- 1 Neurobiology and Animal Physiology, Faculty of Biology I, University of Freiburg, Freiburg, Germany
- 2 Bernstein Center Freiburg, University of Freiburg, Freiburg, Germany
- 3 Department of Bioengineering, Imperial College London, London, UK
- 4 Department of Electrical and Electronic Engineering, Imperial College London, London, UK
- 5 Epilepsy Center, University Medical Center Freiburg, Freiburg, Germany
- 6 Neurobiology and Biophysics, Faculty of Biology III, University of Freiburg, Freiburg, Germany
The spectral power of intracranial field potentials shows movement-related modulations during reaching movements to different target positions that in frequencies up to the high-γ range (approximately 50 to above 200 Hz) can be reliably used for single-trial inference of movement parameters. However, identifying spectral power modulations suitable for single-trial analysis for non-invasive approaches remains a challenge. We recorded non-invasive electroencephalography (EEG) during a self-paced center-out and center-in arm movement task, resulting in eight reaching movement classes (four center-out, four center-in). We found distinct slow (≤5 Hz), μ (7.5–10 Hz), β (12.5–25 Hz), low-γ (approximately 27.5–50 Hz), and high-γ (above 50 Hz) movement onset- and end-related responses. Movement class-specific spectral power modulations were restricted to the β band at approximately 1 s after movement end and could be explained by the sensitivity of this response to different static, post-movement electromyography (EMG) levels. Based on the β band, significant single-trial inference of reaching movement endpoints was possible. The findings of the present study support the idea that single-trial decoding of different reaching movements from non-invasive EEG spectral power modulations is possible, but also suggest that the informative time window is after movement end and that the informative frequency range is restricted to the β band.
Introduction
Traditionally, movement-related EEG responses have been investigated up to the β range, i.e., in frequencies below 30 Hz (Pfurtscheller et al., 1997). From intracranial recordings, however, it has become evident that the motor cortex generates a broad-banded spectral power increase in the high-γ range, i.e., from approximately 50 Hz up to above 200 Hz (Crone et al., 1998; Miller et al., 2007). This movement-related high-γ response has been proposed to be closely related to cortical function, both in space and time, and is therefore especially promising for investigating brain function and for potential brain–machine-interface (BMI) applications (Crone et al., 2006). In non-invasively recorded EEG, this cortical motor response thus far remained elusive. Reports on movement-related responses in higher frequencies have been rare and it was unclear whether they represented movement-related brain activity or, alternatively, electromyographic (EMG) artifacts or whether they could be attributed to, e.g., visual task components. Recently, however, it was demonstrated that, with optimized techniques, movement-related high-γ responses of the motor cortex are detectable non-invasively in the EEG (Ball et al., 2008; Darvas et al., 2010).
A hallmark of the movement-related high-γ response is its directional sensitivity, which has so far been described in detail in intracranial field recordings (Rickert et al., 2005; Pistohl et al., 2008; Ball et al., 2009b). Currently, similar data for the non-invasive EEG is lacking. In the present study, we address this point by investigating the signal-to-noise ratio (SNR) of directional tuning of non-invasive EEG spectral power modulations during a center-out task. Investigations of neural responses during center-out tasks usually focus on the responses triggered by the visual go cue or, alternatively, arm movement onset (Georgopoulos et al., 1982). We found previously that movement end during center-out and center-in movements – defined as the time point when the target is reached – is associated with a peak in the time course of the high-γ activity (Ball et al., 2008). This movement end-related high-γ response was distinct from the first high-γ peak found around movement onset. Here we further analyze the data of our previous EEG study and extend our analyses to spectral power responses occurring after movement end.
A well-characterized movement end-related EEG response is the so-called β rebound, a post-movement power increase in the β band following the typical suppression of β power during movement execution. The β rebound has been interpreted as reflecting a return to post-movement idling or readiness state in the motor cortex (Pfurtscheller et al., 1996), integration of distributed neural activity along the cortico-spinal pathway (Feige et al., 2000), or somatosensory processing (Cassim et al., 2001). The β rebound after movement imagination has been successfully used for BMI applications that communicate binary decisions based on recordings from a single EEG electrode (Bai et al., 2008; Pfurtscheller and Solis-Escalante, 2009). Our findings indicate that the β rebound is the only robust EEG spectral power component modulated by arm movement class and part of a more broad-banded post-movement power increase, including both lower (μ range) and higher (γ range) frequency bands.
Materials and Methods
Subjects
Eight subjects (four females, four males, mean age = 24 years, range = 20–29 years) participated in this study after giving their written informed consent. The study was approved by the Ethics Committee of the University of Freiburg. All participants were self-reportedly without a history of psychiatric or neurological diseases and without previous experience with a similar experimental setup. According to the Edinburgh handedness questionnaire (Oldfield, 1971), all subjects were right-handed (mean = 89%, range = 70–100%).
Experimental Task
Experimental methods and the setup have been described earlier (Ball et al., 2008). Briefly, the task was an un-cued, self-paced center-out, and center-in arm reaching task. There was no visual feedback as the right arm performing the task was shielded from the subjects’ view. The subjects fixed their gaze on a fixation point in front of them. They were instructed to freely choose the direction of each center-out movement out of four horizontal directions (rightward, forward, leftward, and backward), to stop their arm at the outer target position, to wait for approximately 4–10 s, and then to perform the consecutive center-in movement. Targets consisted of buttons with a diameter of 4 cm mounted on a table. Inter-button distances were 20 cm. The center-out and center-in movements were analyzed separately, thus yielding eight movement classes (see Figure 1).
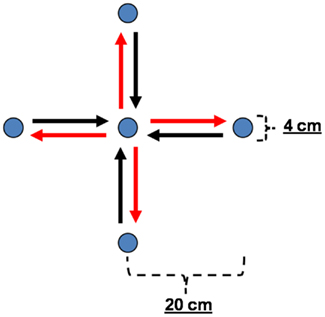
Figure 1. Experimental paradigm. Sketch of the center-out/center-in arm movement task with arrows representing the eight movement classes used for the analyses, i.e., four center-out (red) and four center-in (black) movements. Targets consisted of buttons with a diameter of 4 cm mounted on a table. Inter-button distances were 20 cm.
