay, C. E., et al. (2006). Tract-based spatial statistics: voxelwise analysis of multi-subject diffusion data. Neuroimage 31, 1487–1505. doi: 10.1016/j.neuroimage.2006.02.024
Smith, S. M., Jenkinson, M., Woolrich, M. W., Beckmann, C. F., Behrens, T. E. J., Johansen-Berg, H., et al. (2004). Advances in functional and structural MR image analysis and implementation as FSL. Neuroimage 23(Suppl. 1), S208–S219. doi: 10.1016/j.neuroimage.2004.07.051
Song, S.-K., Sun, S.-W., Ramsbottom, M. J., Chang, C., Russell, J., and Cross, A. H. (2002). Dysmyelination revealed through MRI as increased radial (but unchanged axial) diffusion of water. Neuroimage 17, 1429–1436. doi: 10.1006/nimg.2002.1267
Song, S.-K., Yoshino, J., Le, T. Q., Lin, S.-J., Sun, S.-W., Cross, A. H., et al. (2005). Demyelination increases radial diffusivity in corpus callosum of mouse brain. Neuroimage 26, 132–140. doi: 10.1016/j.neuroimage.2005.01.028
Sweetland, J. D., Reina, J. M., and Tatti, A. F. (2006). WISC-III verbal/performance discrepancies among a sample of gifted children. Gift. Child Q. 50, 7–10. doi: 10.1177/001698620605000102
Szaflarski, J. P., Rajagopal, A., Altaye, M., Byars, A. W., Jacola, L., Schmithorst, V. J., et al. (2012). Left-handedness and language lateralization in children. Brain Res. 1433, 85–97. doi: 10.1016/j.brainres.2011.11.026
Terrassier, J. (2009). Intellectually precocious children. Arch. Pediatr. 16, 1603–1606. doi: 10.1016/j.arcped.2009.07.019
Tournier, J.-D., Mori, S., and Leemans, A. (2011). Diffusion tensor imaging and beyond. Magn. Reson. Med. 65, 1532–1556. doi: 10.1002/mrm.22924
Tsang, J. M., Dougherty, R. F., Deutsch, G. K., Wandell, B. A., and Ben-Shachar, M. (2009). Frontoparietal white matter diffusion properties predict mental arithmetic skills in children. Proc. Natl. Acad. Sci. U.S.A. 106, 22546–22551. doi: 10.1073/pnas.0906094106
Vaivre-Douret, L. (2011). Developmental and cognitive characteristics of “high-level potentialities” (highly gifted) children. Int. J. Pediatr. 2011:420297. doi: 10.1155/2011/420297
Van Beek, L., Ghesquière, P., Lagae, L., and De Smedt, B. (2014). Left fronto-parietal white matter correlates with individual differences in children's ability to solve additions and multiplications: a tractography study. Neuroimage 90, 117–127. doi: 10.1016/j.neuroimage.2013.12.030
Keywords: high intelligence-quotient children, gifted, talented, MRI, diffusion tensor imaging, tract-based spatial statistics (TBSS)
Citation: Nusbaum F, Hannoun S, Kocevar G, Stamile C, Fourneret P, Revol O and Sappey-Marinier D (2017) Hemispheric Differences in White Matter Microstructure between Two Profiles of Children with High Intelligence Quotient vs. Controls: A Tract-Based Spatial Statistics Study. Front. Neurosci. 11:173. doi: 10.3389/fnins.2017.00173
Received: 09 December 2016; Accepted: 15 March 2017;
Published: 03 April 2017.
Edited by:
John Vijay Sagar Kommu, National Institute of Mental Health and Neurosciences, IndiaCopyright © 2017 Nusbaum, Hannoun, Kocevar, Stamile, Fourneret, Revol and Sappey-Marinier. This is an open-access article distributed under the terms of the Creative Commons Attribution License (CC BY). The use, distribution or reproduction in other forums is permitted, provided the original author(s) or licensor are credited and that the original publication in this journal is cited, in accordance with accepted academic practice. No use, distribution or reproduction is permitted which does not comply with these terms.
*Correspondence: Dominique Sappey-Marinier, dominique.sappey-Marinier@univ-lyon1.fr