- School of Biology, University of St Andrews, St Andrews, Fife, UK
Humans are characterized by an extreme dependence on culturally transmitted information and recent formal theory predicts that natural selection should favor adaptive learning strategies that facilitate effective copying and decision making. One strategy that has attracted particular attention is conformist transmission, defined as the disproportionately likely adoption of the most common variant. Conformity has historically been emphasized as significant in the social psychology literature, and recently there have also been reports of conformist behavior in non-human animals. However, mathematical analyses differ in how important and widespread they expect conformity to be, and relevant experimental work is scarce, and generates findings that are both mutually contradictory and inconsistent with the predictions of the models. We review the relevant literature considering the causation, function, history, and ontogeny of conformity, and describe a computer-based experiment on human subjects that we carried out in order to resolve ambiguities. We found that only when many demonstrators were available and subjects were uncertain was subject behavior conformist. A further analysis found that the underlying response to social information alone was generally conformist. Thus, our data are consistent with a conformist use of social information, but as subjects’ behavior is the result of both social and asocial influences, the resultant behavior may not be conformist. We end by relating these findings to an embryonic cognitive neuroscience literature that has recently begun to explore the neural bases of social learning. Here conformist transmission may be a particularly useful case study, not only because there are well-defined and tractable opportunities to characterize the biological underpinnings of this form of social learning, but also because early findings imply that humans may possess specific cognitive adaptations for effective social learning.
In 1963, ethologist Niko Tinbergen argued that to fully understand behavior in biology it is necessary to consider it from four different perspectives, that of its history, ontogeny, function, and causation. Whilst heuristics such of these have the potential to constrain research as much as assist it (Laland et al., 2011a) the realization of answers to Tinbergen’s four questions is often a very helpful target for research, encouraging a broad perspective on behavior, and fostering interdisciplinary approaches. Here we focus on behavioral conformity, a topic that has received considerable attention from at least three of these perspectives. Below we summarize insights into conformity derived from social and developmental psychology, cultural evolution modeling and experimentation, animal social learning, and cognitive neuroscience. As the approaches of these fields do not map cleanly onto Tinbergen’s questions, we organize our analysis on a disciplinary basis, but draw attention to which of Tinbergen’s questions is being addressed in each case. We conclude that the study of conformity has been hampered by definitional inconsistency and experimental limitations and recognize the need for a more comprehensive theoretical framework if researchers are to progress toward a common understanding that spans these diverse fields.
The Social Psychology Literature
The earliest investigations into conformity were carried out by social psychologists during the twentieth century and were focused very much on its causation; that is, on the social contexts that elicited it (Jenness, 1932; Sherif, 1935; Asch, 1955). In an extremely influential paper, Solomon Asch (Asch, 1955) described the observation that adults would willingly abandon their own perceptual judgment in a very simple visual task when faced with a group of confederates who disagreed with them. Asch termed this behavior conformity, supposing the deference to the group norm to be driven by a desire to receive social approval. Such a finding has been replicated a huge number of times across age groups and cultures and a large number of factors that influence whether or not individuals conform have been identified including group size (Asch, 1955; Bond, 2005), task difficulty and importance (Baron et al., 1996), culture (Bond and Smith, 1996) motivation (Griskevicius et al., 2006), and mood (Tong et al., 2008). Whilst social psychology, replete with empirical data, has successfully identified many factors influencing when individuals adopt the decisions of others, it has struggled to unify such findings into a single theoretical framework. Perhaps the most successful attempt is Social Impact Theory (Latane, 1981; Latane and Wolf, 1981; Nowak et al., 1990), which characterizes social influence as a force, analogous to a physical force such as electro-magnetism, that acts on an individual. Factors proposed to influence the magnitude of this force are its strength (determined by factors such as the age and status of the source), immediacy, (proximity in space-time to the observer), and the number of people in the group to which the observer is exposed. Social Impact Theory can effectively explain the diminishing effect of increasing the number of confederates in the Asch experiments (Latane, 1981), and was also extended to cases where a majority conflicted with a minority (Latane and Wolf, 1981). However, its variables of strength and immediacy – precisely that which distinguished it from other models (e.g., Tanford and Penrod, 1984) – came up against conflicting empirical findings and where effects were found they were typically of a very low magnitude (Mullen, 1985, 1986; Jackson, 1986). Furthermore, these theories were largely based on studies involving the adoption of arbitrary or bizarre group decisions and so their ability to understand social influence more generally, particularly in the context of evolution, is limited. Accordingly, the ambitions of theories of social influence from social psychology, although valuable contributions to the study of social learning, were never fully realized.
