- 1 Psychological Sciences, Vanderbilt University, Nashville, TN, USA
- 2 Institute of Imaging Science, Vanderbilt University, Nashville, TN, USA
- 3 Department of Psychology, University of Basel, Basel, Switzerland
- 4 Department of Psychology, Stanford University, Stanford, CA, USA
- 5 Center for Cognitive Neuroscience, Duke University, Durham, NC, USA
Intertemporal choices are a ubiquitous class of decisions that involve selecting between outcomes available at different times in the future. We investigated the neural systems supporting intertemporal decisions in healthy younger and older adults. Using functional neuroimaging, we find that aging is associated with a shift in the brain areas that respond to delayed rewards. Although we replicate findings that brain regions associated with the mesolimbic dopamine system respond preferentially to immediate rewards, we find a separate region in the ventral striatum with very modest time dependence in older adults. Activation in this striatal region was relatively insensitive to delay in older but not younger adults. Since the dopamine system is believed to support associative learning about future rewards over time, our observed transfer of function may be due to greater experience with delayed rewards as people age. Identifying differences in the neural systems underlying these decisions may contribute to a more comprehensive model of age-related change in intertemporal choice.
Introduction
Intertemporal choice describes any decision making scenario that involves selecting between outcomes available at different times in the future. A broad range of decisions made in everyday life (e.g., healthy eating, retirement savings, exercise) require trade-offs between immediate satisfaction and long-term health and well-being. Economic models of age-related change in intertemporal preferences begin with assumptions about how utility changes across the life span. Assertions are made about reproductive fitness or the physical wherewithal available to enjoy rewards and then conclusions are drawn on this basis about how decision making ought to depend on age (Rogers, 1994; Trostel and Taylor, 2001; Read and Read, 2004). This approach has produced theories asserting that delay discounting (i.e., the preference for sooner, smaller rewards relative to larger, later rewards) should decline with age (Rogers, 1994), increase with age (Trostel and Taylor, 2001), or be minimized in middle age (Read and Read, 2004). Empirical results from psychology and behavioral economics are similarly conflicting (Green et al., 1994, 1996, 1999; Harrison et al., 2002; Read and Read, 2004; Chao et al., 2009; Reimers et al., 2009; Whelan and McHugh, 2009; Simon et al., 2010; Jimura et al., 2011; Löckenhoff et al., 2011). One important potential contribution to models of intertemporal choice over the life span, which has been overlooked to date, may be that older and younger adults rely differently on the brain systems that underlie valuation of future outcomes.
Decision neuroscience promises to enable a systems-level understanding of the neural and cognitive changes that underlie the age-dependence of intertemporal choice. Recent decision neuroscience research reveals a network of subcortical and cortical brain regions involved in intertemporal decision making (Peters and Büchel, 2011). Several studies have shown that regions associated with the mesolimbic dopamine system, including the ventral striatum (VS), ventromedial prefrontal cortex, and posterior cingulate cortex, play a primary role in the representation of subjective value (Kable and Glimcher, 2007; Peters and Büchel, 2009, 2010) and are more active in the presence of immediately available rewards in young adults (McClure et al., 2004, 2007; Luo et al., 2009). A different network of brain areas related to cognitive control including the dorso and ventrolateral prefrontal cortex (collectively, LPFC) and the posterior parietal cortex (PPC) has been proposed to promote the selection of relatively delayed outcomes (Peters and Büchel, 2011). Higher levels of activation in LPFC and PPC relative to mesolimbic regions is associated with selection of larger, delayed rewards (McClure et al., 2004), and disruption of left LPFC through transcranial magnetic stimulation leads to increased selection of immediate over delayed rewards (Figner et al., 2010). There is also related evidence that working memory-related anterior PFC activity is associated with increased selection of delayed outcomes (Shamosh et al., 2008). LPFC regions play a causal role in valuation and self-control during decision making (Camus et al., 2009), possibly through top-down interactions with medial prefrontal regions to bias choice toward options with better long-term over short-term value (Hare et al., 2009).
How age-related alterations in the mesolimbic dopamine system and lateral cortical regions combine to affect judgments is an active area of investigation (Mohr et al., 2009). Although some age-related impairments in risky decision making have been linked to cognitive limitations related to processing speed and memory (Henninger et al., 2010) or learning to implement cognitively demanding strategies (Mata et al., 2010), in other decision making scenarios that are not as cognitively demanding the LPFC is similarly engaged and performance is equal between younger and older adults (Hosseini et al., 2010). In contrast, age-related changes in the function of the mesolimbic dopamine system are the focus of the present work. Numerous neurophysiological changes are known to occur as people age. Age-related declines in the striatal dopamine receptors have been well documented and are linked to cognitive impairment (Bäckman et al., 2006). In fact, previous neuroimaging studies have attributed age-related deficits in decision making during novel probabilistic learning tasks to disruption of striatal signals (Mell et al., 2009; Samanez-Larkin et al., 2010).
Although there is evidence for age differences in the function of the striatum in time-limited learning tasks (Aizenstein et al., 2006; Mell et al., 2009; Samanez-Larkin et al., 2010), there is also evidence for stability in striatal responses correlated with reward magnitude (Samanez-Larkin et al., 2007, 2010; Schott et al., 2007; Cox et al., 2008). Behavioral experiments with animals have also demonstrated equivalent sensitivity to both magnitude and delay in younger and older rats (Simon et al., 2010), suggesting that the basic computational resources needed to make intertemporal decisions do not change much with age. Likewise, standard models of discounting fit the behavior of younger and older adults equally well (Green et al., 1999; Whelan and McHugh, 2009), suggesting that similar choice processes are involved. Thus, although the rate of discounting may differ, a differential structure of discounting functions between age groups does not explain any observed differences (Green et al., 1999). In spite of the age-related declines observed in the dopamine system and the striatum, it may be that gradual declines in the dopamine system with age do not disrupt the slow changes in associative learning from repeated experience with delayed rewards over decades of the life course. This experience with the realization of delayed rewards is highly relevant for making intertemporal decisions, as one has to make predictions about the future value of various courses of action at the time of choice (Löckenhoff, 2011; Löckenhoff et al., 2011).
