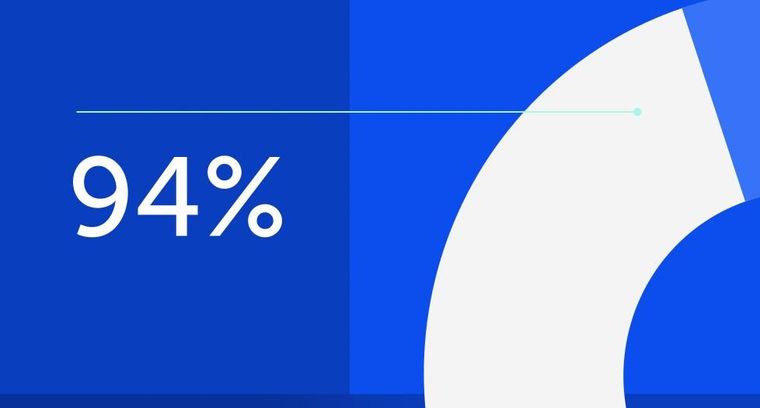
94% of researchers rate our articles as excellent or good
Learn more about the work of our research integrity team to safeguard the quality of each article we publish.
Find out more
PERSPECTIVE article
Front. Neurosci., 11 October 2011
Sec. Decision Neuroscience
volume 5 - 2011 | https://doi.org/10.3389/fnins.2011.00121
This article is part of the Research TopicBehavioral and neuroscientific analysis of economic decision making in animalsView all 14 articles
Probability and risk are important factors for value-based decision making and optimal foraging. In order to survive in an unpredictable world, organisms must be able to assess the probability and risk attached to future events and use this information to generate adaptive behavior. Recent studies in non-human primates and rats have shown that both probability and risk are processed in a distributed fashion throughout the brain at the level of single neurons. Reward probability has mainly been shown to be coded by phasic increases and decreases in firing rates in neurons in the basal ganglia, midbrain, parietal, and frontal cortex. Reward variance is represented in orbitofrontal and posterior cingulate cortex and through a sustained response of dopaminergic midbrain neurons.
Animals in the wild must interact with the environment and harvest primary rewards such as food and reproductive opportunities to maximize the likelihood that their genetic information survives in future generations. Outside the controlled conditions of the laboratory the time and place that these positive events occur can often not be predicted with total accuracy. In order to survive in such an unpredictable and risky world, organisms must be able to assess not only the probabilities attached to future rewards but also the precision of these estimates and use this information to behave appropriately. Behavioral ecologists have studied the effects of uncertainty on foraging in animals for many decades, but only in recent years have we begun to understand how it is coded in the brain and how this information relates to choice.
Before describing their neuronal correlates, we consider briefly the definition of unpredictability and risk and the methodological issues arising from studying them in humans and animals. In the lay concept, risk increases with the perceived chance that a bad outcome (i.e., an event that yields negative subjective value) will occur. In the context of animals living in the wild, this typically translates as the probability of death, either through predation or starvation. However, because these long term hazards carry such extreme negative values it is difficult to examine them quantitatively in the laboratory on a trial-by-trial basis (Real and Caraco, 1986). As a result, the majority of studies at both the behavioral and neural levels have defined uncertainty according to economic and mathematic principles, allowing researchers to define uncertainty at discrete points in time and to study the effects of these parameters on individual decisions. In contrast to the traditional and lay usage of uncertainty, these principles have provided a more precise and quantitative approach.
Economists and decision theorists interested in human behavior typically divide uncertainty into two distinct concepts; risk, where the probabilities of potential outcomes are known and ambiguity, where the probabilities are not precisely known (Knight, 1921; Ellsberg, 1961; “uncertainty” and “ambiguity” are sometimes also used synonymously). However, other forms and conceptualizations of unpredictability are conceivable and the question whether humans outside the lab sharply distinguish between risk and ambiguity could be investigated further. In human terms, a risky decision might be to gamble on the outcome of a fair roulette wheel, whereas an ambiguous decision might be to gamble on the outcome of a football game. Formally, risk can be defined according to the statistical properties of outcome distributions, such as dispersion (i.e., variance or the related SD or coefficient of variation), skewness, or kurtosis (Figure 1; Burke and Tobler, 2011). These objective statistical properties are not precisely known for an ambiguous option, thereby again providing, at least conceptually, a sharp distinction between risk and ambiguity.
Figure 1. Different forms of reward-related uncertainty. Ambiguity arises when the probabilities associated with a reward distribution are not fully known. When probabilities are known, then the situation is risky. The definition of risk used in the described studies is distinct from that used in everyday language (for example, risky prospect is one where the probability of a loss is non-zero). Instead, risk is defined by a number of parameters that describe the properties of the underlying reward distribution. Careful task design can allow researchers to disentangle neuronal responses to different forms of uncertainty through the independent manipulation of these parameters. For example, to show that a neuron responds to variance, it is necessary to hold probability constant and also check that this response does not vary with magnitude (O’Neill and Schultz, 2010). Risk and ambiguity can also be separated through stimulus design (Hayden et al., 2011). Note that entropy, SD, variance, and coefficient of variation correlate with each other (but not monotonically with probability). Their separation is therefore more difficult to achieve through task design and might be particularly sensitive to noise in the data.