Nonetheless, social influence theories were very successful in accounting for group size effects. Moreover, social psychology is also the source of a valuable distinction between informational and normative motivations for conforming to a group norm (Deutsch and Gerard, 1955). This distinction came about as researchers attempted to understand why their subjects were conforming to clearly incorrect decisions. They argued for two goals on the part of the subject, one to be correct, but a second to earn positive appraisal from others through agreement. The former is an informational goal, the latter a normative goal. As the simplicity of the task in the Asch experiments seems to preclude an informational goal, it has been argued that the subjects were conforming in order to achieve a normative reward, received by being in agreement with your group mates. Surprisingly given this, Deutsch and Gerard (1955) found that some subjects would still choose the clearly incorrect answer even when they made their decision in the absence of confederates. They took this to mean that the confederates were also exerting some informational influence and that the subjects may really have believed the group decisions. An alternative explanation is that, even when apparently isolated, individuals may find normative tendencies hard to resist.
Cultural Evolution Modeling
Impetus to the study of conformity was also derived from cultural evolution theory. Starting in the 1970s, a group of theoretical evolutionary biologists began to investigate culture and the social transmission of information using mathematical evolutionary models (Cavalli-Sforza and Feldman, 1981; Lumsden and Wilson, 1981; Boyd and Richerson, 1985). Central to this approach was setting the use of social information in an evolutionary context (that is, considering its “function” and “evolutionary history,” in terms of Tinbergen’s questions) and attempting to understand when and how individuals should come to rely on social transmission to maximize their fitness. Individuals were predicted to be equipped with a wide range of cultural transmission biases that dictate when they copy others and who they copy (Boyd and Richerson, 1985; Feldman et al., 1996; Henrich and Boyd, 1998; Schlag, 1998, 1999; Henrich and Gil-White, 2001; Henrich and McElreath, 2003; Laland, 2004; Enquist et al., 2007; Wakano and Aoki, 2007; Kendal et al., 2009). Cultural evolutionists used the term conformity to describe a particular learning rule by which an individual was disproportionately likely to adopt the majority decision (see Boyd and Richerson, 1985, p.206, see Figure 1). Mathematical models established that conforming is an effective strategy in a spatially variable environment with migration between subpopulations, because it helps individuals to home in on the locally adaptive behavior (Boyd and Richerson, 1985; Henrich and Boyd, 1998; Nakahashi et al., forthcoming). In this respect, the cultural evolutionist notion of conformity fits well with an informational notion of conformity – people are expected to conform because it leads them to acquire valuable fitness-enhancing information. Nonetheless, the evolution of this tendency to conform could also plausibly explain the existence of normative conformity (Boyd and Richerson, 1985; Richerson and Boyd, 2005). Moreover, different groups can conform to different variants under the action of a conformist bias, with the potential to explain the combination of stable intergroup heterogeneity and intragroup homogeneity seen in human populations, and potentially promote cultural group selection (Boyd and Richerson, 1985; Richerson and Boyd, 2005; Kendal et al., 2009).
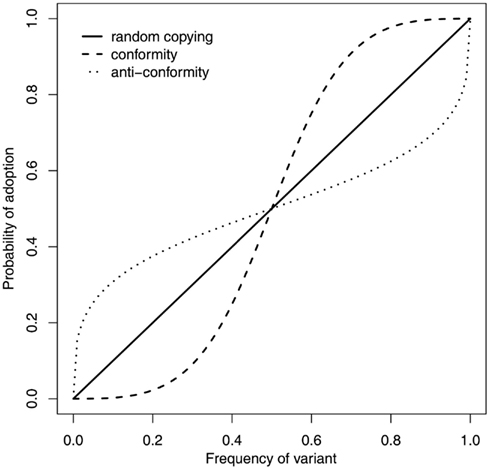
Figure 1. Conformity (the dashed line) is just one of several learning rules that result in increasingly likely adoption of a trait as it increases in frequency, however, it is unique as the tendency toward the most popular trait is disproportionate given its frequency. A proportionate tendency, equivalent to random copying (solid line), results in a probability of adoption equal to trait frequency, whereas anti-conformity (dotted line) resists the most popular choice and has the opposite population consequences to conformity. Of these frequency dependent rules, only conformity leads to homogenous group behavior.