Theories about dopamine function posit that these neurons signal reward value in mesolimbic regions as a consequence of direct associative learning (Montague et al., 1996; Schultz et al., 1997). Reinforcement learning models developed to capture these data are notoriously slow to learn about delayed outcomes (Sutton and Barto, 1998). As a consequence it may take substantial time and experience (Logue et al., 1984) for mesolimbic dopamine regions to develop robust responses to cues predicting rewards at long time delays. Although older adults may suffer from declines in fluid cognitive ability that may constrain their decision making competence, they also have decades of experience over their young adult counterparts with the realization of delayed rewards which may lead to similar decisions behaviorally (Agarwal et al., 2009). Thus, reasoning from such models, we did not make strong predictions about behavioral differences in decision making but did expect that older adults as compared to their younger counterparts may show increased mesolimbic responses to delayed rewards. In the present study, we examined age differences between healthy younger and older adults in the neural systems that support intertemporal decision making. We predicted that younger adults would show a larger difference in mesolimbic neural signal change in the presence of an immediately available reward, but that older adults would show similar levels of neural activation for immediate or delayed rewards.
Materials and Methods
Behavioral Task Design
Twelve younger adults (age range 19–26, mean 22.0; seven female) and 13 older adults (age range 63–85, mean 73.4; six female) completed an intertemporal decision making task while undergoing functional magnetic resonance imaging (fMRI). Older adults were screened with the Mini-Mental State Exam prior to participation to ensure that individuals at risk for Mild Cognitive Impairment or dementia were excluded from participation (all scores above 25). All subjects gave written informed consent, and the experiment was approved by the Institutional Review Board of Stanford University. We measured the blood-oxygen-level-dependent (BOLD) signal of subjects as they made a series of intertemporal choices between early monetary rewards ($R available at delay d; R = reward, d = delay) and later monetary rewards ($R′ available at delay d′; d′ > d; following the methods of McClure et al., 2004). On each trial, subjects viewed the two options, pressed a button to make a selection, and their choice was highlighted on the screen (Figure 1). The task was incentive-compatible. At the end of the experiment one trial was selected at random to be paid out at the chosen time (personal checks were used for both immediate and delayed rewards). All subjects responded with the right hand (index finger for choice on the left, middle finger for choice on the right). The total trial length including the inter-trial interval was 12 s. Decisions were self-paced, the highlighted choice was displayed for 2 s, and the inter-trial interval was set to 10 s minus choice reaction time. Older adults responded more slowly than younger adults, t23 = 2.79, p < 0.05, but on average both groups responded within 4 s (older mean = 3.3 s, SD = 0.25 s; younger mean = 2.4 s, SD = 0.21 s). Adding reaction time as a covariate to any of the analyses reported does not change any of the effects. All significant effects remain significant.

Figure 1. Two sample trials of the intertemporal choice task. Subjects viewed two options, selected one via button press, and their choice was highlighted on the screen.
The early option always had a lower (undiscounted) value than the later option (i.e., $R < $R′). The two options were separated by a minimum time delay of 2 weeks. In some choice pairs, the early option was available “immediately” (i.e., at the end of the scanning session; d = 0). In other choice pairs, even the early option was available only after a delay (d > 0). The early option varied from “today” to “2 weeks” to “1 month,” whereas the later option varied from “2 weeks” to “1 month” to “6 weeks.” Each subject completed 82 trials.
Neuroimaging Data Acquisition and Analysis
Neuroimaging data were collected using a 1.5-T General Electric MRI scanner using a standard birdcage quadrature head coil. Twenty-four 4-mm thick slices (in-plane resolution 3.75 mm × 3.75 mm, no gap) extended axially from the mid-pons to the top of the skull. Functional scans of the whole brain were acquired at a repetition time of 2 s with a T2*-sensitive in-/out-spiral pulse sequence (TE = 40 ms, flip = 90°) designed to minimize signal dropout at the base of the brain (Glover and Law, 2001). High-resolution structural scans were acquired using a T1-weighted spoiled GRASS (gradient acquisition in the steady state) sequence (TR = 100 ms; TE = 7 ms, flip = 90°), facilitating localization and coregistration of functional data. Preprocessing and whole brain analyses were conducted using analysis of functional neural images (AFNI) software (Cox, 1996). For preprocessing, voxel time series were slice-time corrected within each volume, corrected for three-dimensional motion across volumes, slightly spatially smoothed (FWHM = 4 mm), converted to percentage signal change (relative to the mean activation over the entire experiment), and high-pass filtered. Visual inspection of motion correction estimates confirmed that no subject’s head moved more than 4 mm in any dimension from one volume acquisition to the next.
A dual-system model with one present-oriented component and another more delay-oriented component was used to create regressors of interest for the neuroimaging analyses (McClure et al., 2004, 2007). We used a simplified utility function where we approximate these two systems with average discount rates β and δ:

The “δ system” discounts exponentially with factor δ. The “β system” discounts exponentially with factor β to capture the extra weight given to immediate rewards. Lower values of β and δ correspond to steeper discounting. Generally, the more impatient and present-oriented β component of this function discounts reward at a much greater rate than does the more patient δ component. Thus, the δ-system can be interpreted as indexing more modest discounting (i.e., relatively reduced sensitivity to delay when compared to the β-system). This model has been previously associated with functionally distinct neural systems (McClure et al., 2004, 2007), a result we replicate in the present study. The relative weighting of the two valuation systems in determining choice is given by ω (0 ≤ ω ≤ 1). The discount function resulting from the combination of these two exponential systems has been referred to as quasi-hyperbolic (Laibson, 1997).
Based on the observed choices across all presented pairs of rewards (R) and delays (d), four parameters were estimated per subject (β, δ, ω, m) using a simulated annealing algorithm to maximize the likelihood of the observed choices (fits restricted such that 0 ≤ β ≤ δ ≤ 1). Choices were assumed to follow a softmax decision function with temperature parameter m (the slope of the decision function). Higher values of m correspond to a stronger bias for selection of the higher subjective value option, whereas lower values of m correspond to a weaker bias for the selection of the higher subjective value option. Low values of m may indicate more random responding. We did not observe age differences in m. Additionally, although both age groups showed some level of present bias (β), the age groups did not differ in β or any other model parameter. The two groups did not differ significantly in β, Z = 0.136, p = 0.89 (young mean = 0.51, old mean = 0.47), δ, Z = 0.109, p = 0.91 (young mean = 0.99, old mean = 0.99), ω, Z = −0.446, p = 0.66 (young mean = 0.94, old mean = 0.92), m, Z = 0.272, p = 0.79 (young mean = 2.07, old mean = 1.82), or the fit of the model, Z = −0.49, p = 0.62 (log-likelihood: young mean = −27.01, old mean = −26.88). The best fitting β and δ for each subject were used in the regression models described below. Additionally, we fit behavior using a generalized hyperbolic discount function of the form V(R,d) = R(1 + αd)−β/α (Loewenstein and Prelec, 1992). We find no significant differences in either α, Z = 0.326, p = 0.74, or β, Z = 0.218, p = 0.83.