Real and Caraco (1986) identify two problems that all organisms must overcome in a stochastic environment in order to generate adaptive behavior. Firstly, an organism must learn and keep in mind the outcome probability distributions attached to certain actions and then select a strategy for exploiting these distributions to maximize fitness. The goal of neuroscientific research on decision making under uncertainty has been to discover how the brain solves these two problems by coding the parameters and translating this information into actions. The vast majority of such research has been performed using human subjects, primarily in conjunction with functional magnetic resonance imaging (fMRI). This has increased our understanding of the anatomical substrates of reward uncertainty processing to a large degree and has also revealed interesting parallels between sensorimotor and economic decision processes (Braun et al., 2011; Wu et al., 2011). Yet, the low spatial and temporal resolution of fMRI data does not allow researchers to see the fast signaling of reward information by individual neurons. fMRI is also not suited to observing the large degrees of heterogeneity in both response properties and task-related activity of single neurons within small regions of interest. In order to elucidate the temporal propagation of reward uncertainty signals in subcortical and cortical regions, single cell recordings must be made in animals, typically in behaving rats and monkeys.
However, using animals in research on the neural mechanisms of decision making under risk poses a different set of challenges from those in human studies. One such issue is whether the economic definitions of risk, envisaged to provide normative or descriptive explanations of human behavior, apply to animal behavior at all. Indeed, the ability of humans to process uncertainty and exploit the information to succeed in the environment may represent a recent evolutionary addition to our cognitive skills that may not be possessed by animals at all. For example, for foraging animals in the wild, the sharp distinction between risk and ambiguity may not be so clear. Animals have to infer the properties of outcome distributions through repeated sampling and learning, thereby gradually turning ambiguity into risk (a similar process may also occur in more controlled lab conditions; Rosati and Hare, 2011). Moreover, mathematical abilities and the use of numerical representations are more limited in animals compared to humans. For these reasons, the cognitive tasks used to probe behavioral and neural responses to uncertainty in animals differ from those used in human experiments and are typically based on paradigms previously used in animal learning theory. In the present paper we separately review the forms of uncertainty that have been tested experimentally in animals and describe the neurophysiological data relating to each type.
The experiments discussed in this review all use single or multiple microelectrodes to record the extracellular potential changes from cell bodies in the immediate vicinity of the electrode tip. In a similar manner to the normative delineations between different types of uncertainty, the descriptive neurophysiological results can be crudely separated into two groups. The majority of animal experiments on reward uncertainty signals have manipulated reward probability in an effort to elucidate the neural mechanisms of learning or value processing. By contrast, only a small number of studies have been conducted with a specific emphasis on economic risk or reward variance and these have focused primarily on cortical areas.
A simple way to manipulate reward uncertainty is to change the probability with which reward occurs following a cue or an action. Behavioralists have long known that animal decisions are based on reward probability in addition to reward magnitude (Herrnstein and Vaughn, 1980), with the assumed goal of maximizing the reward rate (Stephens and Krebs, 1986). Although a number of studies had previously investigated neural responses to reward expectation (Watanabe, 1996; Schultz et al., 1997), the first experiment to record probability-related activity of single neurons from an economic point of view was probably conducted by Platt and Glimcher (1999). Motivated by previous research implicating the lateral intraparietal (LIP) area as an interface between sensory- and action-related neural information in the brain (Goldberg et al., 1990; Snyder et al., 1997), they hypothesized economically relevant aspects of the decision environments might be represented there for translation into action. Indeed, LIP neurons were sensitive to expected reward magnitudes, but also modulated their firing rates in response to the probability that a specific rewarded action would be instructed (Platt and Glimcher, 1999).
This work laid the foundations for Sugrue et al. (2004) to record from LIP neurons during a harvesting task in which the reward probability of an unchosen option increased with the number of times it had not been chosen. In this task the optimal behavior is to distribute choices for each option according to the relative probabilities that each option would be rewarded. The monkeys were able to perform this task exceptionally well, with similar behavior to computer simulations using an optimal strategy. The activity of LIP neurons correlated with the relative values of targets in the response field of the cells, and this value was related to the probability that a saccade to each target would result in a reward. These recordings robustly support the idea that the brain computes reward probability, although it remains unclear if LIP neurons code probabilities in a pure fashion, separately from other reward-related, sensory, or behavioral information. Other parts of parietal cortex, such as the parietal reach region (PRR) code reward probability between the sensory and motor phases of a memory-guided reaching task. More specifically, the activity of PRR neurons correlated with differential reward probability information during a memory period (1.2–1.8 s) after a cue, the size of which predicted reward with high (p = 0.8) or low (p = 0.4) probability (Musallam et al., 2004). Due to the suspected role of parietal cortex in integrating sensory and action information it is possible that these signals represent late and multiplexed information relevant to the decision process, with afferent or further upstream cells coding more basic reward information, such as probability.
Many neurons in the orbitofrontal cortex (OFC) appear to code reward probability independent of other task-relevant information such as future action, sensory information, or other reward-related parameters. The OFC is innervated by dopaminergic neurons originating in the ventral tegmental area via the mesocortical pathway, and has strong reciprocal connections with other subcortical reward-related regions such as the amygdala and striatum (Barbas and De Olmos, 1990; Cavada et al., 2000). van Duuren et al. (2009) investigated rat OFC responses by pairing different odors with 0, 50, 75, and 100% chance of receiving a rewarding outcome (a food pellet). During the course of one trial, rats were trained to sample an odor for 1.5 s, then proceed to a reward delivery port where they waited for 1.5 s until the outcome was delivered. A number of neurons coded the probability of the reward during the waiting phase (before food was delivered) with increasing or decreasing firing rates. A small number of neurons were found to respond to reward probability in this manner during the movement from odor sampling to reward delivery ports and also after the reward was delivered.