However, theoretical analyses have found conflicting results when investigating the adaptive value of a conformist response to social information. For example, some models have found that conformity evolves alongside less discriminate social learning and fares well even in the face of a spatially and temporally variable environment (Boyd and Richerson, 1985; Henrich and Boyd, 1998). However, these models have been criticized as they assume that individuals have access to all behavioral variants at all times and merely have to choose the correct option. The critics claim that when this assumption is relaxed conformity suffers (Eriksson et al., 2007). However, Eriksson et al.’s models could be argued to be no more realistic than Boyd, Richerson, Henrich et al.’s, as here each incidence of environmental change means an entirely new behavior must be developed from scratch. Equally important is the extent of spatial and temporal variation, since the former promotes reliance on conformity while the latter selects against it (Hoppitt et al., 2010; Nakahashi et al., forthcoming). Thus the extent to which conformity is expected to be adaptive is contested, but the evidence from theoretical models on balance leads us to expect a broad range of conditions under which it will be utilized.
The huge amount of empirical data from social psychology might be thought to clarify this issue, as researchers could empirically determine whether, and under what circumstances, human subjects displayed a conformist tendency. However, this is unfortunately not the case for two reasons. Firstly, although a conformist would be expected to behave like subjects in the Asch experimental paradigm, such experiments are unable to distinguish between multiple possible learning rules that posit a positive relationship between trait popularity and probability of trait adoption. For example, as depicted in Figure 1, conformity, anti-conformity, and random copying all result in more popular traits being more likely to be adopted than less popular traits (Boyd and Richerson, 1985), yet amongst these only conformity would lead to the homogenization of group behavior; anti-conformity erodes any group preferences whilst random copying does not act to change trait frequencies. Secondly, a disproportionate tendency to adopt the majority behavior is only expected in cases where the observing individual is naive (Boyd and Richerson, 1985). This means that the Asch paradigm is unsuitable to investigate conformity in the context of cultural evolution as the simplicity of the tasks used meant that the subjects were far from naive when listening to the decisions of the confederates. Instead, asocial information must be controlled for, either experimentally, by using a design such that subjects genuinely are in a state of naivety, or statistically, such that a measure of asocial information is taken and can be used in analyses to separate the effects of asocial and social information. Given this, empirically minded cultural evolutionists have carried out further studies to investigate the nature of the response to variant frequency.
Animal Experiments
Before considering experiments with human subjects it is well worth noting that social learning researchers have carried out a great deal of work with other animals looking for conformist learning. This provides further insights into the third of Tinbergen’s questions, evolutionary history, as through a consideration of the current taxonomic distribution of conformity researchers are potentially able to infer the most likely evolutionary history of the trait. In fact, evidence in line with conformity exists in a wide range of taxa including fish (Day, 2001; Pike and Laland, 2010), rats (Konopasky and Telegdy, 1977; Galef and Whiskin, 2008), monkeys (Dindo et al., 2009), and great apes (Whiten et al., 2005), although in the latter case the claim for conformity rests on a more normative notion. It should be noted, however, that the methods employed in these studies, as in the Asch experiment, are typically not sufficient to rule out other forms of social learning that involve a positive relationship between trait popularity and the likelihood of its adoption. The only study of which we are aware that provides clear evidence of non-human animals exhibiting a disproportionate tendency to adopt the majority behavior is Pike and Laland’s (2010) investigation of public information use in sticklebacks. Given the taxonomic distance between fish and humans, this finding is most likely to reflect convergent selection for conformity rather than a homologous capability (Laland et al., (2011b). Thus, although intriguing, more detailed experimental work is required to understand both the evolutionary history of the human capability for conformity, and its phylogenetic distribution.
Human Experimentation
Concerning humans, however, there have been several experiments with the required precision to distinguish a disproportionate tendency to adopt the majority decision from other rules that lack the same population level consequences. Efferson et al. (2008) carried out an experiment in which subjects chose between two “technologies.” The subjects knew the alternative technologies had different expected payoffs, but did not know which was the better technology. Over many rounds the subjects repeatedly chose between the two technologies. Half the subjects were asocial learners and were given feedback concerning the payoffs of their decisions, the other subjects were social learners and were only given information on the decisions of the asocial learners. Although conformity was found to be an effective strategy for the social learners, Efferson et al. found that only the behavior of some subjects in the social learning condition, those that self-defined as conformist, could be well explained with a conformist model, whilst the behavior of the other subjects, who did not describe their behavior as conformist, could not. Efferson et al. characterize this difference in terms of a mixed population of conformists and “mavericks,” the latter representing individuals typically reliant on asocial information. There was considerable individual-level variation within the conformist and maverick groups, suggesting that a dichotomy of types would not be an appropriate interpretation – rather, individuals vary in the extent to which they utilize social information and/or tend to conform.