Preprocessed time series data for each individual were analyzed with multiple regression in AFNI. The regression model contained two regressors of interest corresponding to the β-system and δ-system. For the β-system regressor, we modeled the sum of the β-weighted values for the two options available on that trial (i.e., Rβd + R′βd′). Similarly, for the δ-system regressor, we modeled the sum of the δ-weighted values for the two options available on that trial (i.e., Rδd + R′δd′). Additional covariates included residual motion (in six dimensions) and polynomial trends across the experiment. Regressors of interest were convolved with a gamma-variate function that modeled a prototypical hemodynamic response before inclusion in the regression model. Maps of t-statistics representing each of the regressors of interest were transformed into Z-scores, resampled at 2 mm3 and spatially normalized by warping to Talairach space. Statistical maps were then generated using one-sample t-tests to examine effects across all subjects and independent-samples t-tests to examine differences between groups (older adults > younger adults). Voxel-wise thresholds for statistical significance at the whole brain level were set at p < 0.005, uncorrected. All regression analyses were conducted with resampled 2 mm3 voxels with a minimum cluster size of 56 voxels for a p < 0.05 whole brain corrected threshold estimated using AFNI’s AlphaSim (Cox, 1996) using a mask generated from an average brain image across subjects in the study. Small volume correction was applied to the VS by using 16-mm diameter spherical masks bilaterally and at p < 0.005 a cluster size of 9 voxels was estimated using AlphaSim for a p < 0.05 corrected threshold. For follow-up inspection of regression coefficients and timecourse analyses, regions of interest were specified at the peak voxel of significant clusters that emerged in group analyses. These 8-mm diameter spheres were shifted within individuals to ensure that only data from gray matter were extracted. Timecourse analyses examined whether activation during decision making (i.e., signal averaged from time points 4 and 5 in each trial to account for HRF peak shift) differed from baseline in these volumes of interest in the presence of an immediate option (d = “today,” d′ = “2 weeks,” or “1 month”) or absence of an immediate option (d = “2 weeks,” d′ = “1 month,” or “6 weeks”). We did not include the d = “1 month” trials in these timecourse analyses, because this delay can only be combined with d′ = “6 weeks” resulting in far fewer trials for this condition.
In all fMRI analyses, care was taken to minimize potential confounds associated with age differences in subject characteristics, brain morphology, and hemodynamics (Samanez-Larkin and D’Esposito, 2008). Each individual’s brain was warped into Talairach space with reference to 11 hand-placed anatomical landmarks (superior edge of anterior commissure, posterior margin of anterior commissure, inferior edge of posterior commissure, two mid-sagittal points, most anterior point, most posterior point, most superior point, most inferior point, most left point, most right point). Structural and functional brain imaging data were inspected for abnormalities in each individual. None were excluded due to abnormalities. Four additional individuals (not included in the 25 subjects described above) were excluded due to a data acquisition error (68 year old female), excessive motion (75 year old male), not completing the task (19 year old female), or difficulty with data fitting given the selection of the sooner option on every trial (32 year old female).
Results
Behavioral Results
There were no behavioral differences in intertemporal preferences between the younger and older groups on the experimental task we used in the present experiment. The two groups did not differ in the proportion of smaller, sooner choices selected, t24 = 0.20, p = 0.84 (young mean = 0.46, old mean = 0.44). Nor were there age differences in the parameters derived from either of two discount functions fit to the data. For comparison of fMRI results, comparable behavioral responding is advantageous since it reduces the influence of numerous potential confounds and facilitates interpretation of differences in brain responses.
Neuroimaging Results
Across age groups, functional neuroimaging analyses identified brain regions that correlated with the β and δ components of the subjective value function described by Eq. 1. Across all subjects, δ-related neural activity was observed in the right dorsolateral prefrontal cortex, bilateral anterior insula, and a large cluster in the occipital cortex with peaks extending into bilateral PPC (Figure 2A; Table 1). In contrast, β-related neural activity was observed in brain regions associated with the mesolimbic dopamine system including the ventromedial prefrontal cortex and posterior cingulate (Figure 2B; Table 1). A subthreshold-sized cluster also emerged in the left nucleus accumbens (Z = 3.066; −9, 9, −8; 6 voxels) at p < 0.005 uncorrected (see Figure A1 in Appendix). Similar results to the β effects were observed using a hyperbolic model with a single discount factor (see Table A1 and Figure A2 in Appendix).
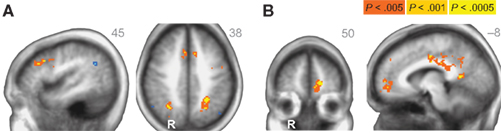
Figure 2. (A) δ-related neural activity in the bilateral anterior insula, dorsolateral prefrontal cortex, and bilateral parietal cortex across all subjects. (B) β-related neural activity in the medial prefrontal cortex, orbitofrontal cortex, and posterior cingulate across all subjects. R = right. R/L, A/P, or S/I value listed in upper right corner of each statistical map. Anatomical underlay is an average of all subjects’ spatially normalized structural scans.
When directly comparing older to younger adults, we observed an age-related shift in the brain areas that respond to immediate and delayed rewards. Comparing brain areas that show low discount rates (δ component) across age groups revealed significant differences in a lateral region of the VS (ventral putamen; VPut) with relatively greater loading on this regressor in older subjects (Figure 3A; Table 2). Further inspection of the coefficients extracted from VPut within age groups revealed a significant relationship with the δ regressor in older subjects, t12 = 2.705, p = 0.02, but not younger subjects, t11 = −1.55, p = 0.15 (Figure 3B). Additional analyses of time courses extracted from the VPut in the younger adults revealed significant activation (greater than baseline) when the earliest reward was available today, t11 = 2.392, p = 0.02, but not when the earliest reward was delayed 2 weeks, t11 = −0.124, p = 0.90. However, for older adults activation of the VPut was greater than baseline when the earliest reward was available either today, t12 = 2.187, p = 0.02, or delayed 2 weeks, t12 = 2.168, p = 0.03 (Figure 3C). This pattern was specific to VPut; age differences did not emerge in the nucleus accumbens (see Figure A3 in Appendix). Overall, the results suggest that the VPut shows modest sensitivity to delay in older subjects.