Data Recording and Preprocessing
Electrical potentials (bandwidth: 0.05–500 Hz) were recorded from 58 standard scalp positions (Sharbrough et al., 1991; see Figure 2A). All channels were recorded against a reference electrode at the vertex (CZ), which is less affected by EMG contamination than more peripheral recording sites (Goncharova et al., 2003). The ground electrode was positioned in the right occipital region (O2). EEG data was visually inspected from 0.88 s before movement onset (defined as the time point when the hand released a button), to 2.12 s after movement end (defined as the time point when the hand reached the target button). We were constrained to this time span because data quality was suboptimal at times further away from movement execution, i.e., during the subjects’ waiting and resting periods between movements. This is reflected by a moderately lower number of trials included after artifact rejection in the present as compared to our previous study (Ball et al., 2008). Moreover, we limited, for each subject, the trials per movement class to be included into the analyses to the minimal number of trials across all movement classes (Table 1). This step allowed us to obtain representative spectral power averages across trials. The number of trials per movement class that were further analyzed ranged from 43 (in subject 1) to 72 (in subject 8). Two sets of data were generated, one using movement onset as trigger and another using movement end as trigger. The data was re-referenced to a common average.
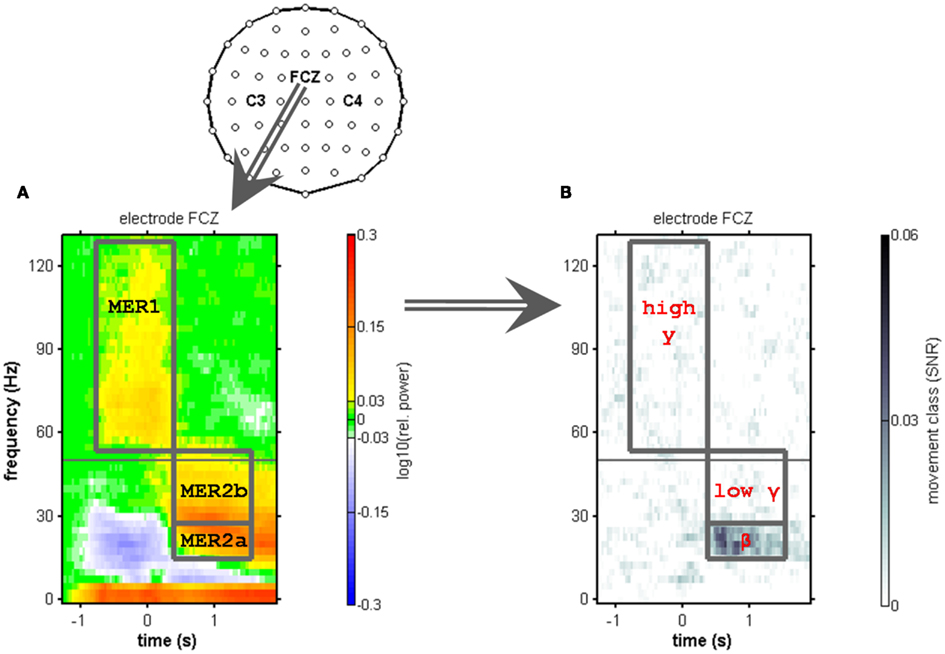
Figure 2. Electrode layout and definition of movement end-related responses using the electrode position FCZ. Anterior is to the top of the topographic map. (A) Median relative spectral power changes across subjects at electrode position FCZ. Movements are aligned to movement end (t = 0 s). The gray horizontal line indicates 50 Hz (the line-hum frequency), where spectral power changes could not be reliably evaluated. A power increase in low frequencies (≤5 Hz) and a suppression of power in the μ and β range (7.5–35 Hz) were observed. At and before movement end, a broad-banded power increase was found in the high-γ frequencies (55–120 Hz range). We refer to this high-γ response as movement end-related response 1 (MER1). After movement end, a power increase involving the β and low-γ frequencies up to approximately 50 Hz is referred to as movement end-related response 2 (MER2). Within the MER2, we distinguished a low (MER2a) and a high (MER2b) component, corresponding to the β and low-γ frequency range. (B) Median signal-to-noise ratio (SNR) of movement class tuning across subjects at the same electrode position (FCZ). The only spectral component that showed robust tuning was the MER2a component (12.5–25 Hz).
Time–Frequency Analysis
We computed a time-resolved Fourier transform. The window length was 400 ms; the time step was 40 ms and the frequency resolution was at 2.5 Hz. Spectral densities were estimated with a multitaper method (Park et al., 1987) and normalized by the number of sample points, thereby reflecting EEG amplitudes in Volts per Hertz. To compute relative spectral power changes, we defined as baseline the period from 0.88 to 0.28 s before movement onset. The data set aligned to movement onset, as well as the data set aligned to movement end, was analyzed against this baseline. A Wilcoxon signed-rank test was used to test for significance of spectral power changes relative to the baseline. A false discovery rate (FDR) procedure (Benjamini and Hochberg, 1995) applicable for the case of correlated P-values was used to account for multiple testing due to the high number of time–frequency bins.
Movement Class Tuning Analysis
The motivation for this study was to detect movement class tuning of spectral power changes with respect to the eight movement classes in our center-out/center-in paradigm. We calculated the SNR of movement class tuning for every frequency bin separately using the approach by Mehring et al. (2003) including their bias correction proposed for a limited number of trials in the presence of noise. Significance levels were calculated with shuffled data, mislabeling the movement classes at random 10,000 times. The same FDR procedure as in the time–frequency analysis (see above) was used.
Averaging Across Subjects
Whenever averages across subjects were computed, we calculated the median effect. Also, when analyses were mean-based on the single-subject level, we calculated median effects on the group-level in order to be robust against inter-subject variability. SEs of the median were computed using the quantile-based method of Sachs (1997).
Definition of Movement End-Related Responses
As illustrated in Figure 2, we identified a movement end-related response 1 (MER1) at and shortly before movement end, consisting of a broad-banded high-γ increase. We further identified a movement end-related response 2 (MER2) at the latency of the classical β rebound, consisting of a broad-banded post-movement spectral power increase involving frequencies up to approximately 50 Hz. Part of the MER2 displayed movement class tuning as quantified by the SNR (c.f. Figure 2B). Hence, we sub-divided the MER2 into a lower-frequency component (12.5–25 Hz) with movement class tuning (MER2a) and into a higher-frequency component (27.5–45 Hz) without tuning (MER2b). The lower component overlaps largely with the classical β band. The higher component corresponds to the low-γ range. We optimized the MER2a component frequency range for every subject individually using a fixed bandwidth of 12.5 Hz based on SNR increases at electrode positions above the sensorimotor areas (see Figure 5; Table 2). We then defined the MER2b component as part of the time–frequency plane just above the MER2a component encompassing frequencies up to 45 Hz, i.e., up to just below the line-hum frequency.