A further experiment (McElreath et al., 2005) also used a design where subjects were required to choose between two technologies, and once again subject differences were found in the use of social information. Furthermore, although subjects did sometimes show a conformist response, they did not do so when the environment was stable, a result at odds with theory that suggests environmental stability is the ideal scenario for conformity to do well (Henrich and Boyd, 1998). In addition to this Toelch et al. (2010) found that subjects track variant popularity over time and in effect anticipate a future majority choice by favoring variants that show increasing popularity. This makes sense in the context of possible environmental change and such behavior may allow individuals rapidly to take advantage of emerging technologies and overcome the cultural inertia that conformity imposes.
Following these conflicting findings, studies are now turning to the idea of flexible conformity and attempting to identify factors that influence whether or not, and under what circumstances, subject behavior is conformist. To this end we carried out a study (Morgan et al., 2011) in which subjects were required to decide whether a pair of 3D shapes were the same shape seen from different angles or different shapes entirely (cf. Shepard and Metzler, 1971). Over multiple trials, subjects were initially allowed to attempt the task themselves and were asked to make a decision and rate their confidence in their decision. They were then shown the decisions of a group of previous subjects who had been faced with the same shape pair (the number of demonstrators was either 4, 8, or 12, one trial per subject involved no social information) and were again asked to make a decision and rate their confidence in it. Crucially, this design recorded subjects’ decisions and confidence both before and after receiving social information, allowing us to separate the social and asocial information in the subjects’ decision making. We found that subjects were disproportionately likely to adopt the social majority decision only when the number of demonstrators was high and subjects were uncertain in their own abilities (see Figure 2). Further analyses examined the effect of social information in isolation and identified a general conformist response underlying subject decision making (see Figure 2). The effect of the popularity of the modal choice interacted with the size of the group of demonstrators, however, with increasing group size corresponding to an increasingly disproportionate response to popularity. This is in concordance with theory that shows that the information provided by a majority of a given size hinges on the size of the overall population (see ESM, Morgan et al., 2011). Accordingly, we provide evidence that there is a conformist bias underlying human decision making and that in at least some circumstances human behavior will match conformist predictions. Finally, we were able to show that subjects’ use of social information in the experiments was adaptive in the sense that it increased their performance across the experiment, in line with the adaptive predictions of evolutionary models.
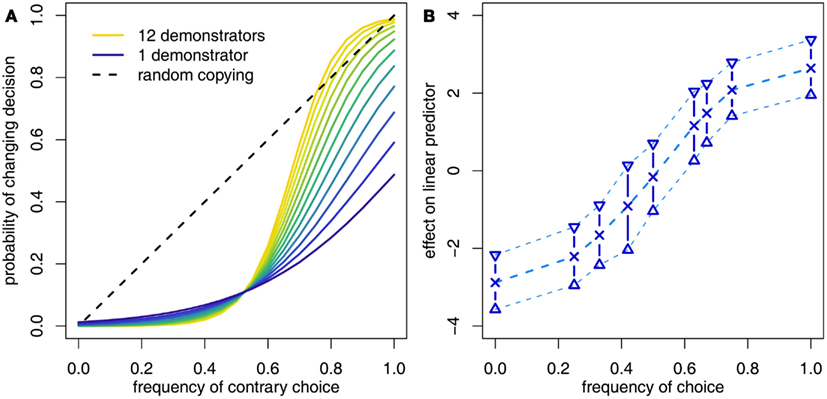
Figure 2. (A) Morgan et al. (2011) found that adult human subjects were disproportionately likely to switch their decision to that favored by the majority only when they were presented with a large group of demonstrators, they were uncertain in their own abilities to solve the tasks and the majority was very large. (B) However, controlling for prior asocial information showed that the response of subjects to the social information in isolation was more generally conformist, as illustrated by the S-shaped curve. In this case the y-axis reflects the change to a linear predictor prior to transformation into a probability and the shape of the curve was not constrained in any way.
The Neural Bases of Conformity
Whilst the aforementioned studies by social psychologists have made ground in isolating the social contexts that elicit conformity, this is just one aspect of the immediate causes of this behavior. A complete understanding requires some knowledge of what goes on in the brains of conforming individuals. However, the neural-level processes underlying conformity have received comparatively little attention.