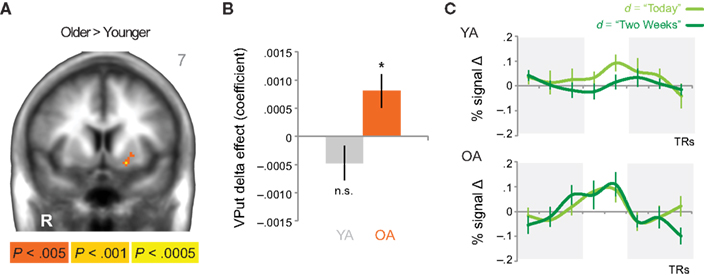
Figure 3. (A) The δ component (low discount rate) was more strongly associated with neural activity in the VPut (ventral putamen) in older compared to younger adults. R = right. A = 7. Anatomical underlay is an average of all subjects’ spatially normalized structural scans. (B) Significant δ-related neural activity in the VPut in older but not younger adults. *p < 0.05, n.s., not significant; error bars are SEM; YA, younger adults; OA, older adults. (C) For younger adults the VPut is active only when the earliest option is available immediately, but not when it is delayed. However, for older adults activation in the VPut increases for both immediate and delayed options. Error bars are SEM.
Age differences were also observed in the LPFC (Table 2). However, the age differences in the LPFC are suspect for two reasons that together lead us to believe it is not of functional importance. First, the regions are located near the edge of the brain where spatial variability across subjects is highest. Second, inspection of the coefficients indicated that the age differences arose from non-significant activation in older subjects and a decrease in activation in younger subjects. No age differences were observed with respect to the β component of the valuation model (Table 2) or when generating regressors based on subjective value using a hyperbolic model (Table A2 in Appendix).
To examine whether activation in the VPut was related to choice behavior, we computed differences in signal change between trials when the later option was chosen versus when the sooner option was chosen and correlated this signal difference with the overall proportion of later choices made (controlling for age). Individuals with larger differences in brain activity in the VPut on trials when they chose the later option relative to the sooner option also made more later choices overall, r = 0.58, p < 0.005, (see Figure 4). A similar relationship was observed in the nucleus accumbens (see Figure A4 in Appendix).
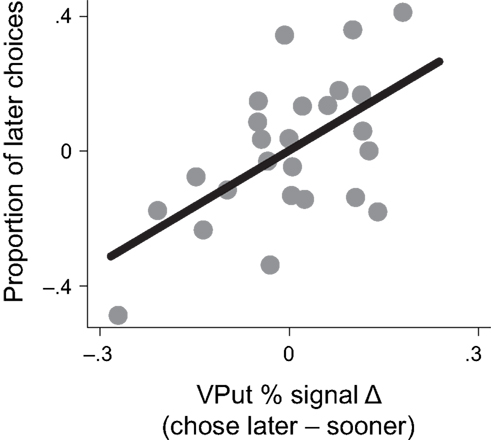
Figure 4. Activity in the ventral putamen (VPut) was associated with behavioral individual differences. Individuals with higher levels of signal change in the VPut on trials where they chose the later option relative to the sooner option also made more later choices overall.
Discussion
In the present study, we did not observe behavioral age differences in decision making with monetary intertemporal choices. The preference for sooner, smaller over larger, later rewards was not significantly stronger in younger compared to older adults. Given the small sample size, statistical power for this behavioral comparison is limited and we are hesitant to conclude anything from this behavioral result. Many prior studies find either stability (Green et al., 1996; Chao et al., 2009) or reductions in discounting across adult age (Green et al., 1994, 1999; Harrison et al., 2002; Reimers et al., 2009; Simon et al., 2010; Jimura et al., 2011; Löckenhoff et al., 2011), but others have reported increases in discounting from young adulthood to older age (Read and Read, 2004). Overall, the existing behavioral literature is conflicting. These discrepancies in existing studies may be partially related to interactions between individual difference variables (e.g., age) and state variables such as context or framing of the decisions (e.g., choices presented as delay lengths or the actual date of receipt; Peters and Büchel, 2011). These potential framing issues will not be fully resolved until they are directly examined in future studies. However, even while these issues remain unresolved, progress toward a more complete understanding of age-related change in intertemporal choice may be aided by examining age differences in the neural systems supporting these decisions.
Although we replicate findings that brain regions associated with the mesolimbic dopamine system respond preferentially to immediate rewards, we find a separate region of the putamen, within the VS, that responds to both immediate and delayed rewards in older but not younger adults. We also showed that relatively greater activation in the VS for delayed over sooner rewards was associated with an overall preference for delayed rewards. This effect may, at first, seem to contradict prior studies linking VS activity with a preference for immediate over delayed rewards in younger adults. However, the results are quite compatible with these prior findings. Specifically, individuals in the upper right quadrant of Figure 4 and Figure A4 in Appendix show greater VS sensitivity to delayed rewards and choose delayed rewards more often. Individuals in the lower left quadrant of Figure 4 and Figure A4 in Appendix show less VS sensitivity to delayed rewards (greater sensitivity to immediate rewards) and choose delayed rewards less often (and immediate rewards more often). Overall, this result clearly confirms a relationship between activation of this region and decision making on the task.
Previous studies, exclusively focused on younger adults, have shown that VS activity leads to more impulsive choice (McClure et al., 2004, 2007; Hariri et al., 2006). Prior work has emphasized that top-down input from the LPFC functions to overcome a VS-mediated present bias and enable more far-sighted choices (McClure et al., 2004, 2007; Figner et al., 2010). The results of the present study suggest that a different mechanism may apply to older adults. It is possible that the contributions of LPFC control are reduced with age as signals in the VS are tuned with experience. We hypothesize that experience may underlie the fact that subregions of the VS show modest sensitivity to time in older subjects.
The age differences were observed when examining regions that corresponded to δ-related representations of delayed reward value, but not for β-related representations of reward value. This pattern is consistent with the results of a recent study that included a much larger behavioral sample of young, middle-aged, and older adults and found age differences in δ-related discount rates but not β-related discount rates (Löckenhoff et al., 2011). That same study is the only experiment that has systematically attempted to explain the mediating variables between adult age differences and intertemporal choice (Löckenhoff et al., 2011). The study shows that emotional and motivational variables account for age differences in intertemporal choice. Basic neuropsychological measures that are presumed to rely on prefrontal resources do not explain the age differences. Consistent with prior research that older adults are better at forecasting future emotional states (Lachman et al., 2008; Nielsen et al., 2008; Scheibe et al., 2011), more positive emotional predictions of delayed rewards are associated with both older age and reduced discounting (Löckenhoff et al., 2011). Although we did not assess emotional forecasts of delayed rewards here, the results of the present study may provide a neural mechanism through which these previously observed age differences operate.