The result that small numbers of OFC neurons code reward probability in a pure manner is also supported by the work of Kennerley et al. (2009), who recorded simultaneously from OFC, anterior cingulate cortex (ACC), and lateral prefrontal cortex (LPFC) of monkeys. In their task, monkeys were trained to choose between abstract stimuli that predicted rewards with different magnitudes, probabilities, or cost (number of lever presses required to obtain the reward). The majority of cells in these areas coded two or more reward parameters, but a number of neurons in all three areas coded reward probability exclusively with increasing or decreasing firing rates. In addition, there were proportionally more neurons in the OFC that were tuned to a single reward parameter (such as probability).
By contrast, ACC neurons were more likely to reflect more than one decision parameter, potentially due to this area’s role in passing value information to motor areas and assigning values to upcoming actions. This result is supported by previous work by Amiez et al. (2006), which showed dorsal ACC neurons integrated both reward probability and magnitude to code the expected value of reward-predicting stimuli. Interestingly, Kennerley et al. (2009) found that the latencies of separate neuronal reward probability signals in the ACC were longer than those of multiplexed value signals, suggesting the ACC receives its reward probability information from multiple regions.
Electrophysiological studies of dopaminergic neurons in the substantia nigra (pars compacta) and ventral tegmental area have provided strong evidence that the brain codes reward probability. Fiorillo et al. (2003) used a Pavlovian conditioning paradigm with abstract visual cues, with each cue predicting a reward (0.15 ml of juice after 2 s) with a different probability (p = 0.0, p = 0.25, p = 0.5, p = 0.75, and p = 1.0). The monkeys showed increased anticipatory licking during cues predicting rewards with higher probabilities. Based on previous work on the phasic response of dopaminergic neurons to reward-predicting stimuli (Schultz, 1998) the researchers predicted that the phasic response to the cue should increase with increasing probability, and the response to reward should decrease with probability. This hypothesis was supported by the data (Figure 2A), with the phasic response fulfilling the necessary requirements of a reward prediction error reflecting probability as predicted by animal learning theory (Rescorla and Wagner, 1972).
Figure 2. Neuronal responses to reward probability, as demonstrated in four separate experiments. The descending rows represent trials with decreasing reward probability. Each column contains data from a separate experiment. (A) Population responses of dopaminergic neurons of the substantia nigra pars compacta and ventral tegmental area during a Pavlovian conditioning task, as described in Fiorillo et al. (2003). As an abstract visual stimulus predicts reward with decreasing probability, the dopaminergic neurons’ phasic response to the stimulus decreases. In addition a sustained response that increases until the time of reward encodes reward risk. (B) An example of the responses of a single cell in the lateral habenula during a similar task as described in [(A) from Matsumoto and Hikosaka, 2009]. Lateral habenula neurons typically show increased firing rates during the presentation of cues that predict reward with decreasing probability. The task did not include trials with 0.75 and 0.25 reward probabilities. (C) Population responses of tonically active neurons in the putamen, as recorded by Apicella et al. (2009). Stimulus-related reward probability information is encoded in the pause and initial peak of a fraction of tonically active neurons. In addition reward probability exerts strong modulation of suppression and subsequent rebound activity at the time of the outcome. (D) Oyama et al. (2010) recorded from the dorsal striatum of the rat, pairing auditory stimuli with reward in a similar paradigm to Fiorillo et al. (2003). Shown here is a single cell demonstrating analogous reward probability coding to dopamine neurons of the VTA and SN, with the absence of a sustained uncertainty response. Note that for p = 0.00, no stimulus was presented to the animal, but a free reward was delivered. All figures reprinted with permission.
The short latency of the dopaminergic neurons’ response to reward-predicting stimuli (about 100 ms after stimulus onset) suggests that these cells carry probabilistic reward information at an early stage of any decision process. It has recently been proposed that a potential input to these cells is the globus pallidus (Hong and Hikosaka, 2008), with neurons of the interior segment of the globus pallidus (GPi) responding to reward expectancy at a similar latency to that of dopamine neurons. Arkadir et al. (2004) partly addressed this question by using the same range of reward probabilities as Fiorillo et al. (2003) and simultaneously recording from the external segment of the globus pallidus (GPe) in an instrumental conditioning task. Very few neurons of the GPe were found to respond exclusively to reward probability, with the majority responding to a combination of response direction and reward probability. The longer latency of these responses suggested that they may not be the source of reward probability signals observed at stimulus onset in dopamine neurons. A follow-up study using a probabilistic classical conditioning task with recordings from GPe, GPi, and substantia nigra pars reticulata (SNr) further characterized responses in these regions to reward-predicting cues (Joshua et al., 2009). This study confirmed that GPi neurons encoded reward probability with latencies of around 250 ms after cue onset, too slow to be the source of the dopaminergic signals demonstrated by Fiorillo et al. (2003). By contrast, SNr cells responded to increasing reward probability with increasing and decreasing firing rates in roughly equal proportions, with latencies in the range of 125 ms, more similar to the latencies of dopamine neurons.