Nonetheless, recent studies investigating the neurobiology of social learning more generally have come up with several relevant findings. Firstly, studies using both mental rotation tasks (Berns et al., 2005) and auditory tasks (Berns et al., 2010) have found that social information affected neural activity in the relatively low level processing areas associated with each task, in addition to areas distinct from these perceptual decision making circuits, suggesting that the social information was affecting the perception of the subjects as well as their decision making, a possibility raised by Asch in the interpretation of his findings (Asch, 1955). In addition to this, ventral striatum activity in a music choice task (Campbell-Meiklejohn et al., 2010) suggests that the social information was directly affecting the perceived value of different songs. These findings are consistent with the idea that social and asocial sources of information are integrated starting at early stages of processing, however, the low temporal resolution of fMRI limits the strength of such a conclusion. Finally, Mason et al. (2009) exposed subjects to symbols that received positive, negative, or no social labeling. Exposure to a socially marked symbol resulted in activity in the medial prefrontal cortex, irrespective of whether it was positively or negatively marked, whilst activity in the caudate coded the valence of the social marking. These findings suggest that it is through the integration of activity in these two areas that individuals distinguish between positively and negatively socially marked stimuli.
For subjects to be employing a conformist learning bias we would predict there to be parts of the brain that evaluate levels of consensus amongst demonstrators. Whilst no data exists for studies using sufficiently large groups of demonstrators with varying levels of consensus, there are nonetheless hints of the existence of such a mechanism in the brain. The music choice experiment (Campbell-Meiklejohn et al., 2010) found that along with activity in the insula cortex and right tempoparietal junction, areas associated with monitoring the decisions of others, anterior insula activity increased when the two “expert” reviewers were in agreement. Although this is suggestive of a consensus evaluating mechanism it should be noted that with a group of only two demonstrators the social information was either in unanimity or in total disagreement, thus the anterior insula may have been responding to social information with an overall message and not the specific level of consensus itself. What is more clear, however, are changes in brain activity caused by disagreement between the subject and the demonstrators. Klucharev et al. (2009) found that disagreement between the subject and the demonstrators caused activity in several areas known to be involved with more general error and conflict processing such as the rostral cingulate zone (Botvinick et al., 2004) and depressed activity in reward centers such as the nucleus acumbens in the ventral striatum. Thus, brain areas that evaluate object value, such as the ventral striatum in the music choice task (Campbell-Meiklejohn et al., 2010), also seem to play a role in rewarding the subject for being in agreement with others. Furthermore the magnitude of the change in activation of these areas predicted changes in subsequent subject behavior (Klucharev et al., 2009; Campbell-Meiklejohn et al., 2010).
Neurobiological experiments are doing more than explaining phenomena from other fields, however, they are also highlighting how those fields need to broaden their perspectives. For example, cultural evolution and social psychology are yet to integrate the study of normative and informational influences into a single framework (Deutsch and Gerard, 1955). Experiments typically attempt to explain subject behavior in terms of one or the other source of influence (e.g., informational; Morgan et al., 2011) and even posit different behavioral responses when subjects are influenced by one or the other (Campbell and Fairey, 1989). However, evidence from neurological studies provide evidence that the two processes may be unavoidably intertwined. For example, Berns et al. 2005) found increased activity when subjects disagreed with human participants as opposed to computers, despite the fact that the task was not obviously normative in nature, although this could be a result of subjects placing more weight on human responses that those of computers. However, other studies have found activity in areas strongly suggestive of a normative response, including the amygdala, an area associated with emotional load, suggesting that subjects found their being in disagreement with others stressful (Klucharev et al., 2009). Potentially suggesting otherwise, a study into the social modification of memory (Edelson et al., 2011) in which subjects were asked questions about a video they had watched several weeks earlier, both before and after being given false information, found that the amygdala showed increased activity only when the information purportedly came from other individuals (as opposed to computers) and when the subject subsequently altered their long-term responses accordingly. That no such activity was seen when behavioral adjustments were temporary suggests the activity was related to memory modification and so an emotional load may not have been involved, however, the activity was only seen when the information came from humans indicative of a normative aspect. A further study (Berns et al., 2010) found similar activity in the insula, an area associated with anxiety and ostracism, whilst another (Campbell-Meiklejohn et al., 2010) found activity in the lateral prefrontal cortex, an area linked with reputation management, this activity also predicted subsequent behavioral adjustments to the group norm. The similarities between the response to human and computer decisions could be interpreted as subjects anthropomorphizing the computers, or alternatively treating human demonstrators as machines. Such findings imply that if researchers are to understand social information use, including conformity, at the behavioral level it may be insufficient to consider it in light of either informational or normative influence in isolation as they may not be distinct processes at the neural level. A more complete theory of social decision making may need to include both, with variable payoffs associated with getting the correct answer and fitting in with group mates. Experiments where both are included and their relative strengths manipulated could help our understanding of how the two interact. From this perspective, social decision making involves a maximization of reward taking into account the information provided by others on the group norm and the level consensus behind it, the expected cost of deviating from such a consensus, the individual’s own information concerning the task, the information provided by others concerning the task, and the expected cost of making an incorrect decision. To proceed with our understanding of social decision making it may be necessary to combine the above elements into a single theoretical framework and to stop thinking of behavior in terms of either normative and social influences.