EMG Preprocessing
The right deltoid and the right flexor digitorum longus muscle, pars indicis, were recorded with electrodes attached to the skin surface in all subjects. EMG activity was high-pass-filtered at 60 Hz applying a sixth order Chebyshev filter twice (forward and backward). The high-pass-filtered EMG activity was squared and logarithmically scaled. The resulting signal was smoothed applying a forth order Savitzky–Golay filter of window length 401.6 ms and, subsequently, downsampled to 25 Hz.
EEG and EMG Signal Correlations
Signal correlations between the lower MER2 component and contralateral arm EMGs were assessed by computing the Spearman rank correlation between the average activities per movement class of EEG and EMG. The significance of Spearman’s rho was assessed using algorithm AS 89 (Best and Roberts, 1975). Finally, the significance of the rank correlation was FDR-corrected (see above) for testing at multiple time points.
Single-Trial Inference of Movement Classes
Single-trial inference of movement classes from EEG or EMG activity was achieved using a regularized linear discriminant analysis (Friedman, 1989). The regularization parameter was optimized on the training set. Trials to train the discriminant were excluded from the test set and the percentage of correctly classified trials, further referred to as decoding accuracy (DA), was calculated using 20-fold cross-validation (Efron and Tibshirani, 1994). We tested every subject’s DA for significance against a binomial cumulative distribution (Mehring et al., 2003). For EEG movement inference analyses we used time- and band-averaged spectral power from electrodes above the sensorimotor areas contralateral to the side of movement, MER2a component in our terminology (see above). The electrodes used for inference were determined based on significant SNR of movement class tuning in at least five of the total eight subjects and were fixed across subjects at positions FC1, C1, C3, CP1, and CP3 (see group results in Figure 6F). For EMG movement inference analyses we used the time-averaged EMG corresponding to the EEG time window. The time window was fixed across subjects to 0.48–1.44 s after movement end (see Figures 2, 6, and 7).
Results
As we have previously shown for the same data set (Ball et al., 2008), there are two peaks in the time course of the movement-related high-γ response, one around movement onset and one around movement end. The same spectral power changes are evident from Figures 3–6 of the present study. Due to the longer time window analyzed in the present as compared to the previous study, spectral power modulations occurring after movement end could be evaluated. This revealed an additional movement end-related response. We refer to the two movement end-related responses as MER1 and MER2 (see Figure 2). The post-movement MER2 included, but was not restricted to, the β rebound, and it typically involved both lower (μ) and also higher (low-γ) frequencies up to approximately 50 Hz. It was preceded by the typical suppression of μ and β range power in most of the subjects, sometimes also including a low-γ band spectral power suppression (Figure 5). Our results on the single-subject level showed significant MER2 occurring in EEG channels with and without a MER1. When both MER1 and MER2 were present, there was, in half of the subjects, a continuous transition from the low MER1 frequencies to the high-frequency MER2 component. This continuous transition was also evident from the group results (Figure 6A). The group results revealed a lateralization of both MER1 and MER2 with markedly reduced responses at the ipsilateral electrode position C4 (Figures 6A–C). For reasons of clarity, we did not show ipsilateral electrode positions in the individual results.
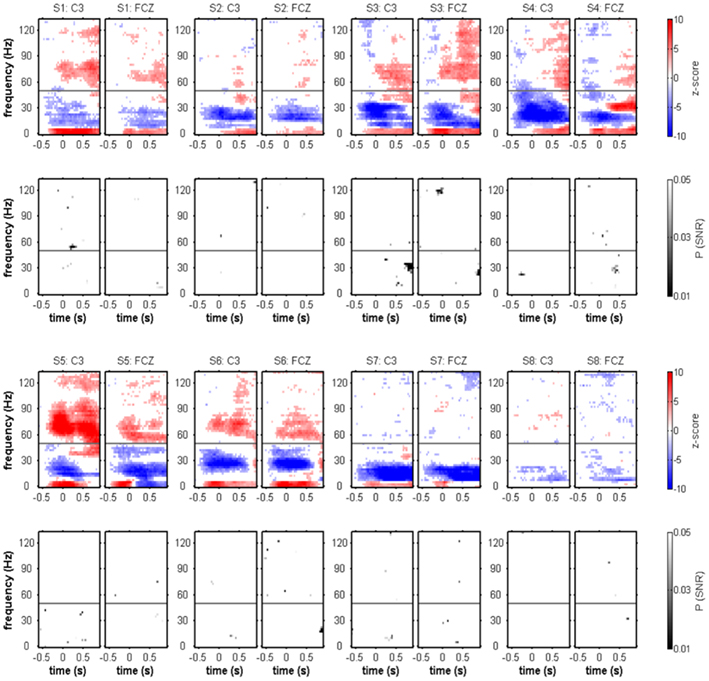
Figure 3. Movement onset-aligned individual spectral power changes and SNR of movement class tuning. For each of the subjects, Z-scores of the evoked response (color-coded: movement class independent) and P-values of the movement class tuning (grayscale-coded: movement class-dependent) at the electrode positions C3 and FCZ are shown. Only significant effects (P < 0.05, FDR-corrected) are displayed. Typical movement onset-related spectral power changes were power decreases (blue) predominating in the μ and β frequency range and power increases (red) in both low frequencies (≤5 Hz) and high-γ frequencies. Although SNR became significant in a few time–frequency bins, there was no clear and reproducible pattern of significant movement class-related SNR across subjects. The gray horizontal line indicates 50 Hz (the line-hum frequency), where spectral power changes could not be reliably evaluated.