Another potential source for the dopaminergic reward probability signal is the lateral habenula (primarily glutamatergic), for example via projection through the rostromedial tegmental nucleus (primarily GABAergic; Jhou et al., 2009; Hong et al., 2011). Neurons in this region code reward probability in an inverse manner to dopaminergic neurons, showing increased suppression of firing rates to stimuli predicting reward with increasing probability (Figure 2B; Matsumoto and Hikosaka, 2009). These neurons also increase their firing rates to stimuli that predict aversive events, suppressing dopaminergic activity in the substantia nigra pars compacta (Bromberg-Martin et al., 2010). The latency of response suppressions reflecting reward probability information in lateral habenula neurons is roughly comparable to that of excitatory responses in SNc and VTA cells. The antagonistic manner of reward and punishment probability coding in the dopaminergic and lateral habenula neurons suggests that downstream structures may contain subpopulations of neurons that code probability for both rewarding and punishing outcomes. The amygdala has been shown to be one such structure, containing cells responsive to cues predicting rewards and punishments and emitting responses that may be modulated by the probability of the outcome (Belova et al., 2007; Bermudez and Schultz, 2010a) as well as being sensitive to reward magnitudes (Bermudez and Schultz, 2010b).
Two of the most-discussed regions that are innervated by dopaminergic neurons are the striatum and the prefrontal cortex (Haber, 2003). However, these structures at least indirectly also project to dopaminergic neurons. Indeed, if the source of reward probability signaling is the GPi as proposed by Hong and Hikosaka (2008), one would also expect to find such signals in the putamen and caudate and recent research has shown this to be the case. In the striatum, cholinergic tonically active neurons (TANs) in the primate putamen have primarily been the subject of investigation with regard to reward probability. These cells typically show suppression of their firing rates when dopaminergic cells show increased activity (Morris et al., 2004), with the level of suppression coding reward probability in classical conditioning tasks (Figure 2C; Apicella et al., 2009). In these cells, reward probability was found to be processed primarily at the time of reward delivery, with increasing suppression of firing rates when reward was delivered with low probabilities, an inverse of the typical dopamine response (and more like lateral habenula neurons’ responses). However, when no reward was delivered, two populations of TANs showed divergent firing patterns. Some cells increased their suppression when reward was predicted with high probability (like dopaminergic midbrain cells) while others showed increasing activity to reward omission with increasing reward probability (like lateral habenula cells). The responses of these neurons are quite variable and appear to only code reward probability in Pavlovian rather than instrumental tasks (Apicella et al., 2011). One potential explanation for the fast latency of TAN suppression is that TANs and dopaminergic neurons are recruited in parallel during the processing of relevant reward information, allowing dopaminergic input to modulate corticostriatal synapses during learning.
By contrast, single-unit recordings from the dorsal striatum in rats have shown responses to reward probability that are more analogous to dopamine than that of TANs. Oyama et al. (2010) recorded from the caudate nucleus while rats performed a similar task to the one used in Fiorillo et al. (2003), with rewards being paired with auditory stimuli at different probabilities. Upon stimulus onset, many neurons were found to code reward probability with increasing firing rates (Figure 2D). At reward delivery, the opposite pattern of activation was found. Interestingly, these neuronal responses to probability were invariant to the satiety of the animal, suggesting that caudate neurons code probability independently of the current state and do not reflect the subjective value of the stimulus (a finding that is reminiscent of veridical probability coding in the human striatum; Tobler et al., 2008).
Neurons that encode the probability of upcoming rewards are present in the basal ganglia, and frontal and parietal cortex. Of these, it seems that the responses of subcortical structures code reward probability in a relatively straightforward manner at the time of a reward-predicting cue. The phasic response of dopaminergic neurons in particular to reward probability perfectly reflects the notion of a reward prediction error signal, implying that probability representations are built up by successive sampling of the reward environment. Fiorillo et al. (2003) also demonstrated that a more sustained response of dopamine neurons in the same probabilistic task reflected the degree of risk on each trial. In the task of Fiorillo et al. (2003) when the animal is presented with a stimulus predicting a reward with p = 0 or p = 1, either no reward (for p = 0) or a reward (for p = 1) will be received with certainty and risk (e.g., variance) is zero on these trials. Risk is maximal for stimuli predicting rewards with p = 0.5, as the animal is equally likely to receive a reward or nothing at all. Risk therefore follows an inverted U-shape as a function of increasing reward probability. Fiorillo et al. (2003) found that approximately 30% of reward probability encoding dopamine neurons showed a sustained response that scaled with the risk on a given trial (Figure 2A). The sustained responses followed the initial phasic reward probability response and increased gradually until the time of reward delivery. It also increased when probability was kept constant at p = 0.5 but the dispersion was increased by manipulating the magnitudes of the two possible outcomes. How this risk signal is interpreted by postsynaptic neurons remains to be explored. Schultz (2010) suggests that the phasic, relatively high frequency spiking of dopaminergic neurons that codes reward probability (and prediction error) may be communicated to postsynaptic neurons through the preferential activation of D1 receptors. By contrast, the sustained, low frequency uncertainty response may preferentially engage postsynaptic D2 receptors due to their high affinity.
Dopamine is unlikely to be the only monoamine neurotransmitter involved in the coding of risk. Long et al. (2009) manipulated the diet of rhesus macaques to rapidly deplete their tryptophan levels and thereby systemically lower serotonin levels. This manipulation made monkeys more risk seeking. In particular, they tended to choose risky options more often (the reward magnitude of the safe option had to be increased by 60% in order to achieve indifference) compared to control conditions with normal serotonin levels. In risk-free choices, reward magnitude discrimination remained unchanged. Thus, serotonin appears to specifically reduce the subjective value of risk.