The Development of Conformity
The fourth of Tinbergen’s questions, ontogeny, is one area that the study of conformity has left relatively untouched. Researchers have tended to assume that any conformist bias is an evolved predisposition, and have not generally sought to investigate how its expression changes during the lifetime of an individual. The study of trust in developmental psychology (Harris, 2007; Harris and Corriveau, 2011) however, is of clear relevance to this topic. Young children have been shown to be remarkably sensitive to a range of factors when deciding how to use social information and although work has generally focused on reliability (Koenig and Harris, 2005, 2007; Fusaro and Harris, 2008; Corriveau and Harris, 2009), studies have replicated the Asch experiment with young children (Corriveau and Harris, 2010) and have found a persistent bias for relying on individuals who fit in well with the child’s cultural group (Corriveau et al., 2009). Were such studies extended to examine the impact of different levels of consensus it would be highly illustrative with regards to the ontogeny of conformity. Indeed, there is already evidence that learning rules vary over time. For example, children of different ages show a shift in sensitivity to reliability assessment that may be experientially triggered (Clement et al., 2004). There is also support for this from neurobiological experiments, for example, the dorsomedial prefrontal cortex, right middle temporal gyrus, and the right superior temporal sulcus at the temporal junction have been found to monitor the reliability of informants in a manner similar to dopaminergic activity in reward learning (Behrens et al., 2008). This implies that expected values are estimated for different sources as the subject gains feedback from their decisions. These areas are also involved in motive attribution in social tasks, suggesting that social reliability takes a multitude of distinctly social factors, such as deceit, into account (Behrens et al., 2008). Similarly, whilst activity in the anterior cingulate sulcus monitors volatility in the expected reward value of non-social decisions, the anterior cingulate gyrus monitors volatility in the expected reward value of following the advice of others (Behrens et al., 2008). These two sources were then combined in the ventromedial prefrontal cortex with the relative activity of the two streams predicting which stream best corresponds with behavior (Behrens et al., 2008). This continuous assessment of the value of social information and demonstrators implies that the ontogeny of social learning biases may be more complicated that many experimentalists have typically assumed.
Conclusion
From the above we can see that Tinbergen’s four questions of history, ontogeny, function, and causation are highly instructive in identifying areas in which our understanding of conformity and social learning rules more generally needs to be developed. With large amounts of theoretical and empirical data on the topic, researchers are beginning to identify when individuals will conform, and with further careful non-human experimental work they will soon be able to understand the current taxonomic distribution of such a bias. However, recent neurobiological experiments show that a complete understanding of conformity likely requires integration across these categories. It may no longer be fruitful to view conformity in a solely normative or informational world, as the human (and likely non-human) brain seemingly does not separate the two. Further work is required to explore how experience can affect the development of conformist learning, with clear implications for both individual differences, and the use of social information in general. Whilst multiple approaches have found a range of exciting results, researchers are now at the point at which integration is required for the biological understanding of conformity.
Conflict of Interest Statement
The authors declare that the research was conducted in the absence of any commercial or financial relationships that could be construed as a potential conflict of interest.
References
Baron, R. S., Vandello, J. A., and Brunsman, B. (1996). The forgotten variable in conformity research: impact of task importance on social influence. J. Pers. Soc. Psychol. 71, 915–927.
Behrens, T. E. J., Hunt, L. T., Woolrich, M. W., and Rushworth, M. F. S. (2008). Associative learning of social value. Nature 456, 245–249.
Berns, G. S., Capra, C. M., Moore, S., and Noussair, C. (2010). Neural mechanisms of the influence of popularity on adolescent ratings of music. Neuroimage 49, 2687–2696.
Berns, G. S., Chappelow, J., Zink, C. F., Pagnoni, G., Martin-Skurski, M. E., and Richards, J. (2005). Neurobiological correlates of social conformity and independence during mental rotation. Biol. Psychiatry 58, 245–253.
Bond, R., and Smith, P. B. (1996). Culture and conformity: a meta-analysis of studies using Asch’s (1952b, 1956) line judgment task. Psychol. Bull. 119, 111–137.
Botvinick, M. M., Cohen, J. D., and Carter, C. S. (2004). Conflict monitoring and anterior cingulate cortex: an update. Trends Cogn. Sci. (Regul. Ed.) 8, 539–546.
Boyd, R., and Richerson, P. J. (1985). Culture and the Evolutionary Process. Chicago: University of Chicago Press.