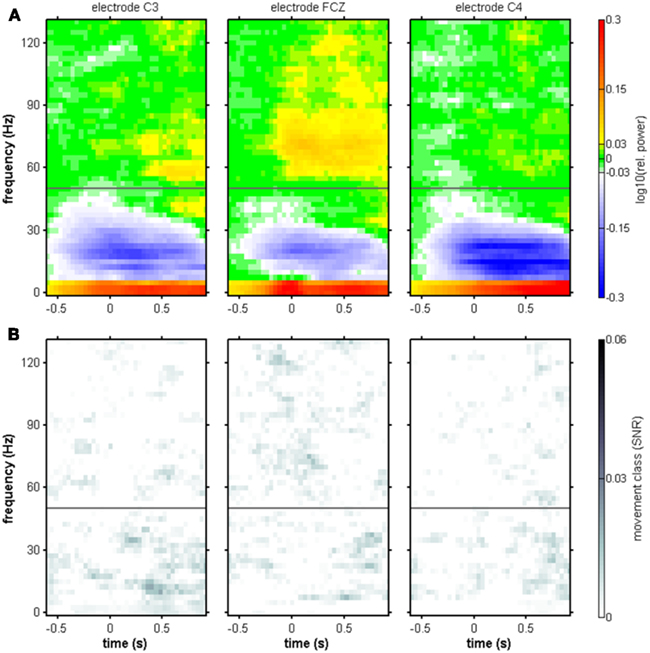
Figure 4. Movement onset-aligned group-averaged spectral power changes and SNR of movement class tuning. Electrode positions C3, FCZ, and C4. (A) Median relative spectral power changes across subjects. As in the individual results in Figure 3, power increases in low (≤5 Hz) and high-γ frequencies (55–120 Hz range), as well as a suppression of power in the μ and β range (7.5–35 Hz), were observed. (B) Median SNR of movement class tuning across subjects. As in the individual results in Figure 3, no clear pattern of movement class-related SNR was apparent.
To test for movement class tuning, a published SNR measure was used (see Materials and Methods). When investigating the SNR of movement class tuning before movement onset and during movement execution in the individual subjects, there was no reproducible pattern of significant SNR increases (Figure 3). This was also true when computing the SNR only for the four center-out movements (results not shown). At approximately 1 s after movement end, however, a prominent SNR increase was present in the β range (12.5–25 Hz, see Figure 5; Table 2). We called this component MER2a. Thus, the MER2a was the only response where we found significant movement class tuning in a reproducible manner across subjects. Note that the two electrode positions on display in Figure 5 are not covering the complete sensorimotor regions where we found significant SNR (c.f. Figure 6F). The group results reveal a lateralization of the MER2a with markedly reduced SNR at the ipsilateral electrode position C4 (Figures 6D,F). The low-γ frequency component MER2b was not modulated by movement class (Figures 5 and 6).
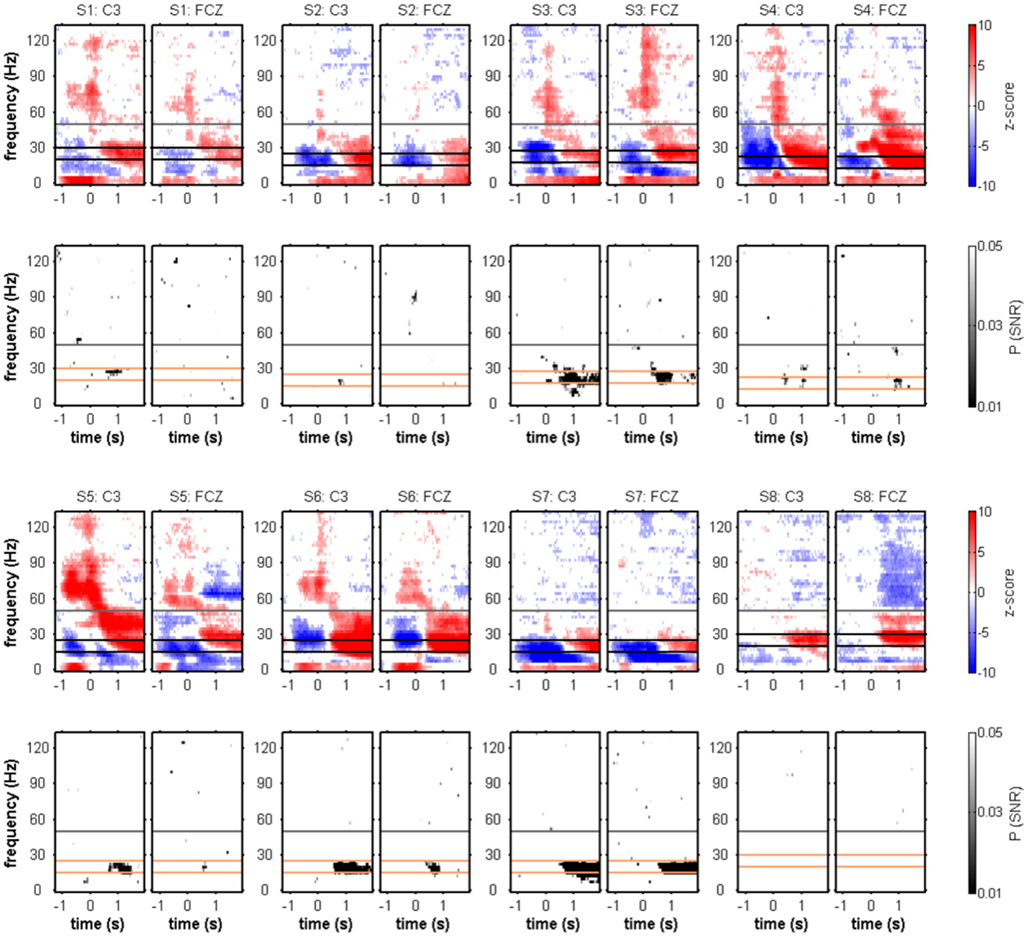
Figure 5. Movement end-aligned individual spectral power changes and SNR of movement class tuning. Conventions as in Figure 3. MER1 and MER2 (see Figure 2) were evident in the individual subjects. Four subjects (S3–6) showed a continuous transition from MER1 to MER2 at either electrode position C3 or FCZ, or both. A reproducible pattern of significant movement class-related SNR could be identified, consisting of an SNR increase in the β band at approximately 1 s after movement end. The orange horizontal lines delimit the 12.5-Hz wide frequency band used for further movement class tuning analyses (see Table 2).