Using a formal definition of risk, coefficient of variation, McCoy and Platt (2005) recorded from the posterior cingulate cortex of monkeys during a visual gambling task. The task involved making a choice between two targets, with one yielding a fixed reward (juice delivered for 150 ms) and the other yielding a risky reward (chance delivery of juice for more than or less than 150 ms, with a mean time of 150 ms). The variance of the risky target’s juice delivery was increased to manipulate risk (i.e., the most risky target would deliver juice for 50 or 250 ms, whereas the least risky target delivered juice for 140 or 160 ms). In contrast to the majority of human studies using such a paradigm, it was found that monkeys significantly preferred risky options to safe options, and that this behavioral preference actually increased with risk. Moreover, the preference could not be explained by novelty. Posterior cingulate neurons increased their firing rates when monkeys chose a risky option, especially for choices when the target was in the neuron’s receptive field (Figure 3A). Interestingly, a number of these cells showed increased firing rates preceding risky choices even during fixation periods, suggesting a role for the posterior cingulate in biasing eye movements to options with higher subjective value. This information may be subsequently passed on to posterior parietal cortex where evidence of the coding of relative subjective value of eye movements has been shown (Dorris and Glimcher, 2004; Sugrue et al., 2004).
Figure 3. Reward variance coding in posterior cingulate and orbitofrontal cortex. (A) McCoy and Platt (2005) recorded from the posterior cingulate cortex during a risky choice task. Neurons in this area were modulated by the reward variance (CV, coefficient of variation) of options inside and outside their respective receptive fields at various stages of the task, but the greatest modulation was observed at 200–400 ms after saccade onset. (B) O’Neill and Schultz (2010) found risk-related activity at various stages of the task in orbitofrontal neurons. OFC neurons code reward variance at short latencies after cue onset (∼100 ms) and continue to code variance even after the reward is delivered, and risk is resolved. The latencies of OFC risk coding neurons (faster than dopaminergic risk signals and the risk responses in the posterior cingulate and comparable to the latency of midbrain and basal ganglia reward probability signals) suggests the OFC may provide risk information to higher cortical regions in preparation for action selection. All figures reprinted with permission.
Risk as dispersion and reward value responses were investigated in detail with single-unit recordings in the OFC by O’Neill and Schultz (2010). In this experiment, monkeys learned to associate different visual stimuli with three binary equiprobable outcome distributions that differed in reward variance. Providing the animal made a correct response, the stimulus associated with high risk reward distributions was followed by either 0.18 or 0.42 ml of juice. By contrast the low risk stimulus was followed by 0.27 or 0.33 ml of juice, and an intermediate risk stimulus was followed by 0.24 or 0.36 ml. Note that the expected value of these reward distributions was equal (0.3 ml). In addition to these risky distributions, they also tested the responses of orbitofrontal neurons to rewards that varied in magnitude but not risk.
When given a choice, the animals preferred increasingly risky options over safe options with the same expected value and responded more quickly to risk-predicting stimuli, suggesting that monkeys were risk seeking in this situation. In areas 11, 12, 13, and 14, 109 orbitofrontal neurons showed activity that increased or decreased with risk (both reward variance and SD) at various stages of the task, most prevalently at cue presentation and during reward delivery (Figure 3B). Most of these cells coded risk at one task epoch, but some coded risk at 2 or more task epochs. Because monkeys were risk seeking in this experiment, a monotonic increase in activity to increasing risk could also indicate a value response. The separate manipulations of value and risk used by O’Neill and Schultz (2010) allowed them to demonstrate the presence of both distinct and combined value and risk signals.
Yet, risk attitude appears to modulate responses of OFC neurons to risk as dispersion, particularly in situations of choice. Roitman and Roitman (2010) recorded from OFC neurons in rats. The animals performed in forced choice and free choice conditions. In free choice sessions, they chose freely between a risky (zero or four pellets, equiprobable) and a safe lever (two pellets for sure). In forced choice sessions, only one lever was available. Risk attitudes as measured in free choice situations were stable across days but differed across animals. In the majority of test sessions the animals were risk seeking (26 out of 42 sessions; 14 animals, each tested in 3 sessions), some were risk neutral (13 out of 42), and only few risk averse (3 out of 42). The activity of OFC neurons decreased or increased after the time of the outcome. These changes were not modulated by risk attitude in forced choice sessions but differed according to risk attitude in free choice sessions. In risk seeking (but not in risk neutral) animals, activation changes to the safe outcome were similar to those induced by the zero outcome of the risky option. Thus, a preference for risk coincided with more pronounced responses to the larger outcome of a risky option in choice situations.
A sizeable number of the neurons in the two studies (O’Neill and Schultz, 2010; Roitman and Roitman, 2010) continued to code risk even after the outcome was delivered to the animal, which is notable because the risk at this time point is zero. O’Neill and Schultz (2010) speculate that these risk signals after the outcome may represent an unsigned reward prediction error that could drive attention. Such a signal has recently been reported in the ACC of monkeys that receive outcomes following ambiguous gambles when reward probabilities are unknown or indiscernible to the animal (Hayden et al., 2011).