Campbell, J. D., and Fairey, P. J. (1989). Informational and normative routes to conformity: the effect of faction size as a function of norm extremity and attention to the stimulus. J. Pers. Soc. Psychol. 57, 457–468.
Campbell-Meiklejohn, D., Bach, D., and Roepstorff, A. (2010). How the opinion of others affects our valuation of objects. Curr. Biol. 20, 1165–1170.
Cavalli-Sforza, L. L., and Feldman, M. W. (1981). Cultural Transmission and Evolution: A Quantitative Approach. Princeton: Princeton University Press.
Corriveau, K., and Harris, P. L. (2009). Preschoolers continue to trust a more accurate informant 1 week after exposure to accuracy information. Dev. Sci. 12, 188–193.
Corriveau, K. H., Fusaro, M., and Harris, P. L. (2009). Going with the flow: preschoolers prefer nondissenters as informants. Psychol. Sci. 20, 372–7.
Corriveau, K. H., and Harris, P. L. (2010). Preschoolers (sometimes) defer to the majority in making simple perceptual judgments. Dev. Psychol. 46, 437–445.
Day, R. (2001). Interactions between shoal size and conformity in guppy social foraging. Anim. Behav. 62, 917–925.
Deutsch, M., and Gerard, H. B. (1955). A study of normative and informational social influences upon individual judgment. J. Abnorm. Soc. Psychol. 51, 629–636.
Dindo, M., Whiten, A., and de Waal, F. B. M. (2009). In-group conformity sustains different foraging traditions in capuchin monkeys (Cebus apella). PLoS ONE 4, e7858.
Edelson, M., Sharot, T., Dolan, R. J., and Dudai, Y. (2011). Following the crowd: brain substrates of long-term memory conformity. Science 333, 108–11.
Efferson, C., Lalive, R., Richerson, P. J., McElreath, R., and Lubell, M. (2008). Conformists and mavericks: the empirics of frequency-dependent cultural transmission. Evol. Hum. Behav. 29, 56–64.
Enquist, M., Eriksson, K., and Ghirlanda, S. (2007). Critical social learning: a solution to Rogers’s paradox of nonadaptive culture. Am. Anthropol. 109, 727–734.
Eriksson, K., Enquist, M., and Ghirlanda, S. (2007). Critical points in current theory of conformist social learning. J. Evol. Psychol. 5, 67–87.
Feldman, M., Aoki, K., and Kumm, J. (1996). Individual versus social learning: evolutionary analysis in a fluctuating environment. Anthropol. Sci. 104, 209–231.
Fusaro, M., and Harris, P. L. (2008). Children assess informant reliability using bystanders’ non-verbal cues. Dev. Sci. 11, 771–777.
Griskevicius, V., Goldstein, N. J., Mortensen, C. R., Cialdini, R. B., and Kenrick, D. T. (2006). Going along versus going alone: when fundamental motives facilitate strategic (non)conformity. J. Pers. Soc. Psychol. 91, 281–294.
Harris, P. L., and Corriveau, K. H. (2011). Young children’s selective trust in informants. Philos. Trans. R. Soc. Lond. B Biol. Sci. 366, 1179–1187.
Henrich, J., and Boyd, R. (1998). The evolution of conformist transmission and the emergence of between-group differences. Evol. Hum. Behav. 19, 215–241.
Henrich, J., and Gil-White, F. J. (2001). The evolution of prestige – freely conferred deference as a mechanism for enhancing the benefits of cultural transmission. Evol. Hum. Behav. 22, 165–196.
Henrich, J., and McElreath, R. (2003). The evolution of cultural evolution. Evol. Anthropol. 12, 123–135.
Hoppitt, W., Kandler, A., Kendal, J. R., and Laland, K. N. (2010). The effect of task structure on diffusion dynamics: implications for diffusion curve and network-based analyses. Learn. Behav. 38, 243–251.
Jenness, A. (1932). The role of discussion in changing opinion regarding a matter of fact. J. Abnorm. Soc. Psychol. 27, 279–296.
Kendal, J., Giraldeau, L.-A., and Laland, K. (2009). The evolution of social learning rules: payoff-biased and frequency-dependent biased transmission. J. Theor. Biol. 260, 210–219.
Klucharev, V., Hytönen, K., Rijpkema, M., Smidts, A., and Fernández, G. (2009). Reinforcement learning signal predicts social conformity. Neuron 61, 140–151.
Koenig, M. A., and Harris, P. L. (2007). The basis of epistemic trust: reliable testimony or reliable sources? Episteme 4, 264–284.