Since the dopamine system is believed to respond in anticipation of future rewards through associative learning (Montague et al., 1996; Schultz et al., 1997), our observed transfer of function may be due to greater experience with delayed rewards as people age. This increase in experience with delayed rewards through associative learning (Enomoto et al., 2011) over the course of decades may contribute to the improvement in forecasting the emotional impact of future events as discussed above. A number of studies have shown that anticipatory activation in the VS is modulated by the magnitude of an upcoming but not yet received reward and this activation is also correlated with anticipatory subjective emotional experience (Knutson and Greer, 2008).
Importantly, we are not suggesting that the appropriate valuation of rewards delayed by several weeks requires decades of experience to accurately estimate. Young adults in their twenties show neural activation in mesolimbic regions that correlates with delayed reward values (discounted subjective value; Kable and Glimcher, 2007; Peters and Büchel, 2009), and midbrain dopamine neurons in monkeys encode both immediate and delayed reward values through associative learning from experience over the course of weeks (Enomoto et al., 2011). Rather, we are suggesting that the additional experience with the realization of delayed rewards that older adults have accumulated over the lifetime may shape the sensitivity of this ventral striatal region. Although adults in their twenties will have some experience with shorter-term delayed rewards, the age differences may be even more pronounced for financial investments, for example, where there is a small but relatively reliable long-term rate of return (e.g., mutual funds). A 22-year old simply has not had the opportunity to appreciate the value of an 8% return over several decades.
Although age-related changes observed in the dopamine system and striatum have been associated with age-related declines in learning and decision making (Aizenstein et al., 2006; Mohr et al., 2009; Samanez-Larkin et al., 2010), it may be that gradual declines in the dopamine system with age do not disrupt the slow changes in associative learning from repeated experience with delayed rewards over decades of the life course. Furthermore, the dopaminergic changes that occur during healthy aging are not likely to be sufficiently dramatic to overwhelm the effects of accumulated experience. In contrast, the much more dramatic dopaminergic changes in Parkinson’s disease have been shown to influence discounting (Housden et al., 2010; Voon et al., 2010). In general, adult age differences are more apparent in decision making tasks that require rapid learning in a novel environment than when decisions can be made based solely on the information presented (Mata et al., 2011) as is the case with these intertemporal choice tasks.
Although experience may play a role in human age differences, other factors likely contribute. Demographic factors like education and income also influence discounting and can interact with age (Green et al., 1996; Reimers et al., 2009). In fact, changes in income over the life span (e.g., related to investment experience) may be partially correlated with the age-related changes that we are attributing to experience. It is important to note that subjects in the present study were recruited by a market research company and matched across age groups on socioeconomic status (education, current or previous profession, income). One limitation of this approach is that the resulting San Francisco Bay area/Silicon Valley sample is healthier, wealthier, and more highly educated than the general population which may limit generalizability. However, a great strength of this targeted sampling strategy is that the contributions of differences in demographic factors to between-group differences in either behavior or neural activity have been minimized here. Aside from demographic factors, there is also recent evidence for behavioral differences in discounting between young and aged rats where experience with delayed rewards over the lifetime is relatively controlled (Simon et al., 2010). Thus, there may be neurobiological changes that are not experience-related that contribute to age differences in intertemporal choice.
Far-sighted behavior is an important target for behavioral interventions to counter challenges like the anemic retirement savings in America and the inability to withstand small inconveniences (e.g., taking medicine daily, exercise) that are critical for long-term health. The majority of evidence for shaping intertemporal decision making in younger adults has focused on prefrontal mechanisms (Peters and Büchel, 2011). However, the same strategies may not apply to older adults. In other domains, it is known that younger adults are best affected by informational messages that presumably alter behavior via the LPFC, whereas older adults respond better to emotional messages that may target regions like the VS and amygdala (Carstensen, 2006; Mikels et al., 2010; Samanez-Larkin et al., 2011). For far-sighted behaviors, a similar difference may exist for younger and older adults. Whereas adults may benefit by targeting cognitive control, individuals may also benefit from nudges to emotional systems.
Conflict of Interest Statement
The authors declare that the research was conducted in the absence of any commercial or financial relationships that could be construed as a potential conflict of interest.
Acknowledgments
This research was supported by a pilot grant from the Stanford Roybal Center on Advancing Decision Making in Aging AG024957 to Samuel M. McClure and National Institute on Aging grant AG08816 to Laura L. Carstensen. Gregory R. Samanez-Larkin was supported by National Institute on Aging pre-doctoral fellowship AG032804 during data collection and post-doctoral fellowship AG039131 during manuscript preparation.
References
Agarwal, S., Driscoll, J. C., Gabaix, X., and Laibson, D. I. (2009). “The age of reason: financial decisions over the life-cycle with implications for regulation,” in Brookings Papers on Economic Activity, 51–117. Available at: http://muse.jhu.edu/journals/eca/summary/v2009/2009.2.agarwal.html
Aizenstein, H. J., Butters, M. A., Clark, K. A., Figurski, J. L., Stenger, V. A., Nebes, R. D., Reynolds, C. F., and Carter, C. S. (2006). Prefrontal and striatal activation in elderly subjects during concurrent implicit and explicit sequence learning. Neurobiol. Aging 27, 741–751.
Bäckman, L., Nyberg, L., Lindenberger, U., Li, S.-C., and Farde, L. (2006). The correlative triad among aging, dopamine, and cognition: current status and future prospects. Neurosci. Biobehav. Rev. 30, 791–807.
Camus, M., Halelamien, N., Plassmann, H., Shimojo, S., O’Doherty, J., Camerer, C., and Rangel, A. (2009). Repetitive transcranial magnetic stimulation over the right dorsolateral prefrontal cortex decreases valuations during food choices. Eur. J. Neurosci. 30, 1980–1988.
Carstensen, L. L. (2006). The influence of a sense of time on human development. Science 312, 1913–1915.
Chao, L.-W., Szrek, H., Pereira, N. S., and Pauly, M. V. (2009). Time preference and its relationship with age, health, and survival probability. Judgm. Decis. Mak. 4, 1–19.
Cox, K. M., Aizenstein, H. J., and Fiez, J. A. (2008). Striatal outcome processing in healthy aging. Cogn. Affect. Behav. Neurosci. 8, 304–317.