Kepecs et al. (2008) extended the work on reward uncertainty by investigating the role of subjective decision uncertainty during choice. In their task, rats were trained to enter a port and sample an odor, which contained information as to whether a reward would be delivered in an outcome port to the left or right of the odor port. The sampled odor was a binary mixture of two separate odorants (caproic acid and 1-hexanol), each of which was associated with either the left or the right side. The proportion of each odorant in the sample was altered (caproic acid: 1-hexanol ratios of 100:0, 68:32, 56:44, 44:56, 32:68, and 0:100%) in order to make it more or less difficult for the rat to decide which outcome port to visit. After the decision, the rats were required to wait for between 0.3 and 1 s before receiving a drop of water if their choice was correct. During this reward anticipation period, Kepecs et al. (2008) analyzed the activity of neuronal units in the lateral OFC. A large number of OFC neurons increased their firing rate with stimulus difficulty, with a smaller proportion showing the inverse encoding pattern. Although this pattern of firing is consistent with the dopaminergic risk signal, the neurons differed in their responses if the rats made correct or incorrect choices, suggesting that the OFC codes decision uncertainty calculated relative to the variance of perceptual information in a single trial, rather than reward risk, which can only be calculated after sampling outcomes over many trials. However, Kepecs et al. (2008) conclude that the decision uncertainty experienced by rats in their task covaries with reward probability and uncertainty (since the probabilities were only manipulated in the range of p = 0.5 to p = 1). Although the OFC is densely innervated by afferent fibers from dopaminergic midbrain, it remains to be seen if the OFC decision uncertainty signal is related to dopaminergic reward risk or probability signals. One speculative idea is that the OFC signal is driven by upstream neurons that maximally fire with coincident input from dopaminergic and lateral habenula neurons. Since these cells have been demonstrated to reliably respond in an opposite fashion to reward probability, neurons that summate over the output of both would be more likely to fire to cues predicting rewards at maximal risk.
The studies described in this review all demonstrate that behaviorally relevant reward parameters such as probability and variance are encoded at the neuronal level and in a distributed fashion. Many of the implicated regions are directly connected, suggesting that a network contributes to the processing of probability and risk. Measuring firing activity from single neurons requires the use of single or multiple microelectrodes to detect discharges. Together with well-controlled behavioral paradigms this technique allows us to correlate neuronal activity with behavior at extremely high temporal resolution. However, due to restricted sampling, electrophysiological recordings are somewhat difficult to interpret on a larger scale. The technique usually targets very small volumes of brain tissue and limited numbers of neurons, and online searching for neurons showing task-related activity may undermine the ability to define specific roles of distinct brain regions or nuclei. There also remains the possibility that reward uncertainty signals are coded in a distributed fashion across networks of neurons, which would be difficult to ascertain in behaving animals using current techniques.
Many of the questions raised by single-unit recordings in reward uncertainty paradigms are beginning to be addressed by researchers. There are however many exceptions and gaps in our understanding, providing many opportunities for further research. Future research may wish to address whether higher-order risk terms and ambiguity are processed in single neurons and the degree to which reward uncertainty signals are processed in a subjective or objective manner. The temporal development of risk signals in the brain remains a complex issue (Table 1), especially with respect to where stimulus identity is decoded and the relevant reward parameters passed onto regions generating appropriate behavioral output. One potential candidate as the source of reward probability and risk signals is the amygdala (Herry et al., 2007), which has been shown to distinguish the valence of conditioned stimuli at latencies as short as 20–30 ms (Quirk et al., 1995). At early stages of processing, reward uncertainty signals appear to be coded separately from other information, consistent with economic theories suggesting that the statistical parameters of reward distributions are detected and represented separately in a mean–variance approach to expected reward processing (Boorman and Sallet, 2009). At later stages the signals are multiplexed with other reward signals and often combine sensory and motor preparatory information.
Table 1. Example latencies (where available) of single units measured in experiments manipulating reward probability and variance.
One problem of comparing the current findings relates to the differences in the behavioral tasks used in different studies. For example, the pathways responsible for passing reward uncertainty signals to output structures may differ depending on the sensory modality of stimuli or whether the task involves Pavlovian or instrumental conditioning. This may particularly apply to striatal neurons that code reward-related information dependent on whether or not an action is required or in choice versus no choice situations (Hassani et al., 2001; Kawagoe et al., 1998; Lau and Glimcher, 2008). The network propagation of these signals could be further elucidated by employing at least three techniques. Firstly, simultaneous recording of (anatomically well defined) pre- and postsynaptic structures would potentially allow researchers to identify the flow of reward uncertainty information. Stimulation of one or more brain regions while simultaneously recording from another could also further enhance our understanding of information flow. Finally, a technique that allows the selective excitation or suppression of distinct classes of neurons within an area would potentially offer researchers a very powerful tool to assess the informational flow of reward uncertainty information. Optogenetics is one such method that was recently used to modulate dopaminergic activity in a reward-based paradigm in the mouse (Tsai et al., 2009).
Understanding the likelihood of a future reward or predicting variability in the quality of potential rewards seems to be just as important as predicting reward magnitudes to animals. The effects of uncertainty are well known to affect the foraging behavior of many species so it is perhaps not surprising that these higher-order reward parameters are coded in large numbers of cells throughout the brain. Additionally, the fact that reward uncertainty is coded in the basal ganglia and midbrain, structures that are largely conserved throughout the vertebrates, supports the adaptive importance of such signals.
The authors declare that the research was conducted in the absence of any commercial or financial relationships that could be construed as a potential conflict of interest.
We thank Mati Joshua and Paul Apicella for helpful discussions. This work was supported with funding from Swiss National Centers of Competence in Research (NCCR) and the Swiss National Science Foundation (PP00P1_128574).
Amiez, C., Joseph, J. P., and Procyk, E. (2006). Reward encoding in the monkey anterior cingulate cortex. Cereb. Cortex 16, 1040–1055.
Apicella, P., Deffains, M., Ravel, S., and Legallet, E. (2009). Tonically active neurons in the striatum differentiate between reward delivery and omission of expected reward in a probabilistic task context. Eur. J. Neurosci. 30, 515–526.