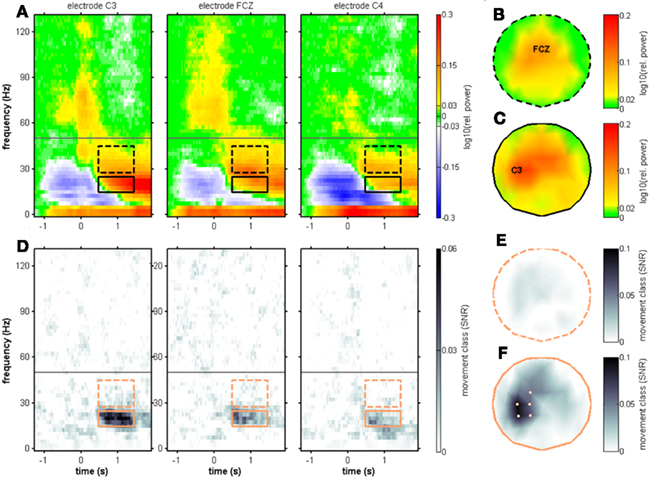
Figure 6. Movement end-aligned group-averaged spectral power changes and SNR of movement class tuning. Electrode positions C3, FCZ, and C4. The only spectral component showing a robust movement class tuning across subjects was the MER2a component and is depicted with solid black boxes. Dashed black boxes represent the MER2b component. (A) Median relative spectral power changes across subjects. The scalp topography for the MER2b component is shown in (B) with its maximum at the electrode position FCZ. The scalp topography for the MER2a component is shown in (C) with its maximum at the electrode position C3. (D) Median SNR of movement class tuning across subjects. A clear SNR increase was found, confirming the individual results in Figure 5. (E) Scalp topography of the SNR of the MER2b component. There were no significantly tuned electrode sites (P < 0.01, FDR-corrected for testing at multiple electrode positions). (F) Scalp topography of the SNR of the MER2a component. The electrode positions FC1, C1, C3, CP1, and CP3 above left sensorimotor areas contralateral to the side of movement where the individual results were significant in at least five of the eight subjects (P < 0.01, FDR-corrected) are highlighted.
To examine the basis of the observed movement class tuning, we tested the hypothesis that differences in EMG activity persisting beyond movement end might explain differences in the MER2a. As a first step, we determined, for each subject individually, a pair of center-out/center-in movements for which the MER2a differences were most pronounced. We indeed found that EMG levels for this individually selected pair of movements were different: EMG levels were lower at the center position as compared to an outer target position. At the same time, MER2a band power was higher at the center position as compared to an outer target position (Figures 7A–C). If the two phenomena were generally related in a reciprocal fashion, as suggested by these results, movement endpoints with low EMG levels should be characterized by relatively high MER2a band power, and vice versa. To test for this, we calculated, for each subject, signal correlations between the movement class tuning of the EMG and the movement class tuning of the MER2a band component. Indeed, all subjects showed significant negative signal correlations with respect to either the deltoid or index-finger muscle activity, or both (Figure 7; Table 3).
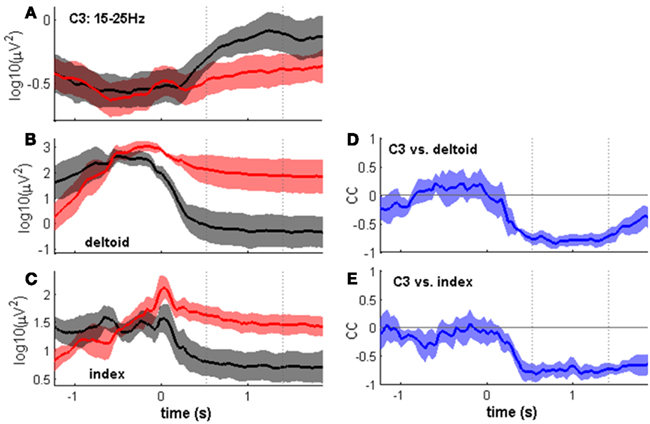
Figure 7. Time course of the MER2a component and of simultaneous EMG activity. All panels show the median time course across subjects ± SEs of the median, aligned to movement end. Dotted lines indicate the post-movement time window of 0.96 s length as in Figures 2 and 6. (A–C) Show center-out movements in red and center-in movements in black (c.f. Figure 1). To examine the basis of the observed movement class tuning, we selected, for each subject, the pair of a center-out and a center-in movement class for which the modulation was most pronounced. (A) Time course of the MER2a component at electrode position C3. (B,C) Same as (A) but for EMG of the contralateral deltoid (B) and flexor indicis (C) muscles. In both cases, EMG levels were higher after the center-out than after the center-in movement. (D,E) Signal correlations (correlation coefficients (cc) on the y axis) between the MER2a component and deltoid EMG (D) and flexor indicis EMG (E) using the trial-averaged activities of all movement classes (see Table 3).

Table 3. Signal correlations between the lower MER2 component at the electrode position C3 and EMG activity.
Finally, we investigated whether the observed EEG spectral power modulations allowed for single-trial decoding of movement endpoints during the center-out and center-in task (Figure 8). We examined a number of scenarios: decoding the four different endpoints of the center-out movements, decoding the endpoints of the four center-in movements (which terminated all on the single center button), and decoding the endpoints of the individual pairs of center-out/center-in movement classes with the highest discriminability (Table 4). Further, we also tested inferring a center-out as opposed to a center-in movement. In all cases, we compared the EEG-based DA with the DA of decoding from contralateral arm EMG (deltoid and flexor indicis EMG). We found that EEG-based decoding yielded significant decoding power in the four scenarios tested in 3, 0, 7, and 6 subjects, respectively. EMG-based decoding was always higher in comparison and was possible for all or most of the subjects in the four scenarios tested.