Cox, R. W. (1996). AFNI: software for analysis and visualization of functional magnetic resonance neuroimages. Comput. Biomed. Res. 29, 162–173.
Enomoto, K., Matsumoto, N., Nakai, S., Satoh, T., Sato, T. K., Ueda, Y., Inokawa, H., Haruno, M., and Kimura, M. (2011). Dopamine neurons learn to encode the long-term value of multiple future rewards. Proc. Natl. Acad. Sci. U.S.A. 108, 15462–15467.
Figner, B., Knoch, D., Johnson, E. J., Krosch, A. R., Lisanby, S. H., Fehr, E., and Weber, E. U. (2010). Lateral prefrontal cortex and self-control in intertemporal choice. Nat. Neurosci. 13, 538–539.
Glover, G. H., and Law, C. S. (2001). Spiral-in/out BOLD fMRI for increased SNR and reduced susceptibility artifacts. Magn. Reson. Med. 46, 515–522.
Green, L., Fry, A. F., and Myerson, J. (1994). Discounting of delayed rewards: a life-span comparison. Psychol. Sci. 5, 33–36.
Green, L., Myerson, J., Lichtman, D., Rosen, S., and Fry, A. (1996). Temporal discounting in choice between delayed rewards: the role of age and income. Psychol. Aging 11, 79–84.
Green, L., Myerson, J., and Ostaszewski, P. (1999). Discounting of delayed rewards across the life span: age differences in individual discounting functions. Behav. Processes 46, 89–96.
Hare, T. A., Camerer, C. F., and Rangel, A. (2009). Self-control in decision-making involves modulation of the vmPFC valuation system. Science 324, 646–648.
Hariri, A. R., Brown, S. M., Williamson, D. E., Flory, J. D., de Wit, H., and Manuck, S. B. (2006). Preference for immediate over delayed rewards is associated with magnitude of ventral striatal activity. J. Neurosci. 26, 13213–13217.
Harrison, G. W., Lau, M. I., and Williams, M. B. (2002). Estimating individual discount rates in Denmark. Am. Econ. Rev. 92, 1606–1617.
Henninger, D. E., Madden, D. J., and Huettel, S. A. (2010). Processing speed and memory mediate age-related differences in decision making. Psychol. Aging 25, 262–270.
Hosseini, S. M. H., Rostami, M., Yomogida, Y., Takahashi, M., Tsukiura, T., and Kawashima, R. (2010). Aging and decision making under uncertainty: behavioral and neural evidence for the preservation of decision making in the absence of learning in old age. Neuroimage 52, 1514–1520.
Housden, C. R., O’Sullivan, S. S., Joyce, E. M., Lees, A. J., and Roiser, J. P. (2010). Intact reward learning but elevated delay discounting in Parkinson’s disease patients with impulsive-compulsive spectrum behaviors. Neuropsychopharmacology 35, 2155–2164.
Jimura, K., Myerson, J., Hilgard, J., Keighley, J., Braver, T. S., and Green, L. (2011). Domain independence and stability in young and older adults’ discounting of delayed rewards. Behav. Processes 87, 253–259.
Kable, J. W., and Glimcher, P. W. (2007). The neural correlates of subjective value during intertemporal choice. Nat. Neurosci. 10, 1625–1633.
Knutson, B., and Greer, S. M. (2008). Anticipatory affect: neural correlates and consequences for choice. Philos. Trans. R Soc. Lond. B Biol. Sci. 363, 3771–3786.
Lachman, M. E., Röcke, C., Rosnick, C., and Ryff, C. D. (2008). Realism and illusion in Americans’ temporal views of their life satisfaction: age differences in reconstructing the past and anticipating the future. Psychol. Sci. 19, 889–897.
Löckenhoff, C. E. (2011). Age, time, and decision making: from processing speed to global time horizons. Ann. N. Y. Acad. Sci. 1235, 44–56.
Löckenhoff, C. E., O’Donoghue, T., and Dunning, D. (2011). Age differences in temporal discounting: the role of dispositional affect and anticipated emotions. Psychol. Aging 26, 274–284.
Loewenstein, G., and Prelec, D. (1992). Anomalies in intertemporal choice: evidence and an interpretation. Q. J. Econ. 107, 573–597.
Logue, A. W., Rodriguez, M. L., Peña-Correal, T. E., and Mauro, B. C. (1984). Choice in a self-control paradigm: quantification of experience-based differences. J. Exp. Anal. Behav. 41, 53–67.
Luo, S., Ainslie, G., Giragosian, L., and Monterosso, J. R. (2009). Behavioral and neural evidence of incentive bias for immediate rewards relative to preference-matched delayed rewards. J. Neurosci. 29, 14820–14827.
Mata, R., Helversen von, B., and Rieskamp, J. (2010). Learning to choose: cognitive aging and strategy selection learning in decision making. Psychol. Aging 25, 299–309.
Mata, R., Josef, A. K., Samanez-Larkin, G. R., and Hertwig, R. (2011). Age differences in risky choice: a meta-analysis. Ann. N. Y. Acad. Sci. 1235, 18–29.
McClure, S. M., Ericson, K. M., Laibson, D. I., Loewenstein, G., and Cohen, J. D. (2007). Time discounting for primary rewards. J. Neurosci. 27, 5796–5804.
McClure, S. M., Laibson, D. I., Loewenstein, G., and Cohen, J. D. (2004). Separate neural systems value immediate and delayed monetary rewards. Science 306, 503–507.
Mell, T., Wartenburger, I., Marschner, A., Villringer, A., Reischies, F. M., and Heekeren, H. R. (2009). Altered function of ventral striatum during reward-based decision making in old age. Front. Hum. Neurosci. 3:34.
Mikels, J. A., Löckenhoff, C. E., Maglio, S. J., Goldstein, M. K., Garber, A., and Carstensen, L. L. (2010). Following your heart or your head: focusing on emotions versus information differentially influences the decisions of younger and older adults. J. Exp. Psychol. Appl. 16, 87–95.
Mohr, P. N. C., Li, S.-C., and Heekeren, H. R. (2009). Neuroeconomics and aging: neuromodulation of economic decision making in old age. Neurosci. Biobehav. Rev. 34, 678–688.
Montague, P. R., Dayan, P., and Sejnowski, T. J. (1996). A framework for mesencephalic dopamine systems based on predictive Hebbian learning. J. Neurosci. 16, 1936–1947.
Nielsen, L., Knutson, B., and Carstensen, L. L. (2008). Affect dynamics, affective forecasting, and aging. Emotion 8, 318–330.