Apicella, P., Ravel, S., Deffains, M., and Legallet, E. (2011). The role of striatal tonically active neurons in reward prediction error signaling during instrumental task performance. J. Neurosci. 31, 1507–1515.
Arkadir, D., Morris, G., Vaadia, E., and Bergman, H. (2004). Independent coding of movement direction and reward prediction by single pallidal neurons. J. Neurosci. 24, 10047–10056.
Barbas, H., and De Olmos, J. (1990). Projections from the amygdala to basoventral and mediodorsal prefrontal regions in the rhesus monkey. J. Comp. Neurol. 300, 549–571.
Belova, M. A., Paton, J. J., Morrison, S. E., and Salzman, C. D. (2007). Expectation modulates neural responses to pleasant and aversive stimuli in primate amygdala. Neuron 55, 970–984.
Bermudez, M. A., and Schultz, W. (2010a). Responses of amygdala neurons to positive reward predicting stimuli depend on background reward (contingency) rather than stimulus-reward pairing (contiguity). J. Neurophysiol. 103, 1158–1170.
Bermudez, M. A., and Schultz, W. (2010b). Reward magnitude coding in primate amygdala neurons. J. Neurophysiol. 104, 3424–3432.
Boorman, E. D., and Sallet, J. (2009). Mean-variance or prospect theory? The nature of value representations in the human brain. J. Neurosci. 29, 1876–1879.
Braun, D. A., Nagengast, A. J., and Wolpert, D. M. (2011). Risk-sensitivity in sensorimotor control. Front. Hum. Neurosci. 5:1. doi:10.3389/fnhum.2011.00001
Bromberg-Martin, E. S., Matsumoto, M., Hong, S., and Hikosaka, O. (2010). A pallidus-habenula-dopamine pathway signals inferred stimulus values. J. Neurophysiol. 104, 1068–1076.
Burke, C. J., and Tobler, P. N. (2011). Reward skewness coding in the insula independent of probability and loss. J. Neurophysiol. doi:10.1152/jn.00471.2011 [Epub ahead of print].
Cavada, C., Company, T., Tejedor, J., Cruz Rizzolo R, J., and Reinoso Suarez, F. (2000). The anatomical connections of the macaque monkey orbitofrontal cortex. A review. Cereb. Cortex 10, 220–242.
Dorris, M. C., and Glimcher, P. W. (2004). Activity in posterior parietal cortex is correlated with the subjective desireability of an action. Neuron 44, 365–378.
Fiorillo, C. D., Tobler, P. N., and Schultz, W. (2003). Discrete coding of reward probability and uncertainty by dopamine neurons. Science 299, 1898–1902.
Goldberg, M. E., Colby, C. L., and Duhamel, J. R. (1990). Representation of visuomotor space in the parietal lobe of the monkey. Cold Spring Harb. Symp. Quant. Biol. 55, 729–739.
Haber, S. N. (2003). The primate basal ganglia: parallel and integrative networks. J. Chem. Neuroanat. 26, 317–330.
Hassani, O. K., Cromwell, H. C., and Schultz, W. (2001). Influence of expectation of different rewards on behavior-related neuronal activity in the striatum. J. Neurophysiol. 85, 2477–2489.
Hayden, B. Y., Heilbronner, S. R., Pearson, J. M., and Platt, M. L. (2011). Surprise signals in anterior cingulate cortex: neuronal encoding of unsigned reward prediction errors driving adjustment in behavior. J. Neurosci. 31, 4178–4187.
Herrnstein, R. J., and Vaughn, W. J. (1980). “The allocation of individual behavior,” in Limits to Action ed. J. E. R. Staddon (New York: Academic), 143–176.
Herry, C., Bach, D. R., Esposito, F., Di Salle, F., Perrig, W. J., Scheffler, K., Lüthi, A., and Seifritz, E. (2007). Processing of temporal unpredictability in human and animal amygdala. J. Neurosci. 27, 5958–5966.
Hong, S., and Hikosaka, O. (2008). The globus pallidus sends reward-related signals to the lateral habenula. Neuron 60, 720–729.
Hong, S., Jhou, T. C., Smith, M., Saleem, K. S., and Hikosaka, O. (2011). Negative reward signals from the lateral habenula to dopamine neurons are mediated by rostromedial tegmental nucleus in primates. J. Neurosci. 31, 11457–11471.
Jhou, T. C., Fields, H. L., Baxter, M. G., Saper, C. B., and Holland, P. C. (2009). The rostromedial tegmental nucleus (RMTg), a GABAergic afferent to midbrain dopamine neurons, encodes aversive stimuli and inhibits motor responses. Neuron 61, 786–800.
Joshua, M., Adler, A., Rosin, B., Vaadia, E., and Bergman, H. (2009). Encoding of probabilistic rewarding and aversive events by pallidal and nigral neurons. J. Neurophysiol. 101, 758–772.
Kawagoe, R., Takikawa, Y., and Hikosaka, O. (1998). Expectation of reward modulates cognitive signals in the basal ganglia. Nat. Neurosci. 1, 411–416.
Kennerley, S. W., Dahmubed, A. F., Lara, A. H., and Wallis, J. D. (2009). Neurons in the frontal lobe encode the value of multiple decision variables. J. Cogn. Neurosci. 21, 1162–1178.
Kepecs, A., Uchida, N., Zariwala, H., and Mainen, Z. (2008). Neural correlates, computation and behavioral impact of decision confidence. Nature 455, 227–231.