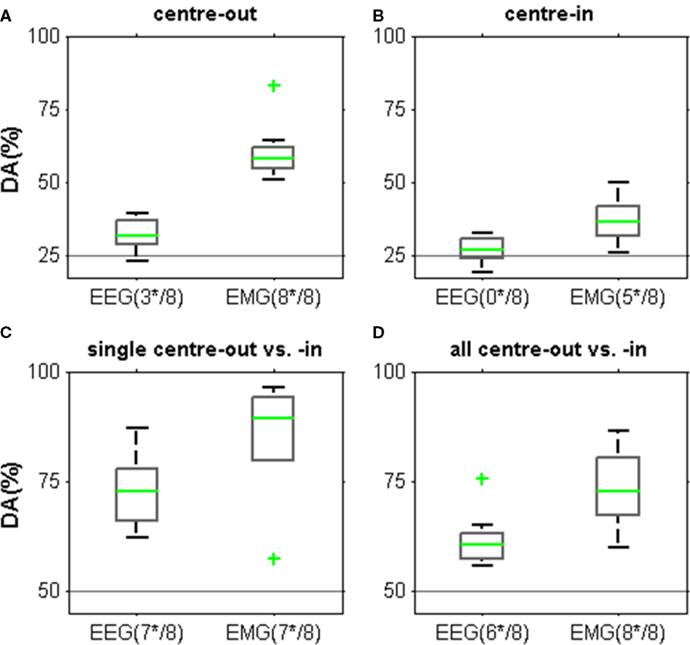
Figure 8. Decoding endpoints of arm movements from the MER2a EEG component and from arm-muscle EMG, both for a time window at approximately 1 s after movement end (c.f. Figure 7). Horizontal lines indicate chance level. The number of subjects with significant DA is indicated below each box plot (e.g., “(3*/8)” = 3 of the 8 subjects). Box plot whiskers extend to DAs within 1.5 times the inter-quartile range, the median, and outliers are indicated in green. (A) Decoding results for the endpoints of center-out movements. EEG-based DA was significantly above chance in three subjects. EMG-based DA was significant in all subjects. (B) Decoding results for the endpoints of center-in movements (which terminated all on the center button). There was no significant EEG-based DA for any of the subjects. EMG-based DA was lower than for the endpoints of center-out movements, yielding significant DA in five subjects. (C) Decoding results for the endpoints of the best pair of a center-out and a center-in movement in each subject (see Table 4). This yielded highest EEG and also EMG DA values. (D) Decoding whether an endpoint was reached after center-out as opposed to center-in movements based on all eight movement classes yielded lower DA values than (C).
Discussion
In the present study, we investigated movement class sensitivity of time-resolved EEG spectral power changes in different frequency bands recorded non-invasively during a center-out/center-in arm reaching task. Recently, such movement class sensitivity has been examined in a range of neural population signals, i.e., in the intracortical local field potential from monkey motor cortex (Rickert et al., 2005; Stark and Abeles, 2007), in the electrocorticogram recorded with subdurally implanted electrodes in humans (Pistohl et al., 2008, 2012; Ball et al., 2009b), and in the non-invasively recorded magnetoencephalogram (MEG; Waldert et al., 2008). All of these studies investigated spectral power modulations up to the high-γ range. Using EEG, decoding parameters of movements has been previously performed based on the low-pass-filtered component, roughly corresponding to the delta frequency range (Bradberry et al., 2010; Presacco et al., 2011, 2012) as well as using alpha and beta band EEG (Yuan et al., 2010), but comparable data for the γ frequency ranges was still lacking. Extracting movement-related directional information from different, and possibly complementary, signal components can be expected to improve the performance of BMI applications for paralyzed patients, which rely on single-trial inference of movement parameters from neural population signals. Moreover, understanding the representation of movement parameters in different neural population signals corresponding to different levels of spatial resolution may reveal new insights into the functional organization of the motor cortex.
The findings of the present study show that, although broad-banded movement-related high-γ responses are clearly detectable in non-invasive EEG, they exhibit no significant movement class tuning. This finding is in line with recent MEG results: by decoding four classes of joystick movements in a center-out task, movement-related power increases up to 90 Hz were found, but no significant single-trial movement class inference from the γ band was possible (Waldert et al., 2008). The present study, together with this previous report, supports the detectability of high-γ activity, but does not support single-trial inference of movement parameters from high-γ EEG/MEG activity. This seems to require intracranial recordings and represents, up to now, a limitation of non-invasive recordings due to a lower SNR and, hence, a lower signal quality (Ball et al., 2009a). An explanation might be that, if the tuning of high-γ activity changes on small spatial scales in the cortex, the amount of detectable tuning may be reduced in the spatially smoothed version of this activity as is provided by the non-invasive EEG measures (but, see Waldert et al., 2009). Additionally, it has been suggested that much higher spatial sampling (“micro-EEG”) than used in the present study (58 EEG channels distributed over the scalp in an approximately equidistant fashion, see Materials and Methods) would be required for optimal detection of γ activity in scalp EEG (Freeman et al., 2000). Thus, detection of movement class-related γ band modulations in non-invasive EEG may, in the future, become possible with more sophisticated EEG recording techniques or with advances in EEG signal processing.
We found movement class-dependent modulation of β band activity occurring at approximately 1 s after movement end. This time–frequency window overlaps with the well-known β rebound (Pfurtscheller, 1981). Our results demonstrate that the post-movement power increases at the latency of the classical β rebound are, in most subjects, not restricted to the β band. Often, neighboring frequencies also showed power increases, particularly the adjacent lower (μ) and higher (low-γ up to approximately 50 Hz) bands, and these power increases were not always preceded by power suppression in the same frequencies. Thus, the present EEG data indicate that the classical β rebound is embedded in a more broad-banded post-movement power increase, MER2 in our terminology. The MER2 can be distinguished from the preceding broad-banded high-γ response at movement end (MER1) – although, in half of the subjects, a continuous transition between the two responses was observed. Whether this transition is due to the limited spatial resolution of the scalp EEG and whether it could also be observed on finer spatial scales remains to be determined.
The spatial distribution of movement class-dependent modulations is an important aspect of the present study. Generally, scalp EEG is prone to artifact contamination by, e.g., eye or head movements or EMG activity. If such artifacts occurred differently for different movement classes, they would contribute to an apparent directional tuning of the EEG. The scalp distribution of the observed effects provides useful information for distinguishing artifact and EEG activity. The lateralized distribution of movement class sensitivity with its maximum above the C3 electrode position is unlikely to result from any known class of EEG artifacts, as – across subjects – they are characterized by different topographic patterns [c.f. eye movements (Yuval-Greenberg and Deouell, 2009) and EMG artifacts (Goncharova et al., 2003)]. While the electrode positions with significant movement class tuning predominated above the sensorimotor areas contralateral to the side of movement, the MER2b component without movement class tuning predominated over the midline, pointing to frontal midline structures, including the supplementary motor area (Ohara et al., 2001). In summary, these findings contribute to a more fine-grained picture of post-movement spectral power modulations. We provide further evidence for functional differentiation within the EEG responses occurring at and after the end of voluntary arm movements (Stancák et al., 2000), thereby providing a useful framework for future studies.