Peters, J., and Büchel, C. (2009). Overlapping and distinct neural systems code for subjective value during intertemporal and risky decision making. J. Neurosci. 29, 15727–15734.
Peters, J., and Büchel, C. (2010). Episodic future thinking reduces reward delay discounting through an enhancement of prefrontal-mediotemporal interactions. Neuron 66, 138–148.
Peters, J., and Büchel, C. (2011). The neural mechanisms of inter-temporal decision-making: understanding variability. Trends Cogn. Sci. (Regul. Ed.) 15, 227–239.
Read, D., and Read, N. L. (2004). Time discounting over the lifespan. Organ. Behav. Hum. Decis. Process. 47, 22–32.
Reimers, S., Maylor, E. A., Stewart, N., and Chater, N. (2009). Associations between a one-shot delay discounting measure and age, income, education and real-world impulsive behavior. Pers. Individ. Dif. 47, 973–978.
Rogers, A. R. (1994). Evolution of time preference by natural selection. Am. Econ. Rev. 84, 460–481.
Samanez-Larkin, G. R., and D’Esposito, M. (2008). Group comparisons: imaging the aging brain. Soc. Cogn. Affect. Neurosci. 3, 290–297.
Samanez-Larkin, G. R., Gibbs, S. E. B., Khanna, K., Nielsen, L., Carstensen, L. L., and Knutson, B. (2007). Anticipation of monetary gain but not loss in healthy older adults. Nat. Neurosci. 10, 787–791.
Samanez-Larkin, G. R., Kuhnen, C. M., Yoo, D. J., and Knutson, B. (2010). Variability in nucleus accumbens activity mediates age-related suboptimal financial risk taking. J. Neurosci. 30, 1426–1434.
Samanez-Larkin, G. R., Wagner, A. D., and Knutson, B. (2011). Expected value information improves financial risk taking across the adult life span. Soc. Cogn. Affect. Neurosci. 6, 207–217.
Scheibe, S., Mata, R., and Carstensen, L. L. (2011). Age differences in affective forecasting and experienced emotion surrounding the 2008 US presidential election. Cogn. Emot. 25, 1029–1044.
Schott, B. H., Niehaus, L., Wittmann, B. C., Schütze, H., Seidenbecher, C. I., Heinze, H.-J., and Düzel, E. (2007). Ageing and early-stage Parkinson’s disease affect separable neural mechanisms of mesolimbic reward processing. Brain 130, 2412–2424.
Schultz, W., Dayan, P., and Montague, P. R. (1997). A neural substrate of prediction and reward. Science 275, 1593–1599.
Shamosh, N. A., DeYoung, C. G., Green, A. E., Reis, D. L., Johnson, M. R., Conway, A. R. A., Engle, R. W., Braver, T. S., and Gray, J. R. (2008). Individual differences in delay discounting: relation to intelligence, working memory, and anterior prefrontal cortex. Psychol. Sci. 19, 904–911.
Simon, N. W., Lasarge, C. L., Montgomery, K. S., Williams, M. T., Mendez, I. A., Setlow, B., and Bizon, J. L. (2010). Good things come to those who wait: attenuated discounting of delayed rewards in aged Fischer 344 rats. Neurobiol. Aging 31, 853–862.
Voon, V., Reynolds, B., Brezing, C., Gallea, C., Skaljic, M., Ekanayake, V., Fernandez, H., Potenza, M. N., Dolan, R. J., and Hallett, M. (2010). Impulsive choice and response in dopamine agonist-related impulse control behaviors. Psychopharmacology (Berl.) 207, 645–659.
Whelan, R., and McHugh, L. A. (2009). Temporal discounting of hypothetical monetary rewards by adolescents, adults, and older adults. Psychol. Rec. 59, 247–258.
Appendix
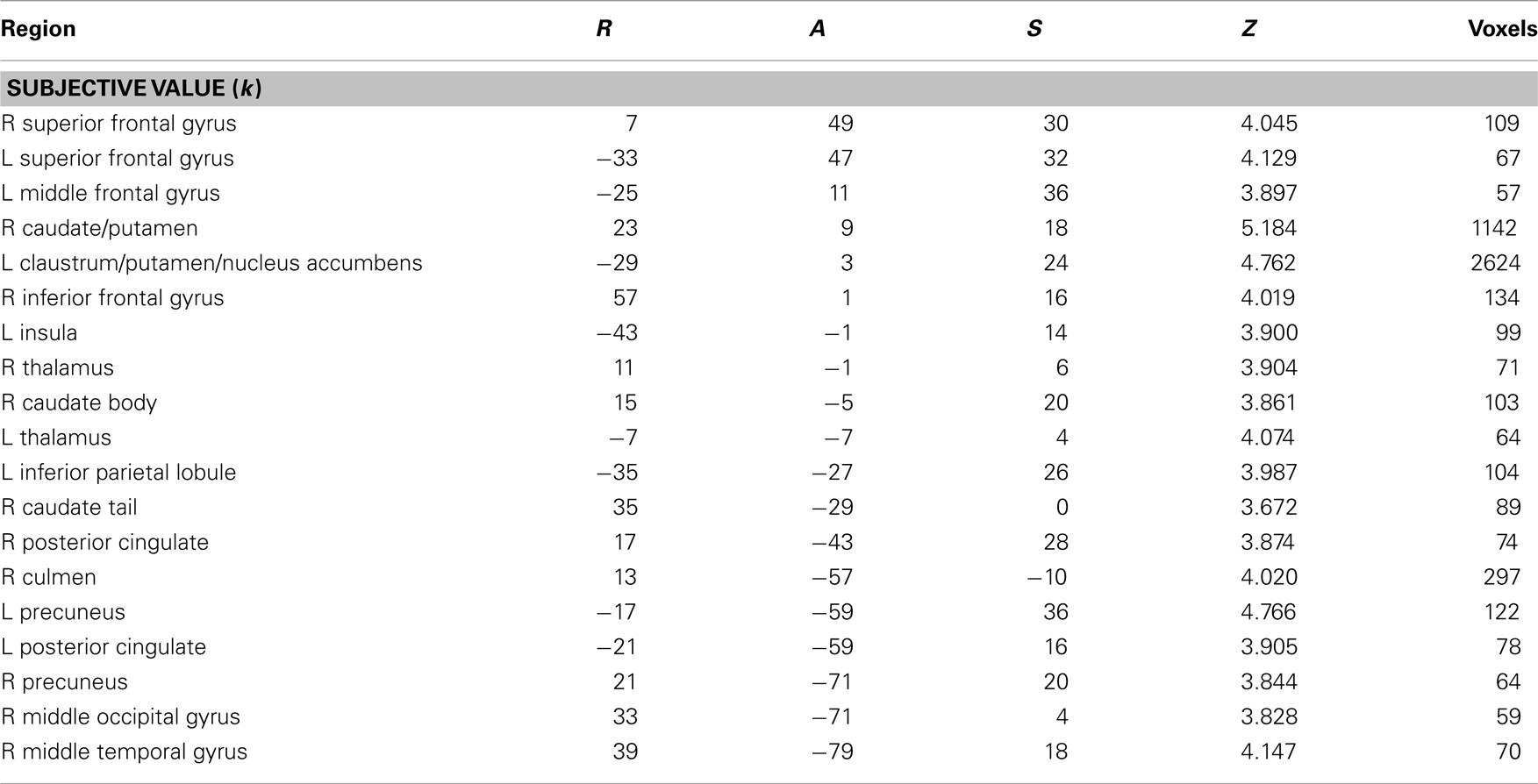
Table A1. Brain regions representing subjective value using a hyperbolic model of discounting (with discounting parameter, k) across all subjects.