Lau, B., and Glimcher, P. W. (2008). Value representations in the primate striatum during matching behavior. Neuron 58, 451–463.
Long, A. B., Kuhn, C. M., and Platt, M. L. (2009). Serotonin shapes risky decision making in monkeys. Soc. Cogn. Affect. Neurosci. 4, 346–356.
Matsumoto, M., and Hikosaka, O. (2009). Representation of negative motivational value in the primate lateral habenula. Nat. Neurosci. 12, 77–84.
McCoy, A. N., and Platt, M. L. (2005). Risk-sensitive neurons in macaque posterior cingulate cortex. Nat. Neurosci. 8, 1220–1227.
Morris, G., Arkadir, D., Nevet, A., Vaadia, E., and Bergman, H. (2004). Coincident but distinct messages of midbrain dopamine and striatal tonically active neurons. Neuron 43, 133–143.
Musallam, S., Corneil, B. D., Greger, B., Scherberger, H., and Andersen, R. A. (2004). Cognitive control signals for neural prosthetics. Science 305, 258–262.
O’Neill, M., and Schultz, W. (2010). Coding of reward risk by orbitofrontal neurons is mostly distinct from coding of reward value. Neuron 68, 789–800.
Oyama, K., Hernádi, I., Iijima, T., and Tsutsui, K. (2010). Reward prediction error coding in dorsal striatal neurons. J. Neurosci. 30, 11447–11457.
Platt, M. L., and Glimcher, P. W. (1999). Neural correlates of decision variables in parietal cortex. Nature 400, 233–238.
Quirk, G. J., Repa, C., and LeDoux, J. E. (1995). Fear conditioning enhances short-latency auditory responses of lateral amygdala neurons: parallel recordings in the freely behaving rat. Neuron 15, 1029–1039.
Real, L., and Caraco, T. (1986). Risk and foraging in stochastic environments. Annu. Rev. Ecol. Syst. 17, 371–390.
Rescorla, R. A., and Wagner, A. R. (1972). “A theory of Pavlovian conditioning: variations in the effectiveness of reinforcement and nonreinforcement,” in Classical Conditioning II: Current Research and Theory, eds A. H. Black, and W. F. Prokasy (New York: Appleton-Century-Crofts), 64–99.
Roitman, J. D., and Roitman, M. F. (2010). Risk-preference differentiates orbitofrontal cortex responses to freely chosen reward outcomes. Eur. J. Neurosci. 31, 1492–1500.
Rosati, A. G., and Hare, B. (2011). Chimpanzees and bonobos distinguish between risk and ambiguity. Biol. Lett. 7, 15–18.
Schultz, W. (2010). Dopamine signals for reward value and risk: basic and recent data. Behav. Brain Funct. 6, 24.
Schultz, W., Dayan, P., and Montague, P. R. (1997). A neural substrate of prediction and reward. Science 275, 1593–1599.
Snyder, L. H., Battista, A. P., and Andersen, R. A. (1997). Coding of intention in the posterior parietal cortex. Nature 386, 167–170.
Stephens, D. W., and Krebs, J. R. (1986). Foraging Theory. Princeton, NJ: Princeton University Press.
Sugrue, L. P., Corrado, G. S., and Newsome, W. T. (2004). Matching behavior and the representation of value in the parietal cortex. Science 304, 1782–1787.
Tobler, P. N., Christopoulos, G. I., O’Doherty, J. P., Dolan, R. J., and Schultz, W. (2008). Neuronal distortions of reward probability without choice. J. Neurosci. 28, 11703–11711.
Tsai, H. C., Zhang, F., Adamantidis, A., Stuber, G. D., Bonci, A., de Lecea, L., and Deisseroth, K. (2009). Phasic firing in dopaminergic neurons is sufficient for behavioral conditioning. Science 324, 1080–1084.
van Duuren, E., van der Plasse, G., Lankelma, J., Joosten, R. N., Feenstra, M. G., and Pennartz, C. M. (2009). Single-cell and population coding of expected reward probability in the orbitofrontal cortex of the rat. J. Neurosci. 29, 8965–8976.
Keywords: uncertainty, dopamine, basal ganglia, orbitofrontal cortex, neuroeconomics
Citation: Burke CJ and Tobler PN (2011) Coding of reward probability and risk by single neurons in animals. Front. Neurosci. 5:121. doi: 10.3389/fnins.2011.00121
Received: 29 April 2011; Paper pending published: 18 July 2011;
Accepted: 16 September 2011; Published online: 11 October 2011.
Edited by:
Marijn Van Wingerden, Heinrich-Heine University Duesseldorf, GermanyReviewed by:
Adam Kepecs, Cold Spring Harbor Laboratory, USACopyright: © 2011 Burke and Tobler. This is an open-access article subject to a non-exclusive license between the authors and Frontiers Media SA, which permits use, distribution and reproduction in other forums, provided the original authors and source are credited and other Frontiers conditions are complied with.
*Correspondence: Christopher J. Burke, Laboratory for Social and Neural Systems Research, Department of Economics, University of Zurich, Blümlisalpstrasse 10, 8006 Zürich, Switzerland. e-mail: christopher.burke@econ. uzh.ch
Disclaimer: All claims expressed in this article are solely those of the authors and do not necessarily represent those of their affiliated organizations, or those of the publisher, the editors and the reviewers. Any product that may be evaluated in this article or claim that may be made by its manufacturer is not guaranteed or endorsed by the publisher.
Research integrity at Frontiers
Learn more about the work of our research integrity team to safeguard the quality of each article we publish.