In the present study, we found no robust movement class-dependent modulations in low frequencies (≤5 Hz). Similarly, Waldert et al. (2008) found only very low single-sensor DA based on MEG spectral power modulations in low frequencies (<7.5 Hz). They successfully increased their DA using an optimized electrode selection (34% for four movement directions). Waldert et al. found also DA based on MEG and EEG low-pass-filtered signals. Their DA based on the low-pass-filtered signal was substantially higher than the one based on spectral power modulations, pointing to movement class-related information in signal phase. Phase analysis of EEG signals was not in the scope of the present study, as our study was entirely focused on the analysis of movement-related EEG spectral power modulations. However, both phase analyses (Aoki et al., 1999) and a comparison of the time–frequency dynamics of EEG–EMG phase inter-relations (Feige et al., 2000) with the present results would be interesting topics for further research.
As a next step in our analyses, we addressed possible reasons for the movement class sensitivity of the MER2a activity. Even slight, albeit sustained stretching of the fingers reduces the amplitude of ß range spectral power responses evoked by median nerve stimulation (Schnitzler et al., 1997). Therefore, we hypothesized that differences in isometric muscle contractions at the endpoints of the different reaching movements might explain the observed β band modulations. To test this hypothesis, we first examined whether EMG activity differed for the different movement classes investigated and we indeed found sustained differences in EMG amplitudes after movement end (Figures 7B,C). The class of the preceding center-out movement (i.e., rightward, forward, leftward, or backward) could be inferred from the EMG in the post-movement time window in all subjects (Figure 8A). In the case of center-out movements, these EMG differences are likely to be explained by differences in the arm position at the different movement endpoints. The EMG-based DA in the post-movement time window was much weaker for center-in movements, but still significant in the majority of subjects (Figure 8B). All center-in movements had the same endpoint – therefore, successful trials (where this endpoint was reached, a selection criterion in the present study) did not allow for a large variability in arm position. The less pronounced EMG differences after center-in movements might be related to slight differences in the final arm position as a function of the direction of the preceding movement.
The crucial test in the context of the present study was whether the level of post-movement EMG and MER2a activity were correlated across the eight movement classes. Indeed, this was clearly the case (Figures 7D,E; Table 3). Correlations were always negative, in line with the previous finding that sustained muscle contraction suppresses somatosensory-evoked β band activity (Schnitzler et al., 1997). The correlation coefficients were often high (in the range of 0.9), but these high correlations occurred with deltoid muscle EMG in some subjects or with flexor indicis EMG in others, or with both. EMG of other arm muscles that were not recorded might explain additional variance in the EEG signals. Whether the fine-tuned β rebound modulations observed in the present study can also be obtained after imaginary movements or in paralyzed patients attempting to move remains to be determined. Considering the close relation of the β band to EMG activity (Witham et al., 2011), it appears possible that the β rebound modulations, as described here, might be reduced or even vanish without the production of EMG (Cassim et al., 2001).
Conflict of Interest Statement
The authors declare that the research was conducted in the absence of any commercial or financial relationships that could be construed as a potential conflict of interest.
Acknowledgments
This study was supported by the German Federal Ministry of Education and Research (BMBF Grants 01GQ0420 to BCCN, 01GQ0830 to BFNT Freiburg/Tübingen, and BMBF-GoBio). We thank Stephan Waldert for discussions on this manuscript.
References
Aoki, F., Fetz, E. E., Shupe, L., Lettich, E., and Ojemann, G. A. (1999). Increased gamma-range activity in human sensorimotor cortex during performance of visuomotor tasks. Clin. Neurophysiol. 110, 524–537.
Bai, O., Lin, P., Vorbach, S., Floeter, M. K., Hattori, N., and Hallett, M. (2008). A high performance sensorimotor beta rhythm-based brain-computer interface associated with human natural motor behavior. J. Neural Eng. 5, 24–35.
Ball, T., Demandt, E., Mutschler, I., Neitzel, E., Mehring, C., Vogt, K., Aertsen, A., and Schulze-Bonhage, A. (2008). Movement related activity in the high gamma range of the human EEG. Neuroimage 41, 302–310.
Ball, T., Kern, M., Mutschler, I., Aertsen, A., and Schulze-Bonhage, A. (2009a). Signal quality of simultaneously recorded invasive and non-invasive EEG. Neuroimage 46, 708–716.
Ball, T., Schulze-Bonhage, A., Aertsen, A., and Mehring, C. (2009b). Differential representation of arm movement direction in relation to cortical anatomy and function. J. Neural Eng. 6, 016006.
Benjamini, Y., and Hochberg, Y. (1995). Controlling the false discovery rate: a practical and powerful approach to multiple testing. J. R. Stat. Soc. Ser. B Methodol. 57, 289–300.
Best, D. J., and Roberts, D. E. (1975). Algorithm AS 89: the upper tail probabilities of Spearman’s rho. J. R. Stat. Soc. Ser. C Appl. Stat. 24, 377–379.
Bradberry, T. J., Gentili, R. J., and Contreras-Vidal, J. L. (2010). Reconstructing three-dimensional hand movements from noninvasive electroencephalographic signals. J. Neurosci. 30, 3432–3437.
Cassim, F., Monaca, C., Szurhaj, W., Bourriez, J. L., Defebvre, L., Derambure, P., and Guieu, J. D. (2001). Does post-movement beta synchronization reflect an idling motor cortex? Neuroreport 12, 3859–3863.
Crone, N. E., Miglioretti, D. L., Gordon, B., and Lesser, R. P. (1998). Functional mapping of human sensorimotor cortex with electrocorticographic spectral analysis. II. Event-related synchronization in the gamma band. Brain 121(Pt 12), 2301–2315.
Crone, N. E., Sinai, A., and Korzeniewska, A. (2006). High-frequency gamma oscillations and human brain mapping with electrocorticography. Prog. Brain Res. 159, 275–295.
Darvas, F., Scherer, R., Ojemann, J. G., Rao, R. P., Miller, K. J., and Sorensen, L. B. (2010). High gamma mapping using EEG. Neuroimage 49, 930–938.
Feige, B., Aertsen, A., and Kristeva-Feige, R. (2000). Dynamic synchronization between multiple cortical motor areas and muscle activity in phasic voluntary movements. J. Neurophysiol. 84, 2622–2629.
Freeman, W. J., Rogers, L. J., Holmes, M. D., and Silbergeld, D. L. (2000). Spatial spectral analysis of human electrocorticograms including the alpha and gamma bands. J. Neurosci. Methods 95, 111–121.
Georgopoulos, A. P., Kalaska, J. F., Caminiti, R., and Massey, J. T. (1982). On the relations between the direction of two-dimensional arm movements and cell discharge in primate motor cortex. J. Neurosci. 2, 1527–1537.