Table A2. No age differences emerged in regions representing subjective value using a hyperbolic model of discounting (older > younger).
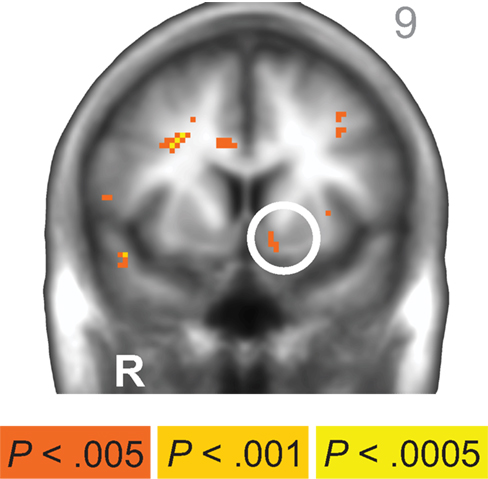
Figure A1. Across subjects, a small cluster of β-system-related neural activity emerged in the left nucleus accumbens (−9, 9, −8; peak Z = 3.066, 6 voxels) at p < 0.005, uncorrected. R = right. A/P value listed in upper right corner. Anatomical underlay is an average of all subjects’ spatially normalized structural scans.
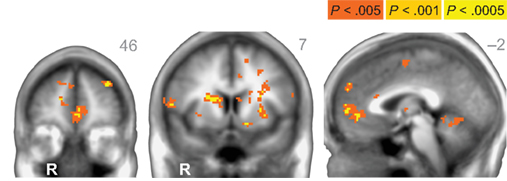
Figure A2. Brain regions representing the hyperbolic discounted value of the sum of the two options. Best fitting values of k for each subject were used to create a regressor of interest for these neuroimaging analyses. Across subjects, activation in a network of mesolimbic regions (similar to what was observed for the β regressor in the β–δ model) including the medial prefrontal cortex and striatum was correlated with subjective value (see Table A1). R = right. R/L or A/P, value listed in upper right corner of each statistical map. Anatomical underlay is an average of all subjects’ spatially normalized structural scans.
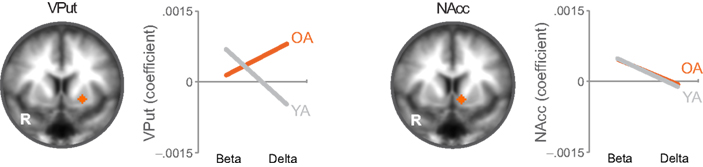
Figure A3. Regression coefficients for β and δ effects were extracted from regions of interest in the ventral putamen and nucleus accumbens. As reported in the main text, older adults (OA) and younger adults (YA) showed significantly different δ but not β effects in the ventral putamen, but the two groups did not differ for either δ or β in the nucleus accumbens. Regions of interest were adjusted within subjects to only extract coefficients from gray matter. Anatomical underlay is an average of all subjects’ spatially normalized structural scans.
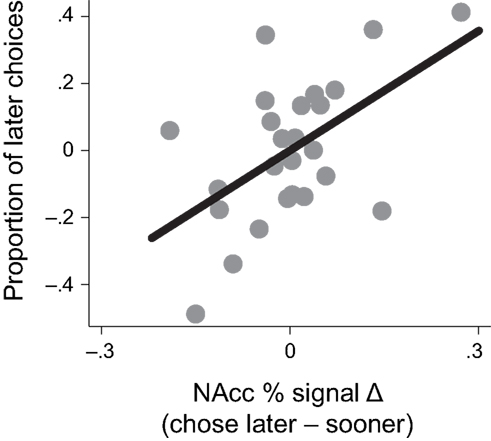
Figure A4. The same relationship between ventral striatal activity and behavior that was observed in the ventral putamen also emerged in the nucleus accumbens (NAcc). Individuals with higher levels of signal change in the NAcc on trials where they chose the later option relative to the sooner option also made more later choices overall, r = 0.54, p < 0.01 (controlling for age).
Keywords: aging, reward, decision making, discounting, intertemporal choice, ventral striatum, experience
Citation: Samanez-Larkin GR, Mata R Radu PT, Ballard IC, Carstensen LL and McClure SM (2011) Age Differences in Striatal Delay Sensitivity during Intertemporal Choice in Healthy Adults. Front. Neurosci. 5:126. doi: 10.3389/fnins.2011.00126
Received: 06 August 2011;
Accepted: 18 October 2011;
Published online: 16 November 2011.
Edited by:
Shu-Chen Li, Max Planck Institute for Human Development, GermanyReviewed by:
Natalie L. Denburg, The University of Iowa, USAMara Mather, University of Southern California, USA
Michael S. Cohen, University of California Los Angeles, USA
Copyright: © 2011 Samanez-Larkin, Mata, Radu, Ballard, Carstensen and McClure. This is an open-access article subject to a non-exclusive license between the authors and Frontiers Media SA, which permits use, distribution and reproduction in other forums, provided the original authors and source are credited and other Frontiers conditions are complied with.
*Correspondence: Gregory R. Samanez-Larkin, Psychological Sciences, Vanderbilt University, 111 21st Avenue South, Nashville, TN 37212, USA. e-mail:Zy5zYW1hbmV6bGFya2luQHZhbmRlcmJpbHQuZWR1