Koenig, M. A., and Harris, P. L. (2005). Preschoolers mistrust ignorant and inaccurate speakers. Child Dev. 76, 1261–1277.
Konopasky, R., and Telegdy, G. (1977). Conformity in the rat: a leader’s selection of door color versus a learned door-color discrimination. Percept. Motor Skills 44, 31–37.
Laland, K. N., Atton, N., and Webster, M. M. (2011a). From fish to fashion: experimental and theoretical insights into the evolution of culture. Philos. Trans. R. Soc. Lond. B Biol. Sci. 366, 958–968.
Laland, K. N., Sterelny, K., Odling-Smee, J., Hoppitt, W., and Uller, T. (2011b). Cause and effect in biology revisited: is Mayr’s proximate-ultimate dichotomy still useful? Science 334, 1512–1516.
Latane, B., and Wolf, S. (1981). The social impact of majorities and minorities. Psychol. Rev. 88, 438–453.
Lumsden, C. J., and Wilson, E. O. (1981). Genes, Mind, and Culture: The Coevolutionary Process. Cambridge: Harvard University Press.
Mason, M. F., Dyer, R., and Norton, M. I. (2009). Neural mechanisms of social influence. Org. Behav. Hum. Decision Process. 110, 152–159.
McElreath, R., Lubell, M., Richerson, P., Waring, T., Baum, W., Edsten, E., Efferson, C., and Paciotti, B. (2005). Applying evolutionary models to the laboratory study of social learning. Evol. Hum. Behav. 26, 483–508.
Morgan, T., Rendell, L., Ehn, M., Hoppitt, W., and Laland, K. (2011). The evolutionary basis of human social learning. Proc. R. Soc. B Biol. Sci. 279, 653–662.
Mullen, B. (1985). Strength and immediacy of sources –a meta-analytic evaluation of the forgotten elements of social impact theory. J. Pers. Soc. Psychol. 48, 1458–1466.
Mullen, B. (1986). Effects of strength and immediacy in group contexts: reply to Jackson. J. Pers. Soc. Psychol. 50, 514–516.
Nowak, A., Szamrej, J., and Latane, B. (1990). From private attitude to public-opinion – a dynamic theory of social impact. Psychol. Rev. 97, 362–376.
Pike, T. W., and Laland, K. N. (2010). Conformist learning in nine-spined sticklebacks’ foraging decisions. Biol. Lett. 6, 466–468.
Richerson, P. J., and Boyd, R. (2005). Not by Genes Alone: How Culture Transformed Human Evolution. Chicago: University of Chicago Press.
Schlag, K. (1998). Why imitate, and if so, how?: a boundedly rational approach to multi-armed bandits. J. Econ. Theor. 78, 130–156.
Shepard, R. N., and Metzler, J. (1971). Mental rotation of three-dimensional objects. Science 171, 701.
Tanford, S., and Penrod, S. (1984). Social-influence model – a formal integration of research on majority and minority influence processes. Psychol. Bull. 95, 189–225.
Toelch, U., Bruce, M. J., Meeus, M. T. H., and Reader, S. M. (2010). Humans copy rapidly increasing choices in a multiarmed bandit problem. Evol. Hum. Behav. 31, 326–333.
Tong, E. M. W., Tan, C. R. M., Latheef, N. A., Selamat, M. F. B., and Tan, D. K. B. (2008). Conformity: moods matter. Eur. J. Soc. Psychol. 38, 601–611.
Wakano, J. Y., and Aoki, K. (2007). Do social learning and conformist bias coevolve? Henrich and Boyd revisited. Theor. Popul. Biol. 72, 504–512.
Keywords: conformity, social learning, cultural transmission, cultural evolution
Citation: Morgan TJH and Laland KN (2012) The biological bases of conformity. Front. Neurosci. 6:87. doi: 10.3389/fnins.2012.00087
Received: 07 March 2012; Paper pending published: 04 April 2012;
Accepted: 24 May 2012; Published online: 14 June 2012.
Edited by:
Guido Biele, University of Oslo, NorwayReviewed by:
Carolyn Yoon, University of Michigan, USAChristopher J. Burke, Harvard College Observatory, USA
Copyright: © 2012 Morgan and Laland. This is an open-access article distributed under the terms of the Creative Commons Attribution Non Commercial License, which permits non-commercial use, distribution, and reproduction in other forums, provided the original authors and source are credited.
*Correspondence: K. N. Laland, School of Biology, University of St Andrews, Bute Medical Buildings, Westburn Lane, St Andrews, KY16 9TS Fife, UK. e-mail: knl1@st-andrews.ac.uk