Goncharova, I. I., McFarland, D. J., Vaughan, T. M., and Wolpaw, J. R. (2003). EMG contamination of EEG: spectral and topographical characteristics. Clin. Neurophysiol. 114, 1580–1593.
Mehring, C., Rickert, J., Vaadia, E., Cardosa de Oliveira, S., Aertsen, A., and Rotter, S. (2003). Inference of hand movements from local field potentials in monkey motor cortex. Nat. Neurosci. 6, 1253–1254.
Miller, K. J., Leuthardt, E. C., Schalk, G., Rao, R. P. N., Anderson, N. R., Moran, D. W., Miller, J. W., and Ojemann, J. G. (2007). Spectral changes in cortical surface potentials during motor movement. J. Neurosci. 27, 2424–2432.
Ohara, S., Mima, T., Baba, K., Ikeda, A., Kunieda, T., Matsumoto, R., Yamamoto, J., Matsuhashi, M., Nagamine, T., Hirasawa, K., Hori, T., Mihara, T., Hashimoto, N., Salenius, S., and Shibasaki, H. (2001). Increased synchronization of cortical oscillatory activities between human supplementary motor and primary sensorimotor areas during voluntary movements. J. Neurosci. 21, 9377–9386.
Oldfield, R. C. (1971). The assessment and analysis of handedness: the Edinburgh inventory. Neuropsychologia 9, 97–113.
Park, J., Lindberg, C. R., and Vernon, F. L. (1987). Multitaper spectral analysis of high-frequency seismograms. J. Geophys. Res. 92, 12675–12684.
Pfurtscheller, G. (1981). Central beta rhythm during sensorimotor activities in man. Electroencephalogr. Clin. Neurophysiol. 51, 253–264.
Pfurtscheller, G., and Solis-Escalante, T. (2009). Could the beta rebound in the EEG be suitable to realize a “brain switch”? Clin. Neurophysiol. 120, 24–29.
Pfurtscheller, G., Stancák, A. Jr., and Edlinger, G. (1997). On the existence of different types of central beta rhythms below 30 Hz. Electroencephalogr. Clin. Neurophysiol. 102, 316–325.
Pfurtscheller, G., Stancák, A. Jr., and Neuper, C. (1996). Post-movement beta synchronization. A correlate of an idling motor area? Electroencephalogr. Clin. Neurophysiol. 98, 281–293.
Pistohl, T., Ball, T., Schulze-Bonhage, A., Aertsen, A., and Mehring, C. (2008). Prediction of arm movement trajectories from ECoG-recordings in humans. J. Neurosci. Methods 167, 105–114.
Pistohl, T., Schulze-Bonhage, A., Aertsen, A., Mehring, C., and Ball, T. (2012). Decoding natural grasp types from human ECoG. Neuroimage 59, 248–260.
Presacco, A., Forrester, L. W., and Contreras-Vidal, J. L. (2012). Decoding intra-limb and inter-limb kinematics during treadmill walking from scalp electroencephalographic (EEG) signals. IEEE Trans. Neural Syst. Rehabil. Eng. 20, 212–219.
Presacco, A., Goodman, R., Forrester, L., and Contreras-Vidal, J. L. (2011). Neural decoding of treadmill walking from noninvasive electroencephalographic signals. J. Neurophysiol. 106, 1875–1887.
Rickert, J., Oliveira, S. C., de Vaadia, E., Aertsen, A., Rotter, S., and Mehring, C. (2005). Encoding of movement direction in different frequency ranges of motor cortical local field potentials. J. Neurosci. 25, 8815–8824.
Schnitzler, A., Salenius, S., Salmelin, R., Jousmäki, V., and Hari, R. (1997). Involvement of primary motor cortex in motor imagery: a neuromagnetic study. Neuroimage 6, 201–208.
Sharbrough, F., Chatrian, G., Lesser, R. P., Lüders, H., Nuwer, M., and Picton, T. W. (1991). American Electroencephalographic Society guidelines for standard electrode position nomenclature. J. Clin. Neurophysiol. 8, 200–202.
Stancák, A. Jr., Feige, B., Lücking, C. H., and Kristeva-Feige, R. (2000). Oscillatory cortical activity and movement-related potentials in proximal and distal movements. Clin. Neurophysiol. 111, 636–650.
Stark, E., and Abeles, M. (2007). Predicting movement from multiunit activity. J. Neurosci. 27, 8387–8394.
Waldert, S., Pistohl, T., Braun, C., Ball, T., Aertsen, A., and Mehring, C. (2009). A review on directional information in neural signals for brain-machine interfaces. J. Physiol. Paris 103, 244–254.
Waldert, S., Preissl, H., Demandt, E., Braun, C., Birbaumer, N., Aertsen, A., and Mehring, C. (2008). Hand movement direction decoded from MEG and EEG. J. Neurosci. 28, 1000–1008.
Witham, C. L., Riddle, C. N., Baker, M. R., and Baker, S. N. (2011). Contributions of descending and ascending pathways to corticomuscular coherence in humans. J. Physiol. (Lond.) 589, 3789–3800.
Yuan, H., Perdoni, C., and He, B. (2010). Relationship between speed and EEG activity during imagined and executed hand movements. J. Neural Eng. 7, 26001.
Keywords: EEG, reaching, beta, gamma, movement onset, movement end, BMI, BCI
Citation: Demandt E, Mehring C, Vogt K, Schulze-Bonhage A, Aertsen A and Ball T (2012) Reaching movement onset- and end-related characteristics of EEG spectral power modulations. Front. Neurosci. 6:65. doi: 10.3389/fnins.2012.00065
Received: 08 March 2012; Paper pending published: 30 March 2012;
Accepted: 16 April 2012; Published online: 07 May 2012.
Edited by:
Cuntai Guan, Institute for Infocomm Research, SingaporeReviewed by:
Dennis J. McFarland, Wadsworth Center for Laboratories and Research, USAJose L. ‘Pepe’ Contreras-Vidal, University of Houston, USA
Copyright: © 2012 Demandt, Mehring, Vogt, Schulze-Bonhage, Aertsen and Ball. This is an open-access article distributed under the terms of the Creative Commons Attribution Non Commercial License, which permits non-commercial use, distribution, and reproduction in other forums, provided the original authors and source are credited.
*Correspondence: Tonio Ball, Epilepsy Center, University Medical Center Freiburg, Engelbergerstr. 21, 79106 Freiburg, Germany. e-mail: tonio.ball@uniklinik-freiburg.de